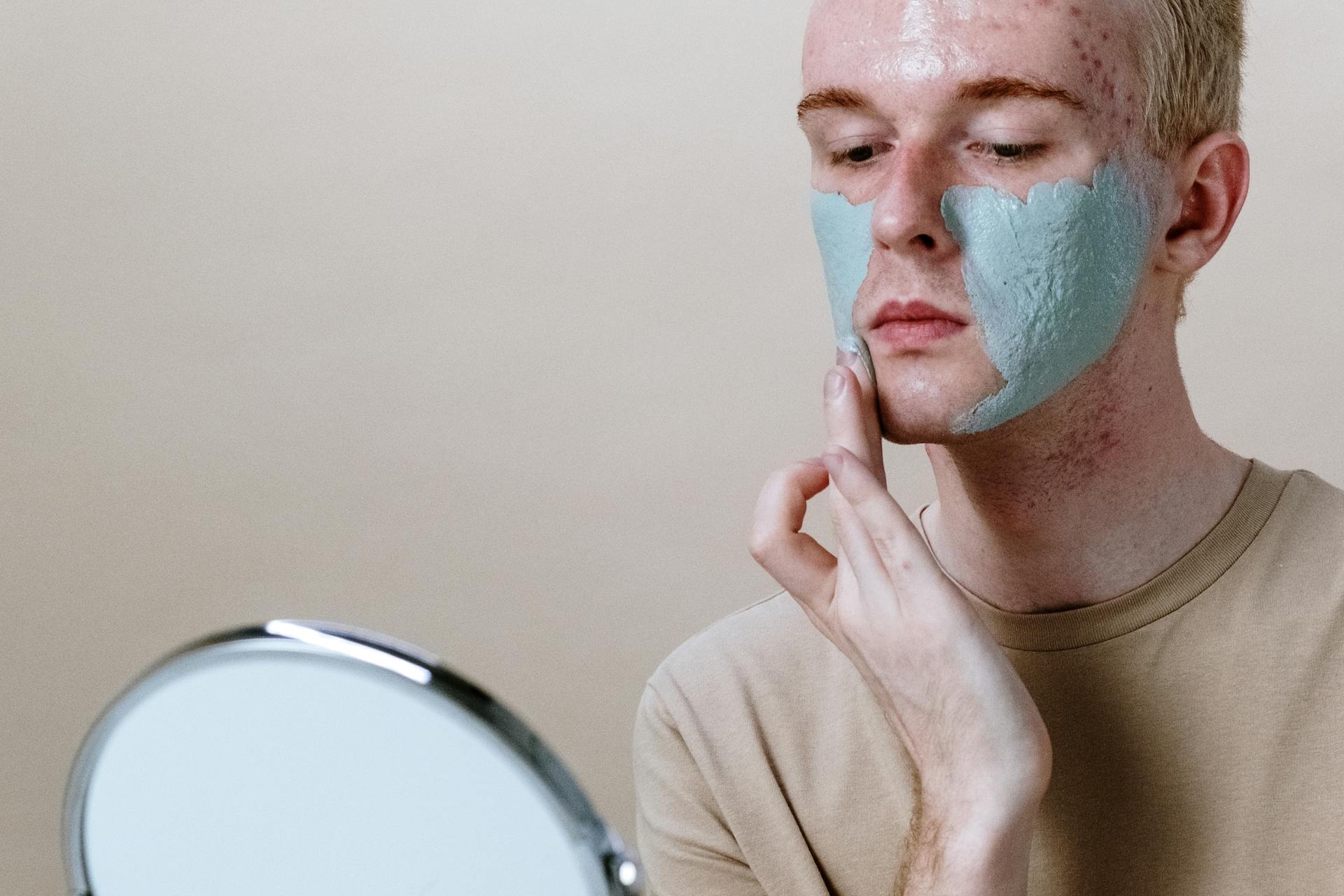
Genai Image is a revolutionary technology that has taken the world by storm.
It's a type of artificial intelligence-powered image generation that can create realistic images from text descriptions.
At its core, Genai Image uses a complex algorithm to analyze and generate images based on the input text.
This technology has the potential to change the way we interact with images and information.
Genai Image can be used in various applications, including art, design, and even education.
For example, it can be used to create custom artwork or designs based on user input.
See what others are reading: Knowledge Based Genai
What is Genai Image?
Genai image is a type of AI-generated image that utilizes trained artificial neural networks to create original, realistic visuals based on textual input.
These generators are trained on extensive amounts of data, which comprise large datasets of images, allowing them to learn different aspects and characteristics of the images within the datasets.
Through the training process, AI image generators become capable of generating new images that bear similarities in style and content to those found in the training data.
Consider reading: Ai Image Training
AI image generators can fuse styles, concepts, and attributes to fabricate artistic and contextually relevant imagery, making them particularly remarkable.
Generative AI, a subset of artificial intelligence, is focused on content creation, which enables AI image generators to produce original images from scratch.
There are various types of AI image generators, including neural style transfer technique, which enables the imposition of one image's style onto another, and diffusion models, which generate images through a process that simulates the diffusion of particles.
History
Generative AI has a rich history that spans several decades.
The earliest example of generative AI was the Eliza chatbot created by Joseph Weizenbaum in the 1960s.
These early implementations used a rules-based approach that broke easily due to a limited vocabulary, lack of context, and overreliance on patterns.
The field saw a resurgence in the wake of advances in neural networks and deep learning in 2010.
This breakthrough enabled generative AI to automatically learn to parse existing text, classify image elements, and transcribe audio.
In 2014, Ian Goodfellow introduced GANs, a deep learning technique that allowed competing neural networks to generate and rate content variations.
GANs enabled the creation of realistic people, voices, music, and text, sparking both interest and fear about the potential misuse of deepfakes.
Explore further: Getty Images Nvidia Generative Ai Istock
Popular Models and Tools
Generative AI models have made significant progress in recent years, enabling the creation of realistic human faces, synthetic data, and even facsimiles of particular humans. Techniques like GANs, VAEs, and transformers have been used to achieve this.
Midjourney is considered one of the most powerful generative AI tools, focusing on creating artistic and stylized images. It's popular for its high quality, but has some limitations, including being on the slower end and not depicting people, even animated ones.
DALL-E 3 is a highly advanced tool that generates detailed depictions of text descriptions, allowing users to create original images and modify existing ones. However, it has some limitations, including only generating one image at a time and lacking diversity and variety in its results.
Canva Magic Design is a user-friendly AI image generator that brings the power of AI to the masses, allowing users to generate images, graphics, or videos in various aspect ratios and visual styles. It's fast and easy to use, but may struggle with recreating finer details like hands, fingers, and eyes.
Here's an interesting read: What Is One of the Key Challenges Faced by Genai
Here are some key features of popular AI image generators:
These tools offer a range of features and capabilities, from artistic and stylized images to highly advanced and detailed depictions. However, each has its own limitations and trade-offs, and users should carefully consider their needs and goals before choosing a tool.
How They Work
Genai image generators are trained on millions of existing images, descriptions, and captions available across the internet. This training process allows them to "learn" what things look like and what they are called.
The AI reaches into its memory of those images and captions when you enter a prompt into a tool. It then produces an image or selection of images it believes fit your prompt.
The training process involves two core components: the generator and the discriminator. The generator creates fake samples, while the discriminator classifies them as real or fake. This adversarial game ensures that both networks are continually learning and improving.
Generative Adversarial Networks (GANs) are a type of machine learning algorithm that harness the power of two competing neural networks. The generator aims to produce fake samples that are indistinguishable from real data, while the discriminator endeavors to accurately identify whether a sample is real or fake.
Here's a breakdown of the GANs architecture:
- The generator neural network creates fake samples.
- The discriminator neural network functions as a binary classifier, determining whether a sample is real or fake.
The process of generating images with AI is a gradual one, much like "diffusion." It starts with random noise and gradually refines the image to align it with the textual description provided. This is achieved through the use of the Latent Diffusion Model (LDM).
Applications and Use Cases
Genai image technology has the potential to spur creativity among artists, allowing them to explore new ideas and styles.
These tools can also serve as a valuable resource for educators, helping to explain complex concepts through visual aids.
By rapidly visualizing new designs, genai image technology can accelerate the product design process, saving time and increasing efficiency.
Marketing and Advertising
Marketing and advertising just got a whole lot easier with the help of AI. AI-generated images can produce campaign visuals in a snap, eliminating the need for photo shoots.
Marketers can use AI to generate high-quality images that can be used in promotional materials. For instance, the magazine Cosmopolitan used AI to create a cover entirely generated by artificial intelligence.
The Cosmopolitan cover was created using DALL-E 2, an AI-powered image generator developed by OpenAI. The input given to DALL-E 2 was "A wide angle shot from below of a female astronaut with an athletic female body walking with swagger on Mars in an infinite universe, synthwave, digital art."
The result was an intricate and futuristic illustration of a female astronaut on Mars, marking the first time an AI-generated image was used as the cover of a major magazine.
Here's an interesting read: What Are the Four Commonly Used Genai Applications
Medical Imaging
Medical Imaging has seen significant advancements with the help of AI image generators. They can generate clearer and more detailed images of tissues and organs, leading to more accurate diagnoses.
In a study, researchers used DALL-E 2 to explore its capabilities in the medical field, specifically for generating and manipulating radiological images. DALL-E 2 was proficient in creating realistic X-ray images from short text prompts.
DALL-E 2 can even reconstruct missing elements in a radiological image, such as creating a full-body radiograph from a single knee image. However, it struggled with generating images with pathological abnormalities.
The synthetic data generated by DALL-E 2 can potentially speed up the development of new deep-learning tools in radiology and address privacy issues concerning data sharing between medical institutions.
Recommended read: Medical Imaging Ai Software Developer
Applications and Use Cases
AI image generators can be a valuable tool for educators, helping to spur creativity and engagement in students.
These tools can accelerate the product design process by rapidly visualizing new designs, saving time and resources.
AI image generation technology has myriad applications, including serving as a valuable tool for educators.
For instance, these tools can help artists explore new ideas and styles, opening up new possibilities for creative expression.
AI image generators can also be used to rapidly visualize new designs, making it easier to test and refine ideas.
Will AI Replace Human Artists?
AI image generators will likely serve as a tool to assist and empower artists, rather than replace them. This is because AI still lacks the nuanced creativity and emotion that human artists bring to their work.
Artists like Kevin Kelley have noted that AI image generators are inherently limited by their reliance on text prompts, which can be stifling. They can't capture the art that humans create that can't be reduced to language.
The artistic process can be enriched by AI, offering new avenues for exploration and facilitating the production of high-quality art.
Limitations and Controversies
AI image generators can create visually stunning and often hyperrealistic imagery, but they also bring several limitations and controversies.
One of the limitations is the quality and authenticity issues, where AI systems struggle to produce images free from imperfections or representative of real-world diversity. The inability to generate flawless human faces is a significant hurdle, with AI models like StyleGAN and DALL-E often producing faces with subtle imperfections or unrealistic features.
The authenticity and quality of AI-generated images heavily depend on the datasets used to train the models, which can lead to bias. For example, a study by Joy Buolamwini at the MIT Media Lab found significant biases in commercial AI gender classification systems across different skin tones and genders.
Achieving the desired level of detail and realism requires meticulous fine-tuning of model parameters, which can be complex and time-consuming. This is particularly evident in the medical field, where AI-generated images used for diagnosis need to have high precision.
AI-generated images might inadvertently resemble existing copyrighted material, leading to legal issues regarding infringement. In January 2023, three artists filed a lawsuit against top companies in the AI art generation space, claiming that the companies were using copyrighted images to train their AI algorithms without their consent.
Determining who owns the rights to images created by AI is a gray area, as seen in the case where an AI-generated artwork won first place at the Colorado State Fair's fine arts competition.
Best Practices and Future
To use genai image effectively, it's essential to consider accuracy, transparency, and ease of use. Clearly label all genai image content for users and consumers, and vet the accuracy of generated content using primary sources where applicable.
To ensure the quality of genai image results, double-check the quality of AI-generated content using other tools, and learn the strengths and limitations of each genai image tool. This will help you work around common failure modes in results.
Here are some best practices to keep in mind:
- Clearly label all genai image content for users and consumers.
- Vet the accuracy of generated content using primary sources where applicable.
- Consider how bias might get woven into generated AI results.
- Double-check the quality of AI-generated content using other tools.
- Learn the strengths and limitations of each genai image tool.
- Familiarize yourself with common failure modes in results and work around these.
The future of genai image is exciting, with advancements in translation, drug discovery, anomaly detection, and the generation of new content on the horizon. Generative AI will continue to evolve, making tools like grammar checkers and design tools more efficient and effective.
Best Practices
To use generative AI effectively, consider essential factors like accuracy, transparency, and ease of use. This will help you achieve your goals and avoid potential pitfalls.
Explore further: What Are Genai Use Cases Agents Chatbots
Clearly labeling all generative AI content for users and consumers is crucial. This ensures that users understand what they're interacting with and can make informed decisions.
Vetting the accuracy of generated content using primary sources is a must. This helps prevent the spread of misinformation and builds trust with your audience.
Bias can be a major issue in generative AI results. Be aware of how bias might be woven into your results and take steps to mitigate it.
Double-checking the quality of AI-generated code and content is essential. Use other tools to verify the accuracy and reliability of your results.
Learning the strengths and limitations of each generative AI tool is vital. This will help you choose the right tool for the job and avoid frustration.
Familiarizing yourself with common failure modes in results is also important. This will help you work around potential issues and achieve better outcomes.
Here are some best practices to keep in mind:
- Clearly label all generative AI content.
- Vet the accuracy of generated content using primary sources.
- Consider how bias might get woven into generated AI results.
- Double-check the quality of AI-generated code and content.
- Learn the strengths and limitations of each generative AI tool.
- Familiarize yourself with common failure modes in results.
The Future of
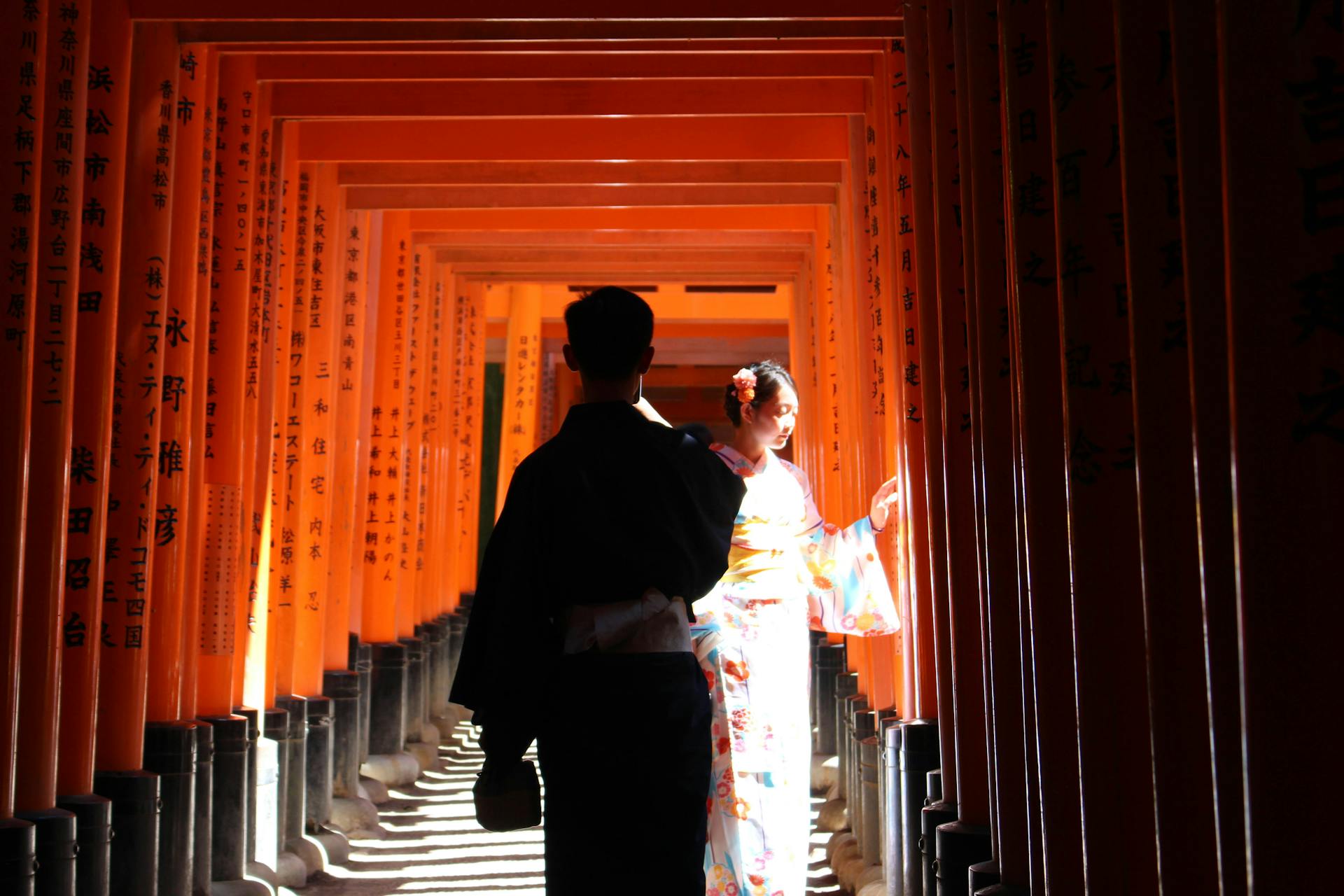
Generative AI is evolving at a rapid pace, making advancements in translation, drug discovery, anomaly detection, and the generation of new content, from text and video to fashion design and music.
The widespread adoption of generative AI tools like ChatGPT has spurred research into better tools for detecting AI-generated text, images, and video.
Industry and society will build better tools for tracking the provenance of information to create more trustworthy AI.
Generative AI will continue to integrate capabilities directly into the tools we already use, making them more efficient and effective.
Grammar checkers will get better, design tools will seamlessly embed more useful recommendations, and training tools will automatically identify best practices to help train other employees more efficiently.
As we continue to harness generative AI tools, we will find ourselves reevaluating the nature and value of human expertise.
Worth a look: Generative Ai by Getty Images
What's New
You can now create professional product photos using AI, which is a game-changer for product shoots. This technology allows you to upload and integrate your product into various AI-generated scenes, reducing production costs and turnaround times.
With Getty Images' AI image generation service, you can maintain consistency in lighting, background, and style while staying aligned with seasonality and trends. This means you can create a cohesive look for your product line without breaking the bank.
You can quickly create storyboards and concepts prior to your official shoots, which saves time and ensures you're on the right track from the start.
Here are some benefits of using Getty Images' AI image generation service:
- Lower production costs
- Faster turnaround times
- Consistency in lighting, background, and style
- Alignment with seasonality and trends
You can also upload your own photos or illustrations to use as reference images, which creates commercially safe AI-generated images based on their composition or color. This feature allows you to use your own creative assets while still benefiting from AI-generated images.
Curious to learn more? Check out: Genai Use Cases
Testing and Evaluation
Testing and evaluation are crucial steps in the genai image development process.
A good testing framework can help identify and fix issues early on, reducing the risk of costly rework later on.
Genai images are typically tested using a combination of manual and automated methods.
Automated testing can be done using tools such as AI-powered testing frameworks, which can simulate user interactions and test the image's functionality.
Manual testing is also important to ensure that the image behaves as expected in different scenarios.
In genai image development, testing and evaluation are often done in parallel with development to ensure that the image meets the required specifications.
This approach helps to catch errors and issues early on, reducing the overall development time and cost.
Genai images are often evaluated based on their performance, accuracy, and user experience.
These factors are critical in determining the overall success of the image in a real-world application.
Ethics and Bias
The convincing realism of genai image makes it harder to detect AI-generated content, which can be a big problem when we rely on it to provide accurate results.
Microsoft's Tay chatbot in 2016 had to be turned off after it started spewing inflammatory rhetoric on Twitter, a clear example of the potential risks of genai image.
The combination of humanlike language and coherence in genai image is not synonymous with human intelligence, and there's great debate about whether genai image models can be trained to have reasoning ability.
One Google engineer was even fired after publicly declaring the company's LaMDA app was sentient, highlighting the complexities and uncertainties surrounding genai image.
Many results of genai image are not transparent, making it hard to determine if they infringe on copyrights or if there are problems with the original sources from which they draw results.
Sources
Featured Images: pexels.com