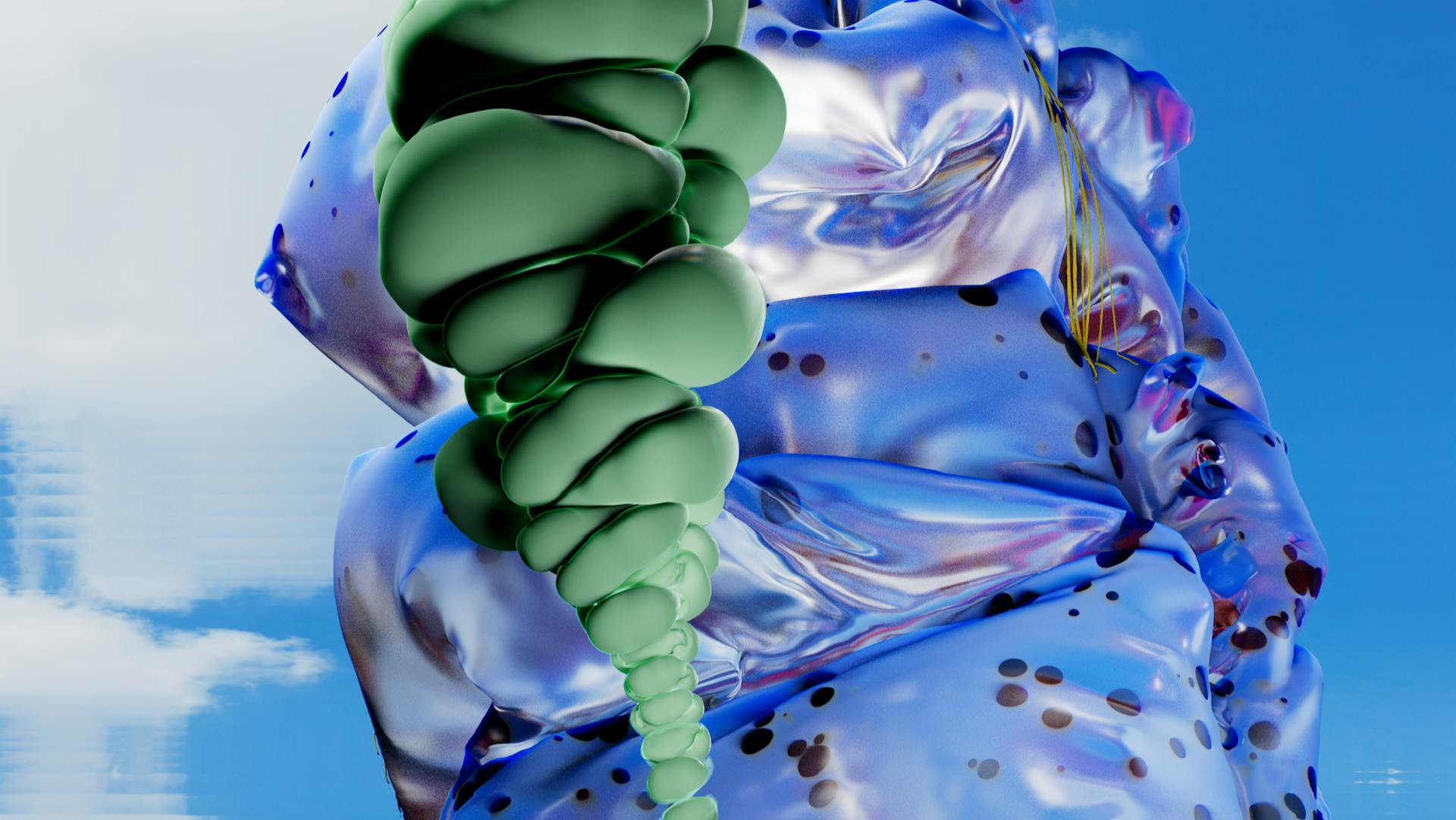
GenAI, or General Artificial Intelligence, is still in its early stages of development, but it's already facing significant challenges. One of the key challenges faced by GenAI in the modern world is the issue of explainability.
The lack of transparency and interpretability of AI decision-making processes is a major concern. According to research, humans are not able to understand why AI models make certain decisions, which can lead to a lack of trust in the technology. This is particularly problematic in high-stakes applications such as healthcare and finance.
The complexity of AI systems is a significant contributor to this issue. AI models are often composed of numerous layers and components, making it difficult to pinpoint exactly how they arrive at a particular decision. This complexity can also lead to AI systems being vulnerable to bias and errors.
As GenAI continues to evolve, it's essential that developers prioritize explainability and transparency in their designs.
Take a look at this: Artificial Intelligence Is the Theory and Development of Computer Systems
Key Challenges
Managing costs is a huge issue when it comes to implementing genAI, as it requires high compute intensity and change management.
McKinsey's Baig stresses that organizations must invest as much in managing costs as they do in digital transformation, as genAI requires changes in workflows, business processes, and new KPIs.
The scarcity and cost of hardware, power, and data needed to train models are fundamental barriers to adopting genAI.
CNH's Kermisch notes that platforms are very expensive to implement and genAI becomes more expensive as it's utilized.
The phenomenon of the "shiny new tool" is real, with users getting excited about new technology but quickly losing interest.
Utilization of genAI tools like Copilot tends to drop off dramatically within 30 days, with users realizing they can't use it effectively.
Finding the right prompt is key to using genAI tools like Copilot, but even then, it's hard to find power users who can utilize it effectively.
Monitoring adoption and use of genAI tools is a challenge, with IT teams struggling to determine whether the tool is a good investment.
Discover more: Genai Copilot
Ethical and Regulatory Concerns
Ethical and Regulatory Concerns are crucial when it comes to GenAI implementation. Ensuring transparency, accountability, and ethical alignment in GenAI decisions is a significant challenge.
Developing and adhering to ethical guidelines prioritizing transparency and accountability can help mitigate this issue. Implementing mechanisms for the explainability of AI decisions is also essential. This can be achieved by establishing governance structures with oversight mechanisms and ethical review boards.
GenAI models have the added risk of seeking training data at massive scale, without considering the creator's approval, which could lead to copyright issues. Algorithmic bias is another source of legal risk, as flawed AI models can propagate downstream bias in the data sets.
Here are some key areas to focus on to address these concerns:
- Conduct comprehensive bias assessments on training data.
- Utilize diverse datasets and employ techniques such as adversarial testing to detect and address biases.
- Involve diverse stakeholders in AI development to ensure fairness and inclusivity.
- Implement content moderation policies and tools to identify and mitigate harmful content.
Bias and Fairness
Bias and Fairness is a crucial aspect of Generative AI (GenAI) that requires careful attention. Flawed AI models can propagate downstream bias in the data sets, and the homogenization of such models can lead to a single point of failure.
For your interest: Key Challenges Faced by Genai Models
Algorithmic bias is a significant source of legal risk, as it can lead to discrimination and unfair outcomes. This can happen when GenAI models are trained on faulty, incomplete, or unrepresentative data.
Conducting comprehensive bias assessments on training data is essential to mitigate biases in GenAI algorithms. This involves utilizing diverse datasets and employing techniques such as adversarial testing to detect and address biases.
Involving diverse stakeholders in AI development is also crucial to ensure fairness and inclusivity. This can include experts from various fields, as well as individuals from underrepresented groups.
Here are some key steps to address bias and fairness in GenAI:
- Conduct comprehensive bias assessments on training data.
- Utilize diverse datasets.
- Employ techniques such as adversarial testing to detect and address biases.
- Involving diverse stakeholders in AI development.
Monitoring AI Misuse and Hallucinations
Monitoring AI misuse and hallucinations is crucial in the era of generative AI systems.
AI models can be easily modified by threat actors to create deep fakes that closely mimic the original content.
Digitally altered media can include voice and video impersonation, fake art, and targeted attacks.
Hallucinations, or made-up "facts", can occur in AI tools' responses, with rates ranging from 10% to 20% depending on the domain.
A different take: Gen Ai Hallucinations
Technical Challenges
Handling technical complexity can be a significant challenge for organizations looking to adopt generative AI (genAI). Generative AI models are incredibly large, with billions or even trillions of parameters, making them impractically large to train for most organizations.
These models require massive compute resources, which can be expensive and ecologically unfriendly. As Arun Chandrasekaran from Gartner notes, most near-term adoption will likely see businesses consuming genAI through cloud APIs with limited tuning.
The difficulty in creating models leads to another issue: the concentration of power in a few, deep-pocketed entities. This can limit access to genAI for smaller organizations or those with limited resources.
Managing costs is a huge issue when implementing genAI. Organizations must invest in managing costs, as the high compute intensity and change management required by genAI can be costly.
The scarcity and cost of hardware, power, and data needed to train models are fundamental barriers to adopting genAI. As Higginson notes, "With such scarcity comes the need to prioritize which solutions have the broadest appeal to the population and can generate the most long-term revenue."
Handling Technical Complexity
Handling technical complexity is a significant challenge in implementing Generative AI. Generative AI models can contain billions or even trillions of parameters, making them impractically large to train for most organizations.
These models require massive compute resources, which can be expensive and ecologically unfriendly. As a result, most near-term adoption will likely see businesses consuming generative AI through cloud APIs with limited tuning.
The difficulty in creating models leads to another issue: the concentration of power in a few, deep-pocketed entities. This is a concern, as it may limit opportunities for smaller organizations to adopt and benefit from Generative AI.
To mitigate these challenges, organizations can invest in high-performance computing infrastructure, including GPUs and specialized hardware accelerators. Leverage cloud computing services to scale computational resources as required, and optimize algorithms and model architectures for efficiency.
Here are some key considerations for handling technical complexity:
- Invest in high-performance computing infrastructure
- Leverage cloud computing services
- Optimize algorithms and model architectures for efficiency
By taking these steps, organizations can better handle the technical complexity of Generative AI and unlock its potential benefits.
Tackling Legacy Systems
Tackling Legacy Systems can be a daunting task, especially when incorporating generative AI into the mix. IT leaders will face decisions on whether to integrate or replace older systems.
Legacy systems can be a significant roadblock to adopting new technologies, including generative AI. This is because they may not be compatible with newer systems, or may require significant updates to work with AI.
Incorporating generative AI into older technology environments could raise additional issues for enterprises. IT leaders will face decisions on whether to integrate or replace older systems.
Legacy systems can be a significant roadblock to adopting new technologies, including generative AI. This is because they may not be compatible with newer systems, or may require significant updates to work with AI.
In some cases, it may be more cost-effective to replace older systems rather than trying to integrate them with new technologies.
Intriguing read: How Generative Ai Is Changing Creative Work
Avoiding Technical Debt
Avoiding Technical Debt is crucial when implementing new technologies, especially Generative AI.
Generative AI can easily become a source of technical debt if not properly integrated into existing systems.
Businesses need to achieve significant change through its adoption to avoid adding more debt to their processes.
Reducing workload, like having human agents handle fewer cases, is not enough to justify the investment in AI.
A business needs to significantly reduce the number of agents in front-line support roles to truly optimize their processes.
If you don't take something away, you haven't optimized, you've just added more debt.
See what others are reading: What Are Genai Use Cases Agents Chatbots
Safety and Reliability
Ensuring GenAI systems operate safely and reliably is a top priority. Prioritizing safety in AI design is essential, especially in critical applications like healthcare and autonomous systems.
Rigorous testing and validation are crucial steps in guaranteeing GenAI systems' safety and reliability. This includes adhering to established industry norms and implementing fail-safe mechanisms to prevent accidents.
Collaboration with stakeholders is vital in establishing and upholding safety standards specific to GenAI. By working together, we can create a safer and more reliable GenAI ecosystem.
Here's a breakdown of the key aspects to consider:
- Rigorous testing and validation
- Adherence to established industry norms
- Implementation of fail-safe mechanisms
- Collaboration with stakeholders
By prioritizing safety and reliability, we can unlock the full potential of GenAI while minimizing the risk of accidents and errors.
Impact and Employment
One of the key challenges faced by GenAI is its potential impact on employment. Job displacement and changes in job roles are a significant concern due to automation.
Investing in workforce development programs for reskilling and upskilling can help mitigate this issue. This can include ongoing learning and adaptability within organizations.
Cultivating an environment that encourages collaboration between humans and AI can lead to the creation of new roles and enhanced productivity.
Impact on Employment:
The impact of GenAI on employment is a pressing concern. Job displacement and changes in job roles are potential challenges that need to be addressed.
Investing in workforce development programs for reskilling and upskilling is a crucial solution. This can help employees adapt to new technologies and stay relevant in the job market.
Organizations can cultivate an environment of ongoing learning and adaptability by providing training and resources for their employees. By doing so, they can stay ahead of the curve and remain competitive.
Here are some potential solutions to mitigate the impact of GenAI on employment:
- Reskilling and upskilling programs for employees
- Cultivating an environment of ongoing learning and adaptability
- Exploring collaboration opportunities between humans and AI
These solutions can help create new roles and enhance productivity, ultimately benefiting both employees and organizations.
GenAI's Long-Term Impact
The honeymoon phase is over, and we're starting to see the hard truths of GenAI. Only 15% of companies have a line of sight into earning improvements from GenAI initiatives, according to McKinsey.
GenAI is not a panacea, and it's not going to solve all our problems overnight. UK-based CNH, an early adopter of Microsoft's Copilot, has found that GenAI is primarily an information synthesizing tool, and off-the-shelf GenAI tools have not been effective for financial or number analysis.
However, IT leaders recognize that GenAI isn't going anywhere, and it's going to be a bumpy ride with some spectacular successes and failures. Phoenix Hospital's Higginson says it's going to be a wild ride over the next five to 10 years.
GenAI requires a strategy and roadmap to be implemented properly, and it's not as easy as the public is experiencing with OpenAI or other generative AI tools. Dave Pawlak, executive director of IT at Consumers Energy, emphasizes the importance of implementing GenAI securely.
The good news is that GenAI will allow you to go from pilot to scale, and it's an incredible opportunity to take advantage of this technology. Aamer Baig, a senior partner and global leader at McKinsey, believes that CIOs need to play a leading role in leading organizations forward.
Frequently Asked Questions
What is one of the key challenges faced by GenAI in terms of consistency tcs?
One key challenge faced by GenAI in terms of consistency is finding a balance between exploring new possibilities and exploiting known good solutions. This delicate balance is crucial for GenAI to consistently improve its performance.
Sources
- https://educationaltechnologyjournal.springeropen.com/articles/10.1186/s41239-023-00411-8
- https://amazic.com/10-regulatory-challenges-with-genai-and-steps-to-overcome-them/
- https://www.cio.com/article/3478772/6-hard-truths-of-generative-ai-in-the-enterprise.html
- https://www.techtarget.com/searchenterpriseai/tip/Generative-AI-challenges-that-businesses-should-consider
- https://www.cloud4c.com/blogs/generative-ai-challenges-for-businesses-and-how-to-solve-them
Featured Images: pexels.com