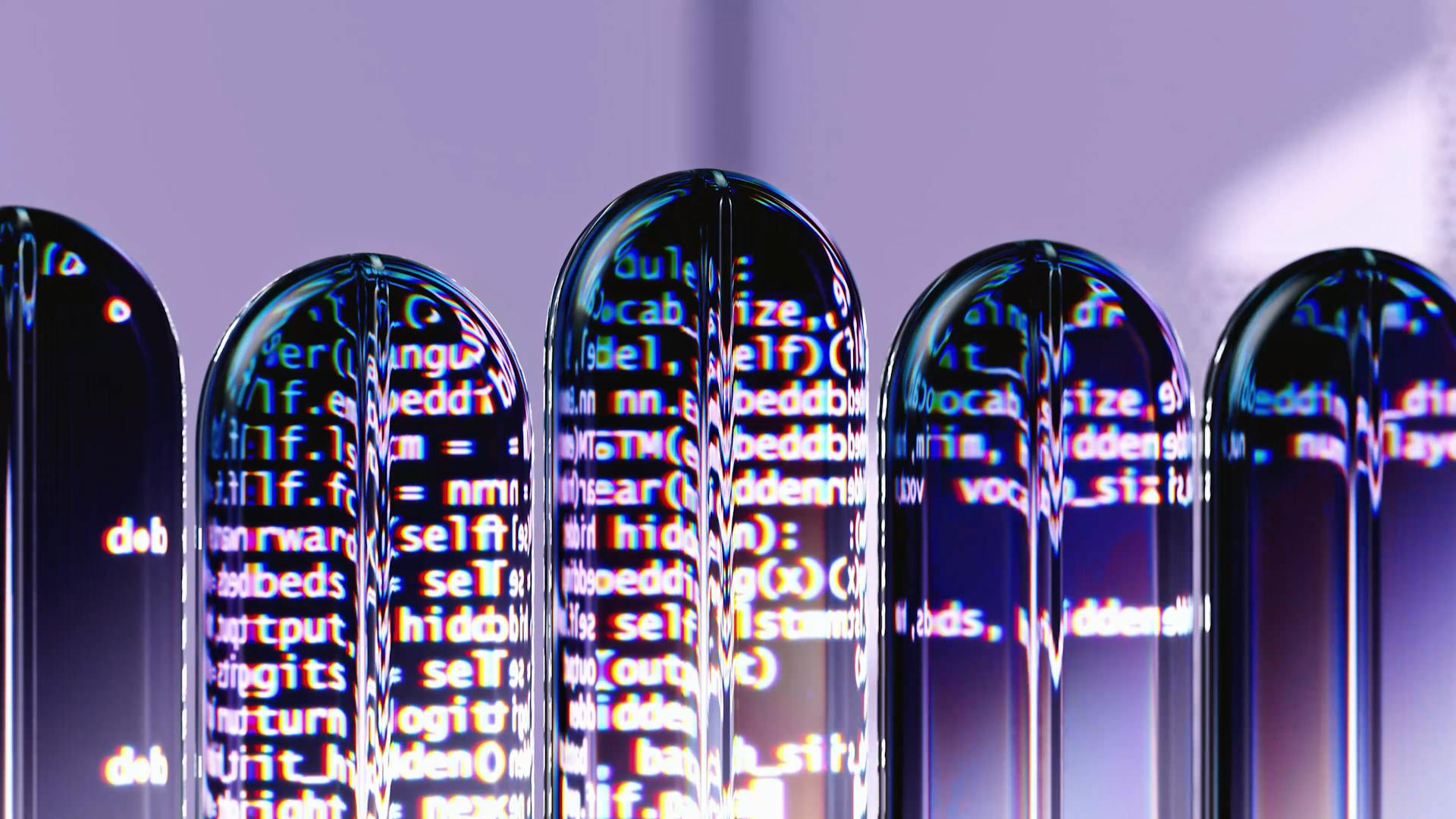
Controlling the output of generative AI systems is crucial because it can prevent the spread of misinformation.
Generative AI systems can produce a wide range of content, from text to images, that can be misleading or false. For example, as noted in the article, a generative model can create fake news articles that are almost indistinguishable from real ones. This can have serious consequences, such as eroding trust in institutions and fueling social unrest.
The lack of control over generative AI output can also lead to biased or discriminatory content. For instance, a model trained on biased data can produce results that perpetuate existing social inequalities, as seen in the article's discussion of facial recognition AI.
By controlling the output of generative AI systems, developers can ensure that the content produced is accurate, unbiased, and respectful. This requires implementing robust content moderation systems and monitoring the performance of these systems to prevent errors.
Here's an interesting read: Generative Ai for Content Creation
Human-Centered Design Approach
A human-centered design approach is crucial when it comes to controlling the output of generative AI systems. By putting the user at the forefront of the design process, you can ensure that your system is fair, transparent, and safe.
To design features with appropriate disclosures built-in, clarity and control are crucial to a good user experience. This means being transparent about how the system works, what data it uses, and what the user can expect from the output.
Consider augmentation and assistance, where the system provides a few options to the user rather than a single answer. This can be particularly useful in cases where there is a high probability that the answer satisfies a diversity of users and use cases.
Model potential adverse feedback early in the design process, followed by specific live testing and iteration for a small fraction of traffic before full deployment. This will help you identify and fix any issues before they become major problems.
Expand your knowledge: Generative Ai Use Cases in Financial Services
Engage with a diverse set of users and use-case scenarios, and incorporate feedback before and throughout project development. This will build a rich variety of user perspectives into the project and increase the number of people who benefit from the technology.
Here are some key principles to keep in mind when taking a human-centered design approach:
- Design features with appropriate disclosures built-in.
- Consider augmentation and assistance.
- Model potential adverse feedback.
- Engage with diverse users and use-case scenarios.
- Incporporate feedback throughout project development.
By following these principles, you can create a generative AI system that is fair, transparent, and safe for users.
Assessing and Mitigating Impacts
Assessing and Mitigating Impacts is crucial when it comes to controlling the output of generative AI systems. This involves systematically looking at the possible impacts of your generative AI on relevant stakeholders.
To do this, make risk matrices analyzing the likelihood and seriousness of harm, considering areas such as fairness and bias, transparency, safety, privacy, and accountability. This will help identify potential issues and show areas needing more ethical safeguards.
Key areas to assess include fairness and bias, transparency, safety, privacy, and accountability. For example, check training data for representation of demographics and subgroups to prevent encoded social biases.
Here's a breakdown of the key areas to assess:
- Fairness and bias
- Transparency
- Safety
- Privacy
- Accountability
By understanding the potential impacts and taking steps to mitigate them, you can ensure that your generative AI system is developed and implemented in a safe, ethical, and sustainable manner.
Conduct Impact Assessments
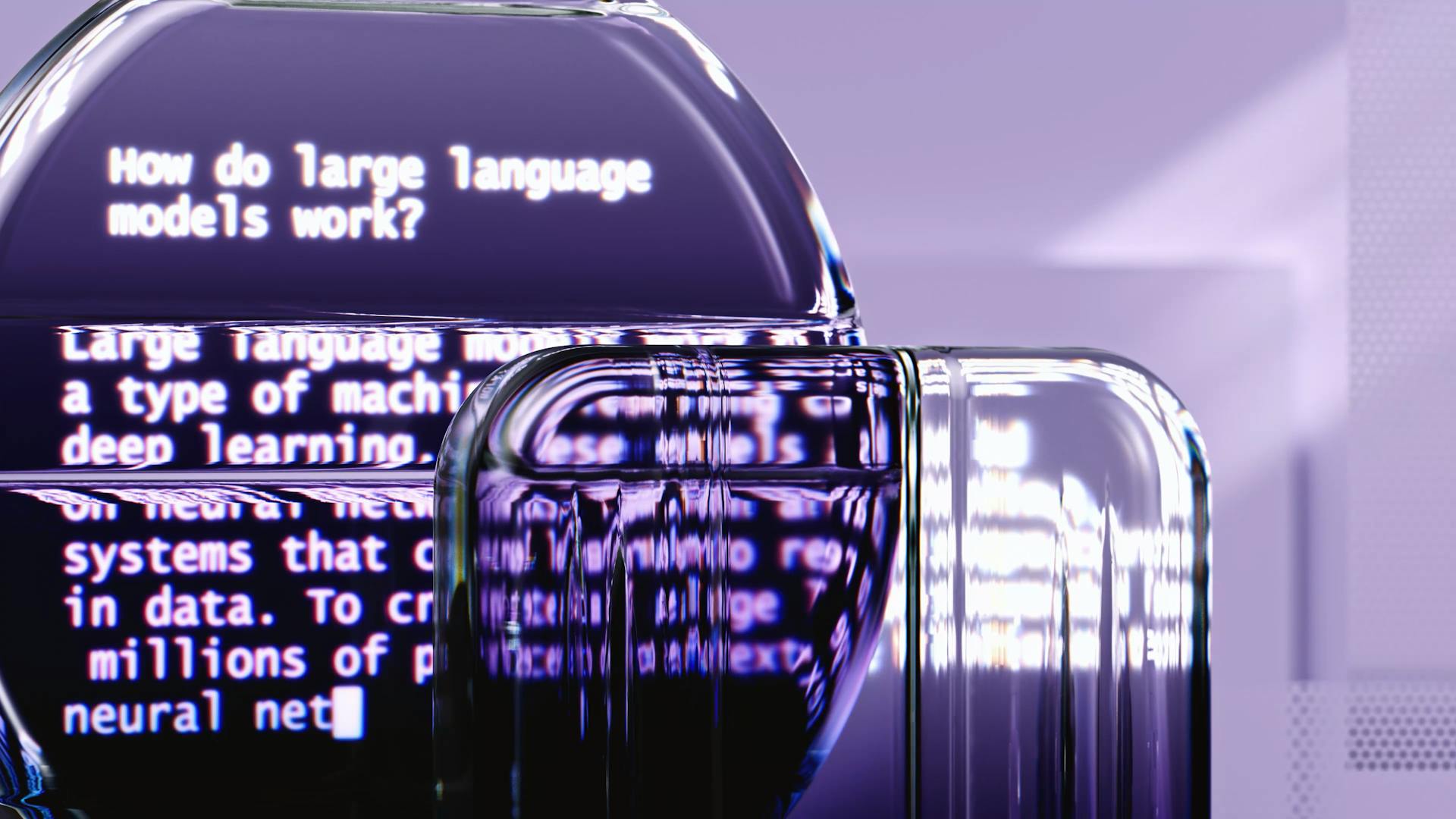
Conducting impact assessments is a crucial step in ensuring that generative AI systems are developed and implemented in a safe and ethical manner. You need to systematically look at the possible impacts of your generative AI on relevant stakeholders.
Some key areas to assess include fairness and bias, transparency, safety, privacy, and accountability. Fairness and bias involve checking training data for representation of demographics and subgroups, and looking for encoded social biases. Transparency requires ensuring explainability, so people can understand how the system works and makes decisions.
Safety assessments should consider potential misuse, while privacy checks should analyze data practices from start to finish. Accountability involves determining who is responsible if things go wrong, and having plans to monitor outputs and fix problems.
To conduct a comprehensive impact review, consider both direct and indirect consequences of using generative AI systems. Direct impacts result from specific AI outputs, such as biased or harmful content, while indirect impacts come from how the existence of AI changes societal dynamics.
Worth a look: Impact of Generative Ai
Here are some key areas to assess in more detail:
- Fairness and bias
- Transparency
- Safety
- Privacy
- Accountability
Keep assessing and discussing risks among stakeholders to identify areas needing more ethical safeguards. This will help you create a comprehensive risk estimation framework and ensure that your generative AI system is developed and implemented in a safe and ethical manner.
If this caught your attention, see: Ethical Considerations for Generative Ai Use in Business
Multiple Metrics for Training Assessment
Assessing the performance of a machine learning model is crucial to ensure it's working as intended. Consider using multiple metrics to get a comprehensive understanding of its strengths and weaknesses.
Using a single metric can be misleading, as it may only capture a narrow aspect of the model's performance. For example, a fire alarm system should prioritize high recall, even if it means occasionally triggering a false alarm.
To get a more accurate picture, consider metrics like feedback from user surveys, click-through rates, and customer lifetime value. These metrics can help you understand tradeoffs between different kinds of errors and experiences.
Explore further: Generative Ai in Performance Testing
Here are some key metrics to consider:
- Feedback from user surveys
- Click-through rates
- Customer lifetime value
- False positive and false negative rates sliced across different subgroups
Ultimately, the metrics you choose should be tailored to the specific context and goals of your system. By using a combination of metrics, you can get a more nuanced understanding of your model's performance and make informed decisions about how to improve it.
Prioritize Fairness and Inclusion
Generative AI risks perpetuating historical patterns of unfairness present in human systems. This is because representation in training data impacts whose voices are heard by AI systems, making it crucial to make intentional efforts to counter exclusion and promote equitable access.
To ensure fairness in generative AI outputs, consider filtering the training data and using diverse and accurate sources. Human oversight and post-processing reviews are also critical in ensuring that AI-generated content meets ethical and legal standards.
Engineer controls are essential in preventing biased outputs. This involves choosing datasets carefully, considering representation, consent, and rights, and removing misleading correlations that encode bias.
On a similar theme: How Generative Ai Can Augment Human Creativity
Key controls to engineer for responsible use include training data selection, model design, outputs checking, release control, monitoring, human oversight, and access controls. These controls should be evaluated and updated regularly to ensure ongoing fairness and inclusion.
Techniques like adversarial debiasing of embeddings, reweighting underrepresented groups, and subgroup validation can help detect and mitigate bias in AI models. Additionally, monitoring outputs for disparate impact and modifying problematic elements of datasets and models is crucial.
Here are some key principles to keep in mind when prioritizing fairness and inclusion in generative AI systems:
- Choose datasets carefully, considering representation, consent, and rights.
- Build interpretability and transparency into the model.
- Check outputs before release for safety, fairness, and alignment with values.
- Use diverse and accurate sources in training data.
- Implement real-time moderation systems to ensure fairness in generative AI outputs.
By following these principles and regularly evaluating and updating our controls, we can create generative AI systems that promote fairness, inclusion, and equity.
Enable Transparency
Enabling transparency is crucial in controlling the output of generative AI systems. This involves making the inner workings of these systems understandable to users and stakeholders.
Engineers should strive to design generative models for interpretability, where possible. Techniques like attention layers, partial dependence plots, and LIME can help shed light on the reasoning behind the AI's decisions.
To achieve transparency, it's essential to document training data characteristics, model details, evaluation metrics, and human oversight controls thoroughly. This information should be published in transparency reports on practices.
Users should be able to ask why specific outputs were generated and trace back the factors that influenced the generative process. This can be achieved by enabling ways for users to ask for explanations and by making AI decision-making processes understandable.
Here are some key considerations for achieving transparency:
- Document training data characteristics, model details, evaluation metrics, and human oversight controls thoroughly.
- Publish transparency reports on practices.
- Enable ways for users to ask why specific outputs were generated and trace back factors that influenced the generative process.
By prioritizing transparency, we can build trust in AI systems and ensure that they are used responsibly.
Testing and Evaluation
Testing and Evaluation are crucial steps in controlling the output of generative AI systems. Rigorous unit tests should be conducted to test each component of the system in isolation.
Conducting integration tests is also essential to understand how individual ML components interact with other parts of the overall system. This helps ensure that the system is working as intended.
Proactively detecting input drift is vital to prevent the system from behaving unexpectedly. This can be done by testing the statistics of the inputs to the AI system.
A gold standard dataset should be used to test the system and ensure that it continues to behave as expected. This dataset should be updated regularly in line with changing users and use cases.
Here are some key testing strategies to keep in mind:
- Conduct unit tests to test each component of the system in isolation.
- Conduct integration tests to understand how individual ML components interact with other parts of the overall system.
- Proactively detect input drift by testing the statistics of the inputs to the AI system.
- Use a gold standard dataset to test the system and ensure that it continues to behave as expected.
- Conduct iterative user testing to incorporate a diverse set of users’ needs in the development cycles.
Fairness in datasets is also crucial, which includes identifying representation and corresponding limitations, as well as identifying prejudicial or discriminatory correlations between features, labels, and groups.
Monitoring and Updating
Monitoring and updating generative AI systems is crucial to ensure their outputs align with societal values and legal standards. This involves continuous monitoring and adaptation to emerging issues and sensitivities.
Language evolves constantly, and staying up-to-date on emerging issues and sensitivities is essential. This means adopting terms preferred by specific communities and avoiding words that become charged or obsolete.
Consider reading: Legal Implications of Ai
To achieve this, consider the following steps:
- Build time into your product roadmap to address issues that will inevitably occur.
- Balance short-term simple fixes with longer-term learned solutions.
- Analyze how candidate and deployed models differ, and how updates will affect the overall system quality and user experience.
By incorporating these steps into your monitoring and updating process, you can ensure that your generative AI system produces high-quality outputs that meet ethical and legal standards.
Monitor Language Trends
Language is constantly evolving, and it's essential to stay up to date on emerging issues and sensitivities.
Adopting terms preferred by specific communities is crucial, as seen in the example of Project Q-Star, where understanding the complexities of language is vital.
Avoiding words that become charged or obsolete is essential, as it can have significant consequences, like the example of Project Q-Star shows.
Changes in language trends over time require adapting criteria to ensure that language remains inclusive and respectful.
Language trends can shift rapidly, making it necessary to regularly review and update language usage to avoid unintended offense or misunderstanding.
A fresh viewpoint: Generative Ai Trends
Monitor and Update System
Monitoring your system after deployment is crucial to ensure it performs well in the real world. Issues will occur, so it's essential to build time into your product roadmap to address them.
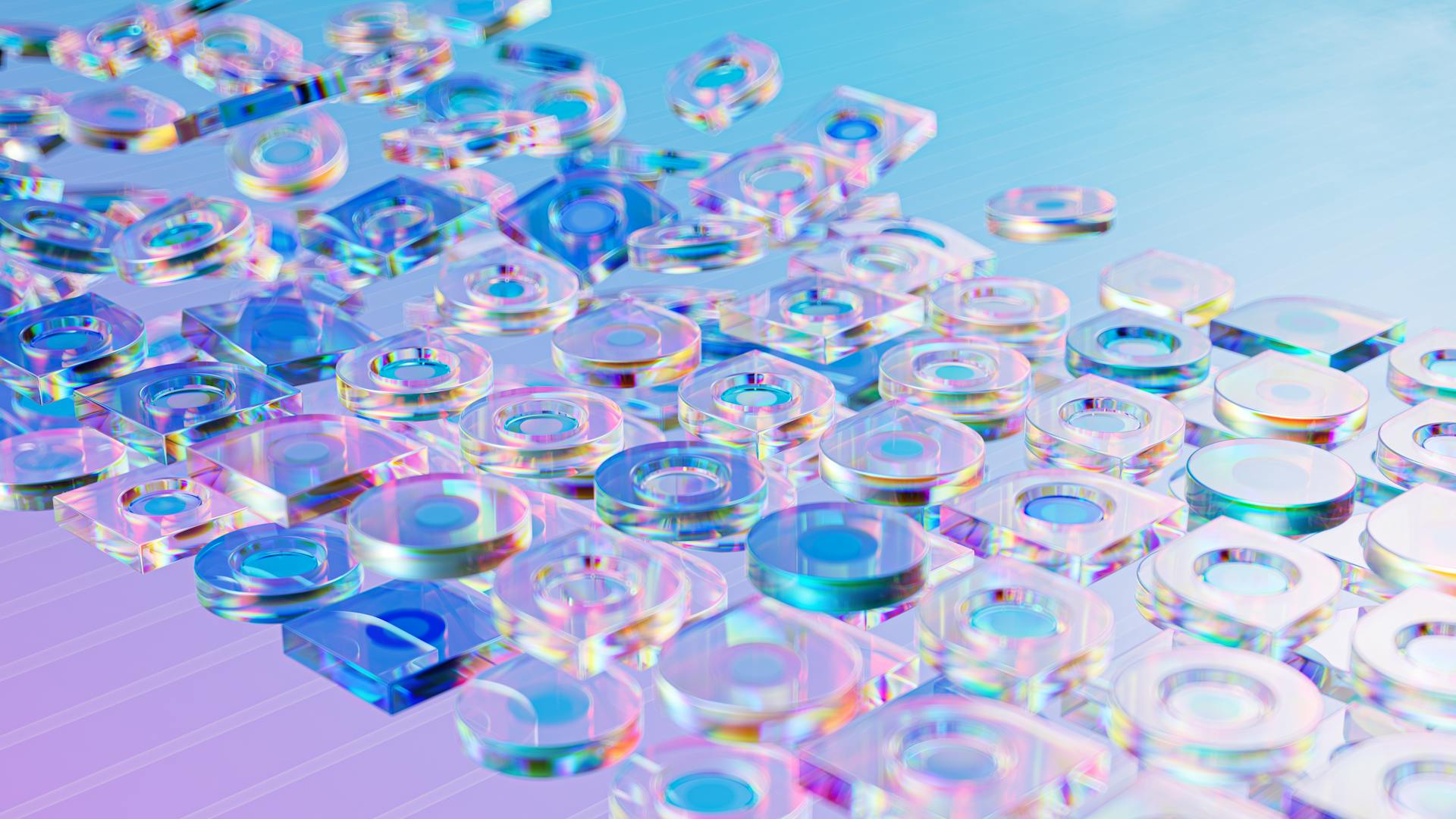
You should consider both short- and long-term solutions to issues. A simple fix like blocklisting may help solve a problem quickly, but it may not be the optimal solution in the long run. It's essential to balance short-term simple fixes with longer-term learned solutions.
Before updating a deployed model, analyze how the candidate and deployed models differ, and how the update will affect the overall system quality and user experience. This will help you make informed decisions and avoid disrupting your system.
Here are some key things to consider when monitoring and updating your system:
- Issues will occur, so plan for them
- Consider both short- and long-term solutions
- Analyze the differences between candidate and deployed models
- Balance short-term fixes with long-term solutions
By following these steps, you can ensure your system continues to perform well and meets the needs of your users.
Enable User Consent
To ensure that users trust generative AI systems, developers must focus on giving users control and getting their permission. This is crucial for building trust in AI systems.
Developers should make sure using AI feels good for users and follow ethical rules for handling data. Giving users a sense of control and agency over the AI system is key to building trust.
Additional reading: Building Generative Ai Applications with Gradio
It's also essential to make AI usable for everyone, no matter their abilities, backgrounds, or identities. Developers should design AI in a way that includes everyone, considering factors like different languages and cultures.
By doing this, developers can reduce unfairness and make sure everyone can benefit from AI. This is a matter of fairness and equal access to technology.
Users also play a role in controlling the output of generative AI systems. They can guide the AI toward creating responsible content by giving it clear and ethical prompts.
Educating users on how to interact with AI responsibly is another layer of control. This is essential for preventing users from creating harmful outputs, such as offensive or misleading content.
In some cases, AI can create harmful content, such as deepfake videos that mislead people by making fake videos that look real. Controlling AI outputs ensures that users are not exposed to dangerous or deceptive content.
Developers should prioritize user safety and take steps to prevent the creation of harmful content. This includes implementing safeguards to prevent the misuse of AI.
See what others are reading: Generative Ai for Everyone
Risks of
Controlling the output of generative AI systems is crucial to prevent the spread of misinformation. Uncontrolled AI systems can unintentionally generate false or misleading content, increasing the risk of misinformation and confusion.
The risks of uncontrolled generative AI outputs are significant, and they include spreading misinformation, security threats, and legal and financial liabilities. Malicious actors can exploit AI outputs to create harmful content, such as phishing emails or scam websites.
Here are some of the risks associated with uncontrolled generative AI outputs:
- Spreading Misinform
Uncontrolled AI systems can unintentionally generate false or misleading content.Security Threats
Malicious actors can exploit AI outputs to create harmful content.Legal and Financial Liabilities
Organizations failing to manage AI outputs may face legal repercussions.
The rapid pace of AI development often outstrips legal and ethical guidelines, making it even more challenging to control AI outputs. Cultural differences also add complexity to the design of AI systems, as fairness can be subjective across cultures.
A unique perspective: Generative Ai Content
Government Regulations
Government regulations are playing a crucial role in controlling the output of generative AI systems. Governments are creating rules to ensure AI is used ethically and safely, and controlling AI outputs is essential to complying with these rules and avoiding legal penalties.
These regulations are necessary because AI can unintentionally generate content that violates intellectual property rights, such as recreating a copyrighted song or image. This can put both the user and the creators of the AI at risk of lawsuits.
In fact, generative AI systems can create content that violates laws, like copying someone else's work or breaking privacy rules. Without control, these systems could accidentally steal intellectual property or violate data protection laws, leading to legal trouble.
To tackle these challenges, governments and organizations must consider how to manage AI systems in a way that complies with the law. Intelligent control systems can help monitor and regulate automated AI outputs, acting as a safety net to ensure AI behaves in ways that align with societal values and legal standards.
Suggestion: Generative Ai Regulation
Here are some key benefits of intelligent control systems:
- Filtering training data to remove biased, outdated, or harmful information
- Monitoring outputs in real-time to flag or remove problematic content
- Post-processing oversight by human reviewers to ensure outputs meet ethical and legal standards
By controlling the output of generative AI systems, we can ensure that AI-generated outputs are reliable, consistent, and free from errors, reducing the risks of misinformation or harmful consequences.
Sources
- https://www.aegissofttech.com/insights/ethical-thinking-into-generative-ai/
- https://ai.google/responsibility/responsible-ai-practices/
- https://techwhistle.tech/why-is-controlling-the-output-of-generative-ai-systems-important/
- https://riziai.com/why-is-controlling-the-output-of-generative-ai-systems-important/
- https://www.linkedin.com/pulse/why-controlling-output-generative-ai-systems-important-nueravi-ai-hvpze
Featured Images: pexels.com