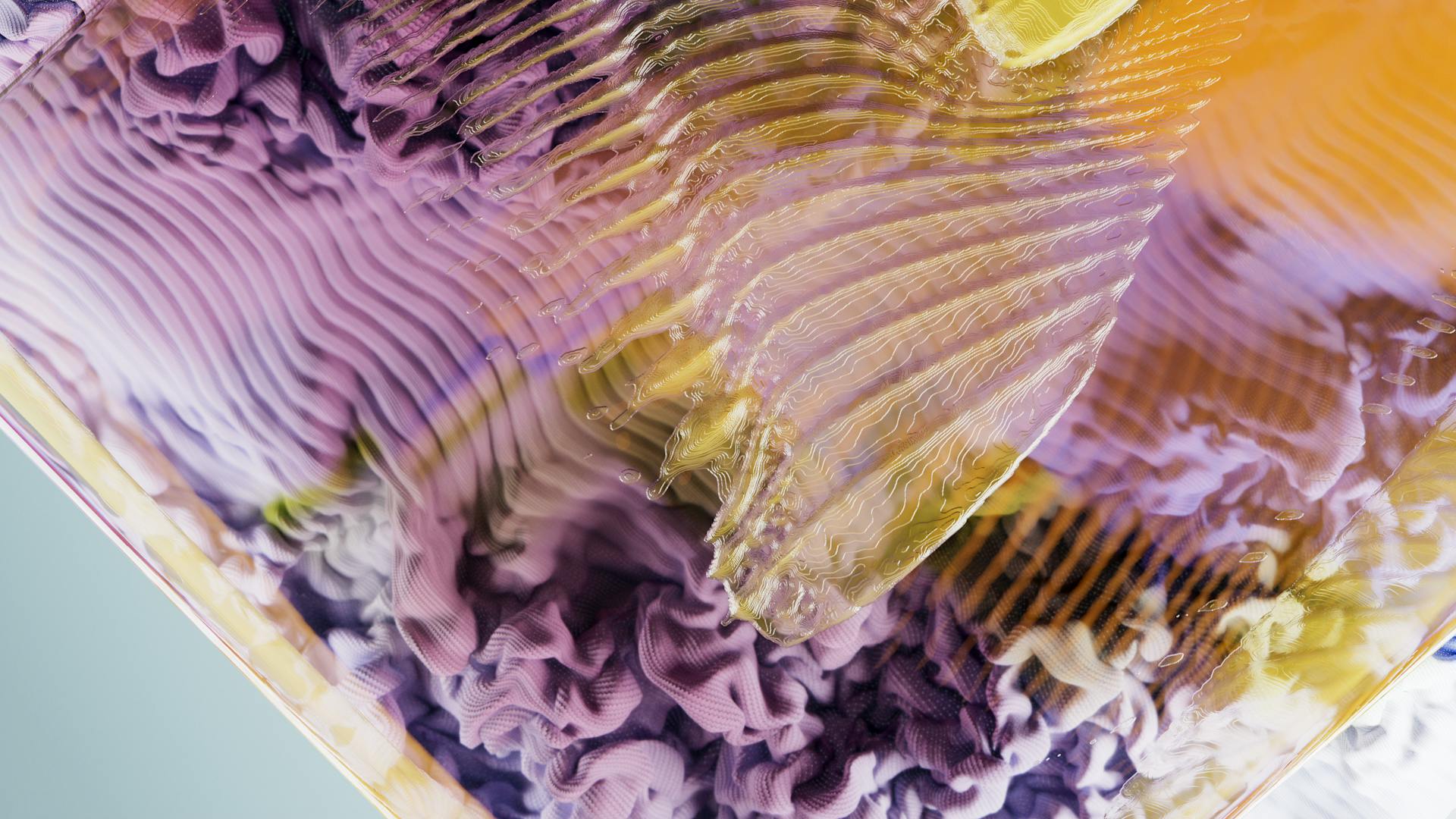
Data labeling companies play a crucial role in the development of artificial intelligence and machine learning models, providing high-quality labeled data that enables these systems to learn and improve.
Many data labeling companies offer a range of tools and services to support their clients, including data annotation, enrichment, and validation.
Some top providers in the industry include Hive, Scale AI, and Clickworker, which offer a variety of data labeling services and tools to meet the needs of different clients.
These companies have developed specialized software and platforms to streamline the data labeling process, making it faster and more efficient.
Data Labeling Companies
Data labeling companies are crucial for training machine learning models, as they provide the necessary labeled data for AI to learn from. Many companies outsource data labeling to these service providers due to the time-consuming nature of the task.
ScaleAI, a $7.3 billion startup, offers a comprehensive suite of solutions for efficient AI model development, including data labeling and annotation services. Their platform, Scale Rapid, facilitates quick project setup and generates high-quality labels promptly.
Dataloop AI specializes in creating data infrastructure and operating systems for AI companies, with its main product being a data management and annotation platform. This platform consists of data management, an intuitive annotation tool with automatic capabilities, and tools for data quality assurance and debugging.
Sama AI provides data labeling services for computer vision, leveraging machine learning to enhance AI development. They cater to sectors like retail, agriculture, and manufacturing, and offer customized workflow solutions tailored to specific project needs.
iMerit is a tech service company that provides precise annotation and labeling services for various data forms, including text, images, and audio. They have a socially conscious business model, hiring from underprivileged communities to contribute to their economic advancement.
Labelbox is a collaborative platform for creating and managing labeled data for machine learning applications. It offers a suite of features to assist with the development of machine learning applications, including auto-computed metrics for easier debugging of models.
SuperAnnotate assists computer vision teams in annotating and managing image data for ML, offering tools for annotating images and videos. Their technology features AI-assisted annotation, facilitating faster and more accurate labeling.
Data labeling is a subset of data preprocessing, providing specific information about data inputs to give ML models a deeper understanding of those inputs. Annotating different objects with more context and detail enhances the system's understanding of its surroundings.
What is Data Labeling?
Data labeling is the process of applying human labels to data in order to help algorithms understand and classify it. This is a crucial step in supervised learning, which requires less data and can be more accurate, but does require labeling to be applied.
The dataset, along with its associated labels, is referred to as ground truth. Analysts estimate humankind sits atop 44 zettabytes of information today, and algorithms have advanced at a phenomenal rate, with their appetite for training data keeping pace.
Supervised learning requires a human to manually apply labels to the data, which can be a time-consuming and labor-intensive process. However, it's a necessary step in helping algorithms learn and improve.
A dataset can be classified under at least 4 overarching formats - text, audio, images, and video. While there are interesting applications for all types of data, we will further hone in on text data to discuss a field called Natural Language Processing (NLP).
Types of Data Labeling
Data labeling is a crucial step in preparing data for machine learning models, and it comes in three main types. These types are defined by the medium of the data being labeled.
Image and video labeling is used in applications like computer vision, where tags are added to individual images or video frames. This type of image classification is used in healthcare diagnostics, object recognition, and automated cars.
Text labeling is used in natural language processing (NLP), where tags are added to words for interpretation of human languages. NLP is used in chatbots and sentiment analysis.
Audio labeling is used in speech recognition, where audio segments are broken down and labeled. Audio labeling is useful for voice assistants and speech-to-text transcriptions.
Here are the three main types of data labeling:
Benefits and Challenges
Data labeling companies can bring numerous benefits to businesses. Accurate predictions are one of the key advantages, as properly labeled data enables machine learning models to make accurate predictions when presented with new data.
By reducing the number of input variables, developers can optimize models to produce better analysis and predictions. This is achieved by labeling input data in a way that specifies the most relevant features and data variables.
Data labeling companies can also enhance innovation and profitability. Once a data labeling approach is in place, workers can focus on finding new uses for labeled data, reducing the time spent on tedious data labeling tasks.
Here are some key benefits of data labeling:
- Accurate predictions
- Data usability
- Enhanced innovation and profitability
Benefits of
Accurate predictions are just one of the many benefits of data labeling. If data scientists input properly labeled data, a trained machine learning model can use that data as a ground truth to make accurate predictions when presented with new data.
Data labeling allows developers to optimize models in ways that produce better analysis and predictions. By labeling input data, developers can specify the features and data variables that are most relevant or important for the model to learn.
Manual data labeling can be time-consuming and expensive, but it's often necessary for important applications. Over 80% of the time spent on AI projects goes into preparing, cleaning, and labeling data, making it a crucial step in the process.
Data labeling enables experts to focus on finding new practical or revenue-generating uses for labeled data. Once a data labeling approach is in place, workers can spend less time on tedious data labeling tasks and more time on innovation and profitability.
Here are some benefits of data labeling:
- Accurate predictions
- Data usability
- Enhanced innovation and profitability
Challenges of
Data labeling can be a costly endeavor, especially when done manually, which can be a significant expense for businesses.
Manual data labeling is a time-consuming process that diverts employees with the needed expertise from their normal duties, taking away from their productivity.
Human error is a common issue in data labeling, leading to inaccurate data processing, modeling, or even ML bias due to coding or manual entry errors.
These errors can have serious consequences, including wasted resources and compromised model performance.
Best Practices and Methods
Data labeling is a crucial step in machine learning, and having the right approach can make all the difference. To ensure high-quality data labels, collect diverse data to prevent bias. This means gathering data sets that are as varied as possible.
A good data labeling team should have domain knowledge of the industry they're serving. Human labelers with outside context guiding them are more accurate than those without, and they bring diverse perspectives to the table. They must also be flexible and nimble, as data labeling and machine learning are iterative processes that evolve over time.
To choose the right data labeling method, consider the size of your data set, the skill level of your employees, and any financial restraints you may have. Some common methods include crowdsourcing, outsourcing, and using in-house staff. A good data labeling team should ideally have 2+ labelers label the same data to ensure accuracy and reduce subjective biases.
Here are some common methods used by data science teams:
To set up a comprehensive data labeling project, it's essential to have clear guidelines and expectations. This includes defining edge cases, such as how to handle sarcasm or jokes, to avoid surprises when the labeled work is complete. Iteration is also key, starting with a small subset of data and reviewing it carefully before scaling up.
Manual vs Automated
Manual data labeling is a time-consuming process that requires immense skills and precision. It's often the first step in the data labeling process, with data scientists and students labeling data themselves to stay close to the ground.
Manual labeling has its advantages, such as full control over data quality and the ability to refine taxonomies as needed. However, it's monumentally time-consuming and subject to human error, making it less efficient than automated labeling.
Automated data labeling, on the other hand, uses AI to label raw data, with human labelers verifying and correcting errors. This approach can save time and reduce human error, but it requires careful training and re-training of the AI model.
To determine the best approach, consider the size and complexity of your data set, as well as the skill level of your employees. If you have a large data set or limited resources, automated labeling may be the better choice. However, if you need fine-grained control over your data, manual labeling may be the way to go.
Here are some key differences between manual and automated data labeling:
Ultimately, the choice between manual and automated data labeling depends on your specific needs and resources. By considering the pros and cons of each approach, you can make an informed decision and choose the best method for your data labeling project.
Methods
Data labeling is a crucial step in machine learning, and the right methods can make all the difference. Iteration is key, as seen in Example 2, where starting with a small subset of data and reviewing it carefully can save time and money in the long run.
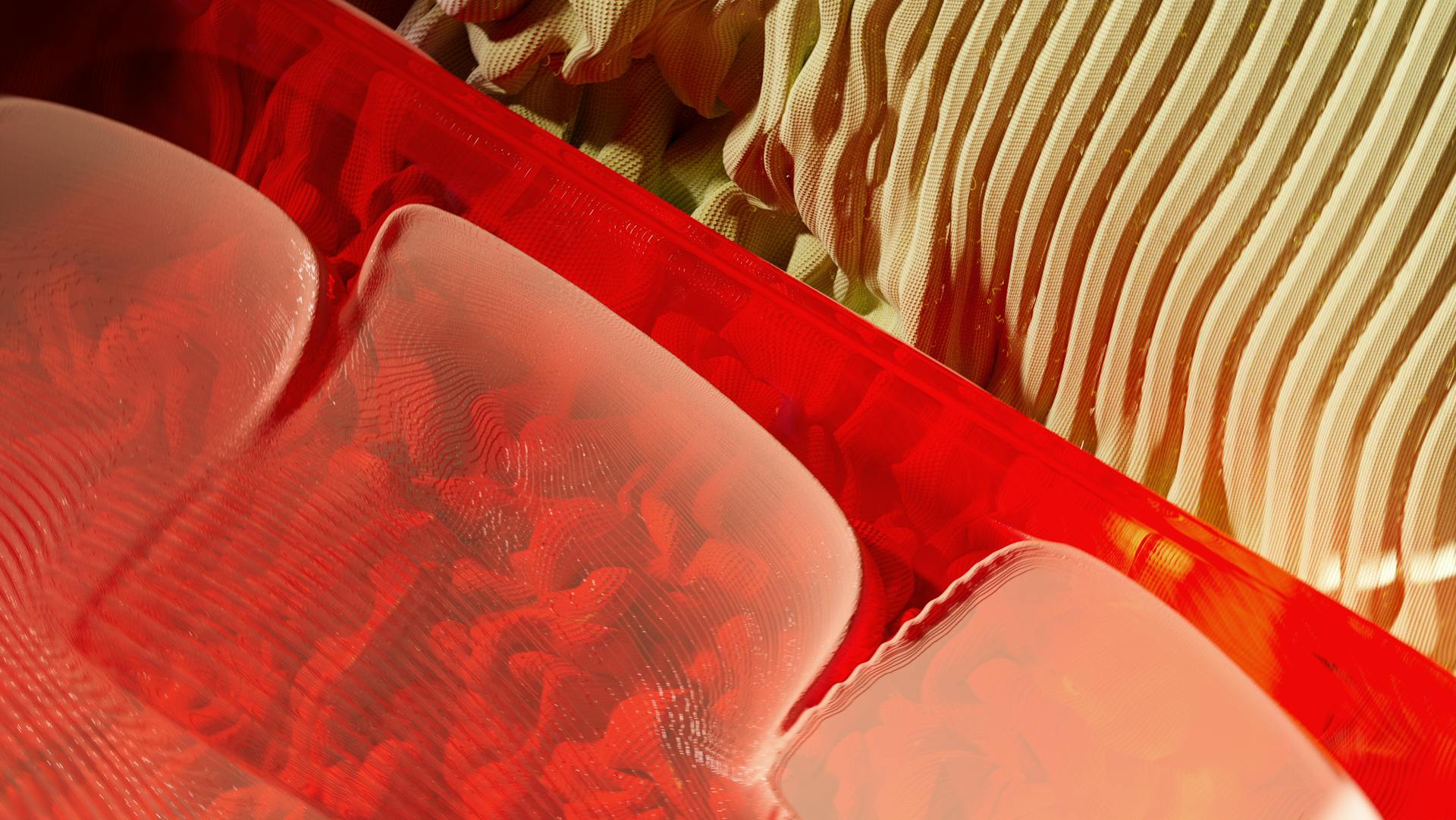
You should also consider labeling redundancy, as humans can make mistakes, especially after a long day. Having 2+ labelers label the same data can help catch errors and ensure accuracy.
A good data labeling team should have domain knowledge of the industry they're working with. This is because human labelers with outside context are more accurate and have diverse perspectives, as mentioned in Example 3.
There are several methods to structure and label data, including crowdsourcing, outsourcing, and in-house staff. You can also use synthetic labeling, which generates new project data using existing data sets, or programmatic labeling, which automates the data labeling process using scripts.
Here are some factors to consider when choosing a high-quality data labeling method:
- Is the business a large enterprise or a small to medium-sized organization?
- What's the size of the data set that requires labeling?
- What's the skill level of employees on staff?
- Are there financial restraints?
- What's the purpose of the ML model being supplemented with labeled data?
Ultimately, the best method will depend on your specific needs and circumstances, so be sure to consider these factors when making your decision.
Tools and Services
Data labeling companies often rely on labeling tools to get the job done.
The most common starting point is an Excel or Google spreadsheet, which is serviceable and requires a relatively low learning curve. However, this approach is not scalable and can be error-prone.
Some companies turn to open-source tools like brat and WebAnno, which are built with labeling in mind and offer customizations. These tools can handle more advanced NLP tasks, but come with a higher learning curve and limited direct customer support.
Crowd-Sourced Services
Crowd-sourced services offer a way to outsource simple tasks to a distributed "crowd" of humans around the world.
Amazon Mechanical Turk was established in 2005 as one of the pioneers in this space. They allow companies to post tasks, known as HITs, that require human intelligence to complete.
These services can be a good option for companies that need large amounts of data labeled quickly, as companies like Appen, Scale, and Samasource can frequently finish labeling data faster than other options.
However, using crowd-sourced services can come with higher costs, as companies like Appen and Scale charge a sizable margin on their data labeling services.
Some companies, like CloudFactory and Data Pure, employ full-time labelers who are fully trained, which can improve data quality but also increases costs.
Fully crowd-sourced solutions can also suffer from labelers who game the system and create fake accounts, which can lead to data leaks.
Tools
Using a spreadsheet like Excel or Google to label data is a common starting point, but it can be error-prone and not scalable for advanced interfaces and workforce management solutions.
The spreadsheet interface was not created for labeling tasks, making it difficult to read and work with text documents.
Open-source tools like brat and WebAnno are popular choices for labeling data, offering a wide array of customizations and being free to set up and host.
However, these tools have a higher learning curve and limited direct customer support.
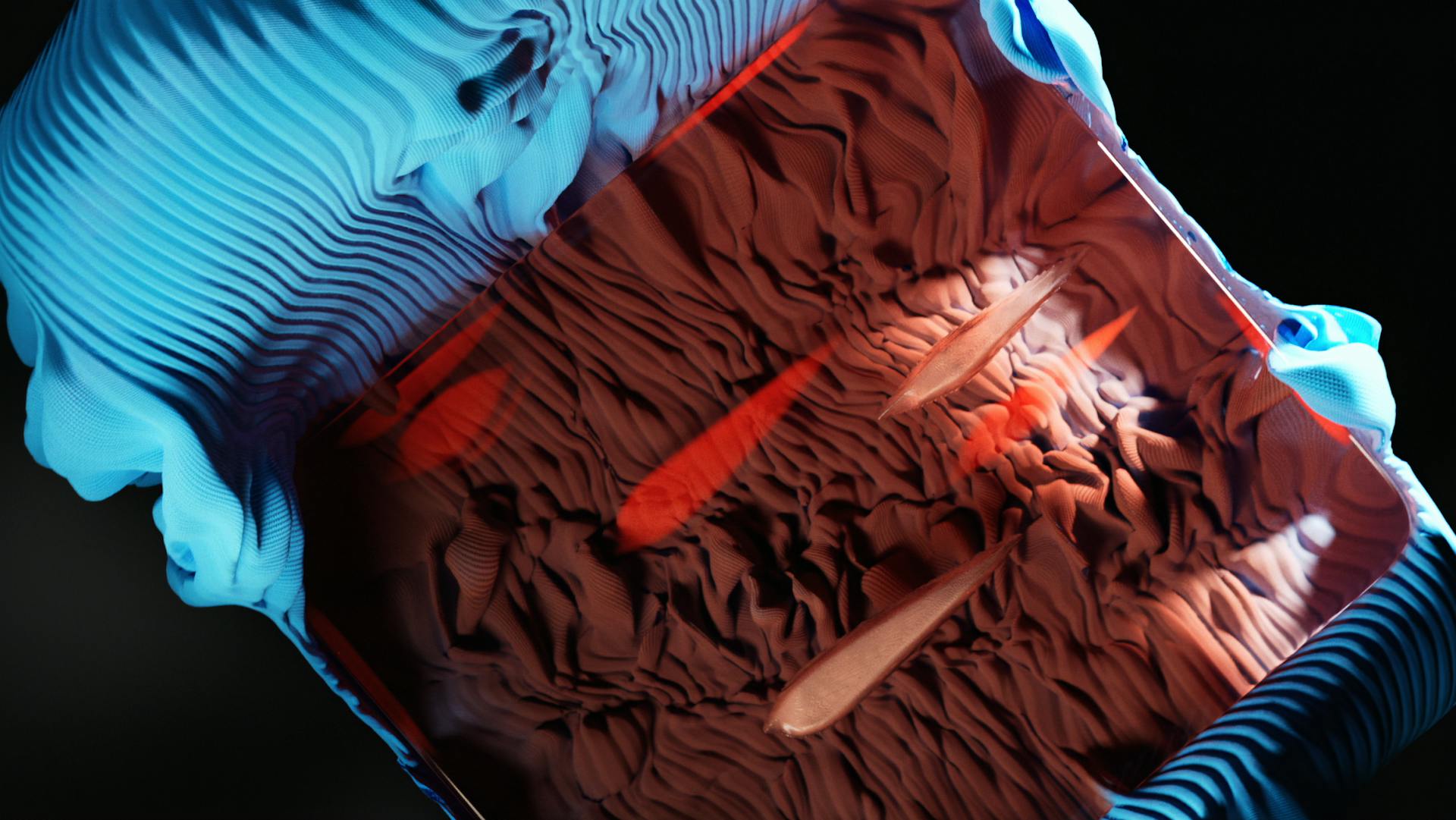
Some companies choose to build their own labeling tools in-house, which can provide full integration with their own stack, but requires a significant investment of engineering time for setup and ongoing support.
Choosing the right tool for the job can make a significant difference in the final output, so it's essential to consider the intuitiveness of the interface, the types of labeling jobs it specializes in, and the level of support offered.
The right tool should also be able to organize and prioritize labeling projects from a single interface, and fit within your budget allocation.
Categories and Companies
Data labeling companies can be categorized into various types, including Back office Outsourcing and Business Process Outsourcing. These categories are essential in understanding the scope of services provided by data labeling companies.
Data labeling is a crucial process in various industries, including gaming, where Game Management Outsourcing plays a significant role. This process involves labeling data to prepare it for use in machine learning models.
Some of the key categories of data labeling companies include:
- Data Entry outsourcing
- Data Labeling Outsourcing
- Content Moderation Services
- Customer Support Services
Categories
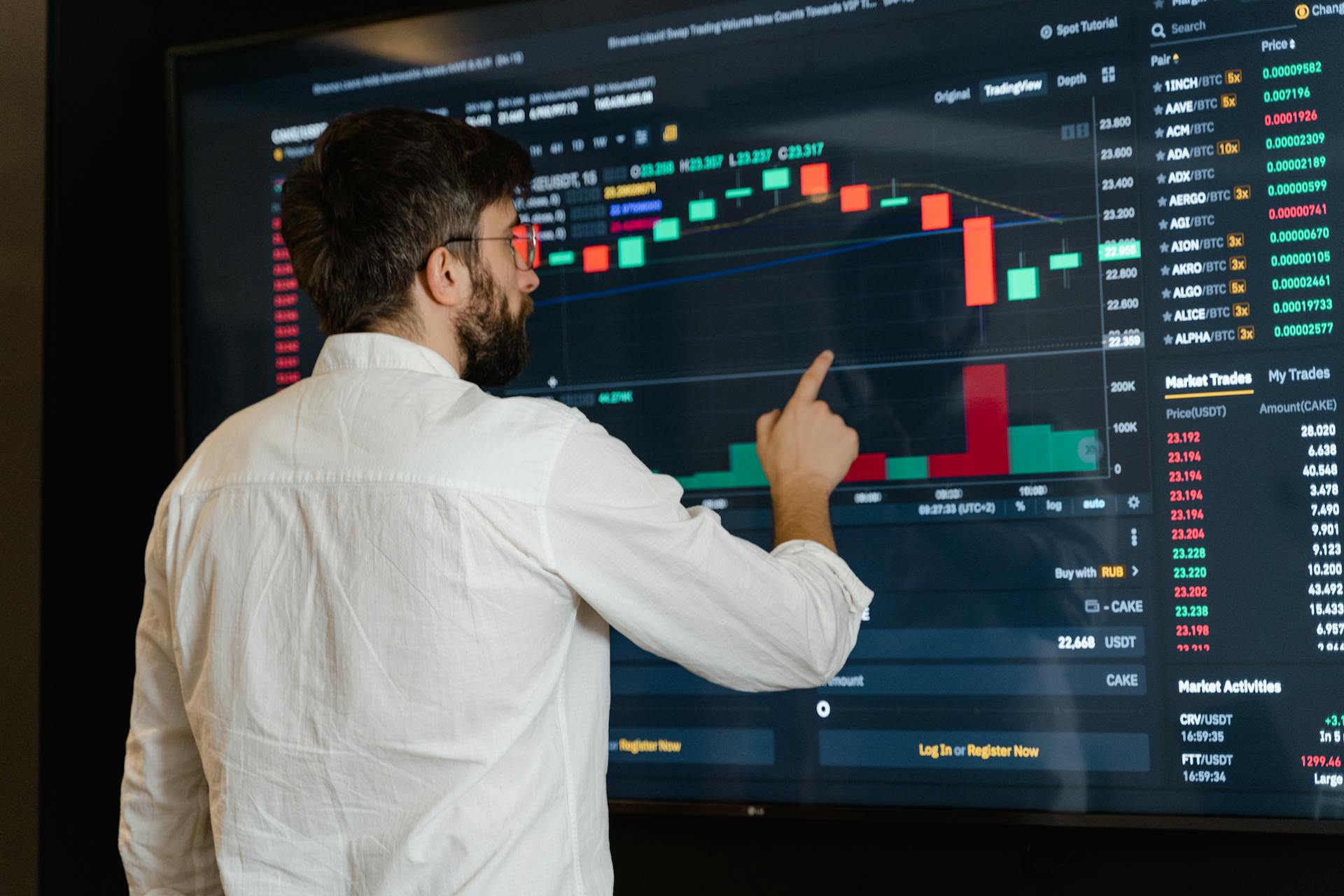
Let's talk about categories and how they relate to companies. Companies often outsource various tasks to other organizations, and these tasks can be categorized into different types.
Back office outsourcing is a common practice, where companies hire another organization to handle tasks such as data entry and customer support.
Some companies specialize in providing content moderation services, which involves reviewing and managing online content to ensure it meets certain standards.
Data labeling is another important task that companies often outsource, as it requires human judgment to label and categorize data accurately.
Here are some examples of categories and their corresponding tasks:
- Back office Outsourcing: data entry, customer support
- Business Process Outsourcing: various business tasks
- Content Moderation Services: reviewing and managing online content
- Data Entry outsourcing: data entry tasks
- Data Labeling Outsourcing: labeling and categorizing data
- Game Management Outsourcing: managing online games
Top Companies
Alibaba uses data labeling for its e-commerce platform to offer product recommendations to customers based on their purchase history.
Amazon also uses a similar approach to generate product recommendations to consumers with its AI-powered recommendation engine.
Facebook takes facial images of its users and labels them to train algorithms for tagging suggestions on photos.
Microsoft relies heavily on data labeling to develop its Azure services, particularly Azure Machine Learning.
Autonomous vehicle manufacturers like Tesla and Waymo use data labeling to train their models and develop autonomous capabilities.
Voice assistant developers like Google Assistant, Apple's Siri, and Amazon's Alexa also use data labeling to train their models and improve speech recognition.
Here are some examples of companies that use data labeling:
- Alibaba
- Amazon
- Microsoft
- Tesla
- Waymo
- Google Assistant
- Apple's Siri
- Amazon's Alexa
Sources
- Trainings (adasci.org)
- Research (aimresearch.co)
- ScaleAI (scale.com)
- Dataloop AI (dataloop.ai)
- Karya AI (karya.in)
- Appen (appen.com)
- Labelbox (labelbox.com)
- iMerit (imerit.net)
- SuperAnnotate (superannotate.com)
- Kili (kili-technology.com)
- Data classification: What it is and why you need it (computerweekly.com)
- iMerit (imerit.net)
- Scale (scale.com)
- Appen (appen.com)
- Amazon Mechanical Turk (mturk.com)
- CloudFactory (cloudfactory.com)
- brat (nlplab.org)
- ‘smart data’ (forbes.com)
- Data labeling companies (bloomberg.com)
- Leap Steam (leapsteam.com)
- video annotation (keymakr.com)
- data labeling (keylabs.ai)
Featured Images: pexels.com