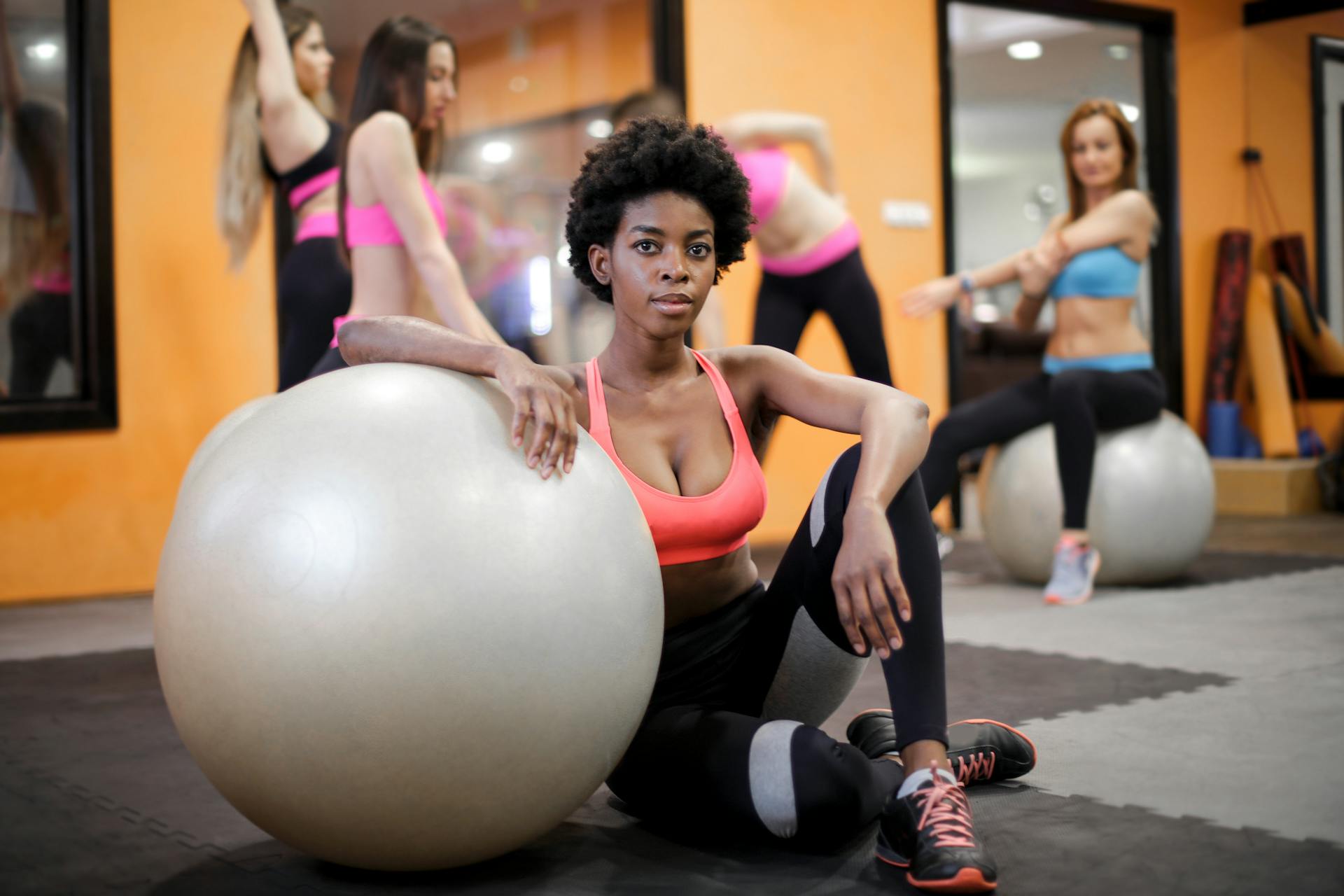
In AI accountability essential training, it's essential to understand the concept of explainability. This means being able to provide clear and transparent information about how an AI model makes its decisions.
AI models can be complex and difficult to understand, but explainability is crucial for building trust with users.
Explainability can be achieved through various techniques, such as feature attribution and model interpretability.
These techniques help to identify which inputs or features are most influential in a model's decision-making process.
Understanding how AI models make decisions is crucial for responsible AI development and deployment.
It's also essential to consider the potential biases and errors in AI models, which can have significant consequences.
Bias in AI models can be caused by various factors, including data quality issues, algorithmic flaws, and cultural or social biases.
Take a look at this: Ai Models Training
Accountability and Ethics
Accountability and ethics are crucial aspects of AI development and deployment. Organizations must be accountable for how their AI systems operate, and industry standards should be used to develop accountability norms.
Accountability in AI systems can be ensured through the use of Machine Learning Operations (MLOps) capabilities, such as registering, packaging, and deploying models, capturing governance data, and notifying and alerting on events in the machine learning lifecycle.
The Responsible AI scorecard in Azure Machine Learning creates accountability by enabling cross-stakeholder communications and empowering developers to configure, download, and share their model health insights.
The UNESCO Recommendation on the Ethics of Artificial Intelligence emphasizes the importance of transparency and fairness in AI systems, and policymakers can translate the core values and principles into action through policy action areas.
The Business Council for Ethics of AI is a collaborative initiative between UNESCO and companies operating in Latin America that promotes ethical practices within the AI industry.
Establishing clear lines of responsibility and accountability is essential to address the ethical concerns surrounding AI systems. Legal frameworks and regulations should be developed to define liability and ensure that developers and organizations take appropriate measures to prevent harm caused by AI systems.
To address fairness and bias in AI, diverse and representative datasets, rigorous testing, and the development of algorithms that mitigate bias are necessary. Ongoing monitoring and adjustment are also required to ensure that biases do not creep into the system over time.
Here are some key policy areas for responsible AI development:
- Data governance
- Environment and ecosystems
- Gender
- Education and research
- Health and social wellbeing
These policy areas are essential for ensuring that AI is developed and utilized in a manner that respects human rights and upholds ethical standards.
Reliability and Safety
Reliability and safety are critical components of AI accountability. AI systems should operate reliably, safely, and consistently, responding safely to unanticipated conditions and resisting harmful manipulation.
To achieve this, developers can use tools like the error analysis component of the Responsible AI dashboard in Azure Machine Learning. This tool enables data scientists and developers to get a deep understanding of how failure is distributed for a model.
Data scientists and developers can use this tool to identify cohorts of data with a higher error rate than the overall benchmark. These discrepancies might occur when the system or model underperforms for specific demographic groups or for infrequently observed input conditions in the training data.
By using this tool, developers can gain valuable insights into their AI systems and make necessary adjustments to improve reliability and safety.
Take a look at this: Ai Running Out of Training Data
Governance: Principles and Strategies
Governance is a crucial aspect of AI accountability. Transparency is a key principle in AI governance, and it's essential to understand how AI systems make decisions.
Improving interpretability requires stakeholders to comprehend how and why AI systems function the way they do. This can help identify potential performance issues, fairness issues, exclusionary practices, or unintended outcomes.
To achieve transparency, Azure Machine Learning provides a Responsible AI dashboard with model interpretability and counterfactual what-if components. These components enable data scientists and developers to generate human-understandable descriptions of the predictions of a model.
The model interpretability component provides multiple views into a model's behavior, including global explanations, local explanations, and model explanations for a selected cohort of data points.
A Responsible AI scorecard is also available in Azure Machine Learning, which is a customizable PDF report that developers can use to educate their stakeholders about their datasets and models' health, achieve compliance, and build trust.
Transparency and explainability are essential in AI governance, as AI models often operate as "black boxes" making it challenging to understand how they arrive at their decisions. Researchers are working on developing more interpretable AI models and creating methods for explaining AI decisions.
Here are some key principles and strategies for AI governance:
- Transparency: Understand how AI systems make decisions
- Interpretability: Comprehend how and why AI systems function the way they do
- Data governance: Understanding the relationship between AI and data is critical
- Education: Begin with education to ensure effective AI usage
- Principles: Mitigate AI risk, align AI with business objectives, and guide learners with best practices
Privacy and Security
Privacy and security are crucial aspects of AI accountability. As AI becomes more prevalent, protecting personal and business information is essential.
Access to data is necessary for AI systems to make accurate predictions and decisions, but this also raises concerns about privacy and data security. AI systems must comply with privacy laws that require transparency about data collection, use, and storage.
Azure Machine Learning enables administrators and developers to create a secure configuration that meets their companies' policies. With Azure Machine Learning, users can restrict access to resources and operations by user account or group.
Restricting incoming and outgoing network communications is also a best practice. This can be achieved by implementing encryption for data in transit and at rest. Regular scans for vulnerabilities are also necessary to identify and address potential security threats.
Microsoft has developed two open-source packages to help implement privacy and security principles: SmartNoise and Counterfit. SmartNoise provides components for building differentially private systems, while Counterfit simulates cyberattacks against AI systems to assess their security.
A fresh viewpoint: Ethics in Ai and Machine Learning
To maintain the accuracy of their data, businesses use machine learning, data analysis, and AI tools. This is essential for complying with regulations like the General Data Protection Regulation (GDPR) and the California Consumer Privacy Act (CCPA).
Here are some key security measures for AI systems:
- Restrict access to resources and operations by user account or group
- Restrict incoming and outgoing network communications
- Encrypt data in transit and at rest
- Scan for vulnerabilities
- Apply and audit configuration policies
Audit
Achieving AI accountability requires specialized training, and AI audit training is essential for mastering this critical skill. This type of training enables IT auditors to achieve the highest level of specialization and mastery for auditing AI technologies.
To audit generative AI, IT auditors need to understand the importance of AI strategy, explore use cases, and address challenges associated with auditing GenAI. This involves a thorough analysis of AI strategy and its implications on the organization.
The Artificial Intelligence Audit Toolkit provides IT auditors with assessment guidance that supports building and demonstrating assurance around the effectiveness of controls supporting this critical area of emerging technology. This toolkit offers a library of AI controls derived from select control frameworks and law.
As AI becomes more integral in enterprise product and service delivery, it will come under more audit scrutiny. This is why having a solid understanding of AI audit principles is crucial for IT auditors.
Effective AI governance is critical for mitigating AI risk, aligning AI with business objectives, and guiding learners with best practices for enterprise AI governance. This involves exploring the importance of AI governance, strategies, and business alignment.
ISACA's recent AI Pulse Poll shows that 85% of digital trust professionals expect to need more AI training within two years to retain their roles or advance their careers. This highlights the need for formal AI policies and responsible AI deployment practices.
Artificial intelligence is having a large impact on the risk landscape in the financial sector, underscoring the need for AI governance frameworks and thorough AI risk assessments. This requires a strategic approach to AI and risk management.
Business and Economic Impact
The widespread adoption of AI and automation technologies has the potential to displace jobs in various industries. This can lead to job loss and economic disruption for certain groups.
To mitigate this impact, investing in retraining and upskilling programs for affected workers is essential. This will enable workers to adapt to the changing job market and acquire new skills to remain employable.
The benefits of AI should be distributed equitably to minimize economic disruption. This can be achieved by developing policies that promote job transition and ensure that the benefits of AI are shared fairly among all stakeholders.
Job Displacement and Economic Impact
The widespread adoption of AI and automation technologies has the potential to displace jobs in various industries. While AI can create new opportunities and increase productivity, it can also lead to job loss and economic disruption for certain groups.
Investing in retraining and upskilling programs for affected workers is crucial to mitigate the negative impact of job displacement. This can help workers adapt to new technologies and find new employment opportunities.
The benefits of AI should be distributed equitably, ensuring that everyone has access to the opportunities created by these technologies. This requires policymakers to develop policies that promote job transition and support affected workers.
Addressing the societal impact of automation requires careful consideration of the economic impact of AI and ML technologies. By prioritizing fairness, transparency, accountability, and privacy, we can minimize harm and maximize the benefits of these technologies.
Machine Learning for Business
Machine Learning for Business can be a game-changer for companies.
This is because Machine Learning for Business Enablement courses, like the one mentioned, empower learners to evaluate machine learning solutions and assess risk more effectively.
The course includes two performance labs for hands-on, practical experience.
It's highly recommended to take Machine Learning for Business Enablement prior to Machine Learning: Neural Networks, Deep Learning and Large Language Models.
This order makes sense because Machine Learning for Business Enablement lays the groundwork for more advanced topics in machine learning.
You might like: Ai and Machine Learning Training
Education and Career Advancement
Education and Career Advancement is crucial for professionals looking to stay ahead in the field of AI.
ISACA training courses, such as the one on AI for auditors, can provide valuable knowledge and skills.
This course explores categories of AI algorithms that auditors will encounter, relevant regulations, and how to audit third-party AI dependencies.
Meghan Maneval, an AI governance expert, suggests that education is a key starting point for more effective AI usage in the enterprise landscape.
Discover more: Generative Ai and Education
Next Steps and Guidance
To ensure AI accountability, it's essential to take the next steps in implementing responsible AI practices. You can start by exploring the Responsible AI dashboard in Azure Machine Learning, which provides valuable insights and guidance.
For more information on implementing Responsible AI, see the Responsible AI dashboard for a comprehensive overview. This dashboard offers a centralized platform to monitor and improve AI systems.
To generate the Responsible AI dashboard, you can use the CLI and SDK or Azure Machine Learning studio UI. This allows you to tailor the dashboard to your specific needs and goals.
For another approach, see: Azure Ai Training
To move beyond high-level principles, focus on practical strategies like the eleven key areas for policy actions outlined in the Recommendation. These areas provide a clear direction for responsible AI development.
Here are some key policy areas to consider:
- Transparency and explainability
- Accountability and governance
- Human rights and dignity
- Security and safety
- Environmental sustainability
- Education and awareness
- Research and development
- International cooperation
- Public engagement and participation
- Monitoring and evaluation
- Standardization and interoperability
Staying ahead of the curve is crucial in AI accountability. Explore curated collections of blogs, infographics, articles, and other resources to gain valuable knowledge and deeper insights into AI.
Frequently Asked Questions
What is accountability mechanism in AI?
Accountability mechanism in AI refers to the system of assigning responsibility for AI-related errors or harm to specific parties. It ensures that those responsible for AI development, deployment, and use can be held accountable for their actions.
What is the artificial intelligence accountability act?
The California Generative AI Accountability Act, also known as Senate Bill 896, is a law that sets guidelines for state agencies to review and regulate AI technologies. This law aims to ensure responsible AI development and use in California.
Sources
- https://learn.microsoft.com/en-us/azure/machine-learning/concept-responsible-ai
- https://www.unesco.org/en/artificial-intelligence/recommendation-ethics
- https://www.intelegain.com/ethical-considerations-in-ai-machine-learning/
- https://www.isaca.org/resources/artificial-intelligence
- https://b2b.worldtrade.org.tw/imgen452/ai-accountability-essential-training-course
Featured Images: pexels.com