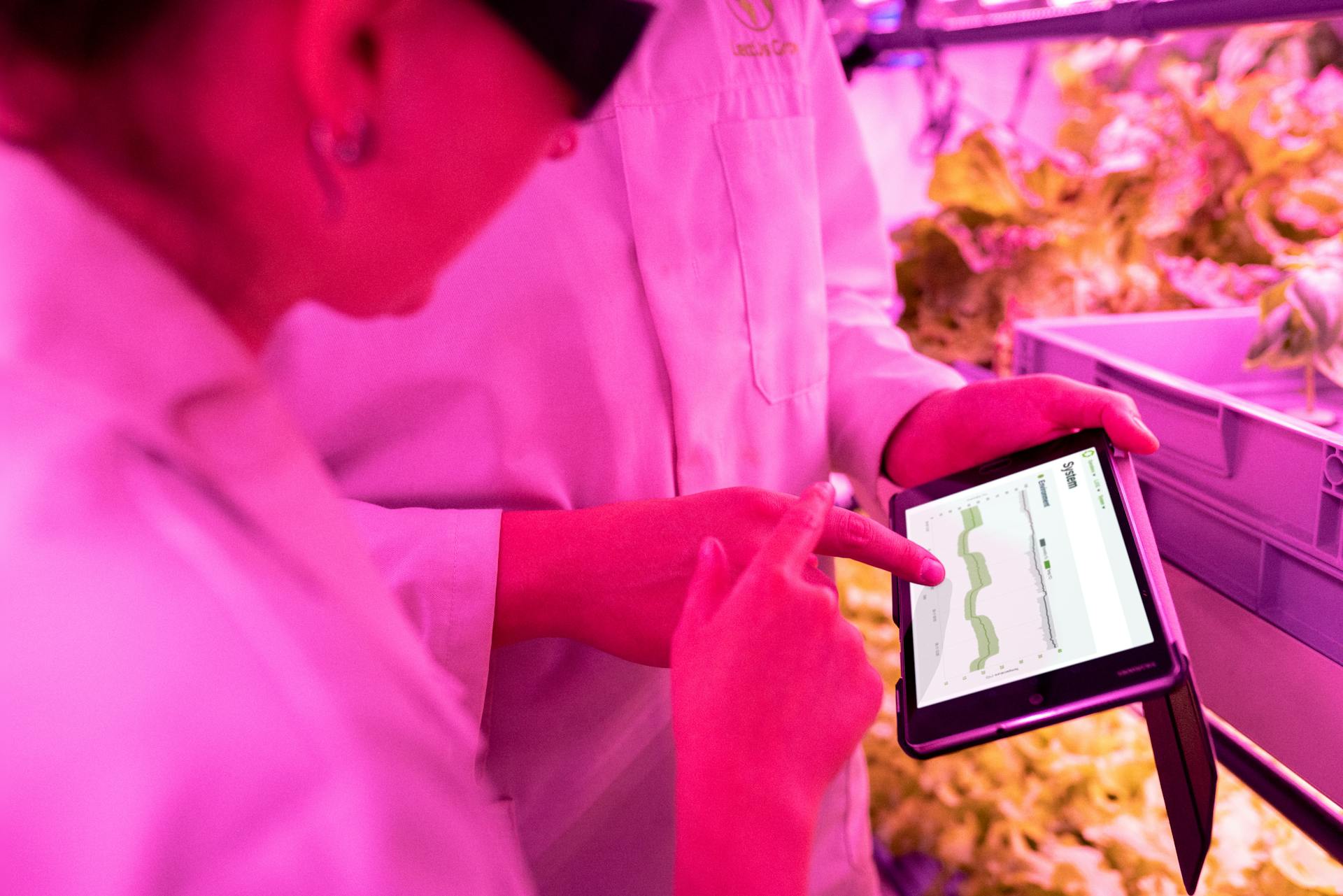
Data science is a field that involves extracting insights and knowledge from data, often using machine learning and statistical techniques. It's like being a detective, searching for clues in a vast amount of data to solve a mystery.
Data science is not just about crunching numbers, but also about understanding the context and story behind the data. According to the article, "Data science involves a multidisciplinary approach, combining statistics, computer science, and domain-specific knowledge to extract insights from data."
AI, on the other hand, refers to the broader field of artificial intelligence, which includes machine learning, natural language processing, and robotics. AI is like a superpower that enables machines to think and act like humans.
AI is not just about creating intelligent machines, but also about making our lives easier and more convenient. According to the article, "AI has the potential to revolutionize industries such as healthcare, finance, and transportation, making them more efficient and effective."
Related reading: Is Ai Computer Science
What Is Data Science?
Data science is a multidisciplinary field that combines statistics, computer science, and domain-specific knowledge to extract insights and knowledge from data.
At its core, data science involves extracting insights and knowledge from data, which is often messy and incomplete, to inform business decisions. Data scientists use a variety of techniques, including data mining, machine learning, and statistical modeling, to identify patterns and trends in data.
The goal of data science is to turn data into actionable insights that can drive business outcomes. This might involve identifying customer segments, predicting customer behavior, or optimizing business processes.
Data science is not just about analyzing data, but also about communicating insights to stakeholders in a clear and actionable way. This requires a deep understanding of the business problem, as well as the ability to present complex data insights in a simple and intuitive way.
Data scientists often use a variety of tools and technologies to extract insights from data, including programming languages like Python and R, as well as specialized software like Tableau and Power BI.
Explore further: Can Generative Ai Solve Computer Science
Relationships Between
The relationships between data science, AI, and ML can be a bit confusing, but let's break it down. AI is the broader concept that enables machines to sense, reason, act, or adapt like humans. Machine learning is an application of AI that allows machines to extract knowledge from data and learn from it autonomously.
Think of AI as an umbrella category that covers various approaches and algorithms, including machine learning, deep learning, robotics, expert systems, and natural language processing. Machine learning is a key component of AI, but it's not the only one.
Here's a simple way to remember the difference: AI is like a car, and machine learning is like the engine that makes the car go. The car (AI) can do many things, but the engine (machine learning) is what powers it.
Let's look at how AI and machine learning work together. An AI system is built using machine learning and other techniques. Machine learning models are created by studying patterns in the data. Data scientists optimize the machine learning models based on patterns in the data. The process repeats and is refined until the models' accuracy is high enough for the tasks that need to be done.
To illustrate this, imagine asking your Google Nest device, "How long is my commute today?" In this case, the device uses AI to perform a task successfully, and machine learning is what allows it to learn from data and provide an accurate answer.
Recommended read: Learn Ai and Ml
Differences and Benefits
Artificial intelligence encompasses the idea of a machine that can mimic human intelligence, but machine learning aims to teach a machine how to perform a specific task by identifying patterns.
Machine learning's scope is limited to identifying patterns, how accurate the prediction was, and learning from the data to maximize performance for that specific task.
AI and ML bring powerful benefits to organizations, including automating tasks, unlocking value, and generating actionable insights to achieve better outcomes.
The business benefits of using AI and ML include automating tasks and unlocking value, but also generating actionable insights to achieve better outcomes.
While AI and ML are closely related, the key difference lies in their interconnection, making it difficult to distinguish between the two without understanding their relationship.
You might enjoy: Self Learning Ai
Differences Between AI, ML, and DS
Artificial intelligence encompasses the idea of a machine that can mimic human intelligence. Machine learning, on the other hand, aims to teach a machine how to perform a specific task and provide accurate results by identifying patterns.
Machine learning is not about enabling a machine to perform a task, but rather about identifying patterns, how accurate the prediction was, and learning from the data to maximize performance for that specific task.
Artificial intelligence and machine learning are very closely related and connected.
The goal of using machine learning in a system is often limited to a specific task, such as analyzing live transit and traffic data to forecast the volume and density of traffic flow.
In this context, the scope of machine learning is focused on identifying patterns and improving performance for that specific task.
Benefits of Using AI, ML, and DS Together
Using AI, ML, and DS together brings numerous benefits to organizations, including automating tasks, unlocking value, and generating actionable insights to achieve better outcomes. This powerful combination is essential for companies of all shapes and sizes, as it helps them stay competitive in today's data-driven world.
AI and ML together help companies automate tasks, which frees up time and resources for more strategic initiatives. By automating manual processes, organizations can also reduce errors and improve efficiency.
The process of using AI and ML together involves building an AI system using machine learning and other techniques. This is done by studying patterns in the data, creating machine learning models, and optimizing them based on those patterns.
Data scientists play a crucial role in this process, optimizing machine learning models to ensure they're accurate enough for the tasks at hand. This process is repeated and refined until the models are high enough in accuracy.
Here are some of the key benefits of using AI, ML, and DS together:
By incorporating AI, ML, and DS into their systems and strategic plans, leaders can understand and act on data-driven insights with greater speed and efficiency. This is essential for companies looking to transform their data into actionable insight and stay ahead of the competition.
Applications of AI, ML, and DS in Various Industries
Companies across various industries are leveraging AI and ML to transform their operations and business models. This includes using data and resources more effectively, driving productivity and efficiency, and enhancing decision-making through predictive analytics.
Companies in several industries are building applications that take advantage of the connection between AI and machine learning. These applications are helping companies transform their processes and products.
In the banking and finance sector, AI and machine learning are being used to detect fraud and predict risk. This proactive approach is providing more effective financial advice to customers.
AI and ML capabilities are being incorporated into strategies and systems across industries, helping organizations rethink how they use their data and available resources.
Additional reading: Applications of Ai and Ml
Process and Cases
Data science, machine learning, and deep learning are all interconnected fields that work together to help us make sense of data and solve real-world problems. The data science process is all about extracting insights from data, and it's closely related to machine learning and deep learning.
Machine learning is a key component of the data science process, and it's used in spam detection to identify and filter out unwanted emails. This is a great example of how machine learning can be used to solve a practical problem.
Deep learning is a type of machine learning that's particularly good at image recognition tasks, like identifying objects in pictures. It's also used in speech recognition technologies, like Google Translate, which can convert spoken words into text in over 100 languages.
Process Cases for AI, ML, and DS
Data science, machine learning, and artificial intelligence are interconnected fields that work together to extract insights and value from data.
The data science process is closely related to machine learning, which is a subset of artificial intelligence.
Data mining is also a key component of the data science process, involving the extraction of patterns and relationships from large datasets.
Data science, machine learning, and artificial intelligence are often used in various use cases, including predictive analytics, natural language processing, and computer vision.
Artificial intelligence is a broad field that encompasses machine learning, deep learning, and data mining, with the goal of creating intelligent machines that can perform tasks autonomously.
Machine learning is a key component of artificial intelligence, enabling machines to learn from data and improve their performance over time.
The data science process involves several stages, including data collection, data preprocessing, and model deployment, which are all crucial for extracting insights and value from data.
Data science, machine learning, and artificial intelligence are often used in conjunction with each other to solve complex problems and make informed decisions.
Mining Process and Cases for AI, ML, and DS
Data mining is a crucial step in the data science process, helping us uncover hidden patterns and trends in large datasets. It's a powerful tool that can be used to identify customer behavior, like Amazon's "Frequently bought together" feature.
The data mining process involves several steps, including data selection, data cleaning, and data transformation, as outlined in the data mining process and use cases section. These steps are essential to prepare the data for analysis.
One of the most interesting applications of data mining is in marketing, where it can help businesses identify new trends and opportunities. For instance, Amazon's use of data mining has led to the creation of this "Frequently bought together" feature.
Data mining can also be used to optimize business processes, like reducing waste and improving efficiency. By analyzing data from various sources, businesses can identify areas where improvements can be made.
The machine learning process often relies on data mining to prepare the data for analysis. This is evident in the example of spam detection, where machine learning algorithms are trained on data that has been mined for relevant features.
Readers also liked: Ai Ml Use Cases
Frequently Asked Questions
Who earns more, AI/ML or data science?
According to current data, AI/ML engineers tend to earn more than data scientists, with median salaries ranging from $98,000 to $137,000 annually. However, salaries can vary widely depending on experience and location.
Is ML harder than data science?
While both machine learning and data science require advanced skills, machine learning is more mathematically intensive, requiring expertise in programming, statistics, and algorithm development. Data science, on the other hand, demands a broader range of skills, including subject matter expertise and data manipulation.
Sources
- https://www.deviq.io/insights/artificial-intelligence-vs-machine-learning-vs-data-science
- https://www.altexsoft.com/blog/data-science-artificial-intelligence-machine-learning-deep-learning-data-mining/
- https://cloud.google.com/learn/artificial-intelligence-vs-machine-learning
- https://ai.engineering.columbia.edu/ai-vs-machine-learning/
- https://azure.microsoft.com/en-us/resources/cloud-computing-dictionary/artificial-intelligence-vs-machine-learning
Featured Images: pexels.com