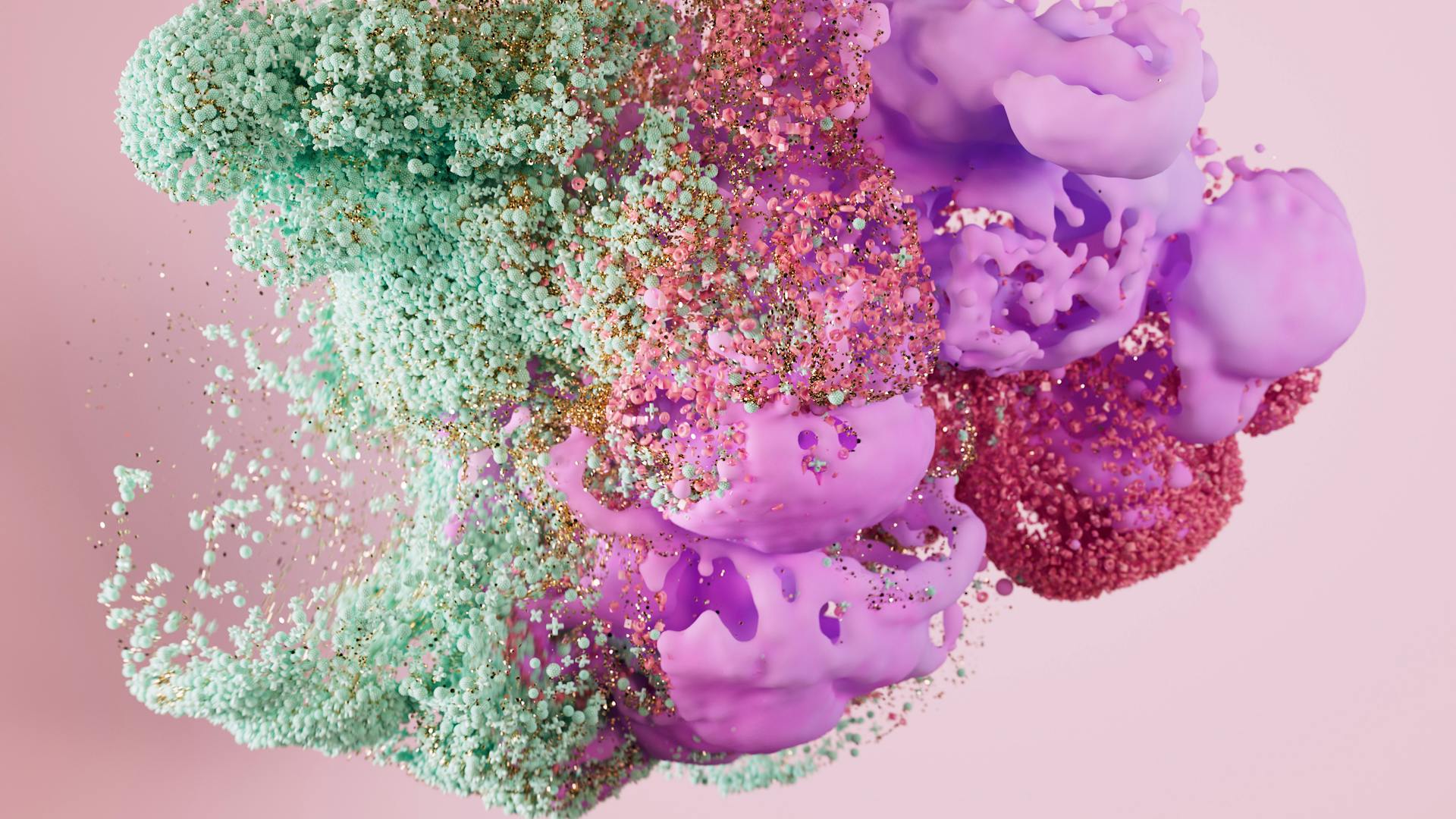
As the use of generative AI continues to grow, so does the risk of fraud. Generative AI models can be used to create fake identities, documents, and even entire personas, making it increasingly difficult to detect fraudulent activity.
According to a recent study, 75% of organizations have experienced a significant increase in AI-generated fraud attempts. This is a stark reminder of the need for robust risk management strategies.
To effectively manage this risk, it's essential to understand the different types of generative AI models used in fraud detection. There are two primary types: adversarial and generative adversarial networks (GANs).
The key to successful risk management lies in detecting anomalies and patterns that may indicate fraudulent activity. By leveraging machine learning algorithms and data analytics, organizations can identify and flag suspicious transactions.
In the next section, we'll dive deeper into the technical aspects of generative AI fraud detection and explore the role of machine learning in identifying and preventing fraudulent activity.
Curious to learn more? Check out: What Are the Two Main Types of Generative Ai Models
Benefits and Applications
Generative AI significantly enhances the accuracy of fraud detection systems, reducing the number of false positives by accurately distinguishing between legitimate and fraudulent transactions.
This capability minimizes disruptions for customers by enhancing customer satisfaction and loyalty.
JPMorgan Chase has integrated generative AI into its fraud detection systems, enabling real-time analysis of transaction data to identify suspicious activities.
Mastercard utilizes generative AI for its Decision Intelligence Pro model, which analyzes billions of transactions to detect potential fraud, improving fraud detection rates by up to 20%.
Generative AI automates routine fraud detection tasks, allowing human investigators to focus on more complex and strategic issues.
Operational efficiency is key, as generative AI reduces operational costs and accelerates the detection and response times, making fraud management more effective and less labor-intensive.
The ability to detect and prevent potential threats in real-time fosters a secure environment for banking operations, strengthening customer trust in the institution’s ability to protect their sensitive information.
Limitations and Challenges
Fraud detection is a complex task, and traditional methods have their limitations. They often struggle to scale with increasing transaction volumes, requiring constant manual updates to adapt to new fraud techniques.
Traditional rule-based systems and machine-learning models can be time-consuming to maintain and may not capture all relevant information for fraud detection. This is because they require manual feature engineering, which can be labor-intensive and may not account for all variables.
Data imbalance is another challenge, as fraud transactions are rare compared to legitimate ones. This can skew a traditional ML model's ability to accurately detect fraud, making it harder to identify potential threats.
Here are 7 drawbacks of existing fraud detection approaches:
- Traditional methods require significant human intervention for model tuning, updates, and verification of flagged transactions.
- Legacy fraud detection models lack adaptability and agility, leading to frequent manual updates or retraining to address evolving fraud techniques.
- They often struggle to detect multi-channel fraud, as current risk decisioning systems might find it challenging to conduct data analysis across different channels.
- Feature engineering overhead can be high, requiring manual updates to adapt to new fraud techniques.
- Data imbalance can skew a traditional ML model's ability to accurately detect fraud.
- Lack of context can limit their effectiveness in identifying more complex or subtle fraud schemes.
- Lack of adaptability can lead to frequent manual updates or retraining to address evolving fraud techniques.
Limitations of Traditional Methods
Traditional fraud detection methods have limitations that can hinder their effectiveness. One major drawback is that they often struggle to scale with increasing transaction volumes, requiring constant manual updates to adapt to new fraud techniques.
Traditional rule-based systems require manual feature engineering, which can be time-consuming and may not capture all relevant information for fraud detection. This process can be frustrating for fraud managers who need to keep up with evolving fraud techniques.
Readers also liked: Anomaly Detection Using Generative Ai
Fraud transactions are rare compared to legitimate transactions, leading to imbalanced datasets that can skew a traditional ML model's ability to accurately detect fraud. This makes it challenging for traditional methods to detect fraud effectively.
Traditional methods may not incorporate a wide range of variables or contextual information, limiting their effectiveness in identifying more complex or subtle fraud schemes. This lack of context can lead to missed opportunities for detecting fraud.
Legacy fraud detection models that rely on static, rule-based algorithms or machine learning models suffer from a lack of adaptability and agility. This makes them less effective in detecting evolving fraud techniques.
Here are the 7 drawbacks of existing fraud detection approaches:
- Limited scalability
- Feature engineering overhead
- Data imbalance
- Lack of context
- Human oversight
- Lack of adaptability
- Difficulty in detecting multi-channel fraud
Challenges and Considerations
Implementing generative AI in fraud detection comes with its own set of challenges. Data privacy and security are major concerns, as sensitive customer data needs to be handled with care.
Banks must ensure that AI systems comply with data protection regulations. This means maintaining high standards of security to prevent unauthorized access.
Additional reading: How Has Generative Ai Affected Security
Generative AI models often operate as "black boxes", making it difficult to explain their decision-making processes. This lack of transparency can lead to issues of bias and fairness in fraud detection.
Banks need to work on improving model explainability to ensure AI-driven decisions are fair and unbiased.
Financial institutions must align their AI initiatives with evolving regulations to ensure compliance.
Techniques and Methods
Generative AI fraud detection is a powerful tool that can significantly enhance the performance of your fraud detection systems. By leveraging the capabilities of generative AI, you can create synthetic data that closely mimics real transaction data.
Data augmentation is a technique used to increase the size and diversity of training datasets, thereby improving the performance of traditional ML models. This is particularly valuable for fraud detection, where synthetic data can be used to augment existing datasets and provide a richer training environment for fraud detection models.
One of the most powerful features of generative AI is its ability to create synthetic data that closely mimics real transaction data. This synthetic data can be used to balance imbalanced datasets, where fraudulent transactions are typically rare compared to legitimate ones.
A different take: Generative Ai in Cyber Security
Generative AI can also generate synthetic examples of fraudulent transactions, which can be used to improve the model's ability to detect fraud. This can be particularly useful in scenarios where traditional machine learning models may struggle to identify patterns.
Synthetic data generated by generative AI can be used to simulate various types of transactions, including those that have not yet occurred but are theoretically possible. This allows the model to be trained on a wider range of scenarios, making it more robust against new and evolving types of fraud.
Here are some of the key benefits of using generative AI for data augmentation:
- Synthetic data can be used to augment existing datasets and provide a richer training environment for fraud detection models.
- Generative AI can generate synthetic examples of fraudulent transactions, balancing the dataset and improving the model's ability to detect fraud.
- Synthetic data can be used to simulate various types of transactions, making the model more robust against new and evolving types of fraud.
By employing generative AI for data augmentation, organizations can significantly enhance the performance of their fraud detection systems. The ability to generate high-quality, synthetic data provides a more robust training environment, leading to ML models that are both more accurate and adaptable to evolving fraud tactics.
The Future of Finance
Generative AI is revolutionizing the way we detect and prevent financial fraud. Its ability to reduce false positives is a significant advantage, minimizing unnecessary disruptions for customers.
In traditional systems, false alarms can be a major headache for banks and their customers. But generative AI models learn to distinguish between genuine and fraudulent activities more accurately, ensuring that only suspicious activities are flagged for further investigation.
The future of finance will see even more advanced generative AI models integrated with other AI technologies to create a comprehensive security framework. This will enable financial institutions to respond more swiftly and effectively to potential threats.
As financial fraud techniques become more sophisticated, the need for adaptive, real-time fraud detection solutions will continue to grow. Generative AI is well-positioned to address these challenges with its ability to learn from new data and detect novel fraud patterns.
Automation of the fraud detection process with generative AI reduces the burden on human investigators, allowing them to focus on more complex tasks that require human judgment. This improves overall operational efficiency and enables banks to detect and respond to fraudulent activities quickly.
For your interest: How Generative Ai Can Augment Human Creativity
Frequently Asked Questions
How will AI detect benefit fraud?
AI can detect benefit fraud by analyzing patterns and connections in complex data, identifying suspicious transactions and networks indicative of fraudulent activity. This helps prevent and uncover organized benefit fraud schemes
Sources
- https://prismetric.medium.com/generative-ai-a-game-changer-for-fraud-detection-in-banking-5434b8f16804
- https://oscilar.com/blog/generative-ai-fraud-detection
- https://www.ey.com/en_ca/industries/financial-services/navigating-the-dual-nature-of-generative-ai
- https://blogs.nvidia.com/blog/bunq-financial-services-generative-ai/
- https://fintechmagazine.com/articles/will-generative-ai-usher-in-a-new-era-for-fraud-detection
Featured Images: pexels.com