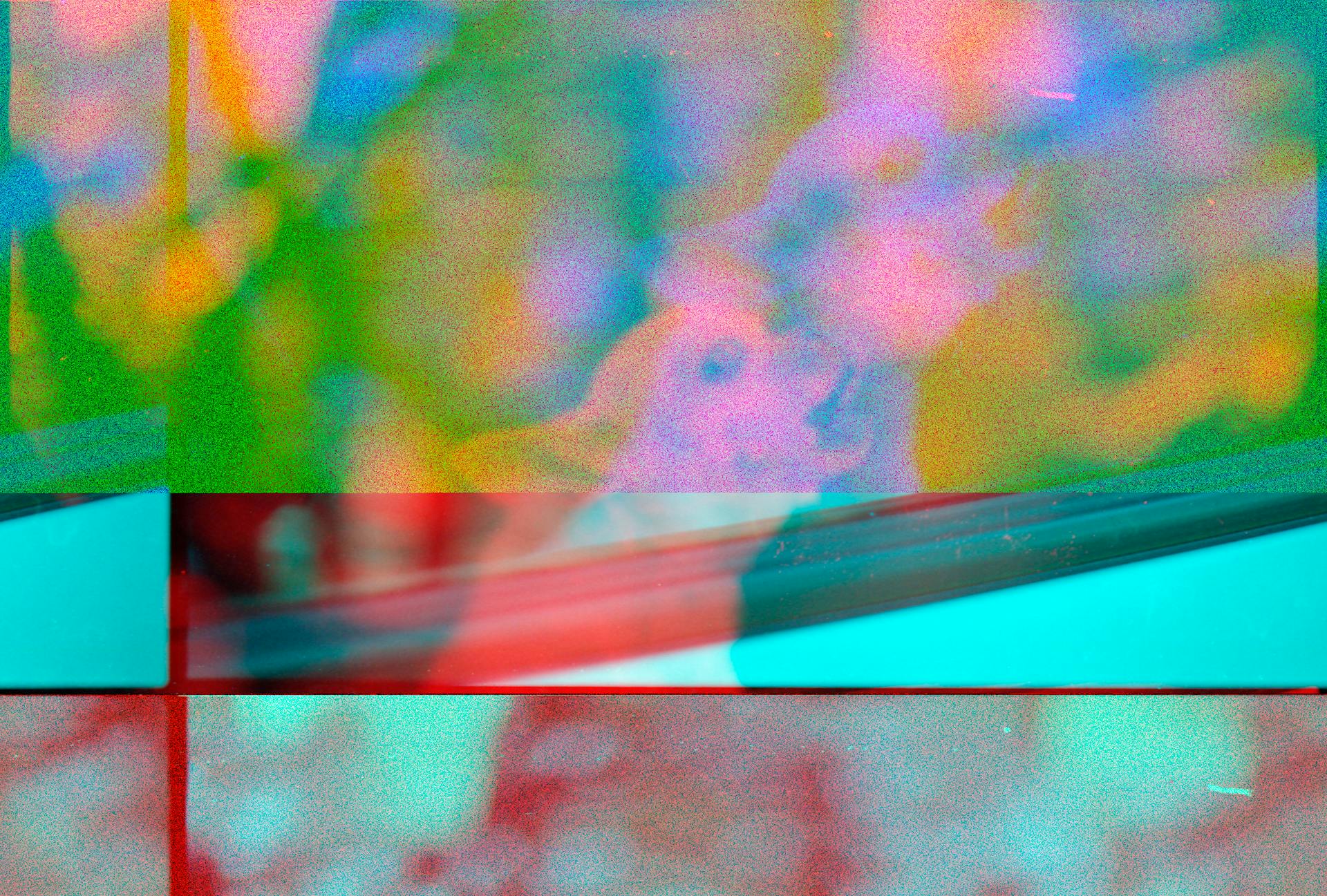
Generative AI interfaces have revolutionized the way we approach research and development, but they're not without their limitations. One example limitation is the lack of human judgment in high-stakes decision-making.
This can lead to biased or inaccurate results, as seen in the limitations of generative AI interfaces in research and development. For instance, a study on AI-generated research papers found that they often perpetuate existing biases and inaccuracies.
The reliance on data can also create a narrow focus, overlooking important contextual factors. This was evident in a project where a generative AI interface was used to generate medical diagnoses, but it failed to account for the patient's medical history.
In such cases, human judgment and expertise are essential for making informed decisions.
A fresh viewpoint: Generative Ai Human Creativity and Art Google Scholar
Generative Model Limitations
Generative AI models face several limitations, including bias, AI hallucination, bugs, and security issues.
These limitations can be caused by a complex combination of factors, making it challenging to identify the root causes.
A fresh viewpoint: Limitations of Generative Ai
Bias in generative AI models can be due to the quality of the training data, which may include high and poor quality data.
Researchers are working on a technique called machine unlearning to address this issue, but it's a highly challenging task.
Fine-tuning generative AI models and having human evaluators rate their outputs can help supplement data insufficiency and provide more accurate responses.
Generative AI models are prone to "hallucinations", generating fictitious information presented as factual or accurate.
Large language models (LLMs) can also produce wrong answers, often presented as correct or authoritative.
The fundamental structure of generative AI models and the release of newer versions can make it difficult to reproduce content consistently.
This is particularly problematic in research and academia, where reproducibility is crucial for establishing credibility.
The nature of generative AI models can result in over-simplified, low-quality, or generic content, especially when given simple prompts.
Many generative AI models are trained on data with cutoff dates, resulting in outdated information or an inability to provide answers about current events.
Here are some common limitations of generative AI models:
- Bias
- AI hallucination
- Bugs
- Security
These limitations can have serious consequences, especially when used in research and academia.
It's essential to always fact-check the information provided by generative AI models, as they can be prone to errors and inaccuracies.
Data and User Concerns
Data and user concerns are a crucial aspect of generative AI interfaces. Data collection and retention policies can be a major issue, as some tools collect user prompts and data for training purposes.
Many generative AI tools allow users to set their own data retention policy, but this doesn't always mean their data is safe. USC researchers, staff, and faculty should be particularly cautious to avoid sharing any student information, proprietary data, or other controlled/regulated information.
Biases in generative AI models can also cause concern, as they can lead to imbalance and partiality in the model's outputs. This can result in the over-reliance on certain knowledge or information, causing the model to overuse certain colors, words, or phrases.
Consider reading: How Are Modern Generative Ai Systems Improving User Interaction
Bias
Bias is a significant concern in AI. Biases in generative AI models can cause imbalance and partiality in their outputs.
These biases often stem from an imbalance in the training data. For instance, AI image generator Midjourney tends to overuse teal and orange, especially in outputs with abstract or broad prompts.
Minor biases can be a characteristic of an AI model, but redundancy can tire out users. Text generation models often overuse a particular set of words or phrases.
Some AI models stick to a certain physical or cultural trait when generating images of a person, which can bring societal issues to light. This can also lead to AI models producing hate speech or politically motivated statements.
Reducing the imbalance present in the data can alleviate these limitations to a certain extent.
Security Issues
Security issues with AI systems can be a concern, especially when it comes to protecting against malicious use. Prompt injection is a technique that exploits AI limitations to induce certain responses, similar to how phishing works on humans.
Large language models, like those used in text generation, process language in broken down bits called tokens and their statistical correlations, but they can't fully detect shifts in user intent. This limitation can be exploited to get sensitive information out of an AI system.
Explore further: Generative Ai with Large Language Models
The term "prompt injection" gained popularity after a Stanford student successfully coerced the Microsoft Bing chatbot to reveal its internal information, including its internal alias "Sydney." This incident showed how vulnerable AI systems can be to manipulation.
Protecting generative AI models from malicious use is a responsibility that falls on the companies that develop them. Humans can control how to build and structure an AI model, but its internal mechanism remains an enigma.
The case of the Microsoft Bing chatbot is a reminder that AI limitations can be used for both good and bad.
Take a look at this: Microsoft Generative Ai Course
Data Privacy Precautions
Extra caution is necessary when working with private, sensitive, or identifiable information. Generative AI services, as well as hosting your own model, require heightened awareness to avoid data breaches.
Many generative AI tools collect user prompts and other user data, presumably for training data purposes. This data collection can be a concern, especially if you're working with sensitive information.
Recommended read: Generative Ai Not Working Photoshop
USC researchers, staff, and faculty should be particularly cautious to avoid sharing any student information, which could be a FERPA violation. This is a serious issue that requires attention to detail.
Some generative AI tools allow users to set their own data retention policy, but it's essential to understand the underlying data collection and retention policies. This will help you make informed decisions about your data.
Sources
- https://midas.umich.edu/research/research-resources/generative-ai-hub/users-guide/
- https://www.icaew.com/technical/technology/artificial-intelligence/generative-ai-guide/risks-and-limitations
- https://datalab.flitto.com/en/company/blog/generative-ai-limitations-5-real-life-examples-and-solutions/
- https://libguides.usc.edu/generative-AI/limitations
- https://durable.substack.com/p/the-limits-of-trial-and-error-interfaces
Featured Images: pexels.com