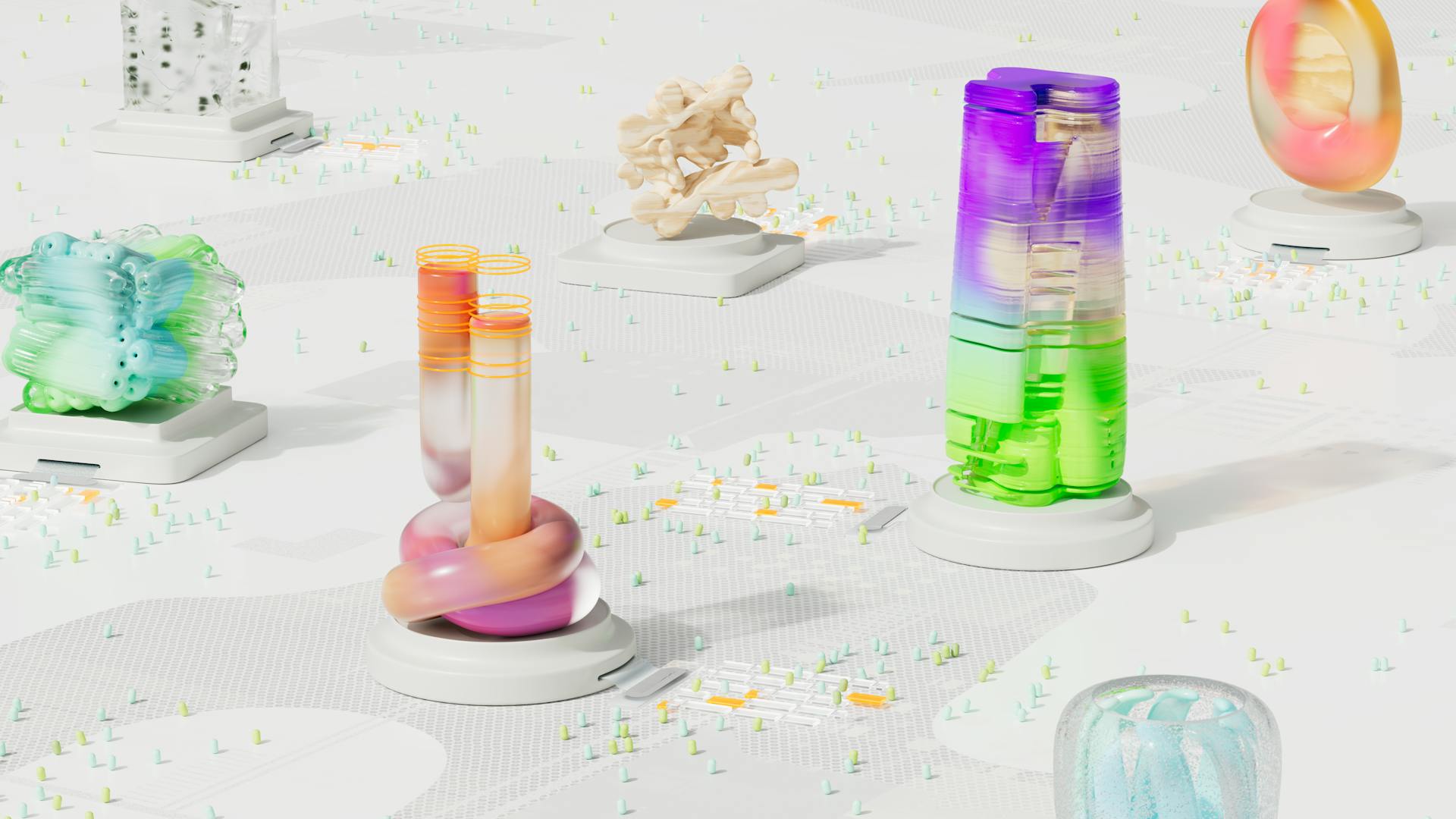
Enterprise generative AI is a powerful tool for businesses, allowing them to automate tasks and create new products and services. It uses algorithms to generate new content, such as text, images, and videos, based on existing data.
These algorithms can learn from large datasets and identify patterns, enabling them to create new content that is often indistinguishable from human-created material. This has the potential to revolutionize industries such as marketing, healthcare, and finance.
One of the key benefits of enterprise generative AI is its ability to process and analyze vast amounts of data quickly and accurately. This allows businesses to make more informed decisions and identify new opportunities for growth.
As a result, many companies are already implementing generative AI in their operations, and the technology is expected to continue to grow in importance in the coming years.
Consider reading: New Generative Ai
What is Enterprise Generative AI?
Enterprise generative AI is a form of artificial intelligence that generates content such as text, audio, videos, and images, to code and simulations.
Generative AI has the potential to create more complex outputs like personalized marketing content, custom applications, and even software.
Business leaders across industries are examining where and how to incorporate generative AI throughout their organizations.
Large enterprises, such as Koch Industries and the U.S. Air Force, have long been digitally transforming with enterprise AI application software.
The emergence of generative AI promises to accelerate such efforts at a rapid pace.
The opportunities are enormous, and the risks of watching from the sidelines just too great.
Generative AI can help organizations boost productivity and creativity across the full customer journey.
It can transform businesses with the power of AI, from content creation to workflow optimization.
If this caught your attention, see: Generative Ai in Software Development
Importance and Benefits
Enterprise generative AI is a game-changer for businesses, and here's why.
It automates repetitive tasks, freeing up employees to focus on what matters most. This is a huge productivity boost, especially in industries where tasks are often mundane and time-consuming.
A recent survey found that 61% of respondents use generative AI primarily for content creation, highlighting its importance in generating personalized messaging that resonates with individual needs.
Generative AI can summarize lengthy documents quickly and easily, allowing you to get right to the heart of the matter. This is a huge time-saver, especially when dealing with complex information.
Here are some key benefits of generative AI:
- Automate repetitive tasks
- Generate personalized content
- Summarize lengthy documents
- Keep employees informed
- Improves enterprise AI
By automating tasks, enhancing creativity, improving efficiency, and generating realistic content, generative AI is revolutionizing industries across the board.
Risks and Limitations
Data privacy and security are major concerns when integrating generative AI in the enterprise. Generative AI models rely on large datasets to function, which means companies must ensure inputs and outputs do not contain sensitive information.
Bias and fairness are also significant risks. Generative AI models are only as good as the data they're trained on, and if they're introduced to biased data, their outputs can be biased too, leading to discrimination and inequity.
Intellectual property issues are another challenge. Generative AI is often trained on copyrighted material, which means the content created could infringe upon intellectual property rights.
To mitigate these risks, companies need to be aware of the potential pitfalls and take steps to address them. Here are some of the biggest risks to consider:
- Data privacy and security
- Bias and fairness
- Intellectual property issues
- Accountability and regulation
Risks Associated with
Generative AI has the most potential across customer operations, marketing, sales, software engineering, and R&D in an enterprise context.
Data privacy and security are major concerns, as generative AI models rely on large datasets to function, which can contain sensitive information such as private customer or company data.
Companies must ensure inputs and outputs do not contain sensitive information, which requires careful data management and protection.
Bias and fairness are also significant risks, as generative AI models are only as good as the data they're trained on, and can perpetuate biases and inequities if introduced to biased data.
Readers also liked: Generative Ai for Customer Support
Intellectual property issues are another risk, as generative AI is often trained on copyrighted material, which can result in AI-generated content that infringes on intellectual property rights.
Accountability and regulation are major challenges, as laws and regulations have not been fully established yet for generative AI in an enterprise setting.
Here are some of the key risks associated with enterprise generative AI:
- Data privacy and security
- Bias and fairness
- Intellectual property issues
- Accountability and regulation
Limitations of Unconventional Solutions
Out-of-the-box solutions don't work because they require extensive fine tuning and customization to align with enterprise-specific needs.
Generative AI and LLM models are powerful entities that need significant adaptations, controls, safety rails, and deep investments of time and resources.
Most LLMs are trained on general-purpose data and lack domain-specific knowledge, making the usability of out-of-the-box LLM-powered solutions not realistic.
There are issues with bias and inaccuracy in these models, which can have serious consequences if not addressed.
A lack of analytics in out-of-the-box solutions makes it difficult to understand their performance and make informed decisions about their use.
Check this out: Generative Ai Solution
Friction in Error Detection
Users are willing to use up to 80% of a model's output, which means the prompt should be considered carefully.
Research suggests that making errors more conspicuous can mitigate users' overconfidence in their ability to spot errors. In a study, users who received nudges in the form of highlighted text missed fewer errors and detected more omissions.
The nudges are a form of what the researchers call "beneficial friction." This concept is essential to understanding how humans react to outputs from machine learning tools.
Experimentation is key to understanding how humans react to outputs from machine learning tools. In the study, users were assigned to one of three groups, with each group receiving different types of nudges.
Here are the takeaways from the study:
- Making errors more conspicuous can mitigate users' overconfidence in their ability to spot errors.
- Given participants' willingness to use up to 80% of a model's output, the prompt (the input into the generative AI tool) should be considered carefully.
- Experimentation is essential to understanding how humans react to outputs from machine learning tools.
Use Cases and Applications
Generative AI can be used to streamline medical image analysis, leading to quicker and more accurate diagnoses. This is especially helpful for radiologists who often face high workloads and potential delays in diagnosis and treatment.
In healthcare, AI can analyze medical images faster and more accurately than humans. This can lead to better patient outcomes and improved quality of life.
Use Cases and Applications
Generative AI is transforming various industries by analyzing vast amounts of data to predict machine failures in manufacturing, reducing downtime and maintenance costs.
In manufacturing, factories can now fix machines before they break, thanks to generative AI's ability to analyze massive quantities of data. This proactive approach extends machine life and lowers maintenance costs.
Medical imaging interpretation can be a time-consuming task, but generative AI can help by streamlining image analysis, leading to quicker and more accurate diagnoses in healthcare.
Retailers can also benefit from generative AI by analyzing customer data to provide a hyper-personalized shopping experience, making them more competitive in the market.
Generative AI can analyze purchase history and customer interactions to provide a tailored experience, making it easier for retailers to connect with their customers.
Explore further: Generative Ai Manufacturing
Voice and Messaging
You can extend LLM-powered and conversational automation experiences to both Voice and Messaging channels.
By integrating with third-party platforms like CRM and customer data platforms, you can provide rich data and allow conversations to power any business action.
LivePerson Conversation Builder helps reduce costs, improve omni-channel customer experiences, and boost agent retention in the contact center.
Build LLM-powered Voice bots and Messaging bots that offer warm, natural, and efficient experiences, which are human-like.
If this caught your attention, see: Generative Ai Customer Experience
Security and Data
Security is a top priority for enterprise Generative AI, encompassing protection from unauthorized access and ensuring data confidentiality, integrity, and availability.
Protecting sensitive data, such as Payment Card Industry data, is crucial, and LivePerson's Generative AI solution masks PCI data before sending it to the Large Language Model (LLM) service.
Implementing robust security measures is essential to prevent data breaches and leaks, and to ensure compliance with data protection regulations.
A robust data platform is also vital for the effective operation of Generative AI models, supporting the storage, processing, and analysis of large volumes of data, including databases, data lakes, and storage systems.
Data masking can be used to protect Personally Identifiable Information (PII), but be aware that it can cause increased latency and potentially less relevant responses from the LLM service.
Recommended read: Generative Ai Security
Security and Privacy
Security and Privacy is a top priority when it comes to Generative AI applications. These applications must be protected from unauthorized access to ensure the confidentiality, integrity, and availability of data.
Data breaches and leaks are a major concern, and measures must be taken to prevent them. Implementing data protection regulations is also crucial to safeguarding data.
LivePerson's Generative AI solution masks PCI data before sending it to the LLM service. This ensures that sensitive payment information remains secure.
PII masking is also available upon request, but be aware that it can cause increased latency and may result in less relevant responses from the LLM service.
Explore further: Develop Generative Ai Solutions with Azure Openai Service
Data Platform
A robust data platform is essential for Generative AI applications to operate effectively. It provides the underlying infrastructure for storing, processing, and analyzing large volumes of data.
Databases, data lakes, and other storage systems are all part of a data platform. These systems enable the storage and organization of data in a way that's easily accessible for analysis and querying.
Data ingestion, transformation, and querying tools and services are also critical components of a data platform. They help to ensure that data is properly formatted and prepared for use in Generative AI models.
Take a look at this: Why Is Controlling the Output of Generative Ai
Frequently Asked Questions
What is the difference between AI and Enterprise AI?
Enterprise AI focuses on large-scale, high-value use cases that drive meaningful business value, whereas AI is a broader term that encompasses various applications. Enterprise AI is a strategic approach to AI that integrates it into business processes for maximum impact.
Sources
- https://www.pega.com/enterprise-generative-ai
- https://learn.microsoft.com/en-us/ai/playbook/technology-guidance/generative-ai/
- https://c3.ai/generative-ai-enterprise/
- https://mitsloan.mit.edu/ideas-made-to-matter/making-generative-ai-work-enterprise-new-mit-sloan-management-review
- https://knowledge.liveperson.com/trustworthy-generative-ai-for-the-enterprise/
Featured Images: pexels.com