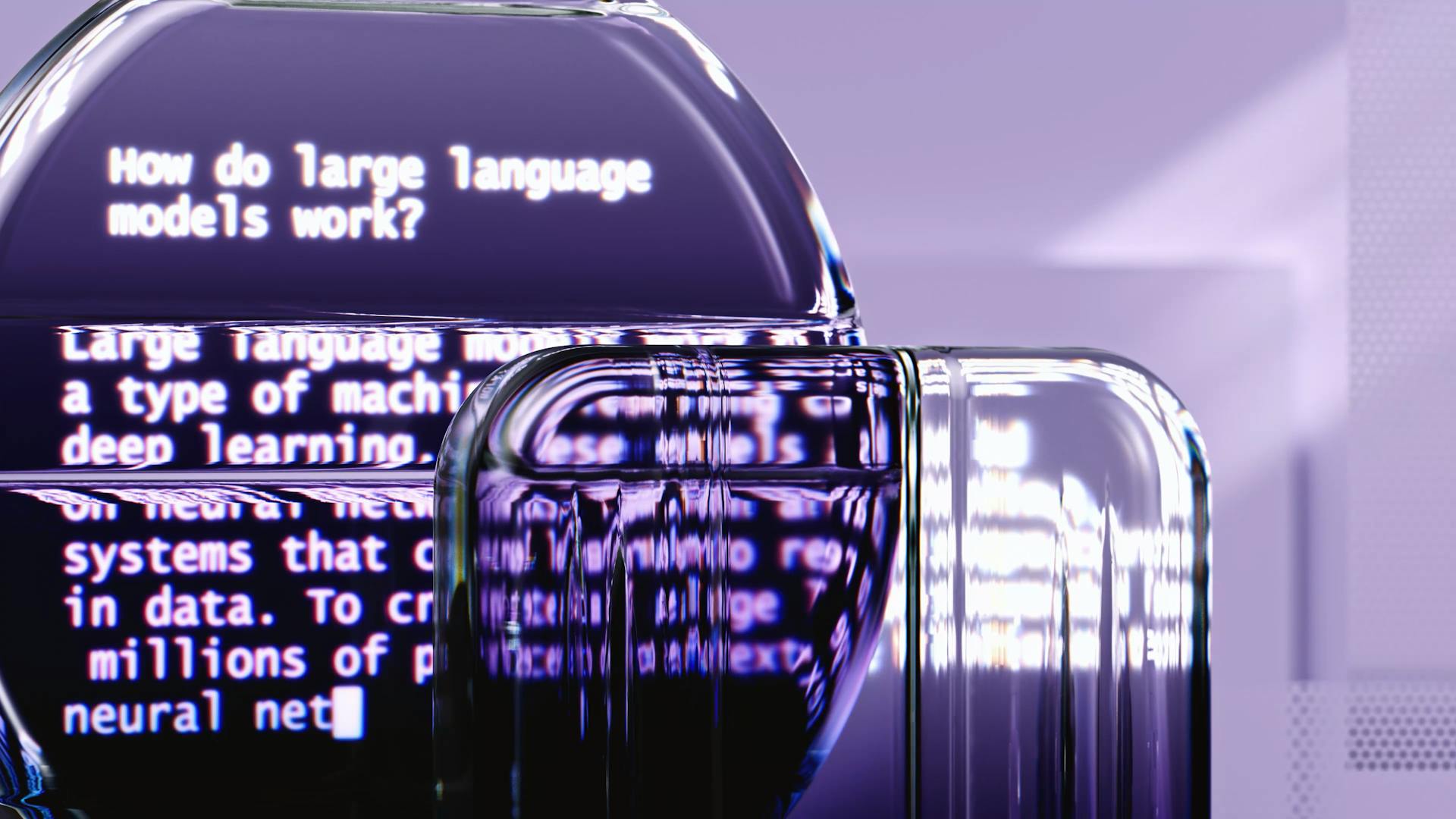
Generative AI is a rapidly evolving field, but it's not without its challenges. One major issue is the lack of transparency in how these models work, making it difficult to understand and trust their outputs.
This lack of transparency is a major concern, as it can lead to biased or misleading results. For instance, a study found that some generative AI models can perpetuate existing social biases, such as racial and gender stereotypes.
Another challenge facing generative AI is the risk of overfitting, where the model becomes too specialized to the training data and fails to generalize to new situations. This can lead to poor performance on unseen data, as was demonstrated in an experiment where a generative AI model struggled to generate coherent text.
The high computational requirements of generative AI models also pose a challenge, requiring significant resources and energy to train and run. This can make it difficult to deploy these models in real-world settings, where resources may be limited.
Explore further: Which Is One Challenge in Ensuring Fairness in Generative Ai
Ethical Challenges
Generative AI is a powerful tool, but it's not without its challenges. One of the biggest hurdles is the potential for biased data, which can lead to discriminatory or unfair outcomes. This is especially concerning in sensitive applications like hiring, lending, and law enforcement.
Bias and fairness are critical issues that need to be addressed. Generative AI systems can perpetuate or even exacerbate biases present in their training data, making it essential to invest in de-biasing techniques and ensure diverse datasets.
Another significant challenge is the creation of deepfakes and misinformation. This can be used maliciously to deceive or harm individuals, making it essential to develop and enforce strict ethical guidelines and policies for the use of generative AI.
The ease with which generative AI can produce realistic text, images, and videos can also be exploited to generate convincing misinformation and fake news. This is a significant concern for companies involved in content generation and social media platforms.
To mitigate these risks, businesses must navigate the fine line between leveraging generative AI for innovation and respecting individual privacy rights. This requires robust privacy-preserving measures and ethical guidelines.
Here are some key ethical challenges in generative AI:
- Bias and fairness: Generative AI systems can perpetuate or exacerbate biases present in their training data.
- Privacy concerns: Generative AI's ability to create realistic synthetic data raises significant privacy concerns.
- Misinformation: The ease with which generative AI can produce realistic text, images, and videos can be exploited to generate convincing misinformation and fake news.
Addressing these challenges requires careful oversight, robust content filtering mechanisms, and the implementation of strict ethical guidelines and policies. By prioritizing transparency, fairness, and accountability, we can ensure that generative AI is used responsibly and for the greater good.
Technical Challenges
Training generative AI models requires vast amounts of data, and the quality of that data directly affects the model's performance.
Data requirements can be a significant challenge, especially if your business deals with a lot of unstructured data, which can require significant resources and expertise to rework into structured data.
Computational costs can also be prohibitive, especially for small to medium-sized enterprises, due to the substantial computational resources required for training and deploying large generative models.
Broaden your view: How to Learn Generative Ai
Overfitting and Lack of Control
Overfitting and Lack of Control can be a major hurdle in implementing Generative AI. This is because generative models often produce outputs that are too closely tied to their training data, making them less effective in new scenarios.
Overfitting occurs when a model is too specific and can't generalize well, which is a challenge that requires ongoing refinement of model training and fine-tuning processes.
To mitigate overfitting, it's crucial to strike a balance between specificity and generality. However, controlling the generation process to align with desired criteria, such as tone or style, is challenging.
Generative models heavily depend on the quality and diversity of their training data, and challenges arise when biases present in the training data are perpetuated in the generated content. This can lead to outputs that reflect and even amplify these biases.
Striking a balance between specificity and generality is crucial, as overly specific outputs may not generalize well to new scenarios. Controlling the generation process requires ongoing refinement of model training and fine-tuning processes to mitigate overfitting and enhance control over the generated content.
Additional reading: What Is a Best Practice When Using Generative Ai
Computational Resources
Training generative AI models demands powerful hardware and substantial time. This can be a significant challenge for smaller organizations with limited resources.
The computational resources required for training and deploying large generative models can create barriers to entry for organizations that lack the financial and technical capacity to leverage generative AI effectively.
Training generative AI models requires vast amounts of data, and powerful hardware to process that data efficiently. This can be a significant challenge for smaller organizations.
The computational costs of training and running sophisticated generative AI models can be prohibitive, especially for small to medium-sized enterprises (SMEs).
Security Concerns
Security Concerns are a major hurdle in integrating generative AI, as these models can be vulnerable to adversarial attacks, potentially leading to the generation of misleading or malicious content.
Over 43% of executive leaders are currently testing generative AI tools, making data privacy a critical concern during this experimental phase. This highlights the need for robust security measures to safeguard generative AI systems.
Adversarial actors can exploit weaknesses in the model to manipulate its output, making it essential to implement techniques to detect and mitigate such attempts. This includes ensuring the deployment environment is secure to prevent unauthorized access and manipulation of the model.
Related reading: Generative Adversarial Networks Ai
Operational Challenges
Generative AI is a powerful technology, but it's not without its operational challenges. One major challenge is the need for vast amounts of high-quality data to train these models. Data quality and relevance are crucial, and businesses often struggle to provide the right data.
The computational resources needed to train and run sophisticated generative AI models can be prohibitive, especially for small to medium-sized enterprises. This can be a significant concern for businesses looking to adopt generative AI.
Integrating generative AI into existing systems and workflows can be complex and require substantial adjustments. This can lead to disruptions in business operations and increased costs.
Maintaining the performance and reliability of AI systems becomes increasingly challenging as businesses scale. This requires significant investment in infrastructure and personnel.
Generative AI models must be protected against theft, corruption, and overexposure to prevent sensitive information from being compromised. This requires robust security measures to be put in place.
Readers also liked: Generative Ai Photoshop Increase Quality
Adoption and Scalability
Scaling generative AI models to handle large volumes of data and complex tasks can be challenging, especially for smaller organizations.
Scalable solutions are key to maintaining competitive advantages, and cloud-based AI platforms offer a cost-effective and scalable option for implementing AI in various settings.
Smaller organizations can utilize cloud-based AI platforms that offer scalable resources and services, making it easier to adopt generative AI.
Curious to learn more? Check out: Google Cloud Skills Boost Generative Ai
Generative AI Adoption Challenges
Generative AI adoption can be a complex process, and it's essential to consider the potential challenges before diving in.
Before jumping all-in on generative AI, you'll need to account for these potential issues.
One significant challenge is the need to address scalability concerns. Cloud-based AI solutions offer a cost-effective and scalable option for implementing AI in call centers, but you'll still need to consider how to handle increased call volume.
Generative AI adoption requires careful planning to overcome the seven key challenges that lie ahead, including understanding what generative AI is and its capabilities.
Generative AI, a form of Artificial Intelligence, possesses the ability to generate novel content across various mediums, spanning text, speech, images, and even code.
Check this out: What Challenges Does Generative Ai Face
Scalability Issues
Scaling generative AI models to handle large volumes of data and complex tasks can be challenging, especially for smaller organizations. This is because generative AI models require a lot of computational power and memory to process and generate high-quality content.
Smaller organizations often struggle to provide the necessary resources, leading to scalability issues. Utilizing cloud-based AI platforms that offer scalable resources and services can help alleviate these challenges.
Cloud-based platforms allow organizations to scale their AI models up or down as needed, making it easier to adapt to changing demands. This is especially important for organizations that need to handle large volumes of data and complex tasks.
Modular AI frameworks are another key solution to scalability issues. These frameworks allow organizations to incrementally scale their AI models as their needs grow, making it easier to maintain a competitive advantage.
Recommended read: Are Large Language Models Generative Ai
Sources
- https://www.forbes.com/sites/amazon-web-services-asean/2024/05/17/8-ethical-challenges-for-generative-ai/
- https://www.joinhgs.com/us/en/insights/hgs-digital-blogs/generative-ai-challenges
- https://blog.cloudticity.com/generative-ai-adoption-challenges
- https://www.itconvergence.com/blog/the-benefits-and-challenges-of-integrating-generative-ai/
- https://www.guvi.in/blog/challenges-and-possibilities-of-generative-ai/
Featured Images: pexels.com