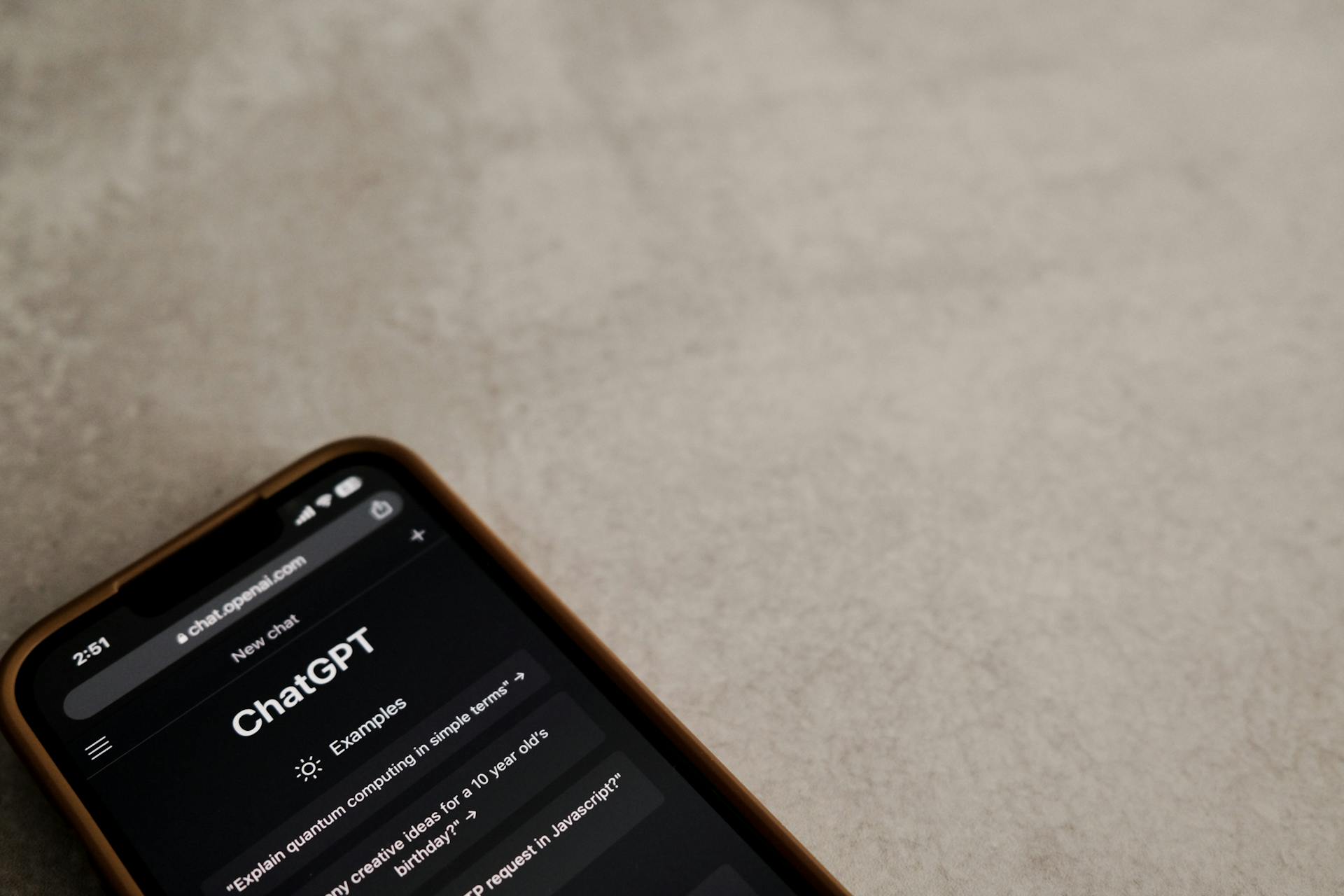
Conversational AI and Generative AI are two distinct types of AI technologies that have been gaining popularity in recent years.
Conversational AI is designed to understand and respond to human language, allowing for more natural and interactive conversations. It's like having a chat with a knowledgeable friend.
Unlike Generative AI, which focuses on creating new content, conversational AI is primarily concerned with facilitating dialogue and answering questions. According to the article, conversational AI can process and respond to up to 10,000 user inputs per hour.
What Is Conversational AI?
Conversational AI is specifically designed for dynamic interactions. It's like having a conversation with a friend - it's meant to be a back-and-forth exchange.
Conversational AI is often more adept at understanding context and user intent. This means it can pick up on subtle cues and respond accordingly.
Its primary goal is to engage in meaningful interactions with users. This can be through chatbots, voice assistants, or even text-based interfaces.
Conversational AI is typically more focused on the conversation itself, rather than generating new content. This allows it to tailor its responses to the user's needs and interests.
If this caught your attention, see: How Are Modern Generative Ai Systems Improving User Interaction
What Is Generative AI?
Generative AI is a type of artificial intelligence that can create diverse forms of content, including text, images, music, and even videos.
It does this by analyzing patterns and structures in the training data, which allows it to generate new content that aligns with the learned patterns.
Generative AI models have the ability to create new content that's unique and varied, making them useful for applications like content generation and creative tasks.
Here are some examples of the types of content Generative AI can create:
- Text
- Images
- Music
- Videos
Applications and Examples
Conversational AI has numerous applications, from virtual assistants to customer support. Virtual assistants like Apple's Siri, Amazon's Alexa, and Google Assistant provide personalized assistance and perform tasks based on user commands.
Conversational AI can facilitate real-time translation between different languages, breaking down language barriers and enabling global communication. It's also a common component in chatbots and virtual assistants customers interact with through text and speech, for self-service interactions.
Some examples of Conversational AI applications include:
- Virtual Assistants: Apple's Siri, Amazon's Alexa, and Google Assistant
- Customer Support: Chatbots and voice bots powered by Conversational AI
- Language Translation: Real-time translation between different languages
- Voice-controlled Interfaces: Devices and systems that can be controlled through voice commands
Creative Versatility
Generative AI is known for its creative versatility, producing unique and novel outputs based on the data it has been trained on.
This ability to generate original content that exhibits creativity and diversity makes Generative AI a powerful tool in various creative domains.
Generative AI can produce a wide range of creative outputs, from art and music to writing and design.
The ability to generate novel outputs based on training data is a key characteristic of Generative AI.
Here are some examples of the types of creative outputs that Generative AI can produce:
- Art and graphics
- Music and audio
- Writing and literature
- Design and architecture
This creative versatility has many potential applications, from entertainment to education and beyond.
Examples of Applications
Conversational AI is all around us, and its applications are diverse. It powers virtual assistants like Apple's Siri, Amazon's Alexa, and Google Assistant, which provide personalized assistance and perform tasks based on user commands.
These virtual assistants have become an integral part of our daily lives, making it easy to control our smart homes, send messages, and access information with just our voice. They're a great example of how conversational AI can enhance our user experience.
Conversational AI is also used in customer support, where chatbots and voice bots handle common queries and guide users through self-service options. This helps reduce the workload of human customer support agents and provides faster solutions to customers.
We've all interacted with chatbots on websites, messaging apps, and customer service platforms. They're designed to provide quick and efficient solutions to common issues.
Conversational AI is integrated into devices and systems, enabling users to interact with them through voice commands. This hands-free control enhances accessibility and convenience.
Here are some examples of conversational AI applications:
These examples showcase the versatility of conversational AI and its potential to improve various aspects of our lives.
How It Works
Conversational AI is designed to understand and respond to user input in a conversational manner, using natural language processing (NLP) to analyze and interpret user queries.
This approach allows conversational AI to engage in dialogue, answering follow-up questions and adapting to the user's tone and language. It can also use context to understand the conversation's flow and intent.
Conversational AI typically uses a combination of machine learning algorithms and NLP to process user input, which enables it to learn from user interactions and improve its responses over time.
A fresh viewpoint: Are Large Language Models Generative Ai
Speech Recognition
Conversational AI systems use advanced algorithms to convert spoken language into textual form.
These algorithms enable the systems to understand and process user inputs in the form of speech or voice commands.
This technology is a key component of conversational AI, allowing users to interact with systems in a more natural and intuitive way.
The algorithms used in speech recognition are complex and sophisticated, but they're also incredibly effective.
By converting speech into text, conversational AI systems can process and respond to user inputs in a timely and accurate manner.
Discover more: Which Term Describes the Process of Using Generative Ai
Dialogue Management
Dialogue Management is the backbone of conversational AI systems, enabling them to maintain coherent and context-aware conversations.
Conversational AI systems employ robust dialogue management algorithms to understand and respond to user inputs in a natural, human-like manner. These algorithms are crucial in ensuring that the conversation flows smoothly and stays on track.
Dialogue management algorithms analyze the user's input, including their intent, context, and entities, to determine the best course of action. By leveraging this information, the AI system can generate responses that are relevant and accurate.
Here's an interesting read: Why Is Controlling the Output of Generative Ai
Here are some key features of dialogue management algorithms:
- Context-aware: Dialogue management algorithms can understand the context of the conversation and adjust their responses accordingly.
- Intent recognition: These algorithms can recognize the user's intent behind their input, determining the action or information they seek.
- Entity recognition: Dialogue management algorithms can identify and extract relevant entities from the user's input, such as names, locations, or objects.
By employing robust dialogue management algorithms, conversational AI systems can create an interactive and dynamic conversational experience that feels natural and intuitive to the user.
How Are Trained?
Conversational AI systems are trained on large datasets of human interactions, which is why they can understand and respond to user inputs in a natural, human-like manner.
To generate human-like responses, Conversational AI systems employ Natural Language Generation (NLG) techniques, which leverage pre-defined templates, machine learning models, or even neural networks.
Conversational AI systems use dialogue management algorithms to maintain coherent and context-aware conversations, enabling them to respond to user inputs in a way that feels natural and intuitive.
Generative AI models, on the other hand, are trained on specific datasets relevant to the type of content they are expected to generate. This training enables them to learn from large datasets and improve the quality and diversity of the generated outputs.
A different take: How Generative Ai Can Augment Human Creativity
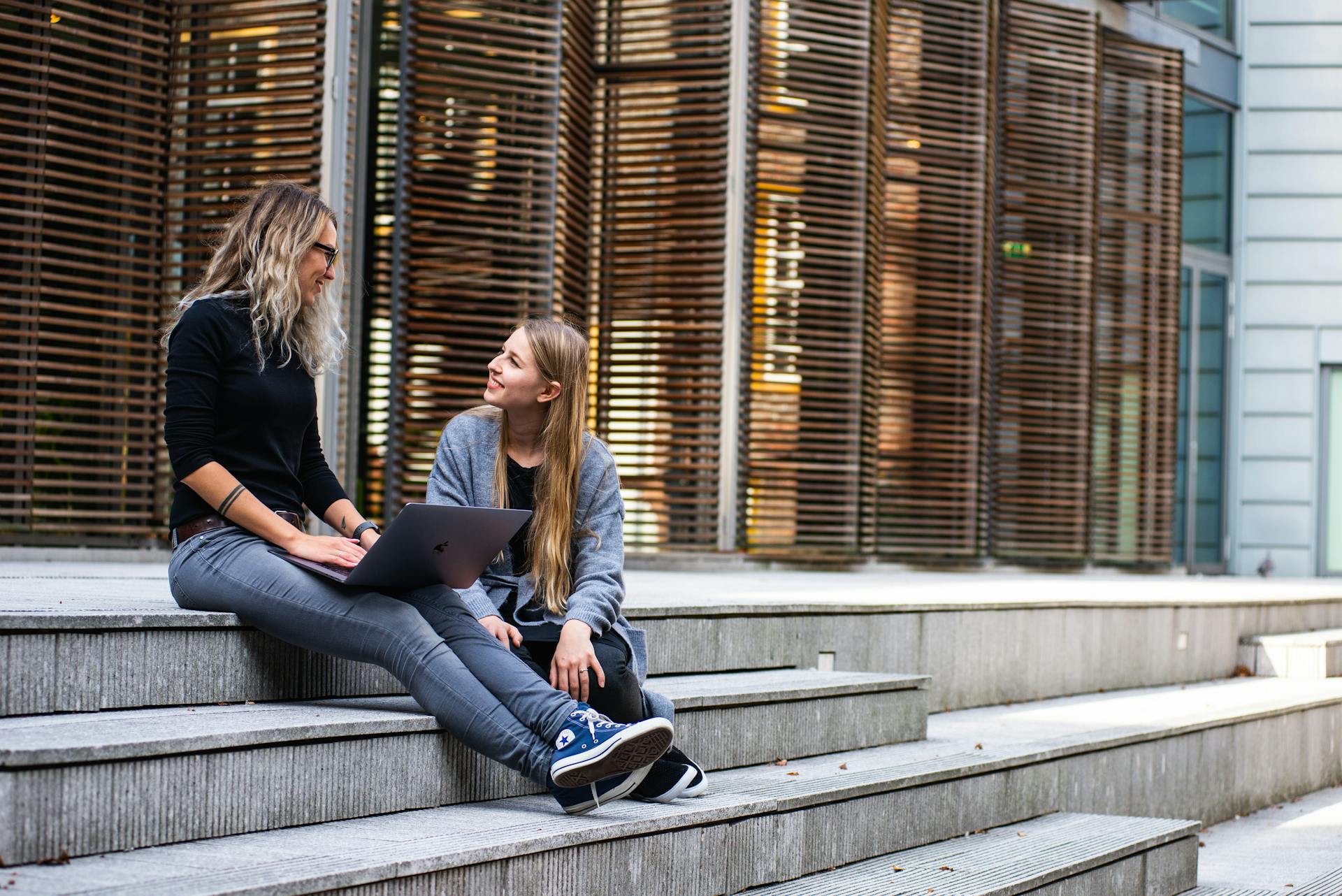
Here's a quick rundown of how both types of AI are trained:
By understanding how these AI systems are trained, we can appreciate the complexity and nuance of their development, and how they're able to generate responses and outputs that feel so human-like.
Functionality and Differences
Conversational AI focuses on human conversations, while generative AI creates content in various forms. The main difference between the two lies in their objectives and goals.
Conversational AI is used for applications such as customer service, virtual assistants, and chatbots. It's trained on large data sets with human input, conversations, user queries, and responses. This training enables conversational AI to understand user intent, context, and maintain dialogue.
Generative AI, on the other hand, is used for tasks like writing works of fiction, marketing content, and meta descriptions. It's trained on different sets of data to learn patterns and create content with predictive patterns.
The primary function of conversational AI is to engage in real-time conversations with users. It responds to user queries and adapts to dynamic user inputs and context changes. In contrast, generative AI creates diverse outputs, including text, images, music, and more, based on learned patterns and structures.
Intriguing read: Chatgpt Openai's Generative Ai Chatbot Can Be Used for
Here's a comparison of the two:
Conversational AI adapts to dynamic user inputs and context changes, while generative AI is versatile in generating various types of content based on input.
Benefits and Limitations
Conversational AI can empower teams to deliver fantastic service across multiple channels 24/7, and it can also help personalize interactions by analyzing previous discussions and real-time sentiment or intent.
Conversational AI can enhance operational efficiency by automating tasks like transcription or translation, and reduce operational costs by boosting agent productivity and reducing workloads.
It can also optimize business insights to help with strategic decision-making and scale endlessly to handle various conversations across numerous channels.
However, conversational AI can struggle with complex linguistic nuances and requests, and may not always understand customer accents or things like humor or sarcasm.
Here are the key benefits of conversational AI:
- Enhance operational efficiency by automating tasks
- Reduce operational costs by boosting agent productivity
- Optimize business insights to help with strategic decision-making
- Scale endlessly to handle various conversations
Generative AI can significantly enhance team productivity and creativity, and guide agents through the process of delivering exceptional customer service by automating repetitive tasks like call summarization.
Generative AI solutions can also simplify the creation of content for training purposes, generate automatic responses to customer queries, and transform customer interactions with personalized insights.
Discover more: Generative Ai for Customer Support
Pros and Cons
Conversational AI can empower teams to deliver fantastic service across multiple channels 24/7, and help personalize interactions by analyzing previous discussions and real-time sentiment or intent.
It can also enhance operational efficiency by automating tasks like transcription or translation, and reduce operational costs by boosting agent productivity and reducing workloads.
Conversational AI can optimize business insights to help with strategic decision-making, and scale endlessly to handle various conversations across numerous channels.
However, conversational AI has limitations, such as struggling with complex linguistic nuances and requests, and not always understanding customer accents or things like humor or sarcasm.
Business leaders need to ensure they have the right security strategies in place to protect sensitive customer data.
Here's a breakdown of the pros and cons of conversational AI:
Generative AI can significantly enhance team productivity and creativity, and guide agents through the process of delivering exceptional customer service.
It can also improve team efficiency by automating repetitive tasks like call summarization, and simplify the creation of content for training purposes.
Generative AI solutions can generate automatic responses to customer queries, transform customer interactions with personalized insights, and respond to a range of types of input, such as images and text.
However, there are risks to generative AI, too, including issues with data governance and AI hallucinations, where not all answers given will be correct.
Generative AI relies on access to large volumes of data, which needs to be protected for compliance purposes.
Related reading: Generative Ai for Customer Experience
Benefits of
Conversational AI offers numerous benefits, including being available 24/7 to answer customer questions and provide support at any time. This is especially helpful for businesses operating globally.
Chatbots powered by conversational AI can handle a high volume of inquiries simultaneously, freeing up customer service agents for more complex issues. This means customers get immediate support, without having to wait on hold or for an agent to pick up.
Having used conversational AI in customer service, I can attest to its efficiency in resolving issues quickly. By automating routine inquiries, customer service agents can focus on more critical tasks.
Here are some key benefits of conversational AI:
- Availability: 24/7 support for customers
- Efficiency: Handling a high volume of inquiries simultaneously
- Faster time to resolution: Immediate support for customers
Generative AI also offers several benefits, including enhanced creativity for artists, designers, and researchers. It can spark new ideas and generate variations on existing concepts.
Generative AI can automate repetitive tasks like image editing or content creation, freeing up human time and resources for more strategic tasks. This can lead to increased productivity and efficiency in various industries.
Generative AI can also personalize experiences by tailoring content or products to user preferences. This can lead to greater engagement and satisfaction among users.
Worth a look: Generative Ai Content
Limitations of
Conversational AI can be prone to misunderstandings due to dialect and language differences or emotional components in a message if it wasn't trained for that.
Misunderstandings can lead to frustration for both users and businesses, so it's essential to have a human-in-the-loop to ensure accurate and effective communication.
Conversational AI may not be able to handle every situation and may not be able to resolve complex customer service issues.
This limitation is crucial to consider when implementing conversational AI solutions, especially in high-stakes industries like finance or healthcare.
Conversational AI interactions often involve collecting data, which raises privacy concerns for some users.
Data collection can be a sensitive topic, and it's essential to be transparent about how user data is being used and protected.
Here are some key limitations of conversational AI:
- Misunderstandings
- Limited capabilities
- Privacy concerns
Generative AI can also be flawed, with issues around copyright, authenticity, and the ethics of creating deepfakes becoming more pressing as AI-generated content becomes more sophisticated.
AI hallucinations can occur when an AI model creates false or illogical outputs that aren't based on any real data but presents the information as fact.
Data privacy and security are also major concerns, as generative AI relies on vast amounts of data, raising concerns about privacy breaches and the misuse of sensitive information used to train the models.
To mitigate these risks, it's essential to keep a human-in-the-loop to ensure the outputs of your AI solution are accurate, ethical, and in line with your brand.
Sources
- https://medium.com/@social_65128/differences-between-conversational-ai-and-generative-ai-e3adca2a8e9a
- https://pwskills.com/blog/conversational-ai-vs-generative-ai-whats-the-difference/
- https://www.techtarget.com/whatis/feature/Conversational-AI-vs-generative-AI-Whats-the-difference
- https://www.cxtoday.com/contact-centre/conversational-ai-vs-generative-ai-which-is-best-for-cx/
- https://www.infobip.com/blog/conversational-ai-vs-generative-ai
Featured Images: pexels.com