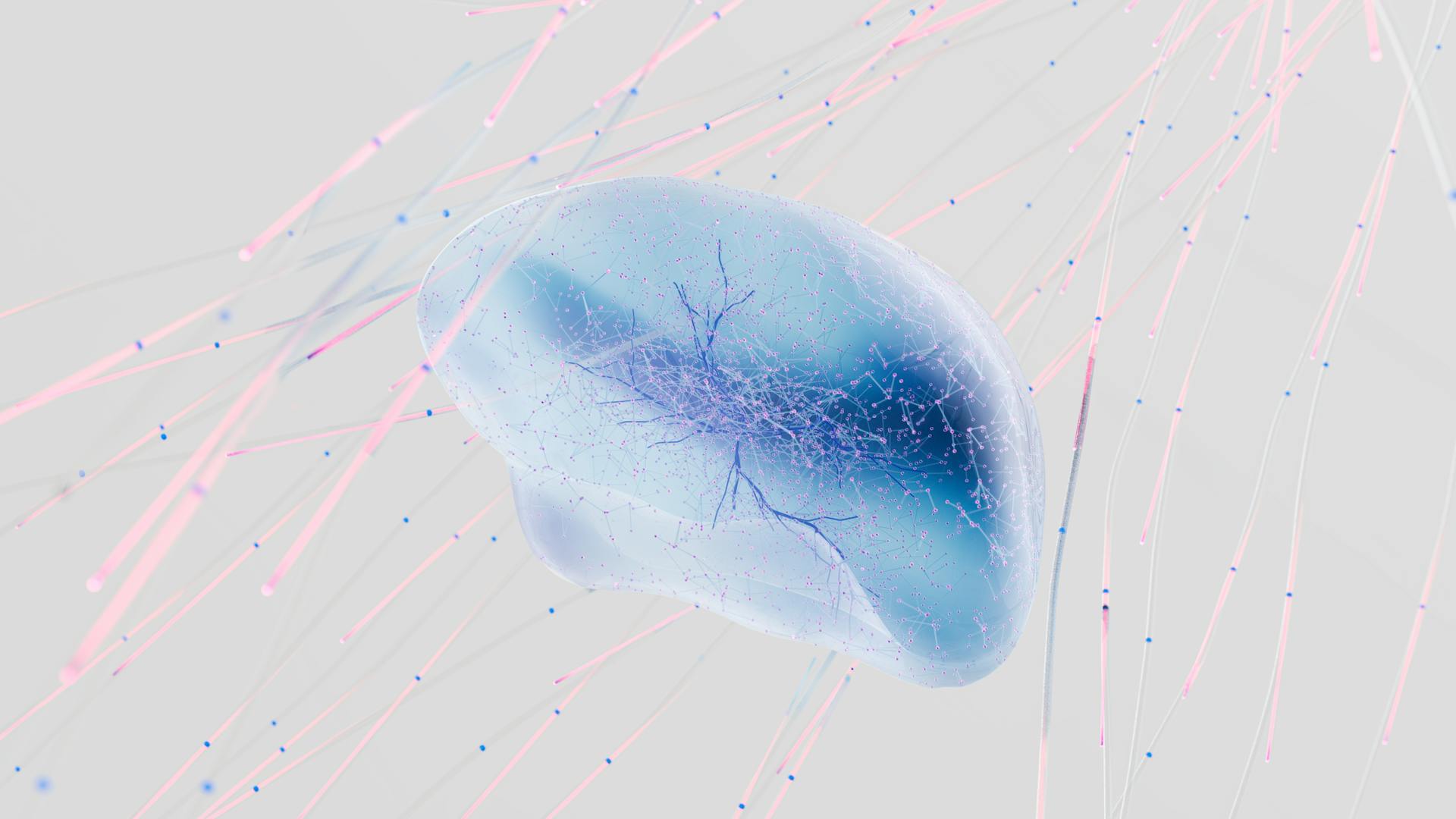
Log linear models are a type of statistical model that's particularly useful for analyzing categorical data. They're especially helpful when you have multiple categorical variables that you want to examine in relation to each other.
Log linear models work by estimating the probability of each possible combination of categories for your variables. This is done through a process called maximum likelihood estimation, which is used to find the best-fitting model.
The key to building a good log linear model is to start with a clear understanding of your data and the research question you're trying to answer. This involves identifying the variables you want to examine and determining the relationships between them.
Related reading: Model Drift vs Data Drift
Fitting Log Linear Models
Fitting Log Linear Models is a crucial step in the process of log linear analysis. It involves determining which interactions are necessary to retain in order to best account for the data.
The process starts with the saturated model, which includes all possible interactions. The highest order interactions are removed one by one, and the likelihood ratio chi-square statistic is computed to measure how well the model fits the data. This is done until the model no longer accurately fits the data.
The likelihood ratio chi-square statistic is used to determine the fit of the model. If the statistic is non-significant, the model fits well, and if it is significant, the model does not fit well.
Here's a summary of the steps involved in fitting a log linear model:
- Start with the saturated model
- Remove the highest order interactions one by one
- Compute the likelihood ratio chi-square statistic
- Check if the model fits well (non-significant statistic) or not (significant statistic)
- Repeat the process until the model no longer accurately fits the data
Model Properties
Log linear models can be complex, but understanding their properties can make them more manageable.
The intercept is a crucial property of log linear models, and it's always included in the model equation.
In a saturated log linear model, all possible interactions between variables are included, which can be computationally intensive.
The number of parameters in a log linear model is determined by the number of variables and their interactions.
A different take: Log Trick Reparameterization Trick
Effect Sizes and Interpretation
Odds ratios are used to compare effect sizes of interactions between variables because they're independent of sample size and not affected by unequal marginal distributions.
In log linear models, interpretation is carried out in terms of odds, which can be calculated using the odds ratio formula. This formula shows that being in one row instead of another is determined only by the distance of the corresponding row main effect values, and hence independent of the column category.
The odds ratio formula implies that the probability of being in one row instead of another, given a certain column category, is equal to the odds ratio. This is a key concept in understanding the relationships between variables in log linear models.
To summarize, the odds ratio formula is:
- For row categories i1 and i2: exp(λi1^X - λi2^X)
- For column categories j1 and j2: exp(λj1^Y - λj2^Y)
Variables
In log-linear analysis, variables are treated equally without a clear distinction between independent and dependent variables.
Variables can be interpreted as either independent or dependent based on their theoretical background.
The variables in log-linear analysis are treated the same, but their interpretation often depends on the context in which they are used.
Effect Sizes
Effect sizes are a crucial aspect of understanding the relationships between variables. They help us quantify the strength and direction of these relationships.
Odds ratios are a preferred measure of effect sizes because they are independent of sample size. This means that the odds ratio will remain the same even if the sample size changes.
Odds ratios are also not affected by unequal marginal distributions. This makes them a more reliable measure of effect sizes compared to other statistics.
In practice, this means that odds ratios can provide a more accurate picture of the relationships between variables, regardless of the sample size or distribution of the data.
Interpretation Via Odds
To interpret the odds, we need to look at the odds ratios. These ratios are used to compare the odds of being in one row instead of another, or one column instead of another.
The odds of being in row i1 instead of row i2 are determined only by the distance of the corresponding row main effect values. This means that the odds are independent of the column category j.
The formula for the odds is given by Equation (4.2), which shows that the odds are simply the exponential of the difference between the row main effect values.
Discover more: What Are the Two Main Types of Generative Ai Models
For example, if we have two rows i1 and i2, and the corresponding row main effect values are λi1 and λi2, then the odds of being in row i1 instead of row i2 are exp(λi1 - λi2).
Similarly, the odds of being in column j1 instead of column j2 are also determined by the difference between the column main effect values.
Here's a summary of the odds ratios:
Note that the odds ratios are independent of the sample size and are not affected by unequal marginal distributions.
Model Identification and Constraints
Model identification is a crucial step in log linear models. Non-identifiability issues arise when the model has more parameters to be learned than it should. This happens when the model has too many free parameters.
To fix this, constraints are imposed on the model. The number of constraints needed is equal to the difference between the number of free parameters of the unconstrained model and the number it should have. For a two-way independence model, this is 2.
Here's an interesting read: Free Gpt Model in Huggingface
Two popular choices for identifiability constraints are:
- Zero-sum constraints, where the sum of the deviations of each category from the overall mean is zero.
- Corner point constraints, where the deviation of one category from the overall mean is zero.
These constraints lead to different interpretations of the lambda parameters, but to the same inference.
Decomposable
A model is decomposable if it has a specific characteristic.
Decomposability is a property of log-linear models that makes them easier to work with.
A log-linear model is graphical if it can be represented as a graph.
The corresponding graph of a log-linear model must be chordal for the model to be decomposable.
Identifiability Constraints
Identifiability constraints are crucial in model identification to ensure that the model is properly specified and can be estimated accurately. They help to fix non-identifiability issues by imposing constraints on the model parameters.
One way to impose identifiability constraints is to set the sum of certain parameters to zero. For example, we can set the sum of the λ_i^X parameters to zero, which means that the deviations of X from the overall mean (λ) are accounted for by the λ_i^X parameters.
Alternatively, we can use reference categories to impose constraints. For instance, we can set λ_I^X = λ_J^Y = 0, which means that the deviations of X and Y from their respective reference categories are accounted for by the λ_i^X and λ_j^Y parameters.
Different identifiability constraints can lead to different interpretations of the model parameters, but they ultimately lead to the same inference. For example, using zero-sum constraints or corner point constraints can result in different expressions for the odds ratio, but the underlying inference remains the same.
Here are some common identifiability constraints used in model identification:
- Zero-sum constraints: λ_1^X + λ_2^X = λ_1^Y + λ_2^Y = 0
- Corner point constraints: λ_2^X = λ_2^Y = 0
These constraints can be used to impose identifiability on the model parameters and ensure that the model is properly specified.
Inference and Fit
Inference and Fit is a crucial aspect of log-linear models. The likelihood function is used to estimate the model parameters, and it's distributed as a product of Poisson distributions for each cell in the contingency table.
The likelihood function can be expressed as a sum of terms involving the log of the expected frequencies and the observed frequencies. This expression is proportional to the sum of the observed frequencies times the log of the expected frequencies minus the expected frequencies.
To fit the model, we need to find the maximum likelihood estimates (MLEs) of the model parameters. This can be done using the method of Lagrange multipliers, which involves finding the values of the parameters that maximize the likelihood function while satisfying certain constraints. The constraints are usually related to the independence of the variables in the model.
The MLEs of the expected frequencies can be used to calculate the MLEs of the model parameters. For the independence model, the MLEs of the expected frequencies are simply the product of the row and column totals divided by the grand total. This can be expressed as:
Inference and Fit
The likelihood function is a key component in determining the fit of a log-linear model. It's a product of the Poisson distribution for each cell, which gives us an idea of how well the model fits the data.
The likelihood function can be expressed as a sum of terms, each representing the contribution of a cell to the overall log-likelihood. This expression is proportional to the sum of two terms: the first term involves the log of the expected frequency, and the second term involves the exponential of the log of the expected frequency.
To make this expression more manageable, we can substitute the relevant expression for the log of the expected frequency. This will give us an expression of the LLM parameters, which we can then use to make inferences about the model.
In the case of the independence model, the log of the expected frequency can be expressed as a product of two terms: the log of the expected frequency for the first variable, and the log of the expected frequency for the second variable. This allows us to obtain MLEs for the LLM parameters using the method of Lagrange multipliers.
Here are the MLEs for the LLM parameters for the independence model:
These formulas give us the MLEs for the LLM parameters, which we can use to make inferences about the model.
Return
Inference and Fit is a crucial step in log-linear analysis. The likelihood function is used to determine how well a model fits the data, with the goal of finding the model that best accounts for the data.
The likelihood function, \(L\), is a product of the probability of each cell in the data, given by the Poisson distribution. It can be simplified to an expression of \(\log E_{ij}\), where \(E_{ij}\) is the expected frequency of cell \((i,j)\). This expression is proportional to the sum of \(n_{ij} \log E_{ij} - e^{\log E_{ij}}\) across all cells.
To proceed with the analysis, we substitute \(\log E_{ij}\) using the relevant expression from the model. For the independence model, this expression is given by Equation (4.1).
The log-linear models can be thought of as a continuum with the simplest model and the saturated model at the extremes. The simplest model assumes that all expected frequencies are equal, while the saturated model includes all possible interactions between variables.
The likelihood ratio chi-square statistic is used to compare the fit of different models. In the saturated model, the observed frequencies equal the expected frequencies, resulting in a likelihood ratio chi-square statistic of 0.
Here's a summary of the key concepts:
- The likelihood function is used to determine how well a model fits the data.
- The likelihood function can be simplified to an expression of \(\log E_{ij}\).
- The expression is proportional to the sum of \(n_{ij} \log E_{ij} - e^{\log E_{ij}}\) across all cells.
- The log-linear models can be thought of as a continuum with the simplest model and the saturated model at the extremes.
- The likelihood ratio chi-square statistic is used to compare the fit of different models.
- In the saturated model, the observed frequencies equal the expected frequencies, resulting in a likelihood ratio chi-square statistic of 0.
By understanding these concepts, you can better navigate the process of inference and fit in log-linear analysis.
Frequently Asked Questions
What is the difference between log-linear model and logistic regression?
Log-linear models and logistic regression differ in their application to data types, with log-linear models handling frequency data and logistic regression handling binary outcome data. Understanding the difference is crucial for selecting the right statistical method for your analysis.
Featured Images: pexels.com