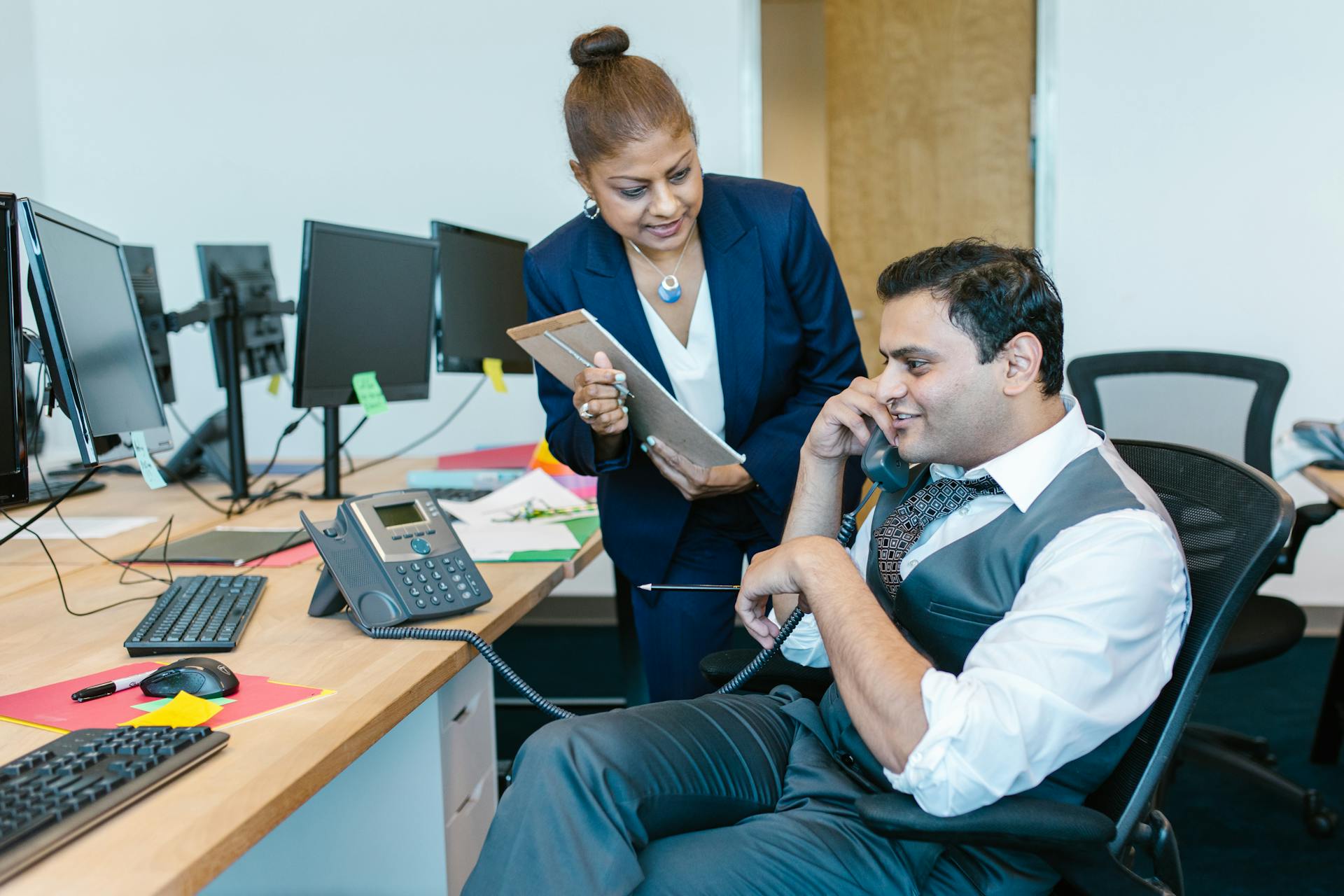
Human in the Loop Automation can be a game-changer for businesses looking to make smarter decisions. By combining the strengths of humans and machines, companies can gain a competitive edge and improve their bottom line.
Businesses can use human in the loop automation to automate routine tasks, freeing up employees to focus on higher-value tasks that require creativity and problem-solving skills. This can lead to increased productivity and efficiency.
According to recent studies, companies that implement human in the loop automation can experience a 20% increase in productivity and a 15% reduction in costs.
Curious to learn more? Check out: Ai in Software Test Automation
Benefits of Human in the Loop Automation
Human in the loop automation offers numerous benefits that can significantly improve business processes. Enhanced accuracy and reliability are just a few of the advantages, thanks to human input and oversight that contribute significantly to the accuracy and reliability of ML models.
Error reduction is another significant benefit, as automation significantly decreases the likelihood of errors that may arise during manual data entry and processing. This results in heightened accuracy in customer data and compliance with regulatory requirements.
You might like: Test Automation for Ai and Ml Code
Human involvement also helps identify and mitigate potential biases in data and algorithms, promoting fairness and equity in ML systems. By incorporating human feedback, machines can learn not only through trial and error, but also through human expertise.
Here are some of the key benefits of human in the loop automation:
- Enhanced accuracy and reliability
- Bias mitigation
- Increased transparency and explainability
- Improved user trust
- Continuous adaptation and improvement
By striking the right balance between AI-powered automation and human intervention, businesses can achieve a more effective onboarding process, where human agents focus on high-value activities that demand their expertise.
Benefits of Automation
Automation can significantly accelerate the onboarding process by automating tasks that require human input. This allows human agents to focus on high-value activities that demand their expertise.
Human error is a major concern in manual data entry and processing, but automation can decrease the likelihood of errors. This results in heightened accuracy in customer data and compliance with regulatory requirements.
Quicker onboarding translates to customers being able to apply your products or services promptly, resulting in increased satisfaction.
A different take: Mlops Continuous Delivery and Automation Pipelines in Machine Learning
Benefits of AI Development
Human involvement in AI development has numerous benefits, making it a crucial aspect of creating accurate and reliable models. The inclusion of human input and oversight significantly contributes to the accuracy and reliability of ML models.
In the field of autonomous vehicles, human drivers or annotators acting as a safety net provide human feedback that informs AI algorithms and helps refine their decision-making process in complex situations. This is essential in improving vehicle safety.
Human involvement helps identify and mitigate potential biases in data and algorithms, promoting fairness and equity in ML systems. By doing so, it ensures that AI models are transparent and explainable.
In content recommendations, human reviewers provide feedback that ensures recommendations match individual tastes while respecting ethical guidelines. This approach leads to safer, more ethical, and more accurate solutions in many sectors.
The benefits of human-in-the-Loop (HITL) include:
- Enhanced accuracy and reliability
- Bias mitigation
- Increased transparency and explainability
- Improved user trust
- Continuous adaptation and improvement
By automating tasks that require human input, AI development can significantly accelerate the onboarding process, liberating human agents from mundane tasks and allowing them to focus on high-value activities.
Improving AI and Customer Satisfaction
Human input and oversight contribute significantly to the accuracy and reliability of ML models, enhancing their overall performance.
In the context of AI development, the "human in the loop" approach is invaluable. Data Labelers with domain-specific expertise contribute their know-how to effectively categorize and classify data sets, directly influencing the quality of the results.
The "human in the loop" concept finds its true effectiveness in its practical applications across a wide range of fields, including autonomous vehicles, content recommendations, and medicine.
To improve customer satisfaction, humans are not simply passive participants; they intervene to optimize model decisions, actively identifying errors and inconsistencies, rectifying them and adjusting the model's operating parameters.
In the field of content recommendations, platforms use HITL to refine algorithms by taking into account user preferences in conjunction with feedback from human reviewers, ensuring that recommendations match individual tastes while respecting ethical guidelines.
Here are some benefits of human-in-the-Loop (HITL):
- Enhanced accuracy and reliability
- Bias mitigation
- Increased transparency and explainability
- Improved user trust
- Continuous adaptation and improvement
By incorporating human feedback into the model training process, HITL enables machines to learn not only through trial and error, but also through human expertise. This leads to safer, more ethical, and more accurate solutions in many sectors.
How It Works
In human-in-the-loop automation, humans interact with the system in various ways to improve its performance. Humans can provide labels for training data, which is essential for supervised learning. This data can be labeled manually or through the use of tools.
Humans can also evaluate the performance of ML models by providing feedback on the model's predictions. This helps identify areas where the model can be improved. Active learning and reinforcement learning are two methods where humans provide feedback to the model, making it learn and improve more effectively.
Here are some ways humans interact with HITL systems:
- Providing labels for training data
- Evaluating the performance of ML models
- Providing feedback to ML models
- Active learning
- Reinforcement learning
How It Works
Human-in-the-loop systems, like those used in document processing, rely on humans to interact with the system in various ways. This can include providing labels for training data, which is essential for supervised learning.
Humans can also evaluate the performance of ML models by providing feedback on the model's predictions. This helps identify areas where the model can be improved.
Explore further: Can Generative Ai Replace Humans
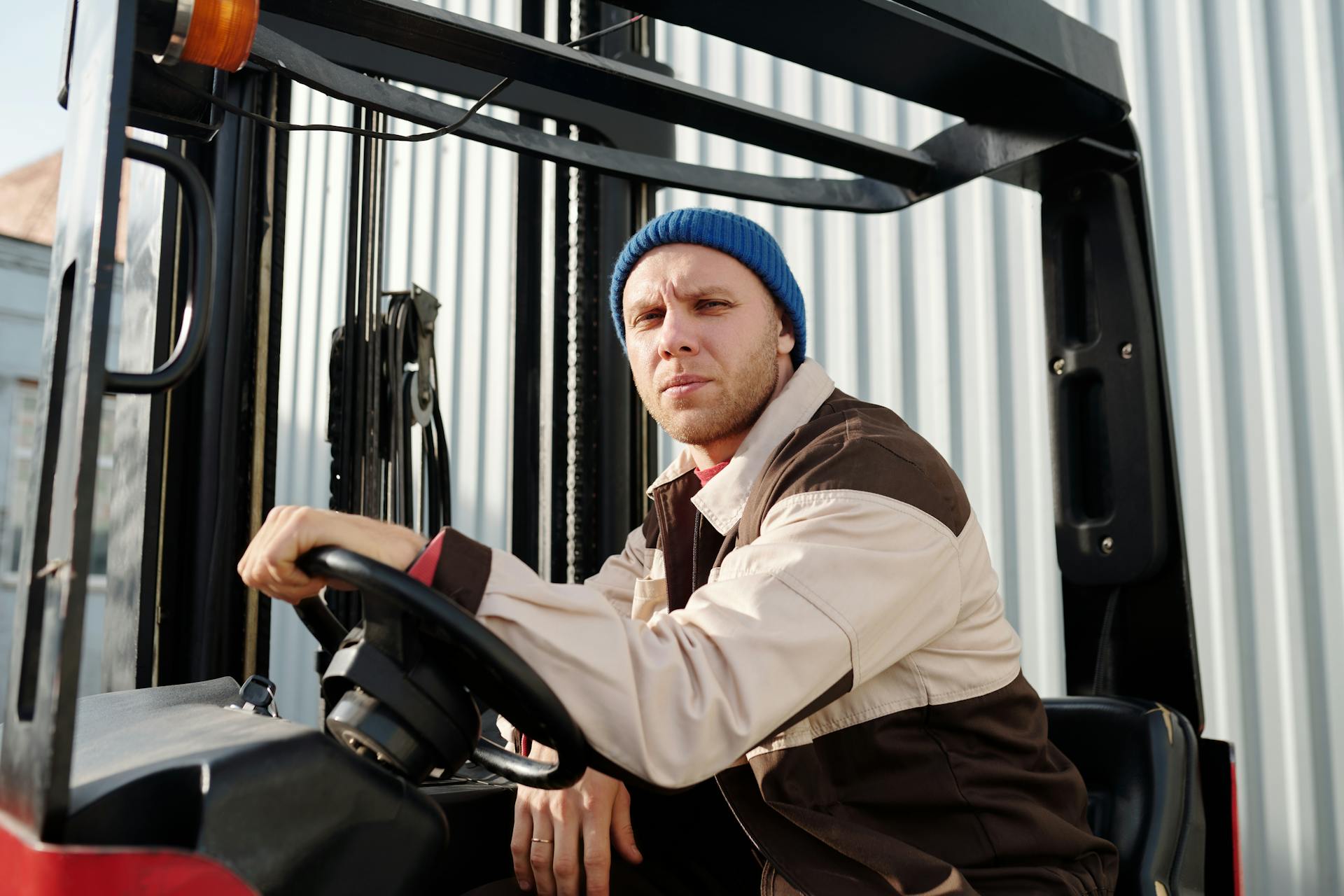
In active learning, the ML model selects the data it wants to be labeled by a human, which can improve the efficiency of the labeling process. Reinforcement learning, on the other hand, uses trial and error to learn, and humans can provide feedback to the model on its actions.
The automation journey begins with using UiPath Document Understanding capabilities, which extract data from unstructured documents using AI and machine learning. This reduces manual data entry and the risk of errors.
Here are the ways humans interact with HITL systems:
- Providing labels for training data
- Evaluating the performance of ML models
- Providing feedback to ML models through active learning or reinforcement learning
In document processing, the robot may encounter errors or low-confidence fields, which are addressed through an Action Center validation step. If the robot is unsure about a particular field or encounters an error, it creates a task in Action Center for a human operator to review and validate the extracted data.
Data Annotation
Data annotation is a crucial step in transforming raw information into resources that can be exploited by ML models. It involves adding labels or annotations to data to help machine learning models understand its meaning.
Check this out: Automated Data Labeling
Humans can interact with data annotation systems in various ways, including providing labels for training data, which can be done manually or through the use of tools. This process is essential for supervised learning, where ML models are trained on labeled data.
Active learning is a method where the ML model selects the data that it wants to be labeled by a human, helping to improve the efficiency of the labeling process. Humans can also provide feedback to ML models, which can help them learn and improve.
Data annotation is not just a casual job, but a profession that requires expertise and attention to detail. Humans can provide additional input data, annotations, evaluations, and corrections to improve the performance of machine learning models.
In some cases, humans can also adjust decision trees and algorithms to meet the specific needs of a task. This is particularly useful in fields like speech recognition, facial recognition, natural language processing, and data classification.
Here are some ways humans can interact with data annotation systems:
- Providing labels for training data
- Evaluating the performance of ML models
- Providing feedback to ML models
Improving AI Models
Improving AI models requires a human touch. Humans are not simply passive participants, but actively intervene to optimize model decisions, identify errors and inconsistencies, and rectify them. This constant feedback loop ensures that AI models align with real-world scenarios and requirements.
By incorporating human participation, AI models can capture the subtleties of various tasks and adapt to complex real-life scenarios. In fact, over 50% of organizations have paused their Copilot initiatives due to data quality and governance concerns, highlighting the importance of human oversight.
Human contributions are invaluable in the context of AI development, particularly in data labeling and annotation. Data labelers with domain-specific expertise contribute their know-how to effectively categorize and classify data sets, directly influencing the quality of the results. This is why HITL is used in many fields, such as speech recognition, facial recognition, natural language processing, and data classification.
Here are some key use cases for HITL:
- Image classification: labeling images for training ML models that can classify images
- Natural language processing: labeling text for training ML models that can understand natural language
- Speech recognition: annotating speech data to improve speech recognition models
- Facial recognition: labeling facial data to improve facial recognition models
Learning Models in AI
Humans are not just passive participants in AI development; they actively intervene to optimize model decisions, identify errors, and adjust operating parameters, ensuring AI models align with real-world scenarios.
The "human in the loop" approach is invaluable in AI development, where domain-specific expertise contributes to effectively categorize and classify data sets, directly influencing the quality of results.
In autonomous vehicles, HITL is essential in the research and development process to improve vehicle safety, with human drivers or annotators acting as a safety net, providing human feedback that informs AI algorithms.
Human expertise is also used in content recommendations, where platforms refine algorithms by taking into account user preferences and feedback from human reviewers, ensuring that recommendations match individual tastes while respecting ethical guidelines.
One of the important roles of humans in the HITL process is algorithm tuning, which enables algorithms to evolve and adapt to complex real-life scenarios through an iterative feedback loop.
Incorporating human participation in AI development not only enriches the dataset but also guarantees the accuracy of the learning process, making it essential for AI models to capture the subtleties of various tasks.
Here are some examples of how humans can contribute to improving AI models:
- Image classification: HITL can be used to label images for training ML models that can classify images, such as object detection, facial recognition, and medical imaging.
- Natural language processing: HITL can be used to label text for training ML models that can understand natural language, such as machine translation, sentiment analysis, and spam filtering.
- Data annotation: Human experts can provide additional input data, annotations, evaluations, and corrections to improve the performance of machine learning models.
By continuously evaluating and adjusting algorithms, AI systems can achieve higher levels of performance, making them more accurate and reliable.
Emergency Situations
In emergency situations, human intervention is crucial for effective problem-solving and decision-making. The complexity of information or systems in these situations makes tasks difficult to automate.
Rapid, adaptive responses are necessary in emergency or fast-moving contexts, where dynamic events unfold quickly. Human intervention is essential to navigate these situations effectively.
These situations require the construction of quasi-autonomous automated models that are complemented by permanent human supervisory intervention. This hybrid approach enables more effective problem-solving and decision-making in emergency situations.
Common Challenges
Human in the loop automation can be a complex process, and certain challenges can arise depending on the application domain and project category. Difficult cases in HITL can vary, but some of the most common ones reported by data annotation specialists include cases with ambiguous or unclear data.
Data annotation specialists have reported that cases with ambiguous or unclear data are a common challenge in HITL. This can lead to inconsistent labeling and decreased model accuracy.
In HITL, cases with complex or nuanced decision-making processes can also be problematic. These cases often require human judgment and expertise to resolve.
Data annotation specialists have also reported cases with conflicting or contradictory information as a common challenge in HITL. This can make it difficult for models to learn and make accurate predictions.
Learning and Adaptation
AI models are not static entities, but dynamic systems destined to evolve continuously. They ingest training data enriched by human expertise, adapt and refine their algorithms according to the flow of information received.
The "human in the loop" approach introduces an iterative learning process that continually evaluates and adjusts algorithms. This enables AI systems to achieve higher levels of performance.
Humans actively identify errors and inconsistencies in AI models, rectify them, and adjust the model's operating parameters. This constant feedback loop ensures that AI models align with real-world scenarios and requirements.
Contextual Ambiguity
Contextual ambiguity is a common challenge in machine learning, especially when dealing with natural language processing. It occurs when artificial intelligence has difficulty understanding the different datasets used to form the model.
In such situations, AI requires human validation to fully accomplish a task. For example, in natural language processing, some expressions may have different meanings depending on the context.
Contextual ambiguity can arise in large-scale outsourcing assignments, where data labelers perform tasks with subjective interpretation. This is why it's essential to define an appropriate annotation strategy and clear rules before starting work on larger or smaller volumes of data.
An automated model may struggle to accurately understand the true intention behind a phrase without taking into account the wider context in which it is used. This highlights the importance of human input in overcoming contextual ambiguity.
AI Models: Learning and Adaptation
AI models are not static entities, but dynamic systems destined to evolve continuously. They adapt and refine their algorithms according to the flow of information received.
The "human in the loop" approach introduces an iterative learning process. This involves incorporating human expertise into the training data, allowing AI models to learn and improve over time.
Incorporating human participation into the training process not only enriches the dataset but also guarantees the accuracy of the learning process. This is especially important in scenarios where extensive datasets are lacking.
By continually evaluating and adjusting algorithms, AI systems can achieve higher levels of performance. This iterative feedback loop enables algorithms to evolve and adapt to complex real-life scenarios.
AI models ingest training data enriched by human expertise and adapt according to the flow of information received. This dynamic process allows AI models to learn and improve continuously.
Frequently Asked Questions
What is the human-in-the-loop control theory?
Human-in-the-loop (HITL) refers to a control theory that involves human interaction with a model or system, requiring human input to operate effectively. This concept is used in various fields, including modeling and simulation, and the development of lethal autonomous weapons.
What is an example of human-in-the-loop machine learning?
Human-in-the-loop machine learning involves involving a human in the decision-making process to ensure accuracy, such as when working with rare language data or sensitive healthcare information. This approach helps improve the reliability of machine learning models in complex or niche domains.
What is the human-in-the-loop protocol?
Human-in-the-loop protocol: A learning approach that involves human interaction to identify and correct model shortcomings before real-world testing, ensuring more accurate and reliable results
Sources
- https://shelf.io/blog/human-in-the-loop-generative-ai/
- https://www.uipath.com/community-blog/tutorials/human-in-the-loop-automation0-for-customer-onboarding-using-action-center
- https://en.innovatiana.com/post/human-in-the-loop-and-ai
- https://cloud.google.com/discover/human-in-the-loop
- https://levity.ai/blog/human-in-the-loop
Featured Images: pexels.com