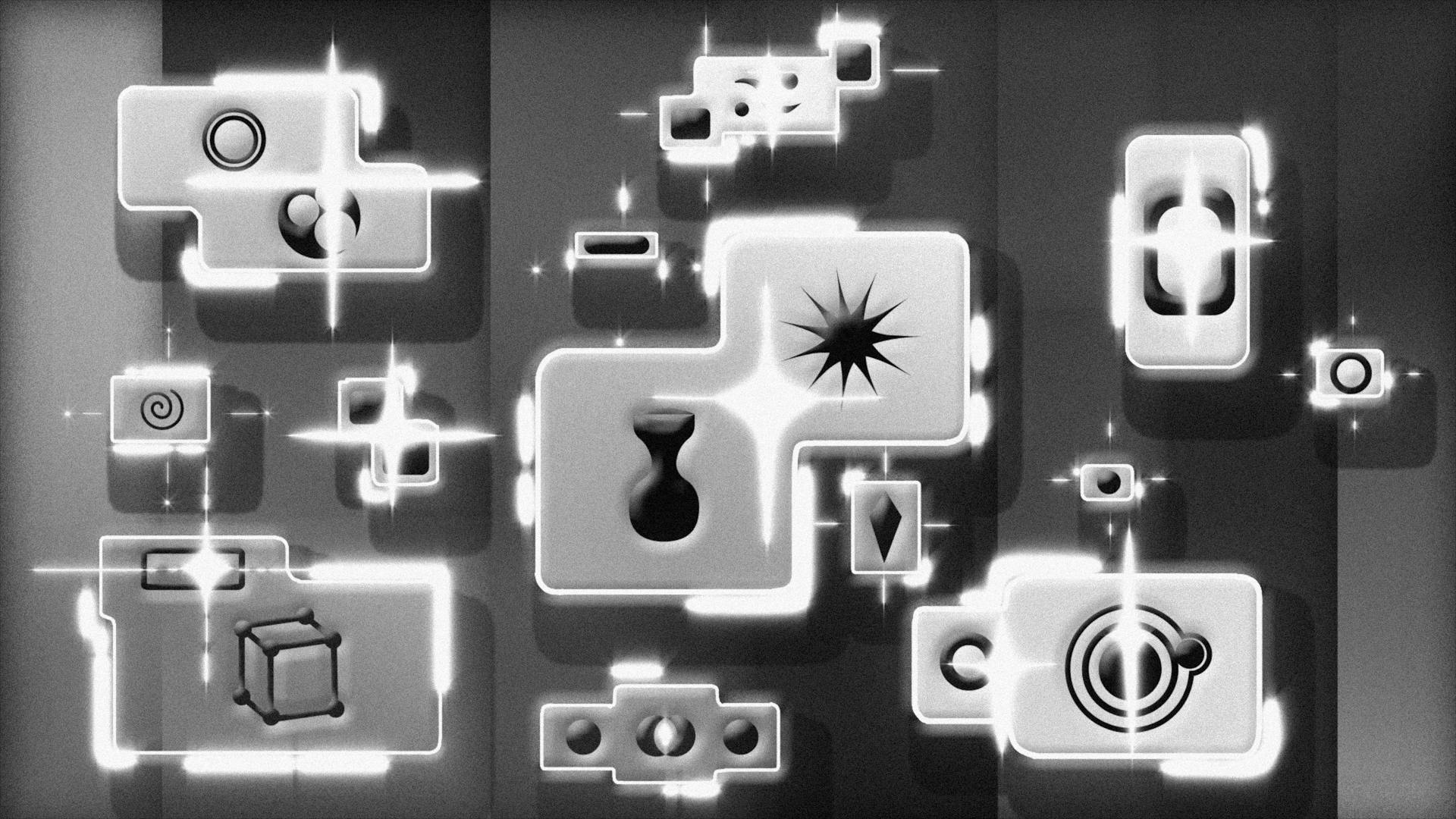
Generative AI has been making waves in the tech world, and its release date is a topic of great interest. The first commercial release of a generative AI model was in 2021 with the launch of DALL-E.
The impact of generative AI on technology has been significant. It has enabled the creation of sophisticated models that can generate realistic images, videos, and even music.
As of now, the most widely used generative AI model is DALL-E, which was created by the AI research lab Meta AI. This model has been trained on a massive dataset of images and can generate new images based on text prompts.
The release of generative AI has also sparked concerns about its potential misuse, such as creating fake news or deepfakes.
A different take: Dall-e Generative Ai
Key Concepts
Generative AI starts with a prompt that could be in the form of a text, an image, a video, a design, musical notes, or any input that the AI system can process.
Generative AI models are trained on vast quantities of existing content to produce new content. They learn to identify underlying patterns in the data set based on a probability distribution.
Early versions of generative AI required submitting data via an API or a complicated process, but now developers are working on better user experiences that let you describe a request in plain language.
Generative AI uses a type of machine learning called deep learning, which allows it to handle more complex patterns than traditional machine learning.
Suggestion: Is Generative Ai Machine Learning
Generative AI vs Other Technologies
Generative AI is often compared to traditional AI, but they're not the same. Generative AI relies on neural network techniques such as transformers, GANs, and VAEs.
One key difference between generative AI and traditional AI is how they approach problem-solving. Traditional AI algorithms follow a predefined set of rules to process data and produce a result, whereas generative AI can autonomously generate many types of new outputs.
Generative AI is particularly valuable in creative fields and for novel problem-solving, whereas traditional AI is more effective for tasks involving rule-based processing and predetermined outcomes. Traditional AI algorithms are often better suited for tasks that require a specific, predictable outcome.
You might like: Generative Ai Has an Intellectual Property Problem
The History of
The first neural networks capable of being trained were invented in 1957 by Frank Rosenblatt, a psychologist at Cornell University.
Modern AI really kicked off in the 1950s with Alan Turing's research on machine thinking and his creation of the eponymous Turing test. This laid the foundation for the development of generative AI.
The Eliza chatbot created by Joseph Weizenbaum in the 1960s was one of the earliest examples of generative AI, but it had limitations due to its rules-based approach and limited vocabulary.
In the 1980s, neural networks gained traction, but it wasn't until the introduction of generative adversarial networks (GANs) in 2014 by Ian Goodfellow and his colleagues that the field of generative AI truly revolutionized.
GANs opened new frontiers in generating images, music, and text by pitting two neural networks against each other to produce increasingly realistic data.
The 2010s saw an explosion in deep learning capabilities, fueled by advances in computing power and the availability of massive data sets, which further expanded generative AI capabilities.
Expand your knowledge: Generative Ai Capabilities
The release of GPT-3 in the 2020s was a watershed moment, showcasing AI's potential to produce coherent, contextually relevant content across various domains.
In 2022, the public release of ChatGPT gave the public access to powerful AI capabilities, reaching an estimated 100 million users within just two months of launch, underscoring the technology's potential to reshape industries and economies.
Applications and Usage
Generative AI has opened up a wide range of creative and lucrative possibilities across different types of media.
One of the most exciting applications is translation, which can break language barriers and facilitate global communication. This technology has the potential to revolutionize the way we interact with people from different cultures.
Generative AI can also be used for creative writing, whether it's academic, business, or fiction. It can even help with code writing, making it easier for developers to create software and apps.
Music lovers can also benefit from generative AI, as it can compose and write songs. Dubbing and dictation are also possible, making it easier to work with audio and video content.
For another approach, see: How Generative Ai Is Changing Creative Work
In addition, generative AI can be used for speech and voice recognition, making it easier to interact with devices and systems. It can also be used for illustration, infographics, 3D modeling, image editing, and architectural rendering.
Here are some examples of how generative AI can be used in different areas:
- Translation
- Creative, academic, and business writing
- Code writing
- Composing and songwriting
- Dubbing
- Dictation and transcription
- Speech and voice recognition
- Illustration
- Infographics
- 3D modeling
- Image editing
- Architectural rendering
Conclusion and Future
The wait is finally over, and generative AI is here to revolutionize the way we live and work. The release date of generative AI has been a topic of much speculation, but we can now confidently say that it's here.
As we've discussed in previous sections, the first public release of a generative AI model is expected to be in 2024, with a major tech company planning to unveil its model in the first quarter of that year. This marks a significant milestone in the development of AI technology.
With the release of generative AI, we can expect to see significant improvements in fields such as art, music, and writing. The possibilities are endless, and it will be exciting to see how this technology is used to create new and innovative content.
Additional reading: Roundhill Generative Ai & Technology Etf
The Bottom Line
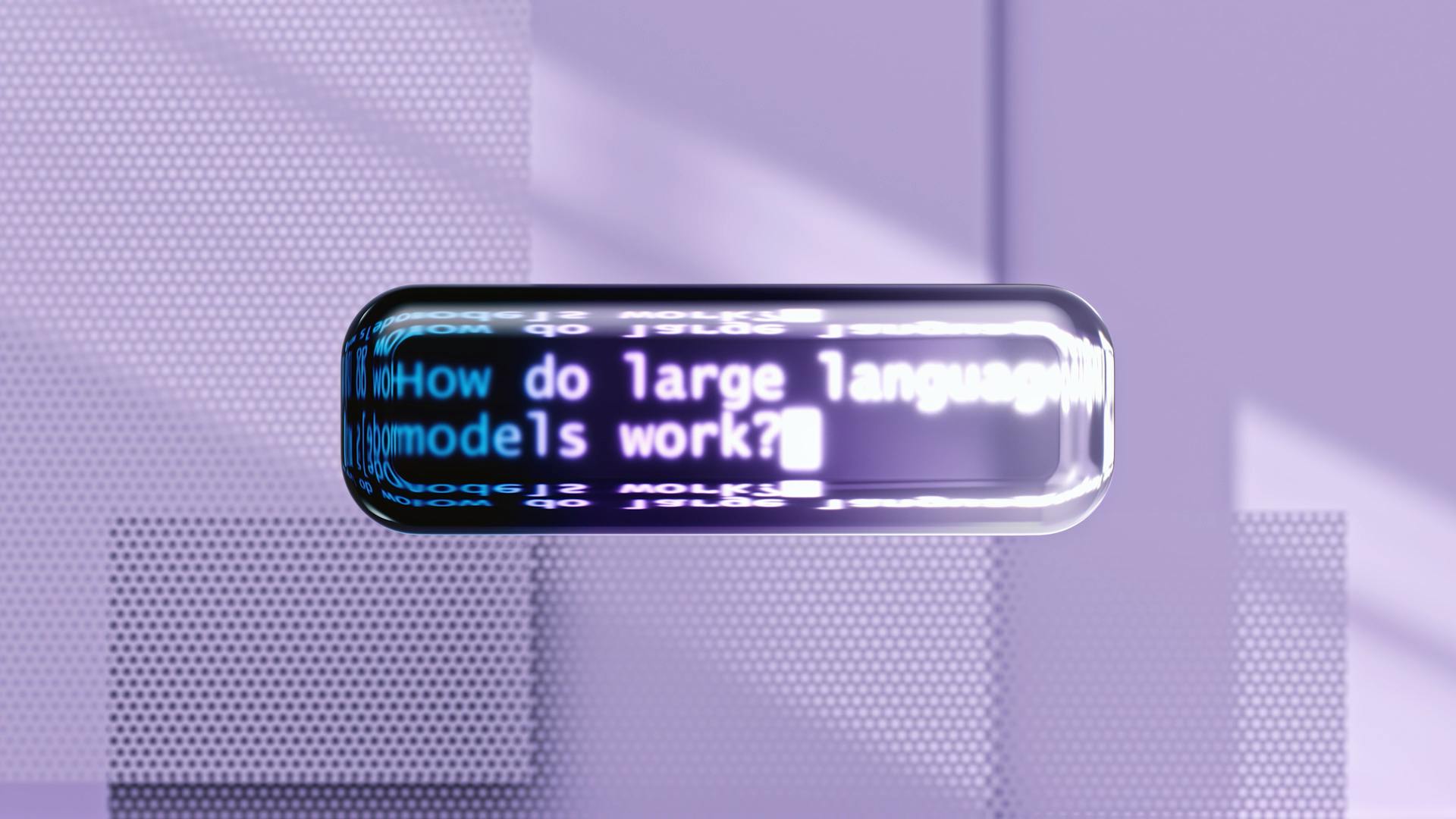
Generative AI is still a relatively new technology with the potential to transform many of the ways we work and live.
Research suggests that the generative AI market is expected to reach $1.3 trillion by 2032, according to Bloomberg.
Its adoption has opened up AI to a much broader user base, with the ability to prompt software in plain language and generate new content in seconds.
However, there are wide-ranging concerns and issues to be cautious of its applications, including legal, ethical, and political implications.
To put this into perspective, a single AI model, ChatGPT, has set a record for the fastest-growing user base, according to Reuters.
This rapid growth has also raised concerns about the environmental impact of generative AI, with some studies suggesting that its carbon footprint could be substantial.
For example, a report by Nature highlights the need to tackle the growing carbon footprint of generative AI.
For another approach, see: New Generative Ai
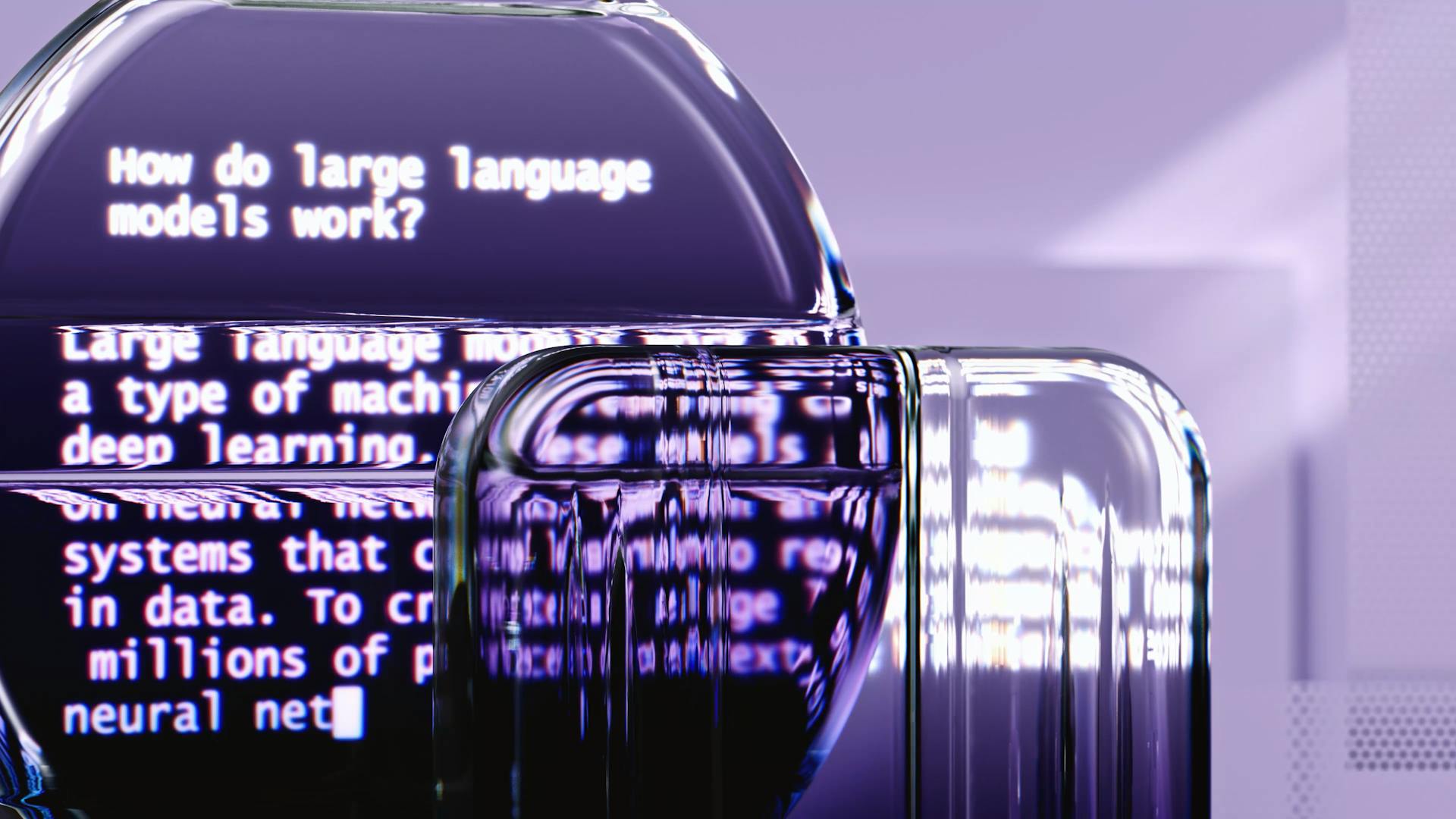
Despite these concerns, many companies are investing heavily in AI, with JPMorgan planning to invest $1 billion or more per year, according to Nikkei Asia.
This level of investment is likely to drive further innovation and adoption of generative AI.
However, it's also essential to address the potential biases and social implications of AI, as seen in the case of Amazon's scrapped AI recruiting tool that showed bias against women, reported by Reuters.
Ultimately, as generative AI continues to evolve, it's crucial to prioritize responsible development and deployment to ensure its benefits are accessible to all.
The Future of Chatbots
The Future of Chatbots is uncertain, and several factors will influence its development.
Government regulation of AI is a possibility, which could affect the innovation of future GPT models.
If regulation occurs, it's unclear who will handle it and how legislation will keep pace with rapid AI developments.
OpenAI's lawsuits against generative AI tools may impact the development of future training models.
Competitors may build ChatGPT alternatives, but government regulation, fear of litigation, and increased API costs could stifle innovation.
For your interest: Generative Ai and the Future of Work in America
Sources
- https://www.techtarget.com/searchenterpriseai/definition/generative-AI
- https://www.investopedia.com/generative-ai-7497939
- https://developer.nvidia.com/blog/nvidia-deep-learning-institute-releases-new-generative-ai-teaching-kit/
- https://www.searchenginejournal.com/history-of-chatgpt-timeline/488370/
- https://www.gsa.gov/about-us/newsroom/news-releases/gsa-releases-generative-ai-acquisition-resource-gu-04292024
Featured Images: pexels.com