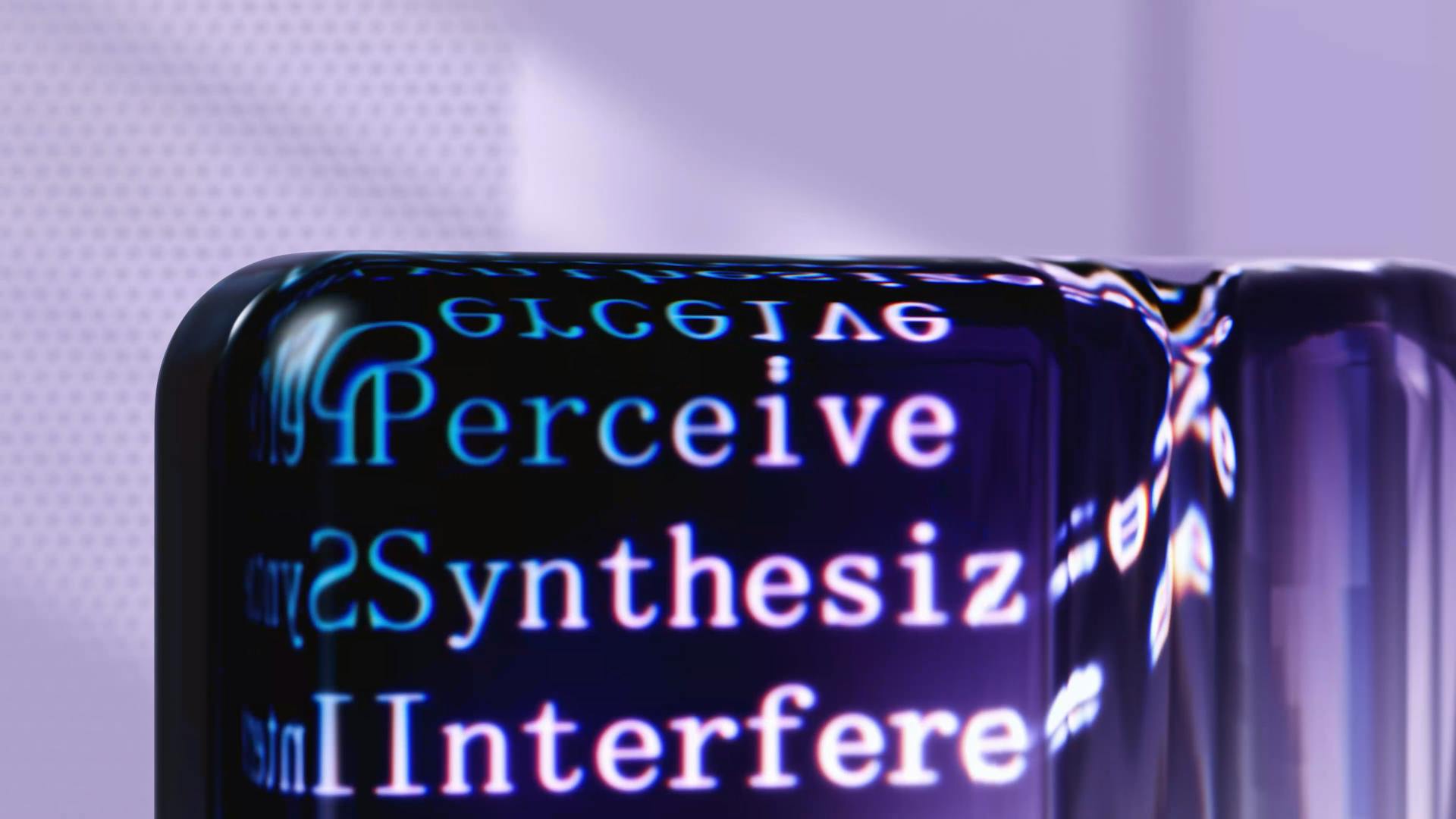
Generative AI has the potential to revolutionize business operations, but it's essential to consider the ethical implications of its use.
Data bias is a significant concern, as AI models can perpetuate existing biases if trained on biased data. This can lead to unfair outcomes and damage to a company's reputation.
To mitigate this risk, businesses must implement robust data curation and validation processes. Regular audits can help identify and address bias in AI decision-making systems.
The consequences of ignoring data bias can be severe, including financial losses, legal repercussions, and damage to customer trust.
For another approach, see: Bias in Generative Ai
Content Risks
Generative AI systems can create content automatically, but this can also lead to harm, either intentional or unintentional. For example, an AI-generated email sent on behalf of a company could inadvertently contain offensive language or issue harmful guidance to employees.
Discriminatory content or content that promotes violence or misinformation can be harmful to your audience. Without careful monitoring, there is a risk that this type of information could be disseminated by generative AI and negatively impact ostracized individuals or communities.
A unique perspective: Generative Ai for Content Creation
To mitigate these risks, it's essential to follow best practices, such as defining a purpose for the content, providing explicit instructions to AI models with guardrails and constraints, and following global guidelines and standards.
- Define a purpose for the content to align content creation with organizational goals.
- Input clear instructions with guardrails and constraints to prevent the generation of biased or discriminatory content.
- Follow global guidelines and standards to maintain ethical standards.
- Use diverse data input methods and sources to reduce the risk of reinforcing existing biases.
- Monitor and evaluate output for accuracy and identify potential ethical issues.
Generated Content Concerns
Generated content can be a double-edged sword. On one hand, it can greatly improve productivity, but on the other hand, it can also be used for harm, either intentionally or unintentionally.
Generative AI systems can create enormous amounts of content automatically, but they can also inadvertently contain offensive language or issue harmful guidance. This is why it's essential to use AI-generated content to augment, not replace humans or processes.
AI-generated content can be particularly problematic if it's not carefully monitored. For example, if an AI-generated email contains offensive language, it could create disharmony within an organization.
To mitigate the risk of generating harmful or inappropriate material, it's crucial to define a purpose for the content and provide explicit instructions to AI models, along with well-defined guardrails and constraints.
Curious to learn more? Check out: Generative Ai Not Working Photoshop
Here are some key considerations to keep in mind:
By following these best practices, you can ensure that your AI-generated content is accurate, relevant, and free from harm.
Optimize Your Content
The generative AI market is set to grow to $1.3 trillion over the next 10 years, but this growth comes with content risks that need to be addressed.
Defining a purpose for the content is the first step in creating AI content that maintains high ethical standards. This helps mitigate the risk of generating harmful or inappropriate material by aligning content creation with organizational goals.
To prevent biased or discriminatory content, provide explicit instructions to AI models, along with well-defined guardrails and constraints. Generative AI tools can only produce results as good as the given prompts.
Following global guidelines and standards is essential to maintaining ethical standards. Many governments and companies have created guidelines and policies about using AI-created content, and it's crucial to follow these guidelines.
You might like: Generative Ai Content
Using diverse data input methods and sources can help reduce the risk of reinforcing existing biases in AI models. This can be achieved by ensuring that input methods and sources for training algorithms are as diverse as possible.
Regularly monitoring and evaluating the output of AI-generated content is critical for ensuring accuracy and identifying potential ethical issues. This can be done by consulting subject matter experts and incorporating quality control processes, such as multiple review stages, to double or triple-check for accuracy, originality, and adherence to ethical standards.
Discover more: What Is One Challenge in Ensuring Fairness in Generative Ai
Copyright and Liability
Using generative AI tools in business can be a double-edged sword. On one hand, it can save time and increase efficiency, but on the other hand, it can also lead to significant legal and reputational risks.
Popular generative AI tools are trained on massive databases from multiple sources, including the internet, which can make it difficult to track the origin of the data. This can be problematic for companies handling sensitive information.
Expand your knowledge: What Are the Generative Ai Tools
Companies must validate outputs from the models to avoid legal challenges. Until legal precedents provide clarity around IP and copyright challenges, it's essential to be cautious.
Intellectual property infringements can result in costly legal battles and reputational damage. Consider the music industry, where a generative AI's music piece closely resembling an artist's copyrighted song could lead to costly lawsuits and public backlash.
The ownership of AI-generated content is a significant ethical concern. Who owns the content, the company using it or the company that owns the generative AI tool that created it? Ambiguity can lead to potential legal exposure for individuals or organizations that publish it without proper authorization.
Artificial intelligence is meant to augment, not replace, humans. If not properly guided and supervised, AI systems may inadvertently reproduce existing content without proper attribution, undermining the principles of intellectual property and fair use.
Additional reading: Generative Ai Music Free
Data Security
Data security is a top priority for businesses using generative AI. Companies should lean towards anonymizing data when training models to minimize the risk of unauthorized use or misuse.
A breach of user privacy or data misuse can trigger legal consequences and erode user trust. GDPR's data minimization principle suggests that only necessary data should be processed.
Personal customer information used to create AI content can be an ethical problem, particularly concerning data privacy regulations. Companies should adopt similar principles to GDPR, ensuring any non-essential personal data is stripped away before training.
Robust encryption methods should be employed during data storage to protect user data. This ensures that even if a breach occurs, the data remains uncompromised.
Companies need to ensure they have proper user data handling and consent management guidelines in place. This will help safeguard privacy rights and prevent potential HIPAA violations.
Readers also liked: Top Generative Ai Companies
Bias and Fairness
Bias and Fairness is a critical consideration for businesses using Generative AI. AI models can mirror the data they're fed, perpetuating biases if they're trained on biased datasets.
To prevent this, prioritize diversity in training datasets and commit to periodic audits to check for unintended biases. Organizations like OpenAI emphasize the importance of diverse training data.
Generative AI tools are only as good as the data used to train the algorithms, and unfortunately, AI models may inadvertently amplify biases present in the data they were trained on. This can neglect or be discriminatory towards diverse groups or reinforce societal biases that aren’t necessarily true.
Discover more: Pre Trained Multi Task Generative Ai
Lack of Explainability
Generative AI systems often group facts together probabilistically, but these details aren't always revealed, which raises questions about data trustworthiness.
This lack of explainability is a major concern because it prevents us from understanding why a particular answer was given. Machine learning models and generative AI search for correlations, not causality, which means we can't always trust the outcome.
To build trust in generative AI, we need model interpretability, where we can see the reason behind the answer. This is crucial when the answer could significantly affect lives and livelihoods.
Unfortunately, many generative AI systems don't provide this level of transparency, which is why we need to be cautious when relying on them for critical information.
You might like: When Was Generative Ai Open Source
Bias
Bias can be a major issue with generative AI, and it's essential to understand where it comes from. Generative AI can potentially amplify existing biases found in data used for training language models.
If a dataset is biased, the AI model will learn from it and perpetuate those biases. This can lead to AI models that inadvertently discriminate against certain groups of people.
Companies working on AI need to have diverse leaders and subject matter experts to help identify unconscious bias in data and models. This can prevent AI models from mirroring the biases present in the data they're fed.
AI that perpetuates societal biases can draw public ire, legal repercussions, and brand damage. Facial recognition software is a prime example of this, as it can wrongly identify individuals based on biased data.
Prioritizing diversity in training datasets is crucial to preventing bias. Organizations like OpenAI emphasize the importance of diverse training data, and companies should initiate partnerships with such organizations to ensure rigorous bias checks and external audits.
Generative AI tools are only as good as the data used to train the algorithms, and AI models can amplify biases present in the data they were trained on. This can neglect or be discriminatory towards diverse groups or reinforce societal biases that aren’t necessarily true.
Suggestion: Telltale Words Identify Generative Ai Text
Explainability
Explainability is a crucial aspect of AI systems, ensuring users understand how they make decisions and providing explanations when requested.
This means that AI systems should be transparent and provide clear insights into their decision-making processes. In cases where fully explainable AI algorithms aren't possible, systems should offer a way to interpret results, enabling users to grasp cause and effect.
AI systems can't always provide explicit explanations, but they should still make their results understandable. This is an essential step towards building trust and ensuring fairness in AI decision-making.
A fresh viewpoint: How Are Modern Generative Ai Systems Improving User Interaction
Workforce and Governance
As generative AI transforms the workplace, it's essential to consider the impact on workforce roles and morale. AI can take over many daily tasks, including writing, coding, and content creation, which may lead to worker displacement and replacement.
The pace of change has accelerated with the innovations in generative AI technologies, and companies are investing in preparing employees for the new roles created by these applications. This includes developing generative AI skills such as prompt engineering.
Businesses need to invest in their workforce to minimize the negative impacts of generative AI and prepare for growth. This involves implementing a robust ethical AI governance framework to ensure responsible use of generative AI.
Human oversight is also crucial to ensure AI systems are behaving as expected and making decisions that align with human values.
On a similar theme: How Generative Ai Can Augment Human Creativity
Workforce Roles
As AI takes on more daily tasks, workforce roles are changing at an accelerated pace. The future of work itself is changing, with companies investing in preparing employees for new roles created by generative AI applications.
Generative AI can do a lot more of the daily tasks that knowledge workers do, including writing, coding, content creation, summarization, and analysis. This is presenting both unprecedented opportunities and significant ethical challenges.
Businesses need to help employees develop generative AI skills such as prompt engineering to prepare for the new roles. The truly existential ethical challenge for adoption of generative AI is its impact on organizational design, work, and ultimately on individual workers.
Investing in workforce development is crucial to minimize the negative impacts of AI on work. This will not only prepare companies for growth but also help them adapt to the changing workforce landscape.
For another approach, see: How Generative Ai Is Changing Creative Work
Human Oversight
Human oversight is crucial in ensuring that AI systems are behaving as expected and making decisions that align with human values. Organizations need to have humans in the loop to monitor and correct AI-driven decisions.
Having humans in the loop also means following laws, regulations, or company policies. This is essential to prevent AI systems from making decisions that are against the law or company guidelines.
Responsibility is also important, which means taking responsibility for the actions of AI systems and being accountable for any negative impacts they may have. This is crucial in preventing brand damage and maintaining trust with customers and employees.
Clear policies and guidelines are necessary to establish accountability and prevent finger-pointing in case of a mishap. This can include feedback loops where users can report questionable outputs, which can be invaluable in maintaining accountability.
Ultimately, implementing human oversight and accountability structures is essential in ensuring the responsible use of generative AI in the workplace.
Related reading: Can Generative Ai Replace Humans
Sources
- https://www.techtarget.com/searchenterpriseai/tip/Generative-AI-ethics-8-biggest-concerns
- https://www.forbes.com/councils/forbestechcouncil/2023/10/17/which-ethical-implications-of-generative-ai-should-companies-focus-on/
- https://www.cognilytica.com/top-10-ethical-considerations-for-ai-projects/
- https://contentbloom.com/blog/ethical-considerations-in-ai-generated-content-creation/
- https://www.linkedin.com/pulse/ethical-considerations-responsible-use-generative-ai-workplace-6otdc
Featured Images: pexels.com