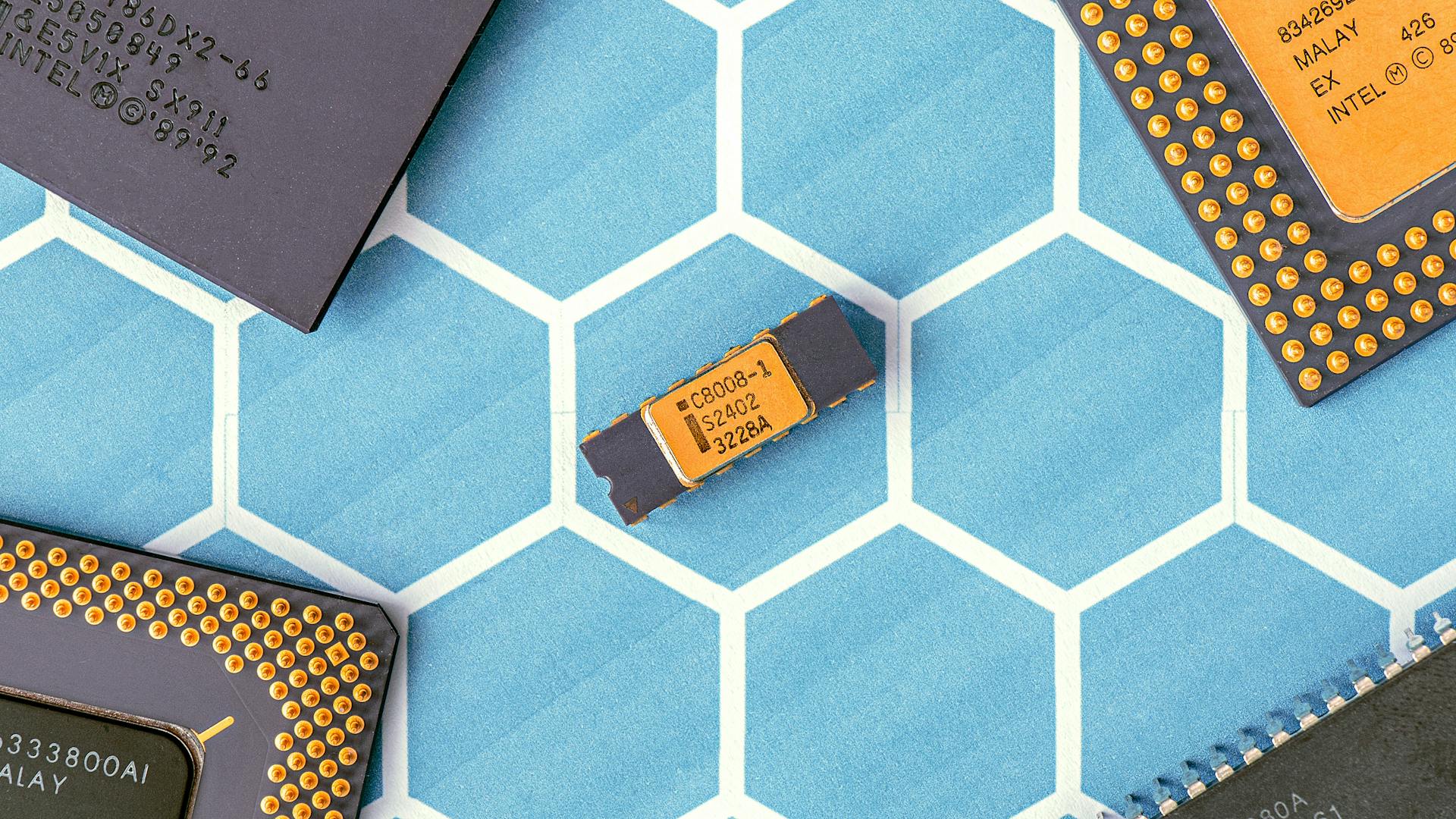
The AI computer chips market is growing rapidly, with a projected Compound Annual Growth Rate (CAGR) of 26.3% from 2023 to 2030.
This growth is driven by increasing demand for AI applications in various industries, including healthcare, finance, and education.
The global AI chip market size is expected to reach $150.9 billion by 2030, up from $12.8 billion in 2020.
Artificial Intelligence (AI) computer chips are designed to handle complex tasks such as machine learning, natural language processing, and computer vision.
Types of AI Computer Chips
NPUs are modern add-ons that enable CPUs to handle AI workloads and are similar to GPUs, except they're designed with the more specific purpose of building deep learning models and neural networks.
NPUs excel at processing massive volumes of data to perform advanced AI tasks like object detection, speech recognition, and video editing. They often outperform GPUs when it comes to AI processes.
NPUs are designed specifically for AI workloads, making them a powerful choice for tasks that require massive data processing.
What Is a Regular Chip?
Regular chips are typically general-purpose and designed to accomplish all kinds of computer functions.
These chips are versatile and can handle a wide range of tasks, from basic calculations to complex operations.
Unlike AI chips, regular chips are not optimized for specific workloads, which can make them less efficient in certain situations.
In fact, regular chips are often used for general computing tasks, such as browsing the internet, checking email, and running office software.
They're like the Swiss Army knife of computer chips - they can do a little bit of everything, but may not excel at any one thing.
GPUs
GPUs are most often used in the training of AI models. Originally developed for applications that require high graphics performance, like running video games or rendering video sequences, these general-purpose chips are typically built to perform parallel processing tasks.
GPUs are connected together to train AI systems synchronously because AI model training is so computationally intensive. This allows them to handle the complex computations involved in AI-related tasks.
Because of their parallel processing capabilities, GPUs are well-suited for tasks that require a lot of data to be processed quickly. This makes them a popular choice for AI applications.
GPUs are often used in conjunction with other chips, like CPUs and NPUs, to create a powerful AI computing system.
MLSoC
MLSoC is a type of AI accelerator chip designed with low power needs and support for fast inferencing.
It has performance ranges between 50-200 TOPS, or theoretical operations per second, at 5-20 Watts.
This is an industry first, with a power efficiency of 10 TOPS per Watt.
The chip combines traditional compute IP from Arm with the company's own machine learning accelerator and dedicated vision accelerator.
It also combines multiple machine learning accelerator mosaics through a proprietary interconnect.
The chipset can theoretically be scaled up to 400 TOPS at 40 Watts.
Originally designed for computer vision applications, it's capable of a range of machine learning workloads like natural language processing.
Broaden your view: Is Computer Vision Ai
Specialized AI Computer Chips
Specialized AI computer chips are designed to accelerate specific tasks, such as image and video processing, making them highly efficient.
FPGAs, or Field-Programmable Gate Arrays, are reprogrammable, allowing them to be "hyper-specialized" for various tasks. They offer superior performance compared to general-purpose processors or other AI chips.
ASICs, or Application-Specific Integrated Circuits, are custom-built for specific applications, such as artificial intelligence. They are optimized for one specific task, resulting in superior performance compared to general-purpose processors.
Some AI chips, like the Colossus MK2 GC200 IPU, are designed to accelerate machine intelligence, with 1,472 powerful processor cores and 900MB In-Processor-Memory. This allows for unprecedented 250 teraFLOPS of AI compute at FP16.16 and FP16.SR.
The WSE-2 chip is the largest ever built, with 2.6 trillion transistors and 40GB of high-performance on-wafer memory. It's optimized for tensor-based, sparse linear operations underpinning inference and neural network training for deep learning.
FPGAs
FPGAs are highly efficient at a variety of different tasks, particularly those related to image and video processing. They can be reprogrammed “on the fly,” making them hyper-specialized.
FPGAs are useful in the application of AI models because they can be reprogrammed to meet the requirements of specific AI models or applications. This flexibility is essential to the advancement of AI.
FPGAs are highly efficient at a variety of different tasks, particularly those related to image and video processing, making them ideal for applications such as data center compute, wireless test equipment, medical image processing, 5G radio and beamforming, and video processing for smart cities.
Unlike general-purpose chips, FPGAs can be customized to meet the requirements of specific AI models or applications, allowing the hardware to adapt to different tasks.
A15 Bionic
The A15 Bionic chip is a System-on-Chip with 15 billion transistors, a significant jump from the 11.8 billion on the A14 in the iPhone 12 models.
It features two high-performance cores, known as Avalanche, and four energy-efficiency cores, known as Blizzard, built on TSMC's 5-nanometer manufacturing process.
The A15 has a 16-core neural engine dedicated to speeding up artificial intelligence tasks, capable of performing 15.8 trillion operations per second, a rise from the A14's 11 trillion operations per second.
Its new image signal processor has improved computational photography abilities.
The system cache boasts 32MB, and the A15 also has a new video encoder, a new video decoder, a new display engine, and wider lossy compression support.
Expand your knowledge: Copilot New Computer Ai
Edge AI Computer Chips
Edge AI computer chips make AI processing possible on virtually any smart device, from watches to kitchen appliances.
This process, known as edge AI, reduces latency by processing data closer to where it originates.
AI chips can be used in a wide range of applications, from smart homes to smart cities.
AI chips improve security and energy efficiency by processing data locally.
By using AI chips, devices can operate independently of the cloud, making them more reliable and efficient.
AI Computer Chip Companies
Nvidia dominates the AI chip manufacturing industry, but it faces competition from other major tech companies like Microsoft, Google, Intel, IBM, and AMD.
Nvidia invented the GPU in 1999, which propelled the growth of the PC gaming market and redefined modern computer graphics, artificial intelligence, and high-performance computing.
The company works on AI and accelerated computing to reshape industries, like manufacturing and healthcare, and help grow others.
Graphcore develops accelerators for machine learning and AI, aiming to make a massively parallel intelligence processing unit, or IPU, holding the complete machine learning model inside the processor.
Suggestion: Ai Self Learning
SambaNova Systems is creating the next generation of computing to bring AI innovations to organizations across the globe, with its Reconfigurable Dataflow Architecture powering the SambaNova Systems DataScale.
Flex Logix provides industry-leading solutions that enable flexible chips and accelerate neural network inference, claiming that its technology offers cheaper, faster, and smaller FPGAs that use less power.
Which Companies Make?
Several companies make AI chips, including Nvidia, which dominates the industry, and other major tech companies like Microsoft, Google, Intel, IBM, and AMD.
Nvidia is a leading manufacturer of AI chips, and its revenue has been steadily increasing over the years. According to the company's financial reports, Nvidia's revenue has grown from $5.7 billion in 2015 to an estimated $26.9 billion in 2024.
Here are some key statistics about Nvidia's revenue:
Nvidia's revenue has also been broken down by region, with the company generating significant revenue from North America, Asia, and Europe.
Nvidia's AI chips are used in a variety of applications, including gaming, professional visualization, and datacenter markets. The company's revenue from these segments has been steadily increasing over the years.
Nvidia's H100 GPUs have been widely adopted by datacenter customers, with estimated shipments of over 100,000 units in 2023.
Cloud 100
The Cloud 100 is a chip designed for AI inference acceleration, addressing specific requirements in the cloud such as process node advancements, power efficiency, signal processing, and scale.
It's built with the 7nm process node and has 16 Qualcomm AI cores, which achieve up to 400 TOPs of INT8 inference MAC throughput.
The memory subsystem has four 64-bit LPDDR4X memory controllers that run at 2100MHz, with each controller running four 16-bit channels, amounting to a total system bandwidth of 134GB/s.
Qualcomm uses a massive 144MB of on-chip SRAM cache to keep as much memory traffic as possible on-chip, making it suitable for larger kernels.
The chip’s architecture supports INT8, INT16, FP16, and FP32 precisions, giving it flexibility in terms of models that are supported.
It also includes a set of SDKs to support a set of industry-standard frameworks, exchange formats, and runtimes.
For your interest: Amazon Chip Ai Training
Gr Labs
Gr Labs is a company that's pushing the boundaries of artificial intelligence. Their goal is to create AI that feels alive and behaves like humans.
Their brain-inspired chips can help machines make decisions in real-time, which is a game-changer for efficiency and productivity. This technology has the potential to save companies a significant amount of money in the long run.
AI Computer Chip Technology
AI chips have finite computational resources, which means they can only handle so much processing power before they max out. This is a big problem because developers are creating bigger and more powerful models, driving up computational demands.
The industry is racing to keep up with the breakneck pace of AI, with AI chip designers like Nvidia and AMD incorporating AI algorithms to improve hardware performance and the fabrication process.
AI chips work on the logic side, handling data processing needs of AI workloads, which is beyond the capacity of general-purpose chips like CPUs. They feature unique capabilities that accelerate computations required by AI algorithms, including parallel processing, which allows multiple tasks to be performed simultaneously.
The Technology
AI chips are designed to handle the intensive data processing needs of AI workloads, a task that goes beyond the capacity of general-purpose chips like CPUs.
They incorporate a large amount of faster, smaller, and more efficient transistors, allowing them to perform more computations per unit of energy, resulting in faster processing speeds and lower energy consumption.
AI chips feature unique capabilities that accelerate computations required by AI algorithms, including parallel processing, which enables multiple calculations to be performed simultaneously.
This parallel processing is crucial in artificial intelligence, allowing multiple tasks to be performed simultaneously and enabling quicker and more efficient handling of complex computations.
AI chips are particularly effective for AI workloads and training AI models, making them a great choice for applications that require rapid precision, such as medical imaging and autonomous vehicles.
Because AI chips are specifically designed for artificial intelligence, they tend to perform AI-related tasks like image recognition and natural language processing with more accuracy than regular chips.
Their purpose is to perform intricate calculations involved in AI algorithms with precision, reducing the likelihood of errors and making them an obvious choice for high-stakes AI applications.
7nm
The 7nm FinFET process has 1.6X logic density compared to the 1-nm FinFET process.
TSMC's 7nm Fin Field-Effect Transistor, or FinFET N7, delivers 256MB SRAM with double-digit yields.
This process results in ~40% power reduction and ~20% speed improvement.
TSMC set another industry record by launching two separate 7nm FinFET tracks, one optimized for mobile applications and another for high-performance computing applications.
The 7nm FinFET process is a significant advancement in AI computer chip technology, enabling more efficient and powerful processing.
It's exciting to think about the possibilities this technology holds for future AI applications.
Market and Demand
The semiconductor industry is booming, driven in part by the growing demand for AI computer chips. Major cloud service providers are expected to increase their capital spending by 36% in 2024, spurred in large part by investments in AI and accelerated computing.
This growth is expected to continue, with data center demand for current-generation GPUs doubling by 2026, a reasonable assumption given the current trajectory. Suppliers of key components will need to increase their output by 30% or more in some cases to keep up with demand.
The demand for AI computer chips is not limited to GPUs, however. Advanced packaging and memory are also in high demand, with makers of chip-on-wafer-on-substrate (CoWoS) packaging components needing to almost triple production capacity by 2026.
Here are some key statistics on the demand for AI computer chips:
- Data center systems total spending worldwide is expected to reach $64.3 billion by 2024.
- The global generative AI market size is expected to reach $13.9 billion by 2030.
- The semiconductor market revenue worldwide is expected to reach $1.3 trillion by 2025.
The supply chain for AI computer chips is complex, with many different elements coming together to meet demand. However, with the right planning and investment, it's possible to meet the growing demand for these critical components.
Data Center Demand
Data center demand is on the rise, driven by the growing need for AI and accelerated computing. Major cloud service providers are expected to increase their year-over-year capital spending by 36% in 2024.
Spending on data centers and specialized chips that power them shows no signs of slowing down. This is due in part to investments in AI and accelerated computing, which are driving demand for GPUs.
GPU demand is expected to continue growing as Large Language Models (LLMs) expand their capabilities to process multiple data types simultaneously. This includes text, images, and audio.
According to Bain's forecasting model, if data center demand for current-generation GPUs doubles by 2026, suppliers of key components would need to increase their output by 30% or more in some cases. This is due to the intricacies of the multi-level semiconductor supply chain.
To meet this demand, makers of chip-on-wafer-on-substrate (CoWoS) packaging components would need to almost triple their production capacity by 2026. This is just one example of the complex web of supply chain elements that must come together to enable AI growth.
Obtaining many of these crucial elements involves long lead times that may make it impossible to keep up with demand. This is why it's essential to understand the intricacies of the supply chain and the risks involved.
Here's a breakdown of the key components that are in high demand:
- GPU demand is expected to grow due to LLMs expanding capabilities.
- Suppliers of key components would need to increase output by 30% or more by 2026.
- Makers of CoWoS packaging components would need to almost triple their production capacity by 2026.
Supply Chain Bottlenecks
Taiwan Semiconductor Manufacturing Corporation (TSMC) makes roughly 90 percent of the world’s advanced chips, powering everything from Apple’s iPhones to Tesla’s electric vehicles.
The demand for these chips is currently far exceeding the supply, making it difficult for companies to get their hands on them.
TSMC's control over the market has created severe bottlenecks in the global supply chain, hindering its ability to meet escalating demand for AI chips.
If you're an AI developer and you want to buy 10,000 of Nvidia's latest GPUs, it'll probably be months or years before you can get your hands on them.
TSMC's subsidiary, Japan Advanced Semiconductor Manufacturing (JASM), is constructing a factory in Kumamoto that is expected to be at full production by the end of 2024, which should help alleviate some of the supply chain pressure.
Prominent AI makers like Microsoft, Google, and Amazon are designing their own custom AI chips to reduce their reliance on Nvidia, which should help mitigate the supply chain bottleneck.
TSMC is also building two state-of-the-art plants in Arizona, the first of which is set to begin chip production in 2025.
Frequently Asked Questions
What is an AI computer chip?
An AI computer chip is a specially designed processor optimized for artificial intelligence tasks, particularly deep learning and machine learning. These chips accelerate AI operations, making them a crucial component of the AI revolution.
What is the most powerful AI chip?
The NVIDIA Blackwell B200 is considered the world's most powerful AI chip, designed to revolutionize various AI applications. Its groundbreaking capabilities make it a game-changer in the field of artificial intelligence.
Sources
- https://www.statista.com/topics/6153/artificial-intelligence-ai-chips/
- https://builtin.com/articles/ai-chip
- https://www.cnbc.com/2023/12/14/intel-unveils-gaudi3-ai-chip-to-compete-with-nvidia-and-amd.html
- https://www.bain.com/insights/prepare-for-the-coming-ai-chip-shortage-tech-report-2024/
- https://www.aiacceleratorinstitute.com/top-20-chips-choice/
Featured Images: pexels.com