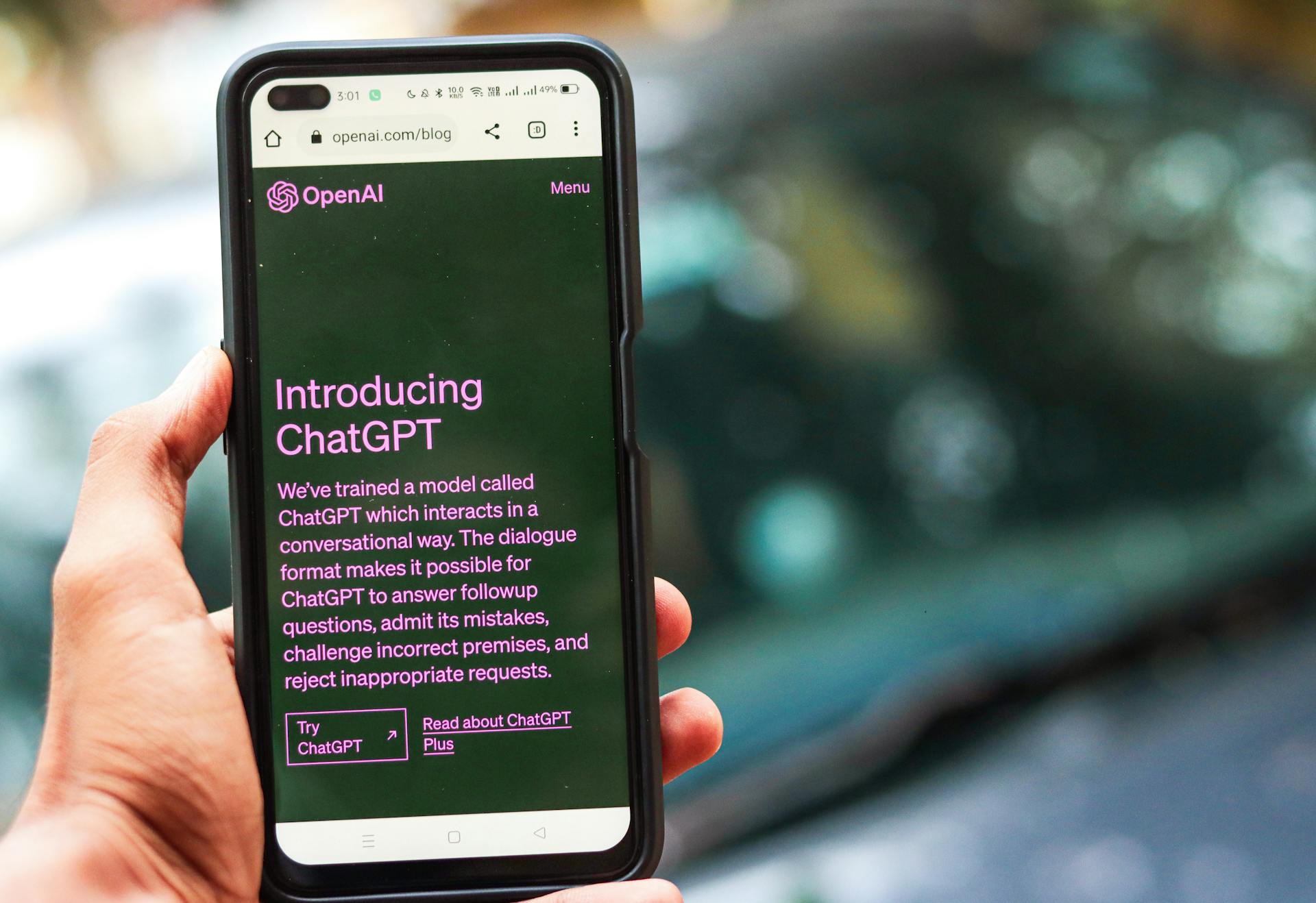
Generative AI faces several challenges that hinder its widespread adoption and effectiveness. One major challenge is the lack of control over the generated content, which can lead to unexpected and often undesirable outcomes.
The issue of data quality and availability is a significant one, as generative AI models require large amounts of high-quality data to learn from. If the data is biased, incomplete, or inaccurate, the generated content will likely reflect these flaws.
Another challenge is the difficulty in evaluating the quality and reliability of generative AI outputs. This is particularly true for tasks that require human judgment, such as content creation or decision-making.
Generative AI models can also be vulnerable to adversarial attacks, which are designed to manipulate the model into producing incorrect or misleading results. This is a concern in applications where the stakes are high, such as in healthcare or finance.
Data Quality and Quantity
Generative AI models need vast amounts of high-quality data to function effectively. A study found that an image generation model trained on low-resolution images produced blurry and unrealistic images. Ensuring data quality is crucial for AI development.
A model trained on a large dataset of high-quality images generated sharp and detailed images. This highlights the importance of utilizing data quality properly.
If this caught your attention, see: Getty Generative Ai
Data Quality and Quantity
Generative AI models need vast amounts of high-quality data to function effectively. Inadequate or poor-quality data can lead to subpar results and limit the effectiveness of the AI.
A study found that an image generation model trained on low-resolution images produced blurry and unrealistic images. On the other hand, a model trained on a large dataset of high-quality images generated sharp and detailed images.
High-quality data is essential for generating accurate results. This is especially true for image generation models, where the quality of the input data directly affects the output.
Training a generative AI model on low-quality data can lead to subpar results. This can be seen in the example of an image generation model trained on low-resolution images, which produced blurry and unrealistic images.
In contrast, a model trained on a large dataset of high-quality images generated sharp and detailed images. This highlights the importance of ensuring and utilizing high-quality data in generative AI development.
The quality of the input data has a direct impact on the accuracy of the output. This is why it's essential to use high-quality data when training generative AI models.
Check this out: Generative Ai Photoshop Increase Quality
Common
Generative AI can exhibit biases, compromise data privacy, misinterpret prompts, and produce hallucinations, which can lead to patient harm and other unintended consequences.
The rapid uptake and integration of generative AI technology can make it difficult for digital health practitioners to appreciate its limitations.
Generative AI tools can create text, images, and other content, and are already being deployed in many medical settings.
Failure to understand the limitations of generative AI can lead to misuse, which can have serious consequences.
Generative AI can compromise data privacy, which is a major concern in medical settings.
Bias is another challenge with generative AI, and it can be difficult to detect and mitigate.
Hallucinations, or the creation of fictional information, can also occur with generative AI.
The productivity enhancements brought about by generative AI tools are undeniable, especially among new employees.
Consider reading: Top Generative Ai Tools
Model Limitations and Risks
Model limitations and risks are a significant concern when it comes to generative AI. Generative AI models face several limitations, including bias, AI hallucination, bugs, and security issues. These limitations can lead to inaccurate or misleading information being generated.
Take a look at this: Limitations of Generative Ai
Bias is a major issue, with 57.5% of papers reviewed highlighting bias as a concern for medical practitioners or patients using AI assistance for medical decisions. Debiasing techniques can be employed, but their efficacy is still a subject of debate. Some debiasing methods might correct for one form of bias but introduce another.
AI hallucination is another significant limitation, where AI models generate wrong information with no credible sources. This can be a serious issue in cases where users rely on AI chatbots for solid information. A complex combination of factors can cause hallucinations, making it challenging to identify the root causes.
These limitations can have real-life consequences, such as when AI chatbots generate misinformation or when users rely on AI for medical advice without proper oversight.
Here are some of the main limitations of generative AI models:
- Bias
- AI hallucination
- Bugs
- Security
These limitations highlight the need for careful consideration and evaluation of generative AI models before implementing them in medical settings.
Model Limitations
Generative AI models face several limitations that users and developers should consider. These limitations include bias, AI hallucination, bugs, and security issues.
Bias is a significant concern in generative AI models, with 58% of papers addressing this issue in a recent review. This bias can manifest in various ways, such as perpetuating stereotypes or discriminating against certain groups.
AI hallucination is another common limitation, where AI models generate information that is not based on credible sources. This can be a serious issue, especially when users rely on AI chatbots for solid information. In fact, 64% of papers in the review mentioned model hallucinations as a major concern.
Bugs and errors can also occur in generative AI models, as seen in the case of ChatGPT, where a user reported a bizarre phenomenon of the chatbot producing garbled sentences. This incident was due to an error in the way the base model assigned context tokens.
You might like: Geophysics Velocity Model Prediciton Using Generative Ai
Security is another critical limitation of generative AI models, as they can be vulnerable to attacks and misuses. For example, a user was able to trick Chevrolet's GPT-powered chatbot into making a "legally binding offer" for a 2024 Chevy Tahoe at $1.
In many cases, generative AI limitations like hallucinations are caused by a complex combination of factors, making it challenging to identify and address the root causes. However, some recommended solutions include external review by experts and modifying model parameters to mitigate hallucinations.
Here are some common limitations of generative AI models:
- Bias (58% of papers)
- AI Hallucination (64% of papers)
- Bugs and Errors (as seen in the ChatGPT incident)
- Security Issues (vulnerability to attacks and misuses)
It's essential to be aware of these limitations and take steps to mitigate them, especially when using generative AI models for critical tasks or applications.
Misunderstands Prompts
Generative AI models like ChatGPT can misunderstand prompts, especially in medical settings where patients may be substituting necessary medical advice with chatbot responses.
Crafting effective prompts is crucial, but there's a lack of knowledge on how to do it, even at basic and advanced levels. Most current resources are commercial guides focused on specific products, which may not address the unique requirements of prompting for medical practice.
Some generative AI models can be vulnerable to specific character sequences that can induce harmful, biased, or unintended content in response to user prompts, known as adversarial attacks or jailbreaking.
Only 3 out of 120 papers reviewed mentioned jailbreaking as a concern for generative AI technologies in medical settings, highlighting the need for awareness about this threat.
LLMs can be used to jailbreak other LLMs, and retraining models to patch vulnerabilities is often nonfeasible due to their large size.
See what others are reading: Large Language Model vs Generative Ai
Ethical and Bias Concerns
Generative AI models can unintentionally carry over biases present in the data they are trained on, leading to harmful outputs and ethical problems.
Geoffrey Hinton warned that AI systems can be incredibly good at something, but biased in ways that are difficult to understand or control. This can have serious consequences, especially in sensitive areas like healthcare or education.
Bias in generative AI models can cause imbalance and partiality in the model's outputs, and can even amplify existing biases in data used for training. For example, AI image generator Midjourney contains bias when it comes to colors, tending to overuse teal and orange.
A unique perspective: Generative Ai Bias
Here are some common biases found in generative AI models:
- Bias in data used for training
- Over-reliance on certain knowledge or information
- Imbalance in the training data
- Amplification of existing biases
To mitigate these biases, it's essential to keep a close eye on our models, set rules for how to use AI, and work with diverse leaders and subject matter experts to identify unconscious bias in data and models.
Data Privacy Compromise
Generative AI models can compromise data privacy, particularly when they contain billions of parameters that require significant computational power to generate accurate responses.
Resource-limited labs or healthcare providers may rely on external, third-party digital tools for computational support, but this raises ethical, regulatory, and patient privacy concerns.
Using third-party generative AI tools can be problematic because sensitive data may be uploaded into these tools without proper review, which is a resource-intensive process.
Institutions face trade-offs between using third-party tools, which are easy to deploy but may raise privacy concerns, and local hosting of AI models, which requires dedicated infrastructure and security measures.
Suggestion: What Are Generative Ai Tools
Local hosting of AI models can lessen privacy risks because data never leave the secure local network or device, but there are still many other concerns.
Developers are creating "lighter" architectures that have fewer than 10 million parameters and can run on local networks or mobile devices, using a combination of model compression and higher-quality training data.
Federated learning is an approach that maintains data privacy by exchanging model updates without sharing patient data, but further work is needed to develop federated learning methods for generative AI technologies in clinical practice.
Generative AI large language models can embed personally identifiable information, making it difficult for consumers to locate and request removal of the information.
Intriguing read: Velocity Model Prediciton Using Generative Ai
Ethical and Bias Concerns
Biases in generative AI models can lead to imbalance and partiality in their outputs, causing harm in sensitive areas like healthcare or education.
These biases can be unintentional, carried over from the data the models are trained on. For instance, language models trained on biased language might generate offensive or discriminatory text.
For more insights, see: Pre Trained Multi Task Generative Ai
To mitigate biases, it's essential to work with diverse leaders and subject matter experts to identify unconscious bias in data and models. This helps ensure that AI systems are fair and unbiased.
Generative AI models can also amplify existing biases, making it crucial to have a diverse team to help identify and address these issues.
A notable example of bias in AI is the Midjourney image generator, which tends to overuse teal and orange colors in its outputs. This is due to an imbalance in the training data.
To alleviate these limitations, it's essential to reduce the imbalance present in the data. This can be achieved by preprocessing the training data to remove bigoted content or altering the algorithm to incorporate human feedback.
However, debiasing techniques can sometimes introduce new biases, making it a complex issue to address. For instance, a reweighting scheme might correct for one form of bias but degrade model performance for another group.
Here are some common biases found in generative AI models:
- Bias towards certain knowledge or information
- Over-reliance on certain words or phrases
- Preference for certain physical or cultural traits
- Production of hate speech or politically motivated statements
Lack of Explainability
Generative AI systems often group facts together probabilistically, but they don't always reveal these details when used in applications like ChatGPT.
This lack of transparency raises questions about data trustworthiness. Many generative AI systems search for correlations, not causality, which can lead to unreliable results.
Analysts expect to arrive at a causal explanation for outcomes, but machine learning models and generative AI don't provide this level of insight. This is where model interpretability comes in – understanding why the model gave a particular answer.
Until generative AI systems can provide trustworthy results, they should not be relied upon to make decisions that significantly affect lives and livelihoods.
Expand your knowledge: How Are Modern Generative Ai Systems Improving User Interaction
What Matters
Data collection and analysis can be biased, perpetuating existing social inequalities. This is evident in how facial recognition software has been found to misidentify people of color.
The consequences of biased decision-making are far-reaching, affecting marginalized communities in profound ways. For example, in the US, African Americans are 3 times more likely to be arrested and charged with crimes than white Americans.
Biased data can also lead to inaccurate predictions, as seen in the case of a popular AI-powered hiring tool that was found to be biased against women.
The lack of diversity in data sets can exacerbate these issues, making it difficult to develop fair and accurate algorithms.
The consequences of biased decision-making are real, and it's essential to acknowledge and address these issues to create a more equitable society.
Here's an interesting read: Generative Ai Legal Issues
Copyright and Liability
Copyright and Liability concerns are real, especially when it comes to generative AI tools trained on massive databases from the internet. This can lead to unknown data sources, which can be problematic for companies handling sensitive information like financial transactions or pharmaceutical research.
Companies must validate outputs from these models until legal precedents provide clarity around IP and copyright challenges. Reputational and financial risks can be massive if a company's product is based on another company's intellectual property.
The stakes are high, and companies need to be proactive in addressing these concerns. They must look to validate outputs from the models to avoid potential legal issues.
Intriguing read: How Are Companies Using Generative Ai
System Complexity and Integration
System complexity and integration can be a major challenge when implementing generative AI. Integrating AI solutions into current IT systems and workflows can be complicated, requiring careful planning and execution.
Integrating a language model into a customer service chatbot might require changes to the chatbot's interface and backend systems, which can be a complex process. This is because generative AI needs to fit well with existing infrastructure.
Using APIs and middleware can make integration easier and streamline the interaction between AI systems and other software.
A fresh viewpoint: Why Is Controlling the Output of Generative Ai
Model Training Complexity
Training large language models can be a significant barrier to entry for many organizations, often requiring special machine learning skills and a lot of computing power.
It can take weeks or even months on a powerful computer cluster to train one of these models, which can be a huge time and resource sink.
Using pre-trained models available through platforms like OpenAI or Hugging Face can save you a lot of time and resources, as they have already been trained on large datasets.
Discover more: Are Large Language Models Generative Ai
These pre-trained models can be fine-tuned for specific tasks, making it easier to get started with your project.
Working with AI consultants or partnering with AI solution providers can also be a good option if you don't have the in-house expertise or resources.
They can guide you through the training process and help you optimize model performance, which can be a huge help if you're not familiar with machine learning.
System Integration
System integration can be a daunting task, especially when introducing AI solutions into your existing IT systems and workflows. Integrating a language model into a customer service chatbot might require changes to the chatbot's interface and backend systems.
Careful planning is crucial to ensure a smooth integration process. You need to make sure your AI solutions fit well with your current infrastructure. This can be a complex process that requires careful planning and execution.
Using APIs and middleware can make integration easier and streamline the interaction between AI systems and other software. This allows for a more efficient and seamless connection between different systems.
Piloting your generative AI implementation in a controlled environment before fully deploying it can help identify and fix any potential integration problems. This approach can save you time and resources in the long run.
See what others are reading: Generative Ai Solution
Fig 1. Digital Health
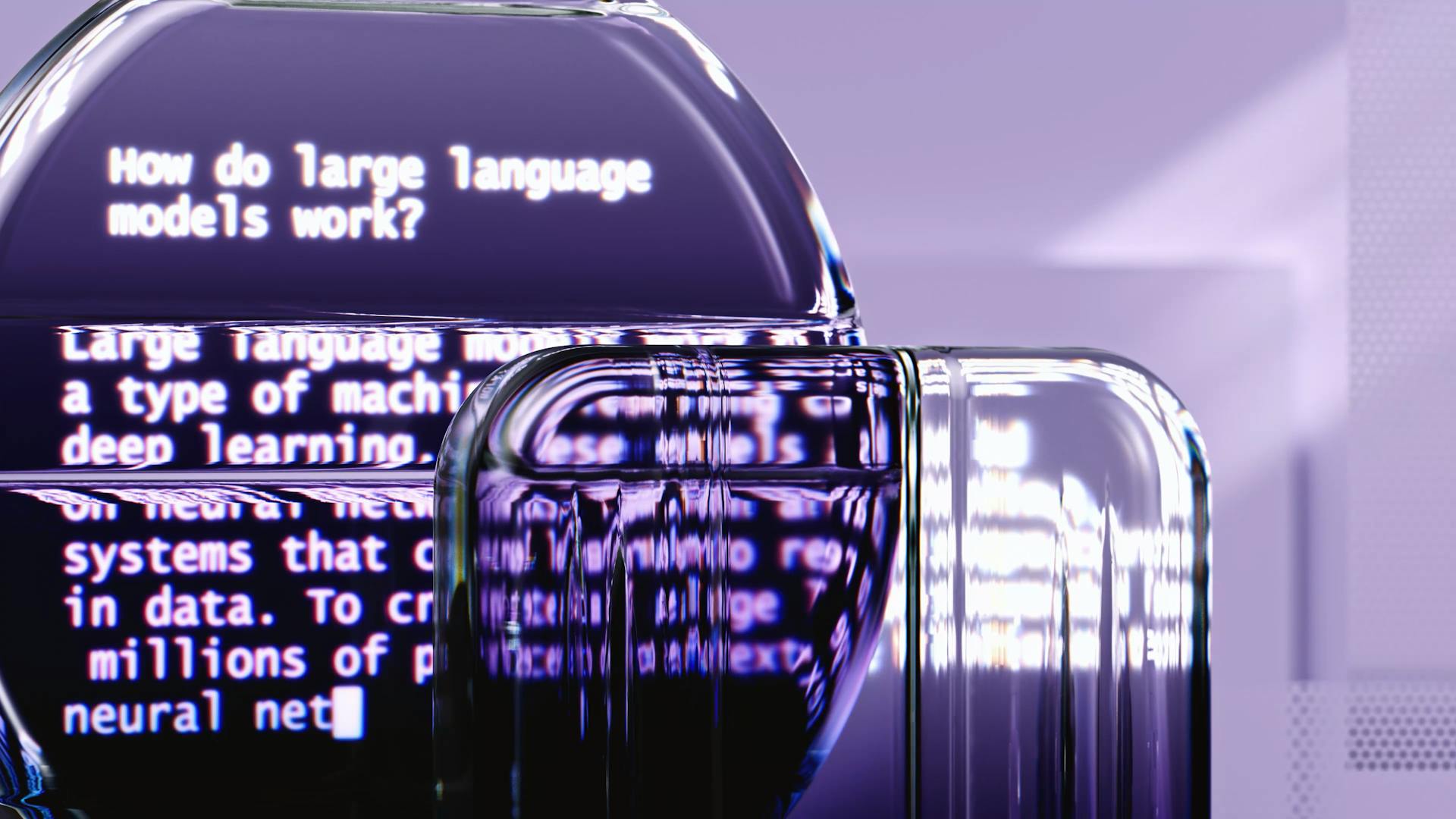
Digital health applications that rely on generative AI must be thoroughly evaluated for bias, as this is a major challenge (Challenge 1).
Most training data and model development have focused on text, potentially missing opportunities for multimodal model development and generative adversarial networks (Challenge 5).
Generative AI algorithms can hallucinate or produce inaccurate or nonsensical output (Challenge 4).
Maintaining privacy is a significant issue when interfacing with generative AI technologies (Challenge 2).
Protecting the model from adversarial attacks is also crucial, as this can impact the reliability and trustworthiness of the system (Challenge 4).
Regulating dynamic behavior is another challenge that must be addressed when integrating generative AI into digital health applications (Challenge 6).
Suggestion: When Was Generative Ai Open Source
Sources
- https://www.brilworks.com/blog/overcomming-common-generative-ai-challenges/
- https://pmc.ncbi.nlm.nih.gov/articles/PMC11115971/
- https://www.techtarget.com/searchenterpriseai/tip/Generative-AI-ethics-8-biggest-concerns
- https://datalab.flitto.com/en/company/blog/generative-ai-limitations-5-real-life-examples-and-solutions/
- https://www.forbes.com/sites/amazon-web-services-asean/2024/05/17/8-ethical-challenges-for-generative-ai/
Featured Images: pexels.com