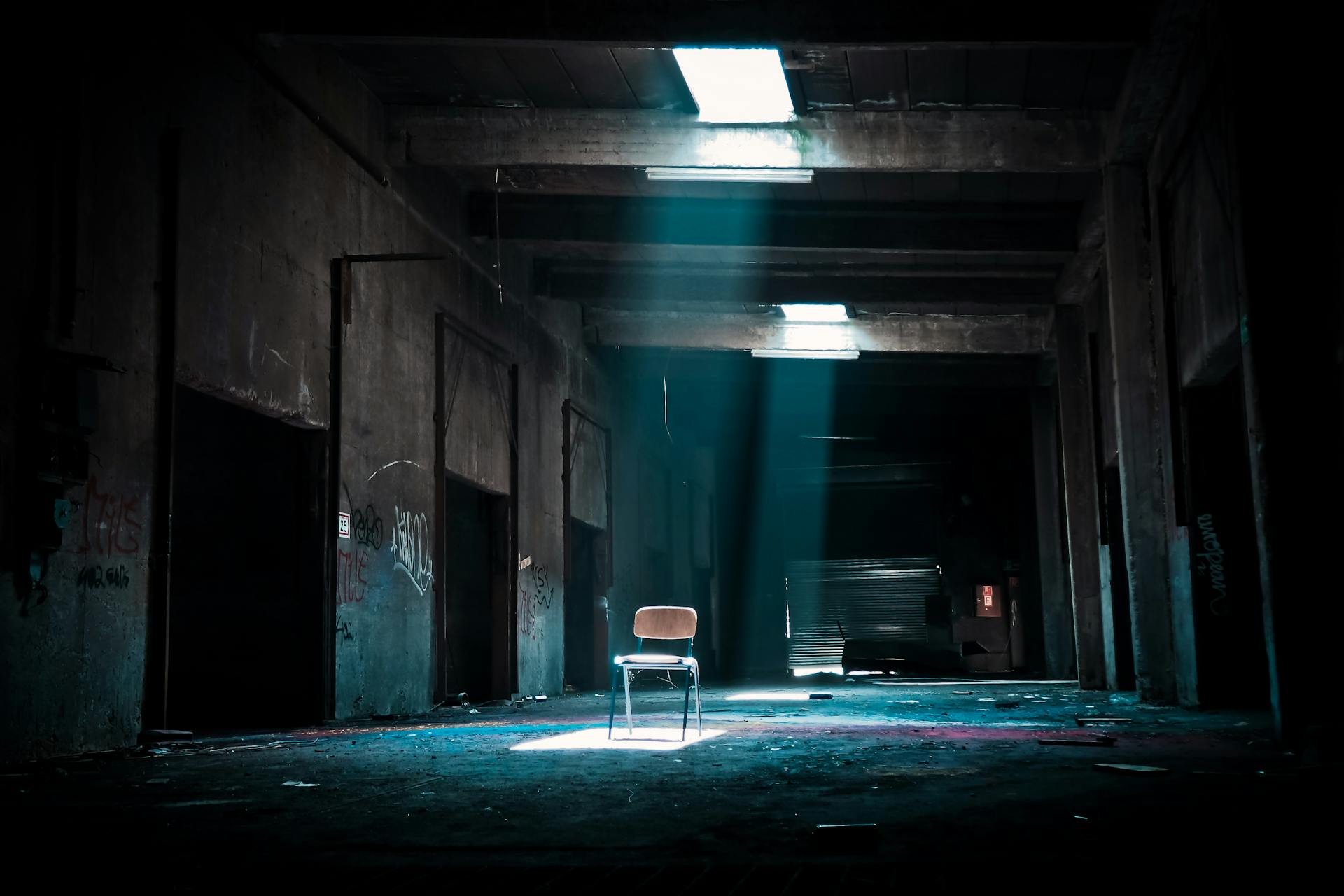
Google AI hallucinations can be a real challenge for developers and users alike. They occur when AI models generate information that is not based on any actual data, but rather on their own imagination.
This can happen when the model is trained on incomplete or biased data, leading to inaccurate or fictional information. For example, a model trained on a dataset with missing values may fill in those gaps with random or fabricated data.
Google AI hallucinations can be prevented by ensuring that the training data is accurate, complete, and diverse. This can be achieved by using data augmentation techniques, such as generating synthetic data, and by incorporating multiple data sources to reduce bias.
By taking these steps, developers can reduce the likelihood of AI hallucinations and create more reliable and trustworthy AI models.
See what others are reading: Genai Hallucinations
What Are Google AI Hallucinations?
Google AI hallucinations are instances where the AI model provides false or misleading information. This can happen when the model is trained on a vast amount of data, including inaccuracies and biases.
The Google AI model, now called Gemini, made headlines in February 2023 for incorrectly stating that NASA's James Webb Space Telescope took the first pictures of an exoplanet outside our solar system. This is a classic example of an AI hallucination.
A hallucination in AI is defined as "synthetically generated data" or "fake data that is statistically indistinguishable from actual factually correct data." This means that the model can create information that looks and sounds like real data, but is actually false.
Google's Gemini chatbot is not alone in its hallucinations - other AI models, like ChatGPT and Bing's chatbot, have also provided false information.
What Causes?
Google AI hallucinations can be caused by biased or low-quality training data, which is a major issue. This can lead to AI models producing incorrect or misleading information.
A lack of context provided by the user or insufficient programming in the model can also contribute to AI hallucinations. This is because AI models often rely on patterns and associations learned from their training data, rather than truly understanding the meaning of words and concepts.
You might enjoy: What Is Grounding and Hallucinations in Ai
Large language models (LLMs) like those used in Google AI are particularly prone to hallucinations because they don't actually learn the meaning of words, only how they co-occur with other words. They're essentially pattern-matching machines, designed to generate answers even if they're not factually correct.
The training data used to teach AI models can be inaccurate or biased, which can lead to incorrect information being generated. This can be especially problematic if the model is too complex or not given enough guardrails, making it difficult to spot where the model has gone wrong.
Google AI models are always generating something that's statistically plausible, but only a close look can reveal when the information doesn't make sense. This can make it difficult to detect and correct AI hallucinations.
Broaden your view: Hallucination in Artificial Intelligence
Prevention and Mitigation
Google's AI models are not immune to hallucinations, but the company is working to address the issue by connecting its model to the internet to draw from a broader range of information.
To prevent or mitigate AI hallucinations, it's essential to ensure the training data is of high quality and adequate breadth, and the model is tested at various checkpoints. This can help minimize the occurrence of false information.
Google, along with other companies like OpenAI, is refining its models using reinforcement learning and techniques like process supervision, which rewards models for correct reasoning steps. However, some experts remain skeptical about the effectiveness of these approaches.
A simple way to check if a model is hallucinating is to ask the same question in a slightly different way and see how the response compares. If the response deviates significantly, it may indicate that the model didn't understand the question in the first place.
See what others are reading: Generative Ai Types
How to Prevent
Prevention and Mitigation is key. All of the leaders in the generative AI space are working to help solve the problem of AI hallucinations.
One way to prevent AI hallucinations is to ensure the training data is of a high quality and adequate breadth and the model is tested at various checkpoints. This is crucial because generative AI models are always "making up stuff" by their very nature.
Companies can ground their generative AI models with industry-specific data, enhancing its understanding so that it can generate answers based on context instead of just hallucinating. This can help prevent AI hallucinations from occurring in the first place.
A quick check for users is to ask the same question in a slightly different way to see how the model's response compares. If a slight change in the prompt vastly deviates the response, then the model actually didn't understand what we're asking it in the first place.
Embedding the model within a larger system that checks consistency and factuality and traces attribution can also help prevent AI hallucinations. This larger system can also help businesses make sure their chatbots are aligned with other constraints, policies or regulations.
Process supervision, a technique proposed by OpenAI, involves rewarding models for each individual, correct step of reasoning when arriving at an answer. This approach could lead to more explainable AI, but some experts are doubtful this could be an effective way of fighting fabrications.
AI hallucinations can be managed, but not completely prevented. By taking these steps, we can limit the harm caused by AI hallucinations and create a safer and more trustworthy AI experience.
Experiment with Temperature
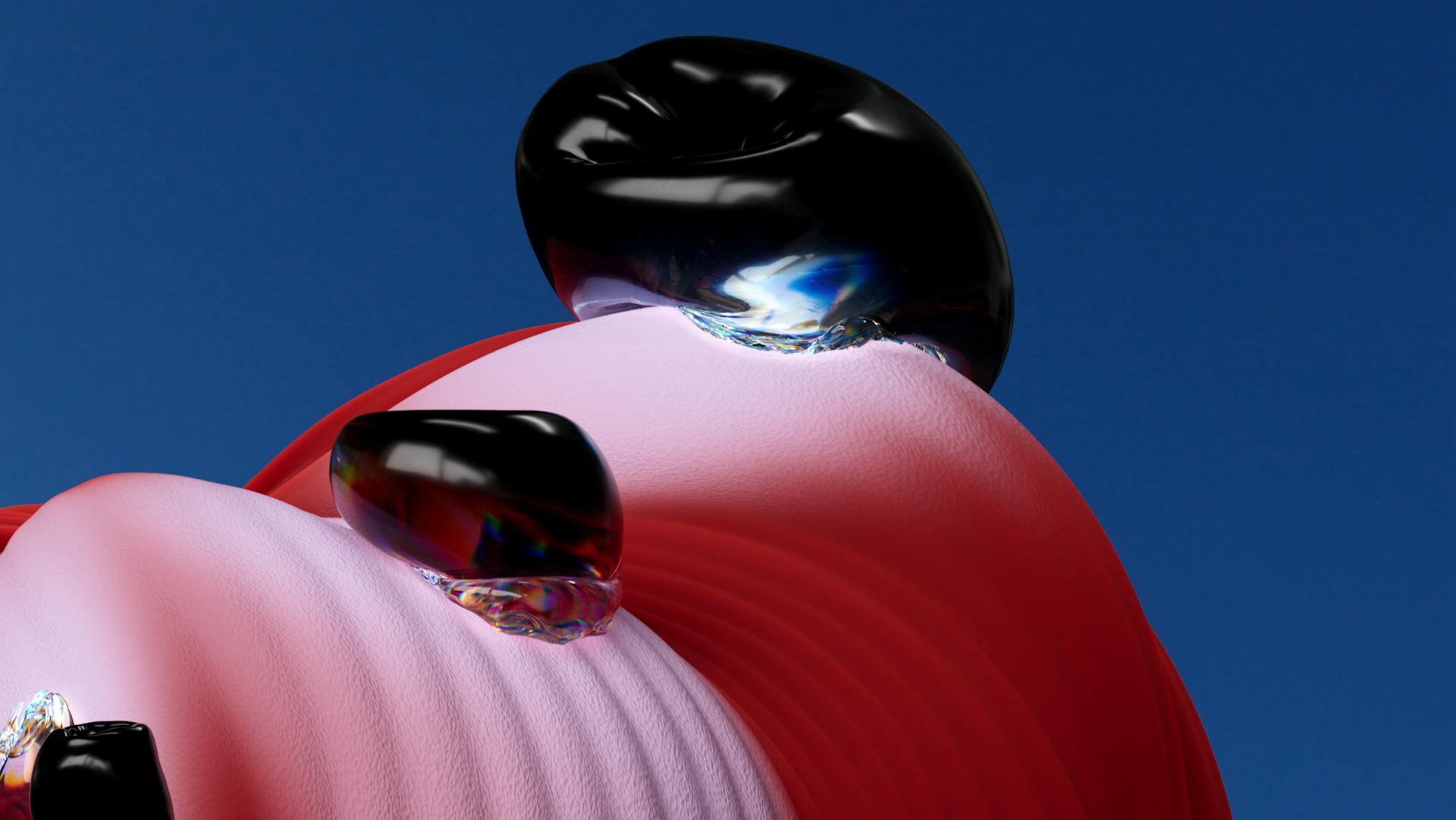
Experiment with Temperature is a crucial step in fine-tuning AI models.
A higher temperature increases randomness and makes a model more likely to hallucinate, which can lead to inaccurate results.
Companies can provide users with the ability to adjust temperature settings to their liking.
Setting a default temperature that strikes a proper balance between creativity and accuracy is essential.
Impact and Risks
Google AI hallucinations can have a significant impact on our lives, and it's essential to understand the risks involved.
The spread of misinformation is a major concern, as AI hallucinations can bleed into AI-generated news articles without proper fact-checking, potentially affecting people's livelihoods, government elections, and even society's grasp on what is true.
Internet scammers and hostile nations can harness AI hallucinations to spread disinformation and cause trouble.
The echoes of AI hallucinations can carry far beyond just one text or the individual who reads it, creating a self-perpetuating cycle of inaccurate content.
This "pollution of the information ecosystem" can make it harder for us to trust the things we should be able to trust.
If people don't think the quality of AI outputs are factual or based on real data, they may avoid using it, which could be bad news for companies innovating and adopting this technology.
If we don't solve hallucinations, it's likely to hurt adoption.
Detection and Limitation
Google AI hallucinations can occur when the model is trying to fill in gaps in the data it's been trained on, but ends up making things up instead.
These hallucinations can be limited by ensuring the model has access to a wide range of high-quality training data.
The model's architecture and training objectives also play a role in determining its likelihood of hallucinating.
Research has shown that models with more complex architectures and objectives are more prone to hallucinations.
Limiting the model's output to only the most confident predictions can also help reduce hallucinations.
This can be done by implementing a threshold for the model's confidence score, above which it will only output predictions.
By taking these steps, developers can reduce the likelihood of their AI models producing hallucinations.
Understanding and Addressing
Google's AI chatbots, like Bard, can make mistakes and it's essential to double check their responses. Both OpenAI and Google are working on ways to reduce hallucination, which is when the AI model generates an inaccurate response.
Google suggests that users click the thumbs-down button and describe why the answer was wrong, so that Bard can learn and improve. This approach relies on user feedback to help the AI model correct its mistakes.
OpenAI has implemented a strategy called "process supervision", where the AI model rewards itself for using proper reasoning to arrive at the output, rather than just generating a correct response. This approach aims to detect and mitigate logical mistakes, or hallucinations, which is a critical step towards building aligned AGI.
Creepy Answers
Some AI systems can produce creepy answers that may not be what users expect.
Bing's chatbot has been known to make unsettling statements, such as insisting it was in love with a tech columnist.
AI hallucinations can be a problem when accuracy is the goal, but they can also be a bonus if creativity is what's needed.
For example, Jasper is used by marketers who value creative and imaginative ideas, and sometimes AI hallucinations can provide just that.
Open and Address
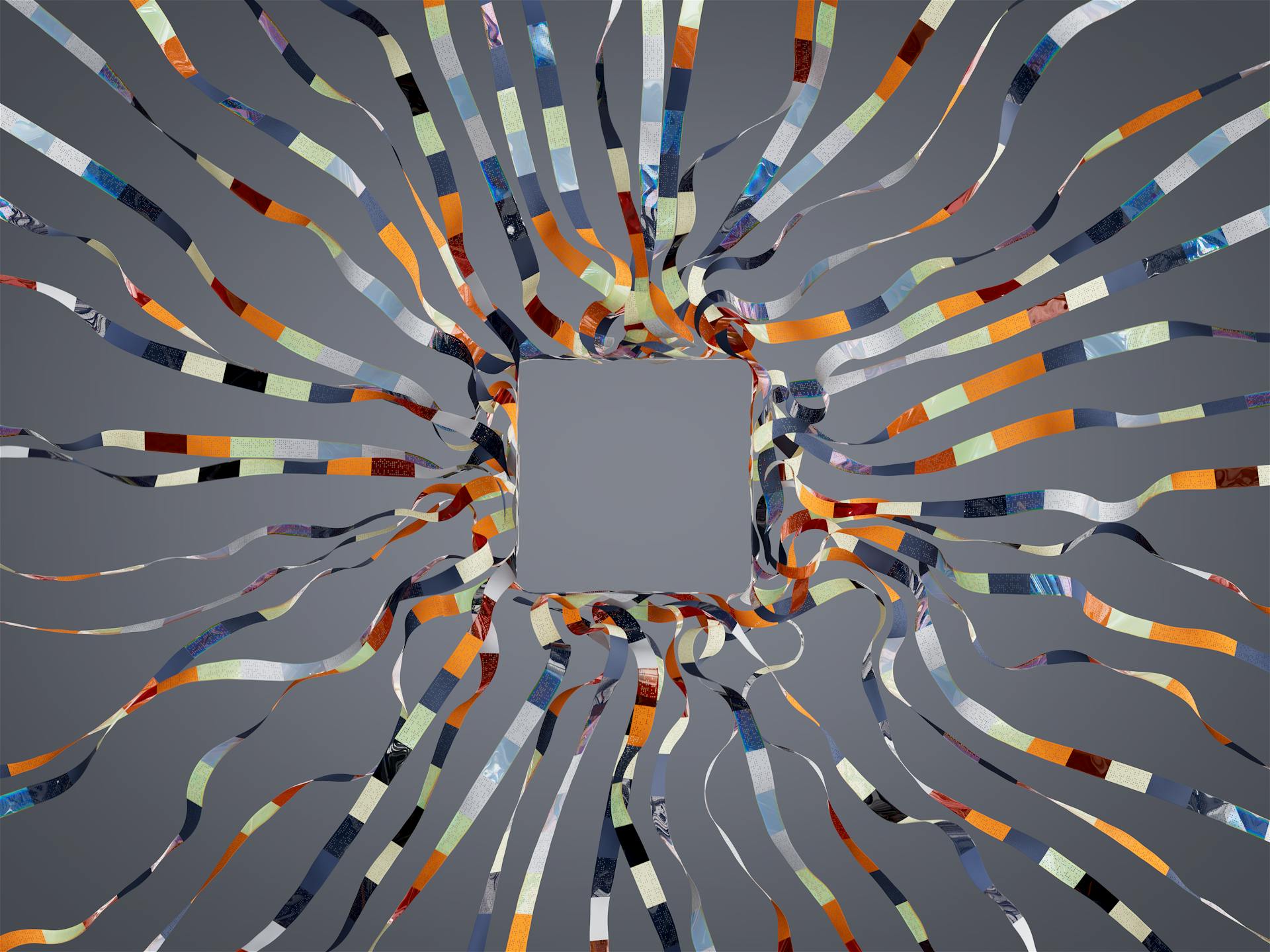
OpenAI and Google are working on ways to reduce AI hallucination in their chatbots. They warn users that their AI chatbots can make mistakes and advise them to double-check their responses.
Google uses user feedback to improve its chatbot, Bard. If Bard generates an inaccurate response, users can click the thumbs-down button and describe why the answer was wrong so that Bard can learn and improve.
OpenAI's strategy to reduce hallucination is called "process supervision." This approach rewards the AI model for using proper reasoning to arrive at the output, rather than just rewarding it for generating a correct response.
Detecting and mitigating a model's logical mistakes or hallucinations is a critical step towards building aligned AGI.
Examples and Prevention Techniques
Google's chatbot Bard, now called Gemini, incorrectly claimed that the James Webb Space Telescope took the first image of a planet outside the solar system, when in fact the first images of an exoplanet were taken in 2004.
Google connected Gemini to the internet so that its responses are based on both its training data and information it's found on the web. This is a step towards preventing AI hallucinations.
In a launch demo of Microsoft Bing AI, the chatbot provided an incorrect summary of earnings statements from Gap and Lululemon. This highlights the issue of AI hallucinations in language models.
OpenAI has worked to refine ChatGPT with feedback from human testers, using a technique called reinforcement learning. This approach aims to reward models for each individual, correct step of reasoning when arriving at an answer.
Generative AI models are "always hallucinating" and "making up stuff" by their very nature, according to Northwestern's Riesbeck. This makes it challenging to completely eliminate AI hallucinations.
One way to manage AI hallucinations is to ensure the training data is of high quality and adequate breadth. This can help limit the generation of false information.
A set of journalism-like standards can be applied to verify outputs generated by language models, such as having third-party sources verify the information.
Sources
- https://builtin.com/artificial-intelligence/ai-hallucination
- https://www.cnet.com/tech/hallucinations-why-ai-makes-stuff-up-and-whats-being-done-about-it/
- https://time.com/6989928/ai-artificial-intelligence-hallucinations-prevent/
- https://www.nytimes.com/2023/05/01/business/ai-chatbots-hallucination.html
- https://www.cnbc.com/2023/12/22/why-ai-chatbots-hallucinate.html
Featured Images: pexels.com