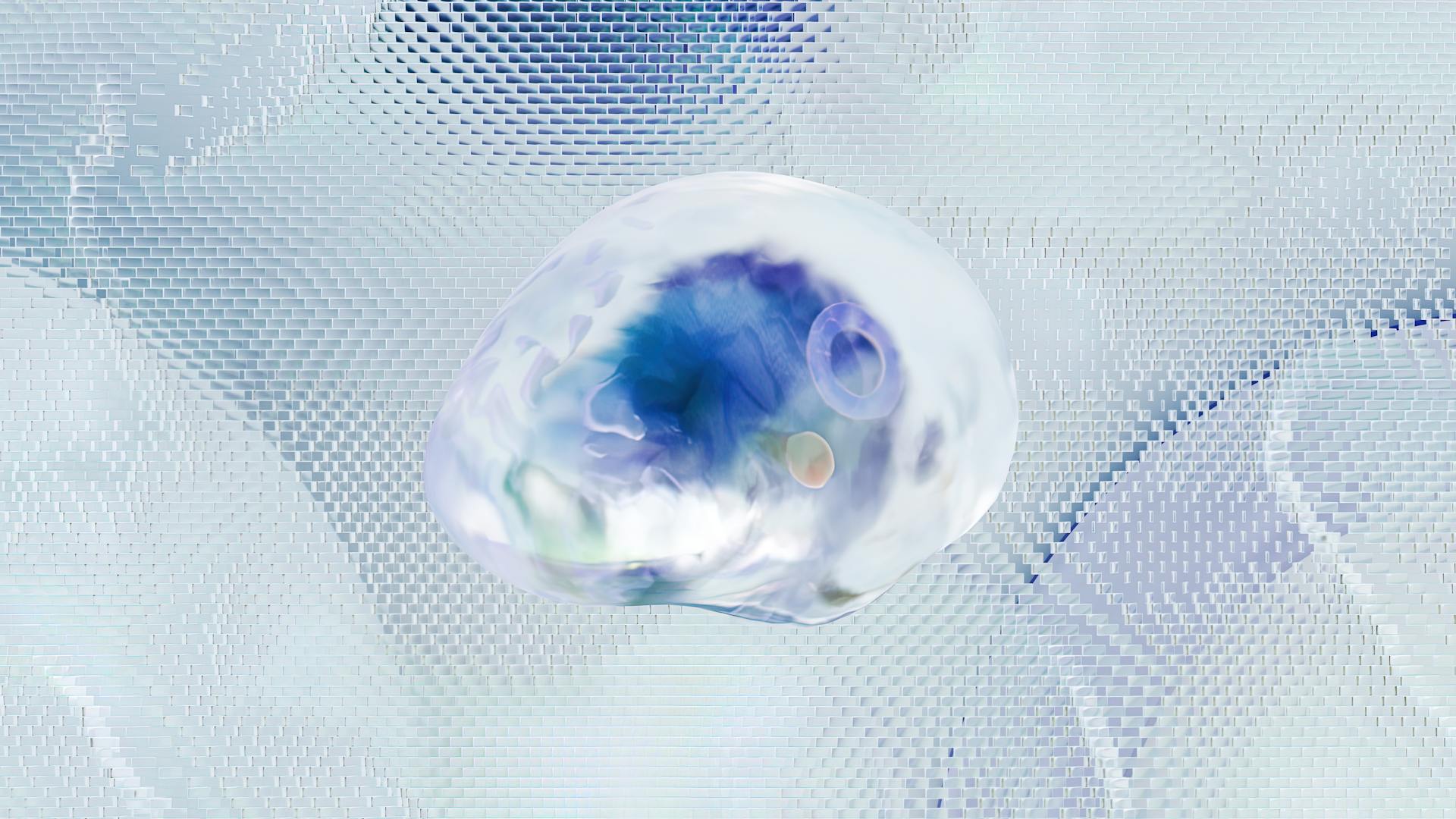
Slack AI training is a game-changer for businesses, allowing them to create AI-powered chatbots that can have natural-sounding conversations with users.
By leveraging the power of machine learning, Slack AI training enables companies to build custom chatbots that can understand and respond to user queries in a more human-like way.
Data is the backbone of any successful AI project, and Slack AI training is no exception. According to the article, Slack's AI training data is sourced from a massive corpus of text, which is used to train the AI models.
This corpus of text is so massive that it contains over 10,000 conversations, providing the AI with a rich source of information to learn from.
Curious to learn more? Check out: Ai Chatbot Training
Data Never Leaves
We take data security very seriously at Slack, and that's why we've made a deliberate choice to ensure that customer data never leaves our controlled VPCs. This means that our customers' data stays safe and secure, and we can guarantee that it won't be retained or trained on by third parties.
For more insights, see: Ai Training Dataset
We couldn't have achieved this without the help of AWS SageMaker, which acts as a trusted broker between the foundational model provider and our customers. This allows us to host and deploy closed-source large language models in an escrow VPC, giving us full control over the lifecycle of our customers' data.
Our decision to use off-the-shelf models, rather than training our own, was also a key factor in keeping customer data safe. We chose Retrieval Augmented Generation (RAG), a stateless approach that ensures the model doesn't retain any data. This not only provides a huge privacy benefit but also makes the results more relevant and accurate for our customers.
The statelessness of RAG means that we can send all the context needed to perform a task within each request, without worrying about the model retaining any sensitive information. This approach also allows us to ground our results in our customers' knowledge base, rather than relying on the public internet.
For your interest: Ai Training Models
Data Control
At Slack, we're committed to building AI products safely, responsibly, and ethically. Trust is our most important value, and we hear from our customers that security is their top requirement as they begin to adopt AI tools.
Slack does not share customer data with LLM providers and does not use customer data to train large language models. This means your data remains private and secure.
We host our LLMs directly within Slack, ensuring that your data remains in-house and adhering to the same security practices and compliance standards that customers expect from Slack itself.
Slack is FedRAMP Moderate authorized, which confers specific compliance requirements, including not sending customer data outside of our trust boundary. This means we're held to a high standard of data protection.
We use AWS SageMaker to host and deploy closed-source large language models (LLMs) in an escrow VPC, allowing us to control the lifecycle of our customers' data and ensure the model provider has no access to Slack's customers' data.
By taking these precautions, we can guarantee that third parties will not have the ability to retain or train on your data.
Expand your knowledge: Slack Ai Training Opt Out
Personalized Search Results
Personalized search results are a game-changer. With AI-powered search, users get clear, concise, personalized answers with direct citations to relevant Slack messages.
You can learn about a new project in no time. Search answers will help you quickly understand the project's goal, the key stakeholders involved, and more. The results are delivered to you based on your company's public communications and your personal conversations.
AI-powered search is like having a friendly colleague who knows everything about your organization. Just ask a question conversationally, and AI will make smart suggestions.
Here are some ways search answers can help:
- Learn about a new marketing campaign or project
- Get up to speed on company policies, such as the deal approval process
- Find internal subject-matter experts to resolve an engineering incident
- Glean insights about past decisions from historical context
- Define unfamiliar acronyms
With AI-powered search, you'll save valuable time and focus on work that really drives an impact.
Bring Your Favorite Tools to Slack
Slack has become a platform for integrating AI tools, with over 13,400 AI custom internal Slack apps built by customers.
You can bring your favorite AI tools into Slack, making it a hub for AI-powered work. Our partner ecosystem is also integrating AI into Slack apps.
Curious to learn more? Check out: Top Generative Ai Tools
PagerDuty's Copilot can be brought into Slack to surface insights and suggest remediation paths, streamlining incident response. Notion's AI summaries can be viewed instantly in Slack when links are shared.
Perplexity's Push integration brings AI-powered insights directly into Slack, allowing teams to stay informed on topics they care about. You can subscribe to topics and automatically pipe updates into Slack.
Here are a few examples of AI tools you can bring into Slack:
- PagerDuty: Copilot for incident response
- Notion: AI summaries for context
- Perplexity: Push for AI-powered insights
Slack AI is available today as a paid add-on for Enterprise plans, and support for additional plans and languages will be coming soon.
Data and Dialogue
Salesforce Channels bring your Salesforce CRM data together with your customer-focused conversations in Slack, keeping work moving forward.
This integration is all about bridging the gap between data and dialogue, making it easier to make informed decisions and respond to customer needs.
By combining data and dialogue, you can create a more seamless and efficient experience for both your team and your customers.
Where Data Meets Dialogue
Salesforce Channels bring your Salesforce CRM data together with your customer-focused conversations in Slack. This integration keeps work moving forward.
By connecting your data and dialogue, you can respond to customer inquiries more efficiently. For example, using Salesforce Channels, you can access customer information right within your Slack conversations.
This seamless integration helps you make informed decisions based on customer interactions.
Behavior
Behavior plays a crucial role in data and dialogue. To build a proof of concept for Slack data integration, one must lean into the Slack API, which is free.
Extracting messages from public Slack channels is a straightforward process. The conversations.history method is used to pull the necessary data. This method allowed the author to pull all the required data from Slack, including user names, dates and times, message contents, and reaction information.
The data extracted from public Slack channels includes the name of the author, date and time of the message, message contents, reactions (emoji type and number of reactions), and number of replies to a message.
Here are the specific data points extracted from public Slack channels:
- Name of the author of the message
- Date and time of message
- Message contents
- Information on the reactions to the message in the form of: emoji type and the number of reactions to said emoji
- Number of replies to a message
This data can be fed into a generative AI model with a simple script, keeping the AI model up to date with the happenings on the Slack channel.
Sources
- https://www.thestack.technology/slack-is-scraping-your-messages-and-files-to-train-ai-by-default/
- https://slack.com/blog/news/slack-ai-has-arrived
- https://slack.engineering/how-we-built-slack-ai-to-be-secure-and-private/
- https://medium.com/slalom-build/feeding-ai-models-with-slack-data-8106550f6485
- https://tibo.ai/blog/slack-faces-backlash-over-sneaky-ai-training-policy-what-it-means-for-your-organization
Featured Images: pexels.com