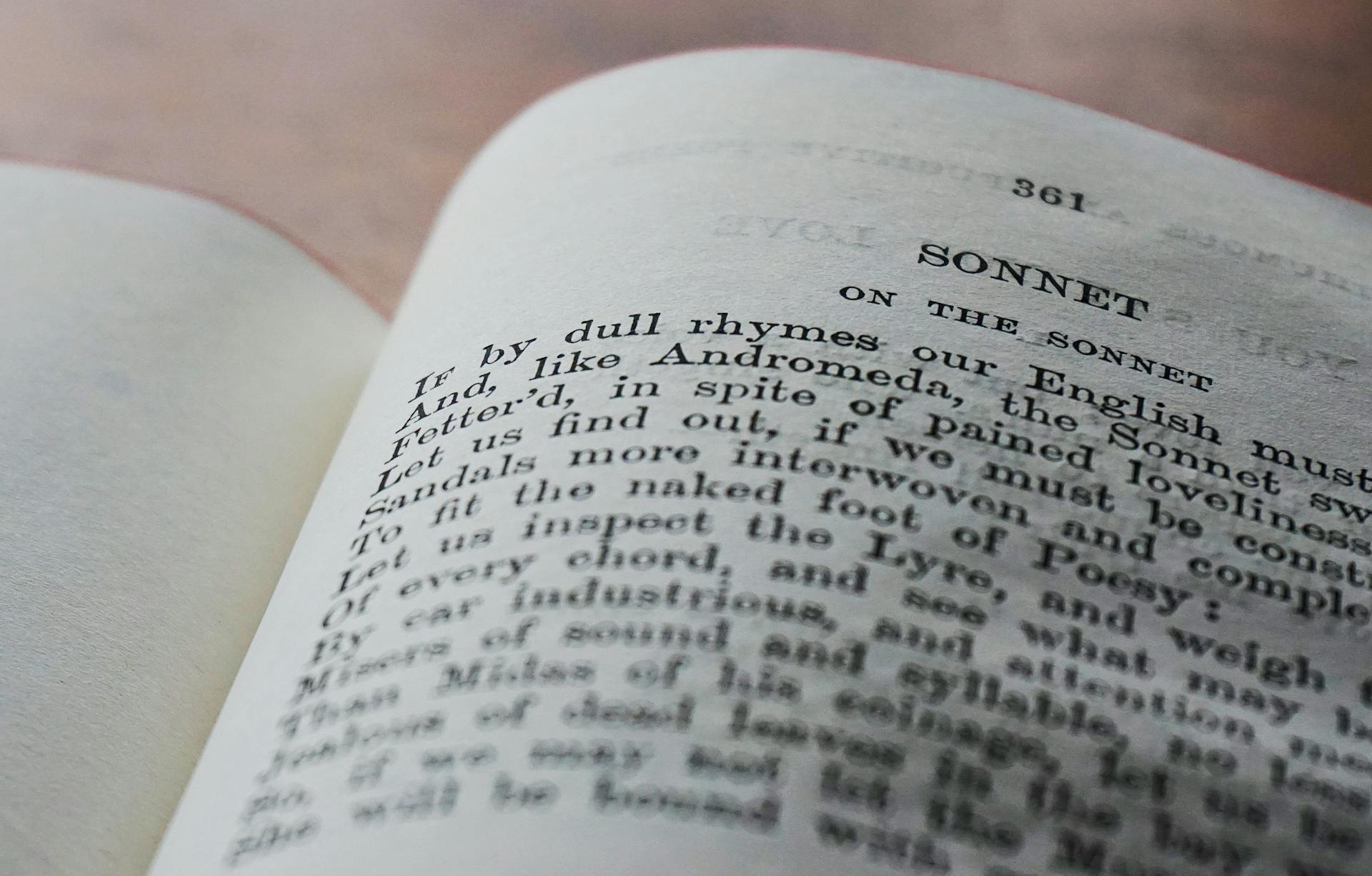
Scaling monosemanticity is a game-changer for AI model interpretability. By analyzing Claude 3 sonnets, we can extract interpretable features that improve our understanding of language.
Claude 3 sonnets are a unique dataset that allows us to study monosemanticity, which refers to the phenomenon where a single word or phrase has multiple, unrelated meanings. This is especially prevalent in language models like Claude 3.
Analyzing Claude 3 sonnets reveals that monosemantic words often appear in specific contexts, such as idiomatic expressions or figurative language. By identifying these patterns, we can develop more accurate and interpretable language models.
The Claude 3 sonnets dataset consists of 100 sonnets, each with 14 lines and a unique combination of monosemantic words.
For another approach, see: Claude 3 Models
Claude 3 Sonnet Analysis
The research on Claude 3 Sonnet demonstrates that sparse autoencoders can scale to larger models, producing high-quality, interpretable features.
With larger models, more monosemantic neurons emerge, which are specialized in responding to specific, single concepts. This leads to improved interpretability, as each neuron has a clearer, specific role.
Intriguing read: Claude Ai Models Ranked
Monosemantic neurons also make the models more efficient by reducing overall complexity and enhancing processing capabilities.
The experiments showed that larger AI models develop more monosemantic neurons, making them easier to understand. This is a key finding in the research on scaling monosemanticity.
The study's findings emphasize the importance of ongoing research to refine these methods and address challenges like data distribution, inability to evaluate, and cross-layer superposition.
A rubric was constructed to score how a feature's description relates to the text it fires, with scores ranging from 0 (completely irrelevant) to 3 (cleanly identifies the activating text).
Here's a breakdown of the rubric scores:
- 0 — The feature is completely irrelevant throughout the context.
- 1 — The feature is related to the context, but not near the highlighted text or only vaguely related.
- 2 — The feature is only loosely related to the highlighted text or related to the context near the highlighted text.
- 3 — The feature cleanly identifies the activating text.
Results and Discussion
The research on scaling monosemanticity has yielded some fascinating results. Larger AI models, like Claude 3 Sonnet, are capable of producing high-quality, interpretable features.
One key finding is that larger models tend to develop more monosemantic neurons, which are specialized in responding to specific, single concepts. This emergence of monosemantic neurons makes the models easier to understand.
Monosemantic neurons also make the models more efficient by reducing overall complexity and enhancing processing capabilities. In fact, the experiments showed that larger models became more efficient as they developed more monosemantic neurons.
The relationship between model size and the number of monosemantic neurons is illustrated in the graphs and tables provided in the research.
Results and Analysis
The research on sparse autoencoders has some fascinating results. Larger AI models, like Claude 3 Sonnet, can produce high-quality features that are easier to understand.
The experiments showed that scaling up AI models leads to the emergence of more monosemantic neurons. These neurons are specialized in responding to specific, single concepts.
As a result, larger models become more interpretable, with each neuron having a clearer role. This makes it easier to understand how the model is working.
Monosemantic neurons also make the models more efficient by reducing overall complexity and enhancing processing capabilities.
Here's a breakdown of the key findings:
- Emergence of Monosemantic Neurons: Larger AI models develop more neurons specialized in responding to specific concepts.
- Improved Interpretability: With more monosemantic neurons, larger models become easier to understand.
- Efficiency: Monosemantic neurons reduce complexity and enhance processing capabilities.
Lessons Learnt
One of the most valuable takeaways from our research is that sparse autoencoders scale well and produce interpretable features, handling large models with ease and extracting meaningful and high-quality features.
Sparse autoencoders can be particularly effective when used in conjunction with L1 regularization, which encourages only a few active features for any input, enhancing model interpretability.
To ensure consistency in breaking down model activations, it's essential to normalize activations before decomposition.
Balancing reconstruction quality and feature sparsity can be achieved by combining reconstruction error with L1 penalty.
Adjusting feature values through clamping can predictably alter model outputs, allowing for more control over AI behavior.
Smaller residual streams can make training cheaper, making them a cost-efficient option.
To minimize loss within a fixed compute budget, optimizing features and training steps using scaling laws is a game-changer.
Automated methods can improve feature analysis, making it easier to evaluate many features quickly and accurately.
Expand your knowledge: Claude 3 Model Card
Stronger activations in a model typically correlate with specificity, making them more useful.
Feature interpretability is more important than just achieving lower losses – understanding model behavior is crucial.
Feature steering can induce specific errors, highlighting the need for careful control to prevent misuse.
Larger sparse autoencoders can capture more diverse and abstract concepts, representing more fine-grained and varied concepts.
The strength of feature activation matters, with high specificity in strong activations being crucial for model behavior.
Here's a quick rundown of some key takeaways from our research:
- Sparse autoencoders scale well and produce interpretable features.
- L1 regularization encourages feature sparsity and enhances model interpretability.
- Normalizing activations before decomposition ensures consistency.
- Combining reconstruction error with L1 penalty balances reconstruction quality and feature sparsity.
- Clamping features can steer model behavior.
- Residual streams can make training cheaper.
- Optimizing features and training steps using scaling laws minimizes loss within a fixed compute budget.
- Automated methods improve feature analysis.
- Stronger activations correlate with specificity.
- Feature interpretability is more important than achieving lower losses.
- Larger sparse autoencoders capture more diverse and abstract concepts.
- Feature activation strength matters.
Sources
- https://transformer-circuits.pub/2024/scaling-monosemanticity/
- https://medium.com/thedeephub/understanding-the-scaling-of-monosemanticity-in-ai-models-a-comprehensive-analysis-f72818fa44ca
- https://ghost.oxen.ai/scaling-monosemanticity-claude-3/
- https://www.pelayoarbues.com/literature-notes/Articles/Scaling-Monosemanticity-Extracting-Interpretable-Features-From-Claude-3-Sonnet
- https://www.summiz.ai/summaries/3ExCe8x
Featured Images: pexels.com