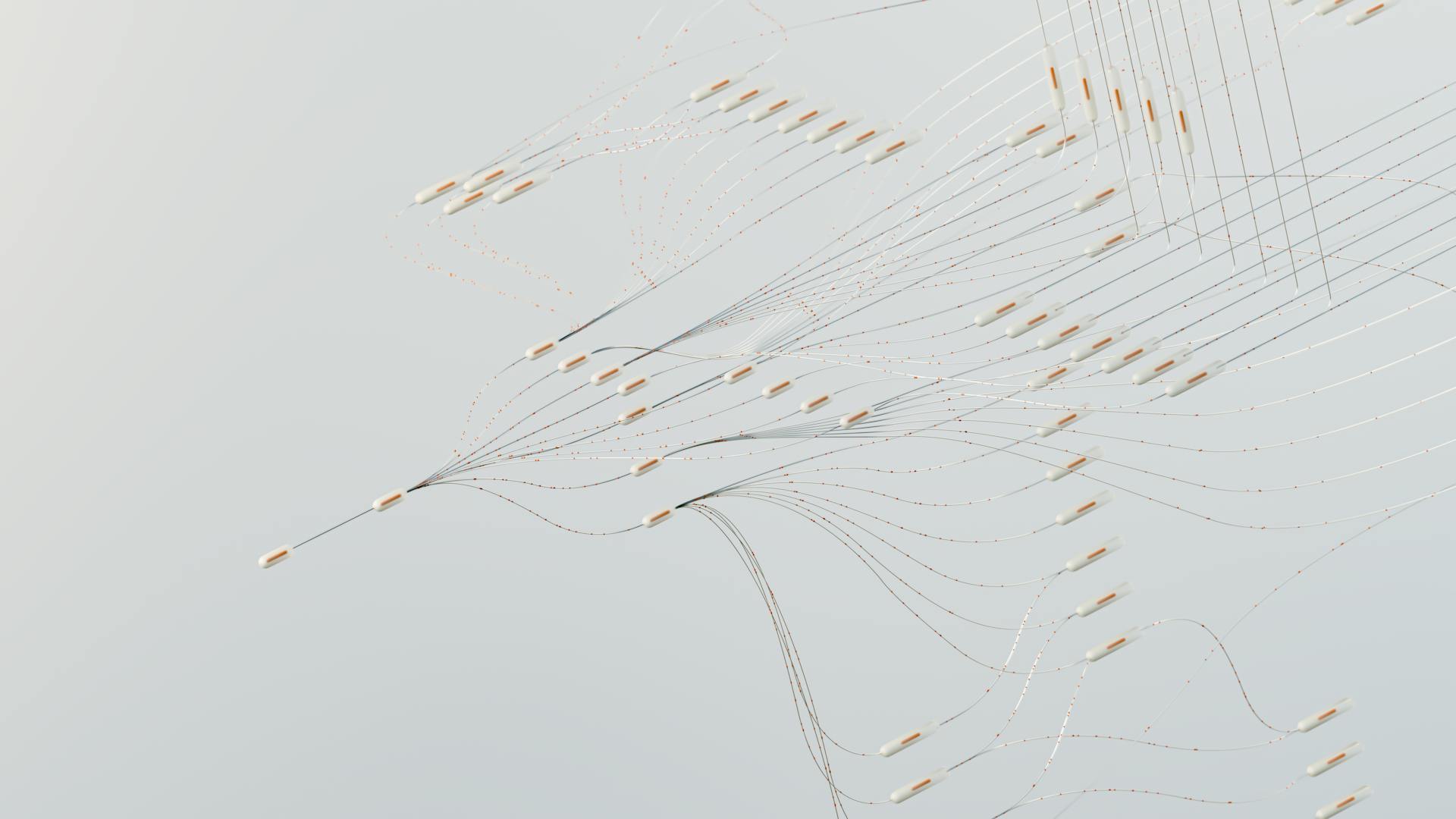
Cognitive AI is designed to mimic the human brain's ability to reason, learn, and solve problems. It's like having a super-smart friend who can understand context and make informed decisions.
Generative AI, on the other hand, is focused on creating new content, such as images, music, or text, based on patterns it's learned from existing data. This can lead to some amazing creations, like AI-generated art.
One key difference between the two is that cognitive AI is typically used for tasks that require understanding and reasoning, while generative AI is used for tasks that require creativity and novelty. For instance, cognitive AI might be used to analyze medical data, while generative AI might be used to create new medical imaging techniques.
Cognitive AI often relies on machine learning and deep learning techniques to improve its performance over time, while generative AI uses these techniques to generate new content.
Expand your knowledge: Google Announces New Generative Ai Search Capabilities for Doctors
Key Differences Between
Cognitive AI and generative AI are two distinct approaches to artificial intelligence, each with its own strengths and weaknesses.
Cognitive AI focuses on analyzing and interpreting existing data to improve efficiency, accuracy, and decision-making, operating within predefined boundaries.
Generative AI, on the other hand, is designed to create new content, such as text, images, music, and models, based on existing data.
Traditional AI models are generally more transparent and interpretable than generative AI models, which often function as "black boxes", making their decision-making processes less transparent.
Generative AI models require substantial computational resources and training time, making it more difficult to scale compared to traditional AI, which can be more efficient, especially when dealing with specific tasks.
Here's a summary of the key differences between cognitive AI and generative AI:
By understanding these differences, you can choose the right approach for your specific needs and goals.
Characteristics and Advantages
Cognitive AI and Generative AI have distinct characteristics that set them apart. Generative AI is an open-ended and rapidly evolving form of artificial intelligence that relies on unsupervised or self-supervised learning, identifying patterns and relationships without explicit labels.
Generative AI uses advanced models like GANs, VAEs, and large language models such as GPT-3 and GPT-4, designed to create new data similar to training inputs. This allows it to generate original content such as text, images, music, and designs from existing data.
One of the key advantages of Generative AI is its adaptability, as it can adapt to new data and continuously improve its outputs without needing explicit rule updates. This makes it particularly useful in data-scarce environments.
Here are some key characteristics and advantages of Generative AI:
- Data-Driven Learning: Identifies patterns and relationships without explicit labels
- Generative Models: Creates new data similar to training inputs
- Creativity and Innovation: Generates original content from existing data
- Adaptability: Adapts to new data and continuously improves its outputs
- Data Augmentation: Easily creates synthetic data to augment existing datasets
- Personalization: Provides users with personalized experiences by crafting content adapted to individual preferences
Characteristics of Cognitive AI
Cognitive AI is a type of AI that thinks and makes decisions like humans. It's a step beyond generative AI, which can create new content but doesn't necessarily understand the underlying context.
Cognitive AI uses machine learning and natural language processing to analyze data and make decisions. This is different from traditional AI, which relies on rules and patterns.
One of the key characteristics of cognitive AI is its ability to learn from data and improve over time. This is similar to how humans learn and adapt to new situations.
Cognitive AI can be used in a variety of applications, including decision-making, problem-solving, and even creativity. It's not just about processing data, but also about understanding the underlying context and making decisions based on that understanding.
Here are some of the key characteristics of cognitive AI:
- Uses machine learning and natural language processing to analyze data and make decisions
- Can learn from data and improve over time
- Understands the underlying context and makes decisions based on that understanding
- Can be used in a variety of applications, including decision-making, problem-solving, and creativity
Cognitive AI has the potential to revolutionize the way we approach complex problems and make decisions. It's an exciting area of research and development that holds a lot of promise for the future.
Advantages of Cognitive AI
Cognitive AI is a game-changer when it comes to tackling complex tasks. It can perform tasks that Traditional AI models struggle with, such as adapting to new situations or variations outside the training data.
One of the key advantages of Cognitive AI is its ability to learn from experience and improve over time. This means it can handle complex or non-deterministic scenarios that Traditional AI models often fall short on.
Cognitive AI can also be used for creativity, unconventional thinking, or novel solutions, which is a significant limitation of Traditional AI models. This is a major breakthrough in the field of artificial intelligence.
Cognitive AI is not limited to specific tasks like Traditional AI models, making it a more versatile and effective tool for a wide range of applications.
Strengths and Limitations of Cognitive AI
Cognitive AI has the ability to think and make decisions like humans, thanks to machine learning and natural language processing. This is a major advancement over traditional AI and generative AI.
One of the key strengths of cognitive AI is its ability to surpass human thought processes and decision making. This is a result of its advanced machine learning capabilities.
Cognitive AI models are able to perform complex tasks and adapt to new situations, which is a major advantage over traditional AI. Traditional AI models struggle with complex or non-deterministic scenarios.
However, cognitive AI also has limitations. It requires vast and diverse datasets to perform effectively, which can lead to potential biases in the training data.
Here are some key limitations of cognitive AI:
- Limited Flexibility: Cognitive AI models can struggle to adapt to new, unseen scenarios, which can hinder their application in dynamic environments
- Lack of Creativity: Cognitive AI is confined to the rules it has been programmed with, meaning it cannot generate new content or solutions beyond its predefined scope
- Transparency and Explainability: Some cognitive AI models can be opaque, making it difficult to understand how they make decisions
- Ethical Concerns: AI is not immune to issues related to bias and decision-making processes arising from the data and rules a given system is using
Despite these limitations, cognitive AI has the potential to revolutionize various industries and applications. Its ability to think and make decisions like humans makes it a valuable tool for solving complex problems.
Limitations and Challenges
Cognitive AI and generative AI have their own set of limitations and challenges. Cognitive AI models struggle to adapt to new situations or variations outside the training data. This inflexibility can hinder their application in dynamic environments.
Gen AI, on the other hand, can be misused to generate deep-fake content and other potentially harmful outputs, fanning ethical challenges of misuse and misinformation. Transparency is also an issue, as the inner workings of deep learning models can be difficult to interpret.
Here are the key limitations of both Cognitive AI and Gen AI:
- Cognitive AI: inflexibility, inability to handle creativity, and struggles with novel problem-solving.
- Gen AI: potential biases in training data, hallucination errors, and data privacy concerns.
These limitations highlight the need for careful consideration and development of these technologies to ensure they are used responsibly and effectively.
Limitations of Cognitive AI
Traditional AI models are limited in their ability to adapt to new situations or variations outside the training data. They struggle to handle tasks that require creativity or unconventional thinking.
TA's scope is limited to specific tasks, making it inflexible and unable to be used for novel solutions. This is a significant limitation, as it restricts its applications to repetitive and well-defined tasks.
Gen AI models, on the other hand, grapple with potential biases inherent in the training data. This can lead to inaccurate or unfair outcomes, which is a major concern.
Creating effective Gen AI models requires significant computational resources and expertise. This can be a barrier to entry for many organizations or individuals.
What's the Downside?
The downsides of generative AI are significant, and it's essential to understand them. One major issue is the lack of transparency, making it difficult to interpret the inner workings of deep learning models.
Generative AI can be misused to generate deep-fake content and other potentially harmful outputs, fanning ethical challenges of misuse and misinformation. This is a serious concern, as it can lead to the spread of false information and harm individuals or organizations.
Another significant drawback is the quality control issue. Making sure that the generated content meets quality standards can be challenging, especially since generative AI is notorious for generating false information. This can lead to a loss of trust in the technology.
Bias is also a significant issue with generative AI models. They can inadvertently learn and propagate biases present in training data to produce unfair outcomes. This can have serious consequences, particularly in areas such as hiring, education, and healthcare.
Here are some of the key limitations of generative AI:
- Lack of transparency
- Ethical concerns
- Quality control issues
- Bias in training data
These challenges highlight the need for careful consideration and responsible development of generative AI technologies. By understanding the downsides of generative AI, we can work towards creating more transparent, trustworthy, and equitable AI systems.
Use Cases and Applications
Cognitive AI and Generative AI have various use cases and applications, each with its own strengths and weaknesses.
Cognitive AI is commonly used in structured data analysis, automated customer support, and process automation. It's also used in image enhancement and editing tools, making precise, rule-based adjustments in existing images.
Generative AI, on the other hand, excels at producing new and unique images, simulating realistic or creative visuals not found in the training data. It's also used in digital art, music, marketing content, and innovative design, helping with complex problem-solving across various domains.
Here's a comparison of some of the key applications of Cognitive AI and Generative AI:
By understanding the differences between Cognitive AI and Generative AI, we can better determine which type of AI is best suited for a particular task or application.
Use Cases and Applications
Generative AI has a wide range of applications, from artistic expression to problem-solving across various industries. It can generate images, text, music, and even videos that are indistinguishable from those created by humans.
One of the most exciting applications of generative AI is in image generation, where models can create realistic images that look like real photographs or paintings. This technology has the potential to revolutionize industries such as advertising, fashion, and art.
Discover more: Ai and Ml Images
Generative AI can also be used for text generation, where models can understand language and context to create written content that resembles human writing. This can be useful for applications such as content creation, where AI can generate articles, social media posts, and other types of written content.
In addition to image and text generation, generative AI can also be used for music composition, where models can analyze patterns and styles to create original pieces. This technology has the potential to revolutionize the music industry and create new opportunities for artists and composers.
Here are some of the key use cases for generative AI:
- Image generation: Gen AI models can generate images that look like real photographs or paintings.
- Text generation: With the ability to understand language and context, Gen AI models can generate written content that resembles human writing.
- Music composition: Specially created models can compose original pieces by analyzing patterns and styles.
- Video synthesis: Gen AI models can combine and manipulate video footage to create new videos.
- Drug discovery: It can assist in identifying new drugs for research and development by learning from chemical compounds and their properties.
Generative AI can also be used in more practical applications, such as process automation, where it can help streamline complex tasks and improve efficiency. It can also be used in digital art, music, marketing content, and innovative design.
In contrast, traditional AI systems are commonly used by businesses and organizations to automate processes, improve efficiency, and gain insights from data. However, generative AI systems are often used by creative professionals, such as artists, designers, and musicians, to augment their creative process and unlock new creative potential.
Additional reading: Can Generative Ai Improve Social Science
Applications of Cognitive AI
Cognitive AI is being used to improve customer service, with chatbots that can understand and respond to customer inquiries in a more human-like way. These chatbots can be integrated into websites and mobile apps to provide 24/7 support.
One example is a chatbot that uses natural language processing to help customers track their packages. This chatbot can understand the customer's question and provide a personalized response, such as "Your package is currently in transit and is expected to arrive by Friday."
Cognitive AI is also being used to analyze customer behavior and preferences, helping businesses to create more effective marketing campaigns. By analyzing customer data, businesses can identify patterns and trends that inform their marketing strategies.
For instance, a company used cognitive AI to analyze customer data and found that customers who purchased a certain product were also likely to purchase a related product. This information helped the company to create targeted marketing campaigns that resulted in a 20% increase in sales.
See what others are reading: Ai Training Company
Cognitive AI is being used to improve healthcare outcomes, with applications such as medical diagnosis and personalized treatment plans. For example, a cognitive AI system was used to analyze medical images and diagnose a patient with a rare form of cancer.
Cognitive AI is also being used to improve education, with applications such as adaptive learning and personalized learning plans. By analyzing student data, teachers can create tailored learning plans that meet the individual needs of each student.
For instance, a cognitive AI system was used to create a personalized learning plan for a student who was struggling with math. The system analyzed the student's strengths and weaknesses and provided a customized learning plan that helped the student to improve their math skills by 30%.
For another approach, see: Create with Confidence Using Generative Ai
Frequently Asked Questions
What is the difference between Cognitive AI and AI?
Cognitive AI systems mimic human thought processes and can learn and adapt, whereas traditional AI is programmed to perform specific tasks and doesn't learn or change. This key difference makes Cognitive AI more dynamic and versatile.
Sources
- https://www.eweek.com/artificial-intelligence/generative-ai-vs-ai/
- https://medium.com/@byanalytixlabs/generative-ai-vs-traditional-ai-understand-key-differences-ca2d3e37c45d
- https://www.mycase.com/blog/ai/ai-vs-generative-ai/
- https://www.rackspace.com/blog/distinctions-ai-ml-generative-ai
- https://csdisco.com/blog/ai-vs-generative-ai-whats-the-difference
Featured Images: pexels.com