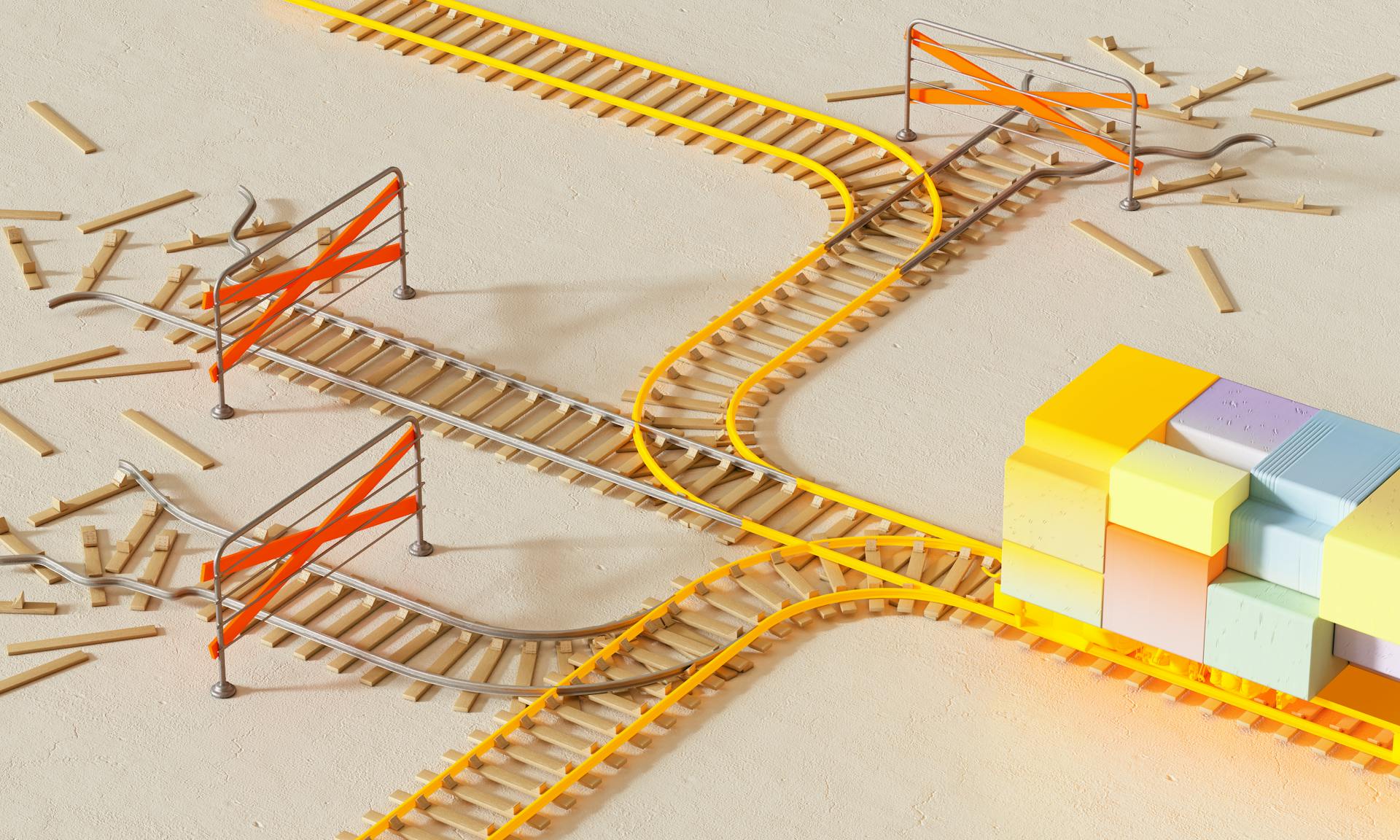
The AI Training Data Center is a crucial component of the AI ecosystem. It's the backbone that fuels the development of artificial intelligence, providing the necessary data to train and refine AI models.
A well-designed data center can handle vast amounts of data, with some centers processing over 10,000 requests per second. This level of performance is essential for training AI models that can learn from vast amounts of data.
The AI Training Data Center is not just about processing power; it's also about data quality and diversity. A good data center should be able to store and manage a wide range of data types, including images, videos, and text. This ensures that AI models are trained on a diverse set of data, making them more accurate and effective.
For your interest: Pre-trained Multi Task Generative Ai Models Are Called
What is an AI Training Data Center?
An AI training data center is a critical infrastructure that houses the necessary equipment to support the high-performance computing needed for AI model training. These data centers are densely connected, network-rich environments that serve as a funnel between endpoints and AI-workloads.
For your interest: Advanced Coders - Ai Training
Low-latency networks and interconnections are essential for AI-capable servers to apply algorithms, make inferences, and guide decisions.
Data centers must be designed to handle large amounts of data, which requires uncorrupted, trusted, and voluminous data.
AI training data centers use a significant amount of power, with some racks handling up to 50kW.
Liquid cooling systems are used to address the power strain, allowing for higher-density deployments without overheating issues.
How AI Training Data Centers Work
AI training data centers are a critical component in the development of AI models. They require densely connected, network-rich data centers that serve as a funnel between endpoints and AI-workloads.
To train AI models, data needs to be uncorrupted, trusted, voluminous, and, depending on the use case, real-time. This is because AI models are being trained with unstructured data collected from all types of endpoints, and all that data will be very useful in the future.
The process of training AI models involves three phases: data preparation, AI training, and AI inference. During the AI training phase, the AI model learns patterns and relationships within the training data to develop virtual synapses to mimic intelligence.
You might like: Ai Training Models
Here are the three phases of developing an AI model:
- Phase 1: Data preparation–Gathering and curating data sets to be fed into the AI model.
- Phase 2: AI training–Teaching an AI model to perform a specific task by exposing it to large amounts of data.
- Phase 3: AI inference–Operating in a real-world environment to make predictions or decisions based on new, unseen data.
AI data center networking plays a crucial role in maximizing GPU utilization and optimizing job completion time. A high-performing network is required to minimize latency and ensure reliability and continued performance.
How Does Work?
AI models are being trained with unstructured data from various endpoints, which will be useful in the future, but for now, they're being refined using enterprise process data and internet scraping to accelerate time to value.
Densely connected, network-rich data centers serve as a funnel between endpoints and AI-workloads, providing low-latency networks and interconnections for AI-capable servers to apply algorithms and make inferences.
Data needs to be uncorrupted, trusted, voluminous, and depending on the use case, real-time to effectively train AI models.
In healthcare research, supercomputers are used to analyze legacy image data to detect disease markers that can't be seen by the human eye, and huge sets of public health data are being analyzed to determine why certain regions are affected by diseases.
Explore further: Time Magazine Ai
High-performance computing (HPC) enables researchers to make correlations at unprecedented rates and scales, teasing out important information from archived data.
Ethernet is an open, proven technology that's best suited to provide a high-performing network in AI data centers, deployed in a data center network architecture enhanced for AI.
This architecture includes congestion management, load balancing, and minimized latency to optimize job completion time (JCT), and simplified management and automation ensure reliability and continued performance.
AI can analyze temperature data in real-time to predict heat patterns, automatically adjusting cooling systems to operate only when necessary, reducing energy use and water consumption.
Dynamic resource allocation improves energy efficiency by improving Power Usage Effectiveness (PUE), a key metric that measures the energy efficiency of a facility, by scaling up resources during peak times and down during low demand.
AI can prioritize renewable energy sources like solar or wind power, seamlessly integrating them into the data center's energy mix, reducing reliance on fossil fuels and promoting a more sustainable operation.
By optimizing energy sources, AI ensures that data centers can make the most of available green energy, lowering their carbon footprint.
AI facilitates better integration with smart grids, allowing data centers to participate in demand response programs, adjusting energy consumption based on grid signals and contributing to overall grid stability.
For your interest: Neural Network vs Generative Ai
What Networking Addresses
To optimize the return on GPU investment, the AI data center network must be 100% reliable and cause no efficiency degradations in the cluster.
The network must be able to handle tens of thousands of GPU servers, with costs exceeding $400,000 per server in 2023.
AI training requires extensive data and compute resources, which can be provided by graphics processing units (GPUs) working in clusters.
Scaling up clusters improves the efficiency of the AI model but also increases cost.
Here are the three phases of developing an AI model, where Phase 2 (AI training) is the most resource-intensive:
- Phase 1: Data preparation–Gathering and curating data sets to be fed into the AI model.
- Phase 2: AI training–Teaching an AI model to perform a specific task by exposing it to large amounts of data.
- Phase 3: AI inference–Operating in a real-world environment to make predictions or decisions based on new, unseen data.
Optimizing job completion time and minimizing or eliminating tail latency are keys to optimizing the return on GPU investment.
Scale and Performance
AI training data centers require a lot of compute resources to support the iterative process of training AI models.
Ethernet has emerged as the open-standard solution of choice to handle the rigors of high-performance computing and AI applications, making it the preferred choice for handling high data throughput and low-latency requirements necessary for mission-critical AI applications.
To give you an idea of the scale, many data centers are using tens of thousands of GPU servers to train large models, with costs exceeding $400,000 per server in 2023.
In these clusters, optimizing job completion time and minimizing or eliminating tail latency are keys to optimizing the return on GPU investment.
Ethernet has evolved over time, including the current progression to 800 GbE and data center bridging (DCB), to become faster, more reliable, and scalable.
Here's a breakdown of the benefits of Ethernet for AI training:
- Faster: Ethernet has evolved to become faster, making it ideal for high-performance computing and AI applications.
- More reliable: Ethernet's current progression to 800 GbE and data center bridging (DCB) has made it more reliable.
- Scalable: Ethernet has become scalable, making it the preferred choice for handling high data throughput and low-latency requirements.
Benefits and Advantages
AI training data centers can bring numerous benefits and advantages. One significant advantage is the ability to analyze temperature data in real time to predict heat patterns, allowing for automatic adjustment of cooling systems and reducing energy use and water consumption.
This smart management of cooling resources can significantly reduce the overall power footprint of the data center, enhancing data center energy efficiency. By optimizing energy sources, AI can also prioritize renewable energy sources such as solar or wind power, seamlessly integrating them into the data center's energy mix.
AI can also dynamically adjust server usage, storage, and network resources based on real-time demand, creating efficient handling of AI workloads and achieving lower PUE values, indicating more efficient use of energy.
For more insights, see: Generative Ai Innovation Center
Automation
Automation is key to an effective AI data center networking solution, and it's not just about automating tasks, but also about providing experience-first operations. This means automation software that's used in design, deployment, and management of the AI data center on an ongoing basis.
Automation automates and validates the AI data center network lifecycle from Day 0 through Day 2+, resulting in repeatable and continuously validated AI data center designs and deployments that remove human error and take advantage of telemetry and flow data.
Juniper's AI data center networking solution leverages three fundamental architectural pillars: massively scalable performance, industry-standard openness, and experience-first operations. This ensures that the solution is optimized for job completion time and GPU efficiency, while also being open and scalable.
A high-capacity, lossless AI data center network design is achieved using an any-to-any non-blocking Clos fabric, which is the most versatile topology to optimize AI training frameworks. This design is complemented by high-performance switches and routers, including Juniper PTX Series Routers and QFX Series Switches.
Check this out: Generative Ai Contact Center
Fabric efficiency is ensured through flow control and collision avoidance, and the solution is open and standards-based, with 800 GbE scale and performance. Extensive automation is provided using Juniper Apstra intent-based networking software, which automates and validates the AI data center network lifecycle from Day 0 through Day 2+.
Here are the three fundamental architectural pillars of Juniper's AI data center networking solution:
- Massively scalable performance–To optimize job completion time and therefore GPU efficiency
- Industry-standard openness–To extend existing data center technologies with industry-driven ecosystems that promote innovation and drive down costs over the long term
- Experience-first operations–To automate and simplify AI data center design, deployment, and operations for back-end, front-end, and storage fabrics
Our Clients Are the Experts
Our clients are the experts in AI development and execution, and we help facilitate their work by providing high-performance computing and low-latency interconnection.
They're not just relying on us for data storage, but for actual AI development and execution.
Media companies have been leveraging our colocation services for years to render imagery in GPU-powered deployments and streamline workflows.
Companies like automotive firms are using our facilities to aggregate data collected by test vehicles and relay it to training models in the public cloud.
This is proof that we can accelerate AI development and play a valuable role in the AI data center ecosystem.
Discover more: Ai Training Development
Challenges and Solutions
The challenges of building an AI training data center are real, and they can be daunting. One of the biggest hurdles is ensuring data quality, as seen in the article's discussion of data curation, which highlights the importance of human oversight in data labeling.
Data storage is another significant challenge, with the article noting that a single AI training dataset can take up to 100TB of storage space. This highlights the need for scalable and efficient storage solutions.
Ensuring data security is also crucial, as the article explains that sensitive data must be properly anonymized to prevent data breaches. This requires robust data encryption and access controls.
To overcome these challenges, implementing a robust data management platform is essential. This can help streamline data curation, storage, and security processes, making it easier to manage large datasets.
Investing in data analytics tools can also help identify areas for improvement and optimize data usage. By leveraging data analytics, data centers can make data-driven decisions and improve overall efficiency.
Explore further: What Is One of the Key Challenges Faced by Genai
Frequently Asked Questions
How is AI being used in data centers?
AI is being used in data centers to optimize energy consumption and grid stability through smart grid integration and demand response programs. This enables data centers to reduce their environmental impact and costs
Where does AI get its training data?
AI training data comes from a variety of sources, including text from the internet, books, and academic papers, as well as audio recordings of human speech
Who is building data centers for AI?
Companies like Google, Amazon, and Microsoft are leading the development of hyperscale data centers for AI. These tech giants are driving the growth of AI-related infrastructure with massive investments.
How much does an AI data center cost?
The estimated cost of an AI data center can exceed $50 billion, including $35 billion for AI server chips. Learn more about the costs and infrastructure behind our AI data center database.
Sources
- https://www.coresite.com/blog/ai-models-ai-providers-and-data-centers-keep-learning
- https://www.juniper.net/us/en/research-topics/what-is-ai-data-center-networking.html
- https://www.bloomenergy.com/blog/ai-data-center/
- https://techhq.com/2024/01/how-the-demands-of-ai-are-impacting-data-centers-and-what-operators-can-do/
- https://blog.datacentersystems.com/the-evolution-of-data-centers-from-traditional-to-ai-powered
Featured Images: pexels.com