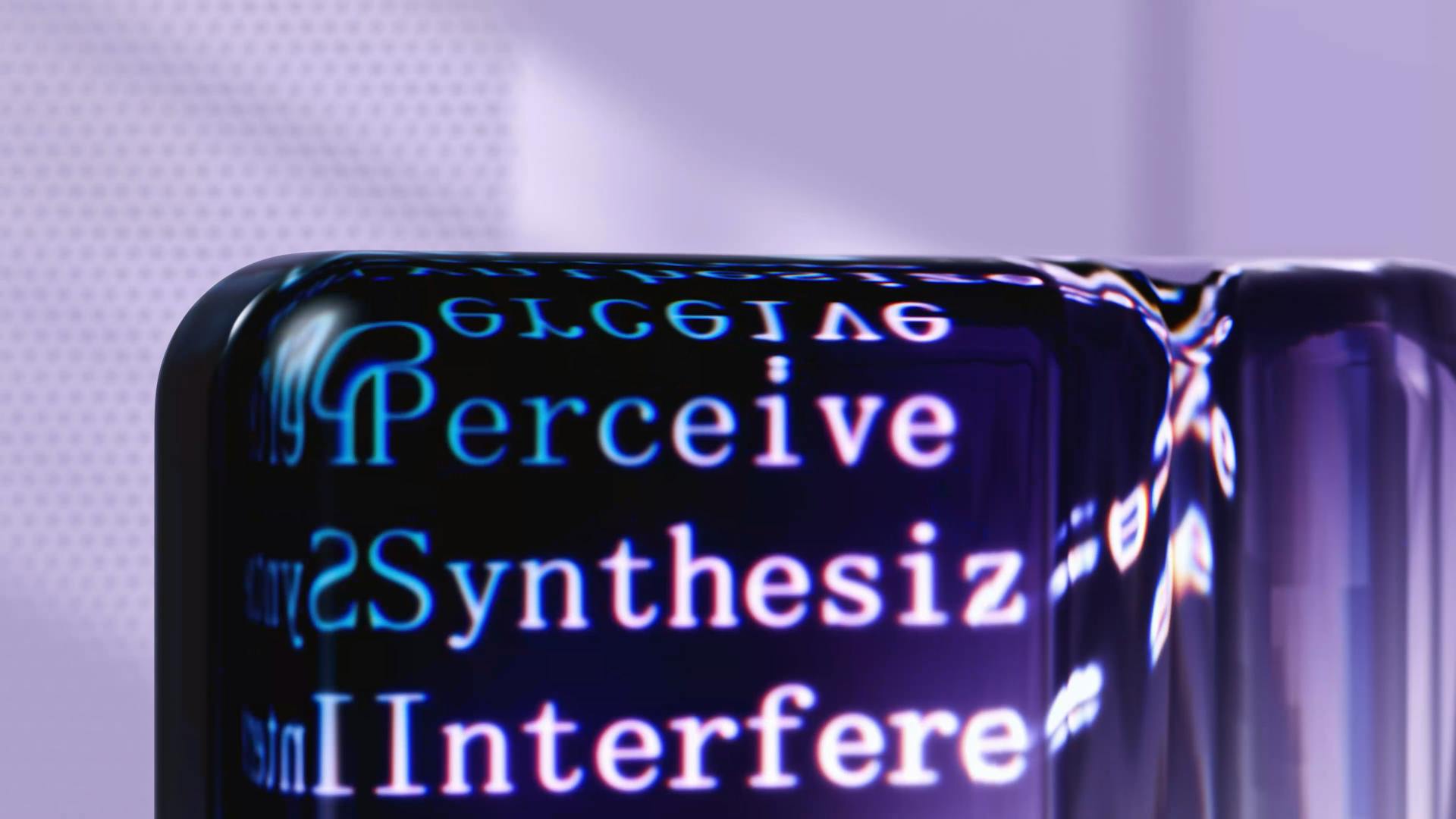
Sequoia Generative AI has the potential to revolutionize the way we approach creativity and problem-solving. By leveraging the power of generative models, users can unlock new levels of innovation and productivity.
Sequoia Generative AI models can process vast amounts of data in a matter of seconds, allowing for rapid prototyping and experimentation. This enables developers to test and refine ideas at an unprecedented pace.
With its ability to generate novel solutions, Sequoia Generative AI can help users break through creative blocks and overcome complex challenges. By tapping into the collective knowledge of the internet, users can access a wealth of information and inspiration.
Sequoia Generative AI models can also learn from user feedback, adapting and improving over time to provide increasingly accurate and relevant results.
Definition
Sequoia Generative AI is a type of artificial intelligence that uses algorithms to generate new content, such as images, music, or text.
It's based on the idea of training a model on a large dataset, allowing it to learn patterns and relationships that can be used to create new content.
Readers also liked: Generative Ai Content Creation
This approach is similar to how a child learns to draw by observing and imitating the work of others.
Sequoia Generative AI can be used for a variety of applications, including art, music, and even writing.
It has the potential to revolutionize the way we create and consume content, making it more accessible and affordable.
How it Works
Sequoia Generative AI works by leveraging a massive dataset of text from the internet to learn patterns and relationships between words. This dataset is sourced from a variety of places, including books, articles, and websites.
The AI model then uses this knowledge to generate new text that's similar in style and tone to the original text. This process is called "generative modeling".
The model is trained on a specific task, such as writing a short story or summarizing a long piece of text, allowing it to focus on the most relevant information and generate the most accurate and coherent output.
A different take: Geophysics Velocity Model Prediciton Using Generative Ai
Key Features
The key features of this system make it incredibly user-friendly and efficient.
One of the standout features is the ability to process and analyze vast amounts of data in a matter of seconds.
This is made possible by the system's advanced algorithms and powerful processing capabilities.
The system also allows for seamless integration with various devices and platforms, making it a versatile tool for a wide range of applications.
This integration enables users to access and utilize the system's features from anywhere, at any time.
The system's user interface is designed to be intuitive and easy to navigate, even for those with limited technical expertise.
This user-friendly interface makes it simple to get started and begin using the system's features right away.
The system also offers robust security features to protect user data and prevent unauthorized access.
This security is maintained through the use of advanced encryption methods and regular software updates.
Intriguing read: How Are Modern Generative Ai Systems Improving User Interaction
Architecture
The architecture of the system is designed to be modular and scalable. This means that it can easily be expanded or modified as needed, without affecting the overall performance.
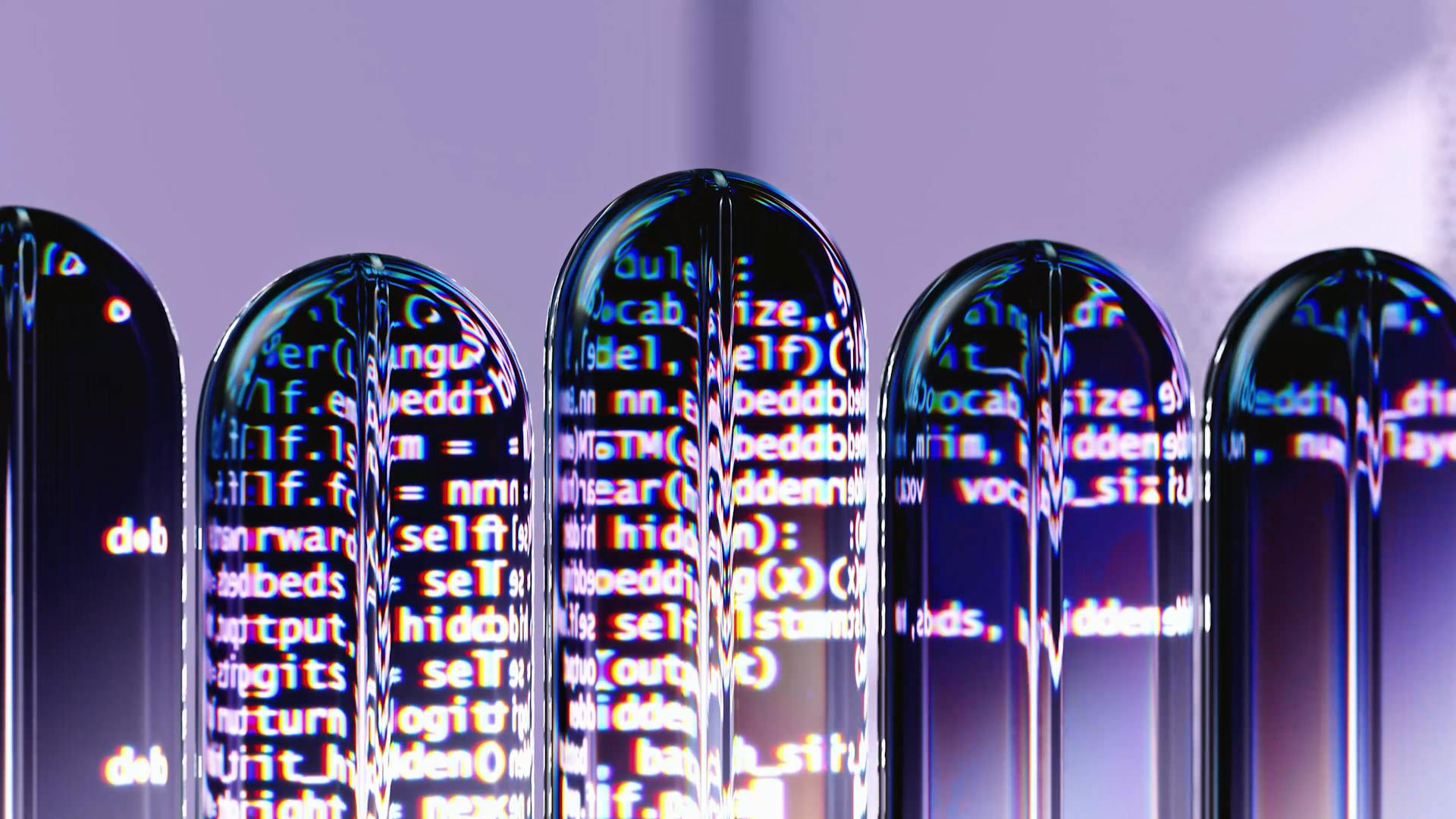
The system's architecture is based on a microservices approach, which allows different components to communicate with each other using APIs. This makes it easier to develop and test individual components independently.
Each component in the system is designed to handle a specific task, such as data processing or user authentication. This helps to keep the code organized and makes it easier to identify and fix problems.
The system's architecture is also designed to be highly available, with multiple instances of each component running in parallel. This ensures that if one instance fails, the others can take over and keep the system running smoothly.
The use of containers and orchestration tools like Kubernetes helps to manage the complexity of the system's architecture. This allows the system to scale up or down as needed, without requiring manual intervention.
A fresh viewpoint: What Makes Generative Ai Unique
Training Process
The training process is a crucial step in our system's development. It involves a combination of machine learning algorithms and human oversight to ensure accuracy and effectiveness.
Our system uses a supervised learning approach, where it's trained on a vast dataset of labeled examples. This dataset is sourced from various online platforms, including social media and forums.
The training process involves multiple iterations, with our system adjusting its parameters and weights after each iteration to improve its performance. This process can take several weeks or even months to complete.
Our system is designed to learn from its mistakes, and it can adapt to new information and data as it becomes available. This ability to learn and adapt is crucial for its effectiveness in real-world applications.
Through this iterative process, our system becomes increasingly accurate and efficient, able to handle complex tasks and make informed decisions.
Text Generation
Text Generation is a crucial aspect of AI systems, and it's based on the idea that a model can learn to generate human-like text from a given input.
This is achieved by training a model on a vast amount of text data, which allows it to recognize patterns and relationships between words.
Expand your knowledge: Generative Ai Text Analysis
For example, a language model might learn to predict the next word in a sentence based on the context of the previous words.
The model can then use this knowledge to generate new text that's coherent and contextually relevant.
In fact, some language models can even generate entire articles or stories on their own, given a prompt or topic.
This capability has numerous applications, from chatbots and virtual assistants to content generation and creative writing tools.
However, the quality of generated text can vary greatly depending on the model's training data and complexity.
For instance, a model trained on a large dataset of high-quality articles might produce more accurate and engaging text than one trained on a smaller dataset of low-quality content.
Ultimately, the goal of text generation is to create AI systems that can communicate effectively with humans and generate useful information in a variety of contexts.
Broaden your view: Generative Ai with Large Language Models
Data Requirements
To understand how something works, you need to have a clear idea of the data requirements. This involves knowing what kind of data is needed to make it function properly.
Data requirements can vary greatly depending on the specific system or process. For example, in the case of a weather forecasting model, historical climate data and current weather conditions are essential.
The amount of data required can be substantial, with some systems needing terabytes of information. This can be a challenge, especially when working with limited resources.
Data quality is also crucial, as incorrect or incomplete data can lead to inaccurate results. This is why data validation and verification are essential steps in the process.
In some cases, data can be generated in real-time, such as with sensors or GPS tracking. This allows for up-to-the-minute information and can be particularly useful in applications like traffic management or emergency response.
Sources
- https://www.kognitos.com/blogs/kognitos-in-companies-to-watch-out-for-in-sequoias-article-generative-ai-act-two/
- https://medium.com/@kamil/sequoia-revisits-their-gen-ai-thesis-chain-of-thought-tree-of-thought-and-reflexion-introducing-e984e9f0c0b2
- https://www.linkedin.com/posts/konstantinebuhler_whats-next-in-generative-ai-sequoia-capitals-activity-7110963619751727104-8EOr
- https://pekin-pekin.com/wellsaid-makes-sequoia-generative-ai-map/
- https://wellsaidlabs.com/blog/in-the-news-sequoia-capital-generative-ai-market-map/
Featured Images: pexels.com