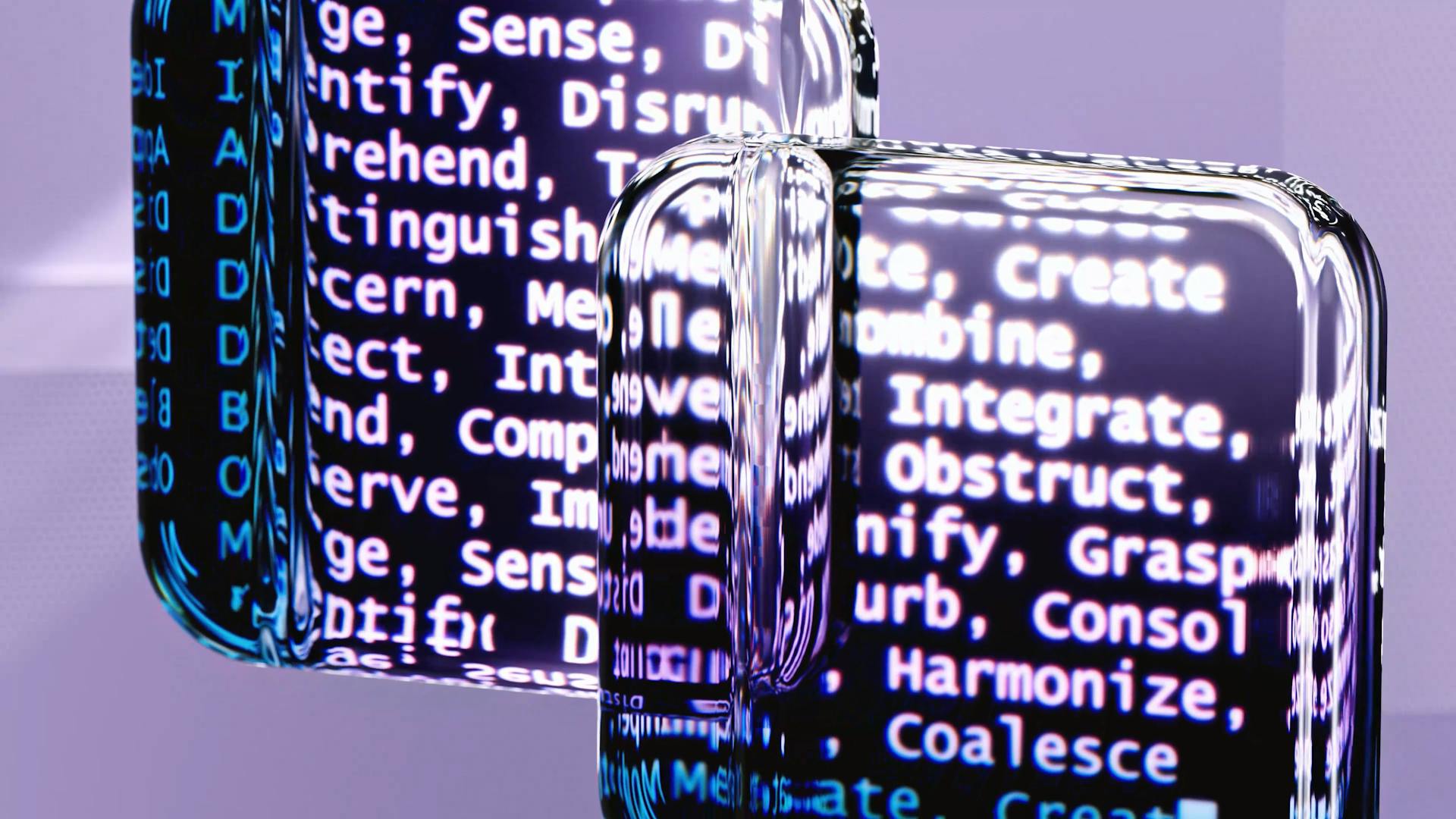
Generative AI in finance is transforming the industry in exciting ways, but it also presents significant challenges. One major issue is the risk of data poisoning, where AI models are trained on flawed or biased data, leading to inaccurate predictions.
Generative AI can create synthetic data that's nearly indistinguishable from real data, which can be a game-changer for financial modeling and forecasting. However, this also raises concerns about data authenticity and the potential for AI-generated data to be used for malicious purposes.
The use of generative AI in finance is still in its early stages, and regulatory frameworks are struggling to keep pace. As a result, there's a lack of clear guidelines and standards for the development and deployment of generative AI in financial applications.
Adoption and Success Stories
Generative AI is transforming the finance industry, and the numbers don't lie. 36% of consumers are interested in using Gen AI for personal finance, with that number exceeding 50% for individuals under 50, according to Marqeta's Consumer Pulse Report.
Client demands are shifting, and executives are making bold bets on Gen AI. 80% of CFOs surveyed in 2022 plan to scale up AI spending in the next two years, with 72% of CEOs citing this technology's funding as their top priority, revealed by Gartner.
The potential for transformation is vast, with Gen AI implementation potentially leading to up to $340 billion in annual cost savings across the finserv industry, as per MIT Technology Review.
Organizations leveraging artificial intelligence report an 18% boost in customer satisfaction, productivity, and market share, with investments offering an average return of $3.50 for every $1 spent.
Top GAI use cases in finance include improved virtual assistants (80%), financial document search (78%), personalized recommendations (76%), and capital market analysis (72%).
Here are some key statistics on the adoption and success of Gen AI in finance:
Real-life examples of Gen AI in finance include Mastercard's use of the technology to detect fraudulent transactions, which doubled the detection rate of compromised cards and reduced false positives by up to 200%. Morgan Stanley also partnered with OpenAI to implement a generative AI platform for synthesizing research data, enhancing advisors' ability to process large volumes of data efficiently.
Driving Factors
Generative AI in finance is driven by several factors that make it an attractive solution for financial institutions. Machine learning algorithms advancements have led to notable progress in the financial industry, enabling the generation of highly accurate predictions.
The growing volume of data in the finance sector is another factor driving the adoption of generative AI. Financial institutions can now harness the power of generative AI to analyze large datasets and generate new insights, predictions, and information.
Automating mundane tasks and slashing operational expenses are also key drivers of generative AI adoption in finance. By automating tasks such as data analysis and fraud detection, financial institutions can enhance their efficiency and lower operational expenses.
Generative AI can also enhance productivity across the board through automation and insights. It can help identify anomalies and patterns invisible to the human eye, bolstering fraud prevention efforts.
Here are some key statistics highlighting the growth of generative AI in finance:
These statistics paint a clear picture of the growing importance of generative AI in finance. As the technology continues to evolve, financial institutions will need to adapt and leverage its capabilities to stay ahead of the competition.
Potential Roadblocks
Integrating generative AI in finance can be a complex task, especially when it comes to legacy technology. This can be a significant roadblock, as institutions must address the shortage of specialized talent to implement and maintain these systems.
Ensuring the reliability and fairness of AI outputs is crucial, as biased data can lead to unfair or skewed decisions. The European Central Bank has warned about the risk of data quality issues in AI systems, which can produce output with more errors.
Navigating regulatory complexities is another challenge, as the financial sector is highly regulated. Generative AI models must comply with strict standards regarding transparency, accountability, and data usage, posing challenges in ensuring compliance with evolving legal frameworks.
Here are some of the key roadblocks to consider:
- Integrating with legacy technology and addressing the shortage of specialized talent.
- Ensuring the reliability and fairness of AI outputs, mitigating bias, and promoting ownership.
- Navigating regulatory complexities governing your solution deployment in critical finance functions.
- Overcoming skepticism and building trust in Gen AI among stakeholders.
- Adapting existing skill sets and developing new competencies for effective technology utilization.
- Avoiding overgeneralization based on limited data and balancing personalization with ethical considerations.
- Enforcing transparency and accountability throughout AI processes.
- Managing the limitations in training algorithms for nuanced financial analysis.
Potential Roadblocks
Integrating generative AI with legacy technology can be a significant challenge. This is because many financial institutions have existing systems that may not be compatible with AI, requiring costly upgrades or workarounds.
Ensuring the reliability and fairness of AI outputs is crucial, as AI models can inherit biases from their training data. This can lead to unfair or skewed decisions, particularly in areas like credit scoring or investment recommendations.
Navigating regulatory complexities is another hurdle, as financial institutions must comply with strict standards regarding transparency, accountability, and data usage. This can be especially challenging in the face of evolving legal frameworks.
Overcoming skepticism and building trust in generative AI among stakeholders is also a significant challenge. This requires financial institutions to be transparent about their use of AI and to provide clear explanations of how it works.
Adapting existing skill sets and developing new competencies for effective technology utilization is essential. This includes bridging the talent gap between AI and finance professionals, which can be a significant obstacle.
Here are some of the key roadblocks to consider:
- Integrating with legacy technology
- Ensuring reliability and fairness of AI outputs
- Navigating regulatory complexities
- Overcoming skepticism and building trust
- Adapting existing skill sets and developing new competencies
Cybersecurity
Cybersecurity is getting a significant boost from generative AI. By analyzing network traffic, transaction data, and user behavior patterns, generative AI can detect anomalies and potential security threats in real time.
Generative AI can predict and simulate various cyber attack scenarios, allowing banks to proactively strengthen their defenses against emerging threats. This proactive approach can help prevent costly data breaches and maintain customer trust.
Generative AI models complex risk scenarios, considering factors like market volatility, geopolitical events, and regulatory changes. It can generate detailed risk reports and forecasts, enabling more informed decision-making at both operational and strategic levels.
Fraud detection is also becoming more effective with generative AI, which creates sophisticated models of normal financial activity and flags suspicious deviations. This helps prevent financial losses due to fraudulent activities.
Applicant-Friendly Denial Explanations
Applicant-Friendly Denial Explanations are crucial for building trust with customers.
AI can play a significant role in generating these explanations, particularly in loan decision-making processes.
Banks and financial institutions use AI to assess customers' creditworthiness, determine credit limits, and set loan pricing based on risk.
However, applicants need clear explanations of AI-based decisions, such as reasons for application denials.
A conditional generative adversarial network (GAN) was used to generate user-friendly denial explanations.
By organizing denial reasons hierarchically from simple to complex, more understandable explanations can be generated for applicants.
Top Use Cases
Generative AI is transforming the finance industry in numerous ways, and understanding its top use cases is essential for anyone looking to stay ahead in this rapidly evolving field. Generative AI is revolutionizing financial services by enhancing customer service, improving fraud detection, providing personalized financial advice, automating report generation, and optimizing trading strategies.
One of the key benefits of generative AI in finance is its ability to automate tasks, freeing up human resources for more strategic initiatives. For instance, AI can automatically generate financial reports, contracts, and other documents, ensuring consistency and accuracy while reducing manual effort and errors.
Generative AI also empowers financial institutions to provide a more personalized and engaging customer experience. AI-powered chatbots can offer 24/7 support, answering customer queries and providing tailored financial advice based on individual needs.
Here are some of the top use cases of generative AI in finance:
- Risk assessment and fraud detection
- Personalized customer experiences
- Automated report generation
- Optimized trading strategies
- Improved financial forecasting and risk management
- Enhanced data insights and analytics
- Customized investment portfolios
- Assisting in financial planning and advisory services
Generative AI can also automate routine tasks in accounting, such as data entry, and streamline complex processes like searching and synthesizing financial documents.
Best Practices for Implementation
Implementing generative AI in finance requires careful planning and adherence to best practices. This ensures compliance with regulations, enhances security, and maximizes the benefits of AI technology.
To start, define a clear, long-term strategy for integrating generative AI that aligns with the company's overall business objectives. This will help you focus on high-impact areas within finance where generative AI can provide the most value.
Focus on domains and use cases such as forecasting or customer interaction. These areas are where generative AI can make a significant impact and provide the most value.
Choose the right AI deployment model based on security, scalability, and data privacy needs. This could be cloud, on-premise, or hybrid, depending on your specific requirements.
Ensure adequate funding for AI initiatives, including investment in technology infrastructure and AI tools. This will help you build a team of skilled professionals with expertise in AI, data science, and finance.
Intriguing read: Impact of Generative Ai on Tax Industry
Assess and manage risks associated with AI, such as data privacy, biases in algorithms, and compliance with regulations. This will help you prepare for organizational change and promote AI literacy.
Here are the 7 aspects to take into account when integrating generative AI into finance:
- Strategy & vision: Define a clear, long-term strategy for integrating generative AI that aligns with the company’s overall business objectives.
- Domains & use cases: Focus on high-impact areas within finance, such as forecasting or customer interaction, where generative AI can provide the most value.
- Deployment model: Choose the right AI deployment model (cloud, on-premise, or hybrid) based on security, scalability, and data privacy needs.
- Funding: Ensure adequate funding for AI initiatives, including investment in technology infrastructure and AI tools.
- Talent: Build a team of skilled professionals with expertise in AI, data science, and finance to implement and manage AI solutions effectively.
- Risk: Assess and manage risks associated with AI, such as data privacy, biases in algorithms, and compliance with regulations.
- Change management: Prepare for organizational change by promoting AI literacy, fostering collaboration, and addressing resistance to AI adoption.
Frequently Asked Questions
What is generative AI in banking?
Generative AI in banking is the use of advanced AI to automate tasks, enhance customer service, and detect fraud, while also providing personalized financial advice and improving overall efficiency and security. This technology is revolutionizing the banking industry with its numerous benefits.
What type of AI is used in finance?
Machine learning (ML) is the primary type of AI used in finance, enabling financial institutions to analyze and make decisions more efficiently. This technology mimics human intelligence to enhance financial management, investment, and risk protection
Is there a finance GPT?
Yes, FinanceGPT Labs offers a finance-focused generative AI framework. This framework helps financial professionals and investors harness the power of AI in their workflows.
What are the risks of GenAI in finance?
GenAI in finance can create or exacerbate financial risks if not properly governed and supervised, including 'hallucination' risk and errors in reasoning. Proper use and oversight are crucial to mitigate these risks and ensure accurate financial outcomes
How is generative AI used in finance?
Generative AI in finance optimizes portfolio and risk management by analyzing data, market trends, and risk factors. This helps increase returns while minimizing the risk of loss.
Featured Images: pexels.com