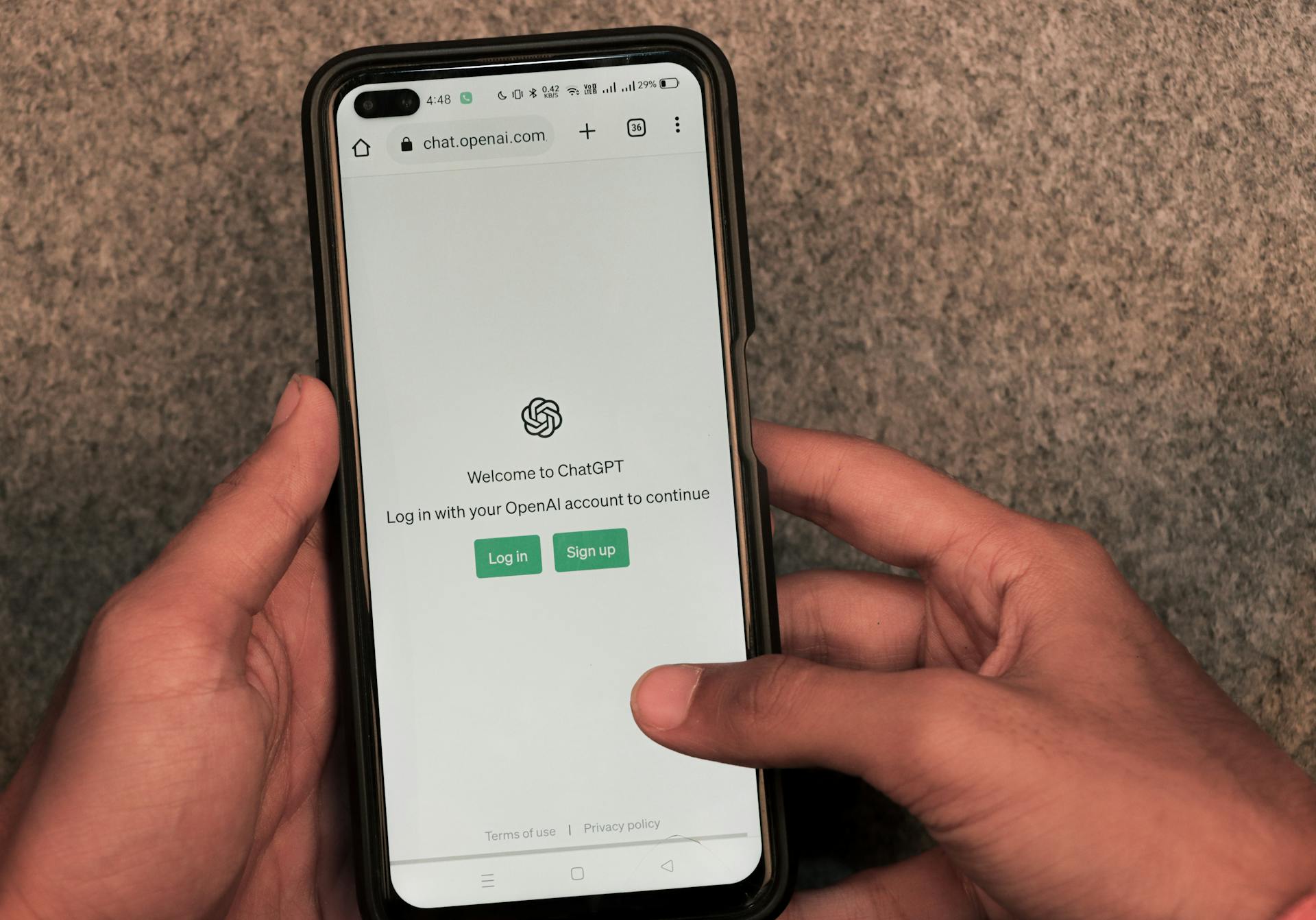
ChatGPT, an OpenAI generative AI chatbot, is a game-changer for businesses and educational institutions. It can be used to automate customer support, freeing up human resources for more complex tasks.
By integrating ChatGPT into their operations, companies can provide 24/7 support to their customers, improving response times and overall customer satisfaction. This can lead to increased customer loyalty and retention.
ChatGPT can also be used to create personalized learning experiences for students, allowing them to learn at their own pace and in their own style. This can be especially beneficial for students with learning disabilities or those who need extra support.
The chatbot's ability to process and respond to natural language inputs makes it an ideal tool for educational settings, where students often have questions and need help outside of traditional classroom hours.
You might like: How Is Ai Used in Robotics
What ChatGPT Can Do
ChatGPT can produce a wide variety of credible writing in seconds, making it a game-changer for businesses that need to produce clear written materials.
Generative AI tools like ChatGPT can respond to criticism to make the writing more fit for purpose, saving time and resources for organizations.
You can use ChatGPT to generate code that's largely correct and instantaneous, which is perfect for IT and software organizations.
ChatGPT can also be used to create more technical materials, such as higher-resolution versions of medical images.
With the time and resources saved, organizations can pursue new business opportunities and create more value.
ChatGPT can be fine-tuned to perform a specific task, like preparing slides according to a specific style, by "learning" how headlines are normally written based on the data in the slides.
On a similar theme: Create with Confidence Using Generative Ai
How ChatGPT Works
ChatGPT is a large language model trained on a massive data set, with billions of parameters that allow it to produce specific outputs in response to text prompts. This is made possible through the use of self-supervised learning, where the model is fed a broad swath of the internet to learn how to generate predictions.
Early versions of generative AI required submitting data via an API or a complicated process, but pioneers in the field are now developing better user experiences that let you describe a request in plain language. This is a significant improvement, making it easier for users to interact with generative AI models.
The training process for ChatGPT and similar models involves teaching the AI how to label inputs according to labels set by researchers, as a McKinsey article notes. This process is the foundation for more advanced models that can generate predictions based on a few words.
With the right amount of sample text, these models become quite accurate, as seen with the success of ChatGPT.
A different take: Which Term Describes the Process of Using Generative Ai
ChatGPT Models
ChatGPT models are a type of generative AI technology developed by OpenAI, based on the GPT model. They use neural networks to learn context and meaning in sequential data by tracking relationships between words.
These models are trained on a broad set of data, including academic articles, patents, and social media posts, to predict the next word in a sentence and provide responses. They can even interpret images, as seen in the example of GPT-4 responding to a photo of a boxing glove and a seesaw.
ChatGPT Plus, a premium version of the tool, uses a significantly larger knowledge base and can generate digital twins in product design and simulation.
Intriguing read: Introduction to Generative Ai with Gpt
Models
Generative AI models combine various AI algorithms to represent and process content.
These models can transform raw characters into sentences, parts of speech, entities, and actions, which are represented as vectors using multiple encoding techniques.
Techniques like GANs and variational autoencoders (VAEs) are suitable for generating realistic human faces and synthetic data for AI training.
Recent progress in transformers has led to neural networks that can not only encode language, images, and proteins but also generate new content.
Developers can use these techniques to create custom generative AI models that can perform specific tasks, such as generating headlines based on data in slides.
With the right training data, generative AI models can also encode the biases, racism, deception, and puffery contained in the data, which is a caution for developers.
Custom GPTs, like the ones offered by OpenAI, allow users to create their own GPTs without coding and can be used for internal purposes or shared with others.
These GPTs can be accessed through a browser-based dashboard and can be used for various tasks, such as searching the web, making images, or analyzing data.
Suggestion: Getty Images Nvidia Generative Ai Istock
What Is GPT-3.5?
GPT-3.5 is a technology developed by Google researchers, specifically a generative pretrained transformer model that learns context and meaning in sequential data by tracking the relationships between words.
These models are trained on a broad set of data, including academic articles, federal and state legal opinions, patents, websites, social media posts, general facts from sources like Wikipedia, books, open-source code, and other resources.
GPT-3.5 uses this training data to predict what the next word in a sentence is most likely to be, which is then used to provide responses.
ChatGPT reflects OpenAI's attempts to continually improve the GPT model, with the premium version, ChatGPT Plus, recently upgraded to support GPT-4.
Available GPTs
OpenAI's GPT store offers millions of GPTs, but you'll likely only be interested in a few. A range of GPT custom apps are available, including AllTrails personal trail recommendations, a Khan Academy programming tutor, and a Canva design tool.
Some GPTs can even generate digital twins in design and simulation, or create images from text prompts. For example, there's a coloring book picture creator and a logo generator that use OpenAI's Dall-E tool.
Check this out: Modern Generative Ai with Chatgpt and Openai Models Pdf
You can also find GPTs that offer personalized services, such as a book recommender, a fitness trainer, and a haiku writer. Even a Pearl for Pets for vet advice bot is available.
If you're looking to build your own custom GPT, OpenAI allows you to do so without coding, using their browser-based dashboard. You can customize ChatGPT to fit your specific needs, but it will only be accessible to users with a ChatGPT Plus subscription.
OpenAI's Rag Assistants can also be used to build custom assistants with their own applications, but this requires developer-level coding.
Here's an interesting read: Develop Generative Ai Solutions with Azure Openai Service
What Are the Benefits of?
Generative AI can be a game-changer for businesses, making it easier to interpret and understand existing content, and automatically creating new content.
Automating manual processes is one of the key benefits of generative AI. It can help with tasks like writing content, responding to emails, and summarizing complex information into a coherent narrative.
Broaden your view: Generative Ai for Content Creation
Developers are exploring ways to improve existing workflows with generative AI, and some potential benefits include:
- Automating the manual process of writing content
- Reducing the effort of responding to emails
- Improving the response to specific technical queries
- Creating realistic representations of people
- Summarizing complex information into a coherent narrative
- Simplifying the process of creating content in a particular style
By using generative AI, programmers can frame up bigger projects or fill in details, making it easier to write software and develop applications.
Use Cases and Industries
Generative AI can be applied in various use cases to generate virtually any kind of content, making it a versatile tool for users of all kinds.
Some of the use cases for generative AI include implementing chatbots for customer service and technical support, deploying deepfakes for mimicking people or specific individuals, and improving dubbing for movies and educational content in different languages.
Generative AI can also be used to write email responses, dating profiles, resumes, and term papers, create photorealistic art in a particular style, and optimize new chip designs.
Here are some notable use cases across industries:
- Finance: building better fraud detection systems by watching transactions in the context of an individual's history
- Legal: designing and interpreting contracts, analyzing evidence, and suggesting arguments
- Manufacturing: identifying defective parts and the root causes more accurately and economically
- Film and media: producing content more economically and translating it into other languages with the actors' own voices
- Medical: identifying promising drug candidates more efficiently
- Architectural firms: designing and adapting prototypes more quickly
- Gaming companies: designing game content and levels
Generative AI tools like GPT-3.5 and GPT-4 have a wide range of applications across functions, including marketing and sales, operations, IT/engineering, and risk and legal.
Use Cases by Industry
Generative AI has the potential to profoundly impact various industries, and it's essential to understand how it can be applied in each sector. Finance, for example, can use generative AI to build better fraud detection systems by analyzing an individual's transaction history.
In the legal field, generative AI can be used to design and interpret contracts, analyze evidence, and suggest arguments. This can help legal firms streamline their processes and improve the quality of their work.
Manufacturers can use generative AI to identify defective parts and their root causes more accurately and economically. This can be achieved by combining data from cameras, X-ray, and other metrics.
Film and media companies can use generative AI to produce content more economically and translate it into other languages with the actors' own voices. This can help them reach a wider audience and reduce production costs.
The medical industry can use generative AI to identify promising drug candidates more efficiently. This can be done by analyzing vast amounts of data and identifying patterns that may indicate a potential new treatment.
For more insights, see: Telltale Words Identify Generative Ai Text
Architectural firms can use generative AI to design and adapt prototypes more quickly. This can help them respond to changing client needs and reduce the time it takes to bring a project to completion.
Gaming companies can use generative AI to design game content and levels. This can help them create more engaging and dynamic games that keep players interested.
Here are some specific examples of how generative AI is being used in different industries:
Cheating in School
ChatGPT can be a double-edged sword in the classroom, as it can be used to cheat on assignments and exams.
Microsoft's partnership with OpenAI has made ChatGPT a powerful tool for students, but educators fear it will replace original thought and work.
Companies that sell anti-plagiarism software now flag text they believe was generated by AI.
Not everyone is opposed to ChatGPT, though - some see it as a tool akin to Google search and Wikipedia articles that can help students.
Using calculators on exams was once considered cheating, but now they're a standard tool in many workplaces.
Dartmouth's dean of engineering, Alexis Abramson, believes students should learn how to use AI tools responsibly.
Abramson encourages her students to disclose when they use ChatGPT to write a first draft or polish their grammar.
Students who use ChatGPT should include what they did when they turn in their assignment, Abramson suggests.
Consider reading: What Is a Best Practice When Using Generative Ai
Predictive vs Conversational
Predictive vs Conversational AI is a crucial distinction to understand in the world of artificial intelligence. Predictive AI uses patterns in historical data to forecast outcomes, classify events and provide actionable insights.
Organizations rely on predictive AI to sharpen decision-making and develop data-driven strategies. It's a game-changer for businesses that want to make informed decisions.
Conversational AI, on the other hand, helps AI systems like virtual assistants and chatbots interact with humans in a natural way. It uses techniques from NLP and machine learning to understand language and provide human-like text or speech responses.
Predictive AI is all about analysis, while conversational AI is about interaction. Understanding this difference can help you choose the right tool for the job.
Recommended read: Conversational Ai vs Generative Ai
ChatGPT Status and Limitations
ChatGPT is not very up to date, which can be a problem. It's trained on data up through April 2023, but it's not like a search engine that crawls news sites constantly for the latest information.
This limitation can be seen in searches that use ChatGPT to process results, where it may not have the latest information on certain topics. For example, it may not know about the release of the ChatGPT Android app, which is still an issue despite the Microsoft-OpenAI partnership that can tweak ChatGPT Plus results.
Traditional search engines can help "ground" AI results, making them more accurate and reliable.
Intriguing read: Generative Ai Not Working Photoshop
ChatGPT's Current Status
ChatGPT is not very up to date, and that can be a problem.
It's trained on data up through April 2023, but it's not like a search engine that can crawl news sites for the latest information.
A Bing search using ChatGPT to process results may not always be accurate, and traditional search engines can help to "ground" AI results.
Here are some limitations of ChatGPT's current status:
This means that ChatGPT may not always have the most up-to-date information, and it's not a substitute for traditional search engines.
Using ChatGPT for Malicious Purposes
OpenAI tries to block uses that involve sexual or violent material, racist caricatures, and personal information like Social Security numbers or addresses.
It acknowledges the difficulties in preventing harmful uses, such as generating potentially harmful content like advice on planning attacks or hate speech.
GPT-4 can represent various societal biases and worldviews that may not be representative of the user's intent, or of widely shared values.
Red teaming, a process where experts try to find unsafe uses of its AI and bypass protections, identified lots of problems and tried to nip them in the bud before GPT-4 launched.
For example, a prompt to generate jokes mocking a Muslim boyfriend in a wheelchair was diverted so its response said it couldn't provide jokes that may offend someone based on their religion, disability, or any other personal factors.
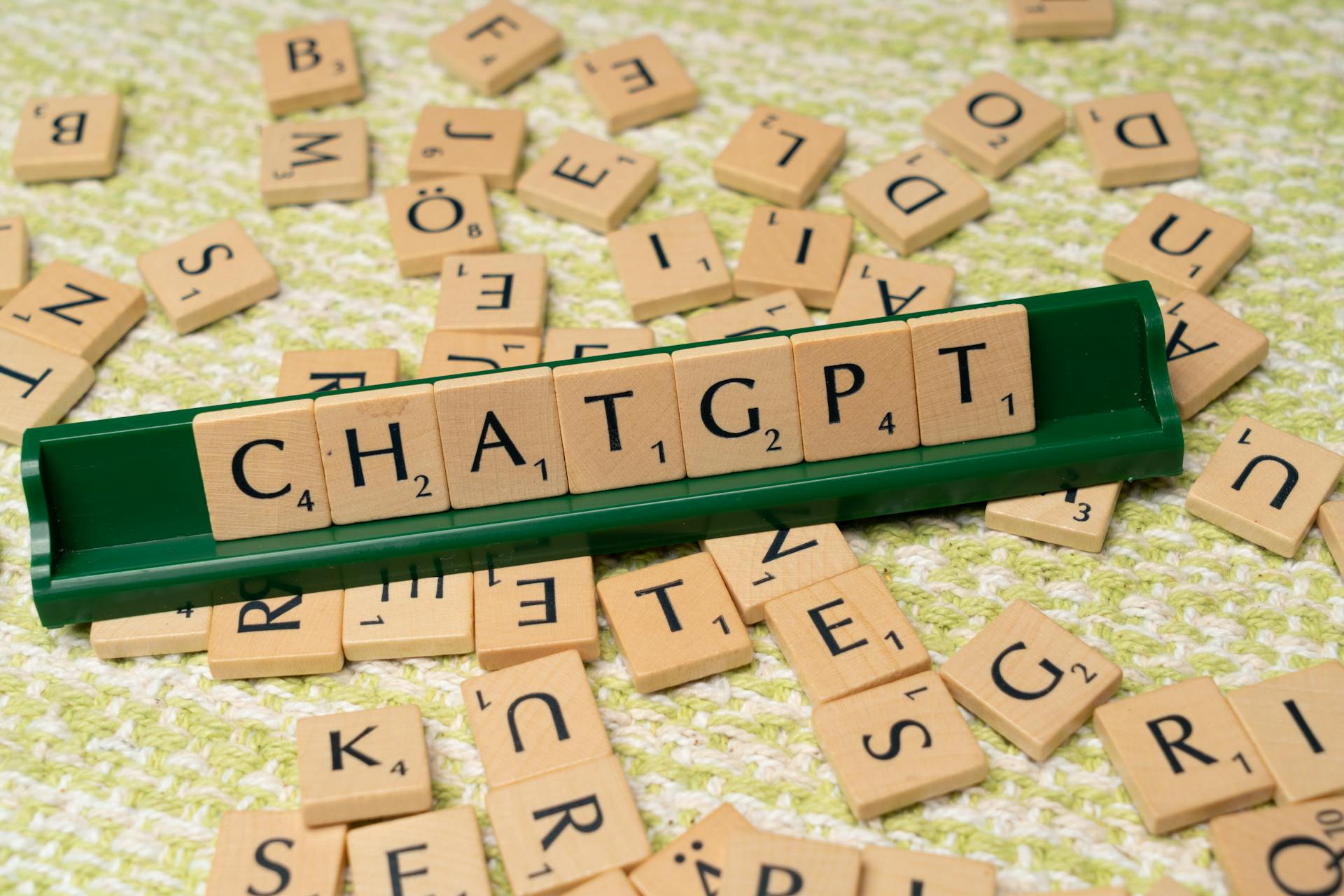
Researchers are still probing LLM limits, and Italian researchers discovered they could use ChatGPT to fabricate fake but convincing medical research data.
Google DeepMind researchers found that telling ChatGPT to repeat the same word forever eventually caused a glitch that made the chatbot blurt out training data verbatim, which is a big no-no.
OpenAI barred the approach, but it shows that LLMs are still new and expect more problems and more patches.
Customization and Comparison
You can create your own custom version of ChatGPT without coding, using OpenAI's browser-based dashboard.
These custom GPTs can be made for personal or company use, but require a ChatGPT Plus subscription to access.
They reside at chat.openai.com, but can't be easily embedded on a website like a widget or live chat.
Custom GPTs can connect to other applications using APIs, but enterprise customers can deploy internal-only GPTs.
Chat logs aren't available to everyday GPT builders, but developers can use APIs to connect GPTs to other applications.
OpenAI's Rag Assistants can be compared to Google's Vertex Search and Conversation, but let's dive deeper into that comparison in the next section.
Worth a look: Amazon Connect Generative Ai
History and Development
The history of generative AI is a story of rapid progress and innovation. Generative AI has its roots in the 1960s with the creation of the Eliza chatbot by Joseph Weizenbaum.
These early implementations used a rules-based approach that was limited by a lack of vocabulary and context. Early chatbots were also difficult to customize and extend.
In the wake of advances in neural networks and deep learning in 2010, the field saw a resurgence. This enabled generative AI to automatically learn to parse existing text, classify image elements, and transcribe audio.
Ian Goodfellow introduced GANs in 2014, providing a novel approach for organizing competing neural networks to generate and rate content variations. This led to the creation of realistic people, voices, music, and text.
Techniques like VAEs, long short-term memory, transformers, diffusion models, and neural radiance fields have expanded generative AI capabilities since then.
Additional reading: Generative Ai Text Analysis
Sources
- https://www.mckinsey.com/featured-insights/mckinsey-explainers/what-is-generative-ai
- https://www.techtarget.com/searchenterpriseai/definition/generative-AI
- https://biztechmagazine.com/article/2023/03/what-generative-ai-and-how-can-tools-chatgpt-help-businesses-perfcon
- https://www.cnet.com/tech/computing/chatgpt-heres-what-you-get-with-the-popular-gen-ai-tool-that-started-it-all/
- https://customgpt.ai/exploring-generative-ai-chatbots-openai/
Featured Images: pexels.com