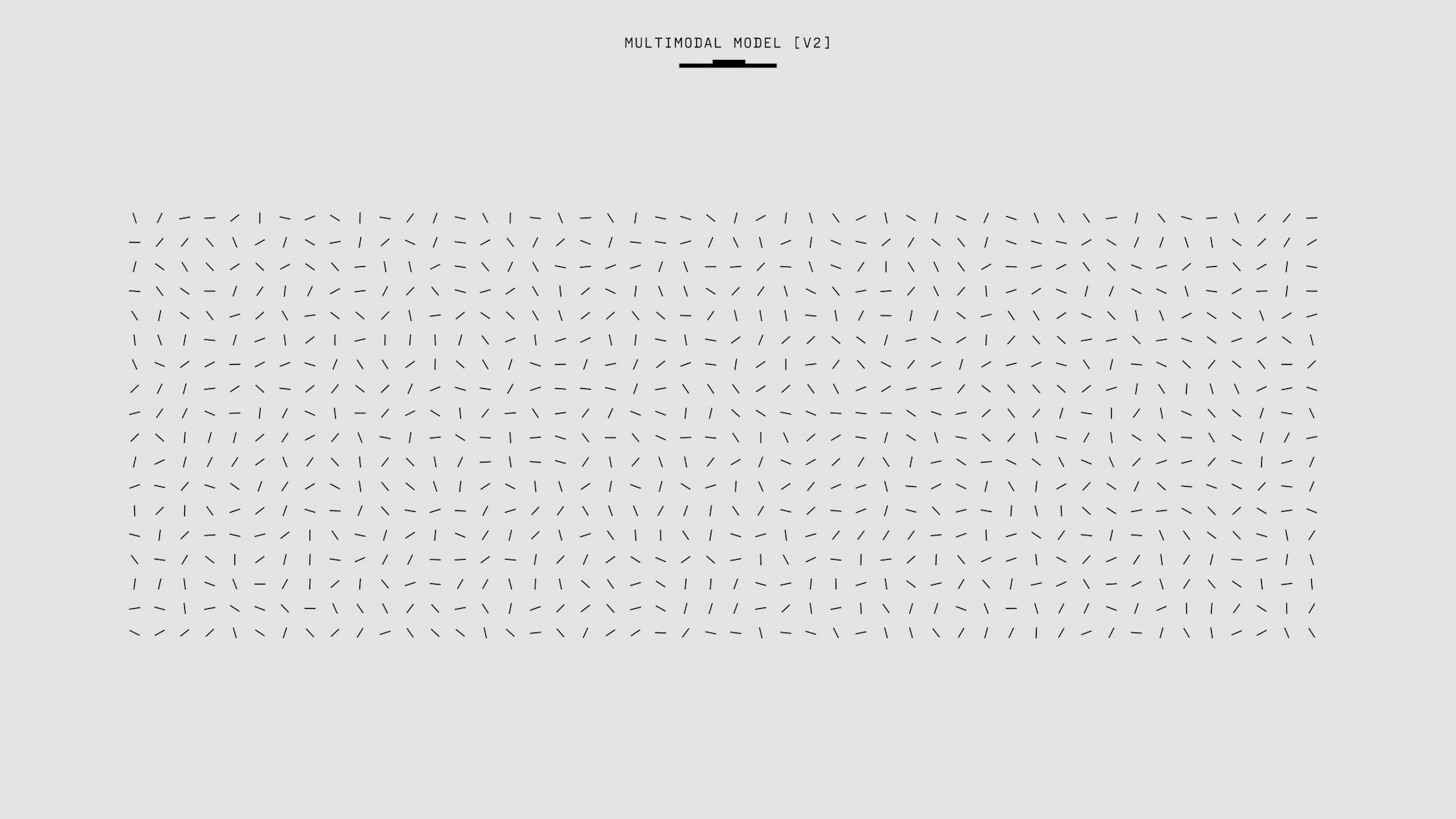
The AWS AI ML Certification is a highly sought-after credential that can significantly boost your career in the field of artificial intelligence and machine learning.
To be eligible for the certification, you need to have a strong foundation in programming languages such as Python, Java, and C++.
The certification exam assesses your skills in areas like data science, machine learning, and deep learning.
It's essential to have hands-on experience with AWS services like SageMaker, Rekognition, and Comprehend to excel in the exam.
Discover more: Ai / Ml Certification
What Is AWS AI ML Certification?
AWS AI ML certification is a series of exams that validate your skills in artificial intelligence and machine learning on Amazon Web Services (AWS).
There are different certification options available, with one geared towards machine learning engineers and another towards data scientists with a strong background in machine learning.
The AWS Certified Machine Learning - Specialty exam is focused more on data science topics and less on AWS Services, requiring a deeper understanding of neural networks and the ability to select appropriate algorithms.
This exam also includes questions where you need to memorize formulas and perform calculations, making it a challenging but rewarding experience for those who prepare well.
Broaden your view: Ai Ml Services
Benefits and Goals
Having an AWS AI ML certification can open doors to new career opportunities and increase your earning potential. You'll gain recognition on a global scale for your knowledge, skills, and expertise, and it's one of the highest-paying data-tech certificates worldwide.
With an AWS certification, you'll be able to stand out from the competition and boost your credibility in the industry. This can lead to better job performance and career advancement, especially in roles such as sales, marketing, and product management.
Here are some benefits of holding an AWS certification:
- Credibility: Having a certification will improve both your credibility and your knowledge of the services.
- Obtaining the title of “AWS Certified” will open up several career options.
- Employers want AWS-certified individuals because they help with business development.
- You can obtain market support, AWS use credits, training subsidies, and other benefits by joining the AWS Partner Network.
Employers are willing to pay more for AI-skilled workers, with a significant increase in salary for professionals in various roles, including sales, marketing, finance, business operations, and IT.
Goals
The goals of pursuing an AWS Machine Learning certification are clear and achievable. You'll demonstrate your ability to create, build, implement, and maintain ML solutions for business problems.
To achieve this, you'll need to select and validate the precise ML technique for the specified business issue, and choose the relevant AWS administrations to put ML solutions into action. This requires a deep understanding of machine learning concepts and AWS services.
See what others are reading: Ai Software for Business
Here are the specific goals you'll need to focus on:
- Demonstrate your ability to create, build, implement, and maintain ML solutions for business problems.
- Select and validate the precise ML technique for the specified business issue.
- Select the relevant AWS administrations to put ML solutions into action.
- Design and implement adaptable, cost-effective, reliable, and secure ML solutions.
These goals are achievable with the right combination of knowledge, skills, and experience. With an AWS Machine Learning certification, you'll be well on your way to advancing your career in machine learning engineering.
You might like: Ai Self Learning
Benefits
Having an AWS Machine Learning certification can significantly boost your career, and here's why:
You'll be recognized globally for your knowledge, skills, and expertise in machine learning, which is a huge advantage in the industry.
According to a November 2023 survey conducted by AWS, employers are willing to pay 43% more for AI-skilled workers in sales and marketing, 42% more for those in finance, 41% more for business operations, and 47% more for IT professionals.
Having a certification like AWS Certified AI Practitioner can lead to better job performance and career advancement, especially for professionals in sales, marketing, and product management.
Employers want AWS-certified individuals because they help with business development, which is one of the prerequisites for higher-tier AWS Partner Network memberships.
Recommended read: Aws Generative Ai Certification
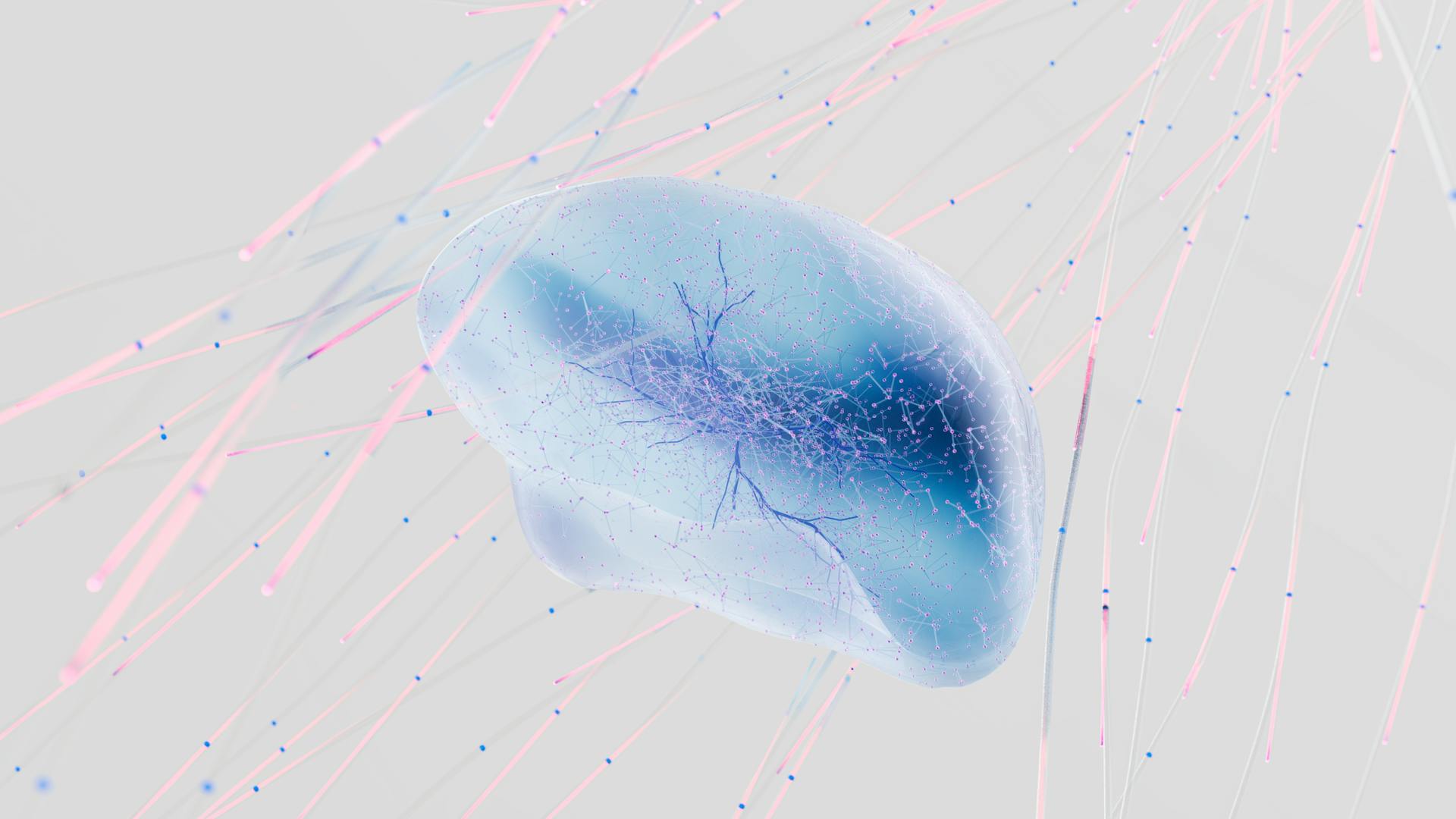
Here are some benefits of having an AWS-approved machine learning certification:
- Confirms your ability to build, train, and deploy the machine learning model utilizing the AWS Cloud.
- Gives you recognition on a global scale for your knowledge, skills, and expertise.
- One of the highest-paying data-Tech certificates worldwide.
- Adds a qualification to your resume, helping you to stand out from the competition.
- Enhances your ability to obtain more opportunities to advance as an AWS engineer.
Compliance and Governance
Compliance and Governance is a crucial aspect of AI development, and it's essential to understand the regulatory standards that apply to AI systems. ISO and SOC are two key standards that organizations must adhere to.
To ensure compliance, AWS provides tools like AWS Config and Amazon Inspector. These tools help organizations monitor and maintain their systems, ensuring they meet the required standards.
Data governance is also a critical aspect of AI development, and it involves managing data throughout its lifecycle. This includes monitoring, retaining, and properly managing data to ensure it's secure and compliant.
Creating policies and scheduling regular reviews are essential steps in following governance protocols. Using frameworks like the Generative AI Security Scoping Matrix can also help organizations stay on track.
Maintaining transparency and training your team effectively are also vital components of governance. By doing so, organizations can ensure that their AI systems are secure, compliant, and trustworthy.
Take a look at this: Ai for Training and Development
Preparation and Prerequisites
To get started with the AWS AI ML certification, you'll need 1-2 years of experience designing, implementing, or executing machine learning/deep learning workloads on the AWS Cloud.
Having the capability to convey the intuition underlying fundamental ML techniques is also a must. This means you should be able to explain the reasoning behind various machine learning concepts in a clear and concise manner.
You'll also need proven proficiency with fundamental hyperparameter optimization, which is the process of adjusting the settings of a machine learning model to achieve the best results. Additionally, being proficient in machine learning and deep learning frameworks is essential for success in this field.
Being able to adhere to excellent practices in model-training and deployment and operating best practices is crucial for a successful certification.
Prerequisites
To get started with AWS ML, you'll need 1-2 years of experience designing, implementing, or executing machine learning/deep learning workloads on the AWS Cloud.
You should have the capability to convey the intuition underlying fundamental ML techniques, which is essential for understanding how to apply them in practice.
Proven proficiency with fundamental hyperparameter optimization is also crucial, as it directly impacts the performance of your models.
To work with machine learning and deep learning frameworks, you'll need to be proficient in them, which will allow you to build and train models efficiently.
Being able to adhere to excellent practices in model-training is vital to ensure your models are accurate and reliable.
To deploy and operate your models effectively, you'll need to follow best practices, which will help you to maintain and improve your models over time.
For more insights, see: Ai Ml Model
Implementation and Operations
To excel in the Implementation and Operations segment of the exam, you need to focus on building machine learning solutions that deliver robust performance. This involves implementing appropriate machine learning services and features.
The exam will test your ability to build ML solutions for robust performance. This means you should be familiar with the various machine learning services and features available.
Recommended read: Ai & Ml Solutions
Applying AWS security practices is also crucial in this segment. This includes ensuring the confidentiality, integrity, and availability of your machine learning data.
To deploy and operationalize ML solutions, you need to consider factors such as scalability, reliability, and monitoring. This will help you ensure that your solutions are running smoothly and efficiently.
Here are some key areas to focus on in the Implementation and Operations segment:
- Build ML Solutions for Robust Performance
- Implement Appropriate ML Services and Features
- Apply AWS Security Practices
- Deploy and Operationalize ML Solutions
Logistics of the Real
You can schedule your exam for the Machine Learning AWS specialty here (MLS-C01) and take it from home due to Covid-19.
The testing process involves taking pictures of your room and showing it with your webcam, so make sure you have a quiet and distraction-free space. You won't be able to have any pen or paper during the exam.
You'll need to close all applications on your computer and run the software full screen, so make sure you save any important work beforehand.
If you need earplugs, you'll need to request them specifically prior to the exam, as I did, and it was a struggle for me to focus without them.
Domain 4: Responsible Guidelines
Domain 4: Responsible Guidelines is a crucial aspect of the AWS Certified AI Practitioner AIF-C01 exam. This domain accounts for 14% of the exam and focuses on guidelines for responsible AI.
The key aspects of responsible AI include fairness, inclusivity, safety, and accuracy. You'll need to understand how to use tools like Guardrails for Amazon Bedrock to identify these features.
In this section, you'll learn about the importance of selecting models with environmental sustainability in mind. This is a critical consideration, as generative AI can have a significant impact on the environment.
Potential legal risks of generative AI are also covered, including intellectual property issues and biased outputs that could damage customer trust. You'll need to understand how to mitigate these risks.
The importance of using inclusive and diverse datasets is emphasized, as bias and variance can lead to inaccuracies. Tools like Amazon SageMaker Clarify and Model Monitor can help detect and monitor bias.
You'll also learn about the differences between transparent, explainable models and those that are not. Tools like Amazon SageMaker Model Cards can help identify which models are clear and understandable.
The tradeoffs between model safety and transparency are discussed, including balancing how interpretable a model is against its performance. You'll need to understand these tradeoffs to develop effective AI solutions.
The principles of human-centered design are also covered, ensuring AI is explainable and user-friendly. By understanding these principles, you can develop AI solutions that meet the needs of users.
Course Details and Topics
The AWS Certified Machine Learning Specialty exam focuses on four key domains: Data Engineering, Exploratory Data Analysis, Modeling, and ML Implementations and Operations. Each domain represents a significant portion of the exam.
The exam domains are divided as follows: Data Engineering (20%), Exploratory Data Analysis (24%), Modeling (36%), and ML Implementations and Operations (20%). This breakdown indicates the relative importance of each domain.
Some of the specific topics covered in the exam include: S3, Kinesis, Glue, Athena, AWS Data Stores, and AWS Data Pipelines in Data Engineering; Data Types and Distribution, Time Series, and Amazon Athena in Exploratory Data Analysis; CNN, RNN, and Amazon Sagemaker in Modeling; and SageMaker Production Variants, Neo, and IoT Greengrass in ML Implementations and Operations.
Here is a breakdown of the key areas covered in the exam:
Senior Generative Readiness Specialist
As a Senior Generative Readiness Specialist, you'll be diving into the world of AI and machine learning, where you'll have the opportunity to work with cutting-edge technologies like Amazon SageMaker and AWS AI Services.
The AWS Machine Learning - Specialty certification is a great starting point, covering topics such as CNN, RNN, and Ensemble methods, which are crucial for building and deploying machine learning models.
To prepare for this role, you'll want to focus on developing your skills in data engineering, exploratory data analysis, and modeling. This includes understanding data types and distribution, time series, and dealing with missing data.
Some key areas to focus on include:
- Data Engineering: S3, Kinesis, Glue, Athena, and AWS Data Stores
- Exploratory Data Analysis: Data Types and Distribution, Time Series, and Visualizing Data for Machine Learning
- Modeling: CNN, RNN, Tuning neural networks, and Ensemble methods
By mastering these skills, you'll be well-prepared to take on the challenges of a Senior Generative Readiness Specialist role and contribute to the development of innovative AI and machine learning solutions.
Topics
The AWS Certified Machine Learning Specialty exam focuses on four key domains: Data Engineering, Exploratory Data Analysis, Modeling, and ML Implementations and Operations. Each domain represents a significant portion of the exam, with Data Engineering accounting for 20%, Exploratory Data Analysis making up 24%, Modeling taking up 36%, and ML Implementations and Operations covering 20%.
Data Engineering is a critical aspect of machine learning, and the exam covers a range of topics, including S3, Kinesis, Glue, Athena, and AWS Data Stores. You'll also need to be familiar with AWS Data Pipelines, AWS Batch, AWS DMS, and AWS Step Functions.
Exploratory Data Analysis is another essential domain, and the exam covers data types and distribution, time series, Amazon Athena, Quicksight, Ground Truth, EMR, Spark, and data binning. You'll also need to understand how to deal with missing data, imbalanced data, and outliers.
Modeling is the largest domain, making up 36% of the exam, and covers a wide range of topics, including CNN, RNN, tuning neural networks, regularization, gradient descent method, L1 and L2 regularization, and ensemble methods. You'll also need to be familiar with Amazon Sagemaker, Amazon Algorithms, and Amazon AI Services.
ML Implementations and Operations is the final domain, and covers topics such as SageMaker Production Variants, Neo, IoT Greengrass, encryption at rest and in transit, VPC, IAM, logging, monitoring, instance types, and spot instances.
Here's a breakdown of the exam domains and their corresponding percentages:
Remember, each domain is critical to your success on the exam, and you'll need to be well-prepared in all areas to pass.
Frequently Asked Questions
Is AWS ML hard?
The AWS Machine Learning certification is designed for beginners, covering foundational AI concepts and AWS services in a straightforward and accessible way. It's a great starting point for those new to AI and machine learning.
Sources
- https://www.linkedin.com/pulse/exploring-aws-machine-learning-certifications-andy-kroll-btvac
- https://digitalcloud.training/new-aws-certified-ai-practitioner-and-machine-learning-engineer-associate-certifications/
- https://k21academy.com/ai-ml/aws/aws-certified-machine-learning-specialty/
- https://tutorialsdojo.com/aws-certified-ai-practitioner-aif-c01-exam-guide/
- https://www.capitalone.com/tech/machine-learning/advice-for-taking-the-aws-machine-learning-specialty-exam/
Featured Images: pexels.com