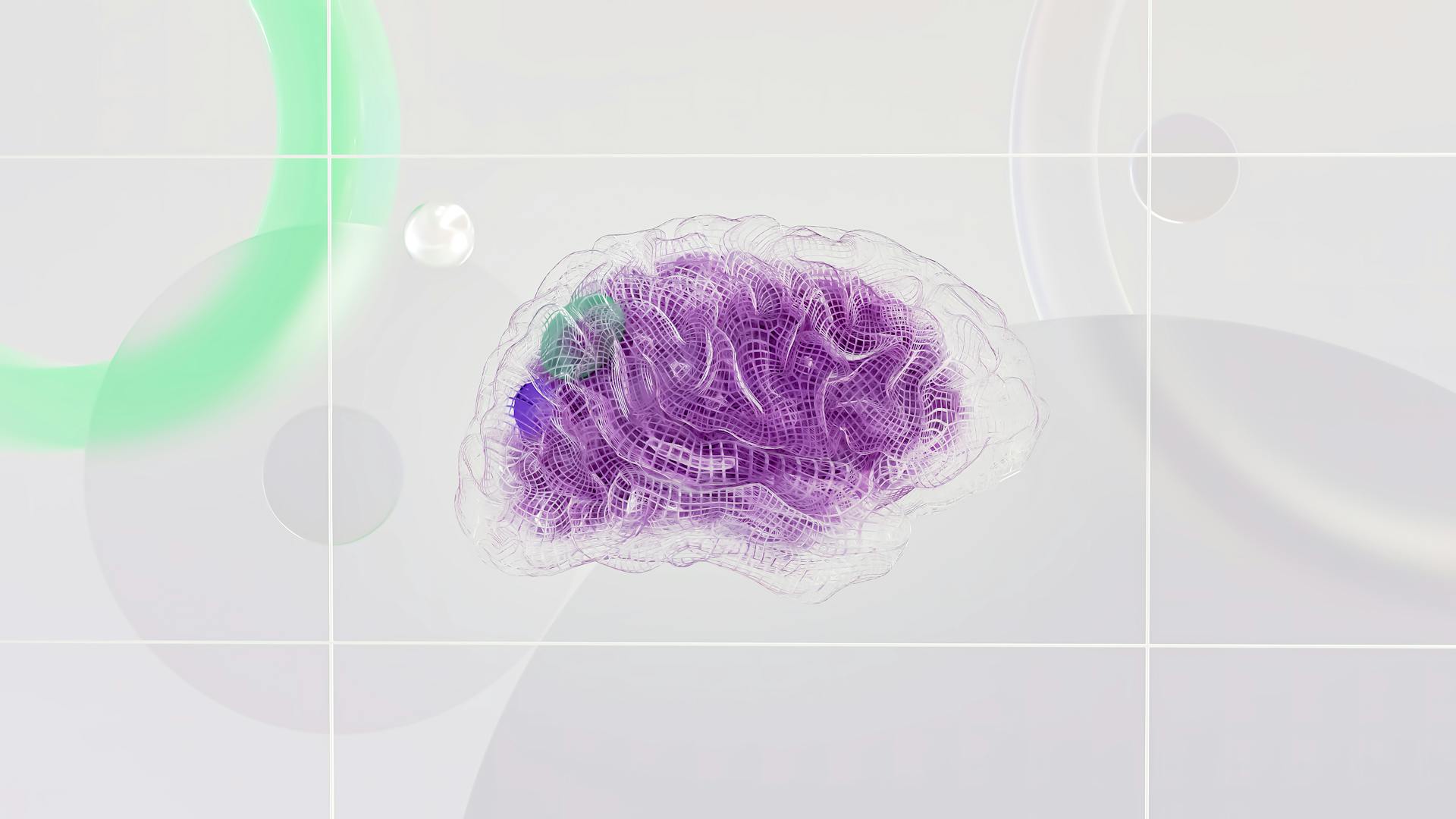
AI and brain computer interface (BCI) technology is revolutionizing the way we interact with the world. BCI systems can read brain signals and translate them into digital commands, giving people with paralysis or other motor disorders a new way to communicate.
This technology has the potential to greatly improve the lives of millions of people worldwide. For example, a paralyzed person can use a BCI to control a computer or even a robotic arm with their thoughts.
One of the most significant benefits of BCI technology is its potential to restore communication abilities in people with severe paralysis. In fact, studies have shown that BCI systems can help people with locked-in syndrome, a condition in which a person is aware but unable to move or speak.
BCI systems are also being used to help people with neurological disorders such as epilepsy and Parkinson's disease. Researchers are working on developing BCIs that can detect seizures or tremors, allowing for early intervention and improved treatment.
See what others are reading: Ai Is the Theory and Development of Computer Systems
Brain-Computer Interface (BCI)
Brain-Computer Interface (BCI) is a technology that translates neural signals into functional movement commands, enabling early intervention for effective rehabilitation in patients with motor impairments resulting from conditions like stroke, spinal cord injuries, and traumatic brain injuries.
BCIs are increasingly being used for neurorehabilitation, and researchers are exploring the possibility of using them to map and convert thoughts into actionable commands and even physical objects, which could significantly benefit individuals with severe physical disabilities.
The integration of AI enhances the capabilities of BCIs by improving how these systems interpret neural data, filtering out noise from brain signals caused by muscle movements or external environmental factors.
Deep learning models are used to distinguish relevant neural patterns from noisy datasets, which is essential for the accurate functioning of BCIs.
BCIs are being developed to extend beyond basic command execution to more sophisticated applications such as decoding thoughts, extending human memory, and even facilitating telepathy-like communication.
AI plays a pivotal role in enhancing the capabilities of BCIs, and standardized protocols and more sensitive neuroimaging technologies are needed to overcome the challenges faced by the field.
For your interest: Ai Capabilities
Data and Workflow
The brain-computer interface (BCI) system starts with Signal Acquisition, where brain signals are captured using methods like EEG, which records electrical activity from the brain.
This process is crucial for improving the accuracy of the feature extraction that follows. The raw signals acquired are then pre-processed to enhance signal quality and reduce noise.
The workflow of a BCI system involves several stages, each with a specific function. Here are the main stages:
- Signal Acquisition: captures user's intentions or mental events
- Pre-processing: enhances signal quality and reduces noise
- Signal Processing - Feature Extraction: extracts relevant features from pre-processed brain signals
- Pattern Recognition: analyzes extracted features to recognize patterns associated with different brain activities or commands
- Post-processing: refines control signals to ensure accuracy
- Control Signal: converts processed information into a control signal for operating an external device
Open and Transparent Data
Having open and transparent data is crucial for the advancement of AI-BCI technologies. This is because diverse, comprehensive datasets are essential for training AI systems.
By sharing BCI data openly, researchers can collaborate more effectively, accelerating technological advancements and innovation. This is why open data initiatives are so important.
Open datasets help ensure that AI systems are trained on diverse data, reducing the risk of bias and improving generalizability. This is a key factor in building public trust and ensuring ethical compliance.
Transparency in data usage and algorithmic function allows for greater scrutiny and validation of AI-BCI systems. This is achieved through open data initiatives that promote accountability.
Here are the benefits of open and transparent data in a nutshell:
- Fosters innovation by accelerating technological advancements and collaboration
- Ensures bias mitigation by training AI systems on diverse data
- Increases accountability by promoting transparency and scrutiny
System Workflow
System Workflow is a crucial aspect of any brain-computer interface (BCI) system. It's the process by which the system captures, processes, and interprets brain signals to control external devices.
The workflow begins with Signal Acquisition, where sensors placed on the scalp record electrical activity from the brain using methods like EEG. This is typically done to capture the user's intentions or mental events.
Pre-processing is the next step, where raw signals are enhanced and noise is reduced to improve signal quality. This is crucial for improving the accuracy of feature extraction that follows.
Signal Processing - Feature Extraction involves extracting relevant features from pre-processed brain signals. These features represent the meaningful information that differentiates various mental states or intentions.
Pattern Recognition analyzes the extracted features to recognize patterns associated with different brain activities or commands. This involves statistical or machine learning models that classify features into predefined categories corresponding to specific commands or thoughts.
The system's workflow also includes Post-processing, where data may undergo additional processing to refine control signals. This ensures they are accurate and suitable for controlling a device.
Here's a breakdown of the system workflow:
- Signal Acquisition: captures brain signals using EEG or other methods
- Pre-processing: enhances signal quality and reduces noise
- Signal Processing - Feature Extraction: extracts relevant features from pre-processed signals
- Pattern Recognition: analyzes features to recognize patterns associated with brain activities or commands
- Post-processing: refines control signals to ensure accuracy
- Control Signal: converts processed information into a control signal
- Device: operates or manipulates a device based on user's intention
- Feedback: provides feedback to the user to adjust mental commands in real-time
Machine Learning and Performance
Convolutional Neural Networks (CNNs) excel in extracting spatial features from multidimensional data, autonomously recognizing spatial hierarchies crucial for identifying patterns linked to specific neural activities.
Deep Belief Networks (DBNs) are instrumental for unsupervised feature learning, delving deeper into data structures to pinpoint subtle features that simpler models might overlook.
Support Vector Machines (SVMs) are typically employed due to their effectiveness in handling high-dimensional spaces, which is typical of EEG data.
Recurrent Neural Networks (RNNs) and Long Short-Term Memory Networks (LSTMs) are utilized for their prowess in classifying data sequences, making them ideal for tasks requiring an understanding of temporal dynamics, such as continuous motor movements or speech processes.
Epilepsy Management
Epilepsy Management has seen significant advancements with the application of AI-enhanced Brain-Computer Interfaces (BCIs). These systems can analyze EEG data to identify patterns that precede epileptic seizures.
AI algorithms can detect subtle changes in brain activity that may indicate an impending seizure, often well before the patient experiences any symptoms. This allows for preemptive medical interventions that can significantly improve patient safety and quality of life.
One key benefit of using AI in this context is its ability to enhance the accuracy and timeliness of seizure predictions. By continuously monitoring EEG signals, AI algorithms can detect subtle changes in brain activity that may indicate an impending seizure.
The goal is to integrate these systems into a seamless interface that can provide real-time feedback and intervention, enhancing the autonomy and safety of individuals living with epilepsy.
Machine Learning Models
Deep learning models, particularly Convolutional Neural Networks (CNNs) and Deep Belief Networks (DBNs), are pivotal in extracting spatial features from multidimensional data.
These models excel in autonomously recognizing spatial hierarchies crucial for identifying patterns linked to specific neural activities.
Convolutional Neural Networks (CNNs) are ideal for tasks that require spatial pattern recognition, such as identifying neural activities in EEG data.
Deep Belief Networks (DBNs) are instrumental for unsupervised feature learning, delving deeper into data structures to pinpoint subtle features that simpler models might overlook.
Recurrent Neural Networks (RNNs) and Long Short-Term Memory Networks (LSTMs) are utilized for their prowess in classifying data sequences, making them ideal for tasks requiring an understanding of temporal dynamics.
These models are particularly useful for tasks such as continuous motor movements or speech processes.
Support Vector Machines (SVMs) are typically employed due to their effectiveness in handling high-dimensional spaces, which is typical of EEG data.
Transfer learning is a notable approach that allows BCIs to adapt to new users or changing conditions without extensive retraining, leveraging pre-trained models that can transfer learned features to new datasets.
This approach enables BCIs to learn from existing knowledge and adapt to new situations, making them more efficient and effective.
Explore further: Copilot New Computer Ai
Ethics and Considerations
As we explore the exciting world of AI and brain computer interface (BCI) technologies, it's essential to acknowledge the ethical considerations that come with them. Privacy and consent are paramount, as AI-BCI systems handle sensitive neural data.
To ensure the security of this information, users must be fully informed about how their data will be used and must consent to these uses explicitly. This is a crucial step in maintaining trust in these technologies.
Dependence on AI-BCI systems can also be a concern, potentially impacting individual autonomy. It's essential to ensure that these technologies enhance rather than reduce autonomy.
The accessibility and equity of AI-BCI technologies are also critical issues that need to be addressed. If not, we risk creating a 'neurotech divide' where only certain segments of the population benefit from these advancements.
To better understand the challenges ahead, let's break down the key ethical considerations:
- Privacy and Consent: Users must be informed about data use and consent explicitly.
- Dependence and Autonomy: AI-BCI systems should enhance, not reduce, individual autonomy.
- Accessibility and Equality: Addressing the neurotech divide to ensure equal access to these advancements.
Sources
- https://pmc.ncbi.nlm.nih.gov/articles/PMC10728818/
- https://medium.com/dataduniya/ai-and-brain-computer-interfaces-connecting-minds-and-machines-c8e8ad04f683
- https://bionichaos.com/BCI-AI/
- https://engineering.cmu.edu/news-events/news/2024/04/30-noninvasive-bci.html
- https://www.forbes.com/sites/bernardmarr/2023/08/11/ais-next-frontier-are-brain-computer-interfaces-the-future-of-communication/
Featured Images: pexels.com