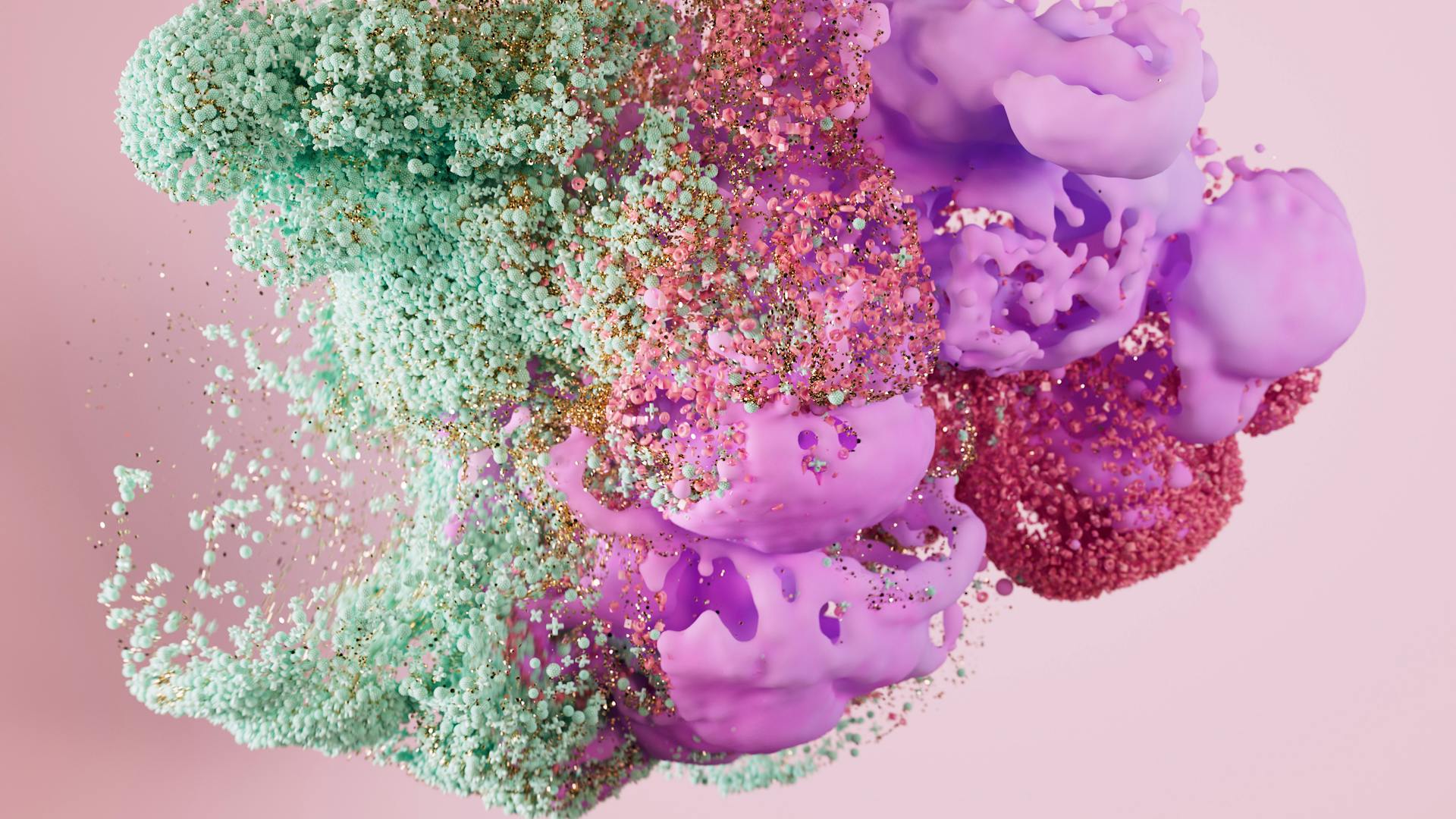
Generative AI is a type of artificial intelligence that can create new content, such as images, music, and text, based on patterns and structures it's learned from existing data.
This technology has the potential to revolutionize various industries, from art and design to music and advertising. Generative AI can produce unique and often surprising results, making it a valuable tool for creatives and businesses alike.
At its core, generative AI is based on machine learning algorithms that allow it to learn from large datasets and generate new content that's similar in style and structure. For example, a generative AI model can learn from a dataset of images of cats and then generate new images of cats that are not in the original dataset.
The possibilities of generative AI are vast, and it's already being used in various applications, from generating realistic-looking faces for video games to creating new music and art.
For your interest: Generative Ai Music Free
What is Generative AI?
Generative AI is a type of artificial intelligence that enables systems to learn from data and generate new content based on that data. This is made possible through Machine Learning (ML) techniques that process and learn from vast datasets.
Machine Learning is a subfield of AI that trains GenAI systems to analyze datasets and recognize patterns. One important type of ML used to train GenAI models is Deep Learning, which involves layering neural networks to understand complex data.
Large Language Models (LLMs) are a type of GenAI model that specialize in processing human language, exemplified by OpenAI's GPT series. These models use Machine Learning, especially Deep Learning, to power their Natural Language Processing (NLP) abilities.
A text-based generative AI model can generate new content based on its input, such as generating a new paragraph or story based on a sentence or paragraph. Similarly, an image-based generative AI model can generate a new similar image but not identical to the original.
Here are some key characteristics of GenAI models:
- They can learn from data and generate new content based on that data.
- They use Machine Learning techniques to process and learn from vast datasets.
- They can be used for various applications, such as generating text or images.
The History of
The History of Generative AI is a fascinating story that dates back to the 1950s, when computer programmers developed the first neural networks (NNs) inspired by the human brain.
These early NNs used machine learning to independently analyze data sets and identify patterns, laying the foundation for future developments.
The 2000s-2010s marked a turning point in the evolution of Generative AI, with AI researchers revisiting NNs and introducing new techniques.
In 2014, computer scientist Ian Goodfellow introduced Generative Adversarial Networks (GANs), a breakthrough in the field of GenAI, which enabled the production of highly realistic data.
The transformer model, first introduced in 2017 by Google researchers, revolutionized GenAI by allowing Large Language Models (LLMs) to process large unlabeled bodies of text and identify word meanings from context.
This breakthrough enabled the development of models like OpenAI's GPT, which gained over 100 million users in just two months after its release in November 2022.
Expand your knowledge: Telltale Words Identify Generative Ai Text
Types and Models
Generative AI models come in different forms, each with its unique capabilities and applications. Generative Adversarial Networks (GANs) are a type of model that uses two neural networks, a generator and a discriminator, to create highly realistic content such as images, videos, and audio.
GANs are used for generating text, artwork, images, audio, video, style transfer, and more. They're also capable of creating lifelike "deepfake" videos that are almost indistinguishable from real videos.
Other notable models include Variational Autoencoders (VAEs) and Transformers. VAEs specialize in generating representations of data, while Transformers possess self-attention mechanisms and layered neural networks, making them widely used in natural language processing and machine learning tools.
Here are some key features of these models:
- GANs: Consist of a generator and discriminator, used for creating realistic content
- VAEs: Specialize in data representation, generation, and augmentation
- Transformers: Use self-attention mechanisms and layered neural networks, widely used in NLP and machine learning
What Are Dall-E, ChatGPT, Bard & MosaicML?
Dall-E, ChatGPT, Bard, and MosaicML are popular generative AI tools.
Dall-E is an image generation model that was first released by OpenAI in January 2021. It generates images based on textual descriptions and also offers prompt rewriting assistance and adjustments to image quality and detail.
Curious to learn more? Check out: Dall-e Generative Ai
ChatGPT is a Large Language Model (LLM) designed to generate human-sounding text or data visualization in response to prompts. It was first released in November 2022 by OpenAI and gained over 100 million users in just two months.
Bard is an LLM released by Google in March 2023 that functions similarly to ChatGPT and generates human-like content based on prompts. It can also help with math equations and analyze YouTube videos.
MosaicML is a platform for enterprises to create and customize their own Generative AI models. It was first launched in 2021 and then acquired by Databricks in July 2023 for $1.3 billion dollars.
Here's a brief comparison of these popular generative AI tools:
Types of Models
Generative AI models have come a long way in recent years, and there are several types of models that have gained popularity. One of the most notable types is Generative Adversarial Networks (GANs), which use two neural networks to generate new data points that mimic a given dataset.
For another approach, see: What Are the Types of Data in Generative Ai
GANs are used for generating text, artwork, images, audio, video, and more. They're also capable of creating lifelike "deepfake" videos that are almost indistinguishable from real videos.
Another type of model is Variational Autoencoders (VAEs), which specialize in generating representations of data. VAEs consist of two neural networks: an encoder that maps the essential features of input data and a decoder that generates new data resembling the input data.
VAEs are used for data compression, detecting anomalies in data, "denoising" or clarifying data, labeling data, machine learning, image generation, enhancing image resolution, style transfer, and more.
Transformers are also a type of model that has gained significant attention in recent years. They're versatile GenAI models widely used in natural language processing and machine learning. A key feature of Transformers is their self-attention mechanism, which enables them to consider different parts of the input data simultaneously.
Here are some key characteristics of these models:
Transformers are the foundational framework of OpenAI's GPT series, Google's BERT, and various other natural language processing and machine learning applications. They're used for computer vision, speech recognition, robotics, medical drug discovery, and more.
In summary, these models are the building blocks of Generative AI, and each has its unique strengths and applications. By understanding these models, you can unlock the full potential of Generative AI and explore new possibilities in various fields.
Applications and Benefits
Generative AI has a wide range of applications that include text generation, image and video creation, voice generation, music generation, and game development. These applications are used in various industries such as retail, manufacturing, and travel.
Some of the benefits of generative AI include rapid content generation, data augmentation, personalized solutions, and fostering creativity. For example, Amazon launched GenAI tools to help sellers write product descriptions and enable advertisers to create custom product images.
Here are some specific use cases for generative AI:
- Text generation for content creation, such as automatically generated articles, posts, and captions.
- Image generation and manipulation, such as creating images based on textual descriptions using tools like Dall-E.
- Data augmentation for machine learning models, such as creating synthetic data to enhance training datasets.
- Personalized solutions, such as virtual customer support agents and chatbots that offer tailored customer experiences.
These applications and benefits make generative AI a valuable tool for businesses and individuals looking to streamline their creative process and improve their content generation capabilities.
What Are the Benefits of?
Generative AI offers rapid content generation, which can save businesses time and resources. This is especially useful for companies that need to produce a high volume of content, such as social media posts or blog articles.
Data augmentation is another key benefit of generative AI. By creating synthetic data, businesses can enhance their training datasets for machine learning models, leading to improved accuracy and reduced costs.
Intriguing read: Generative Ai Content
Generative AI can also foster creativity, allowing users to explore new ideas and possibilities. For example, tools like Dall-E can generate images based on textual descriptions, opening up new possibilities for artists and designers.
Here are some specific benefits of generative AI:
Personalized solutions are another benefit of generative AI. By generating customized content and solutions, businesses can provide users with tailored experiences that meet their individual needs.
Broaden your view: Develop Generative Ai Solutions with Azure Openai Service
Gen in Retail
In retail, GenAI is changing the game. Major online retailers like Amazon, L’Oreal, and Wayfair are already utilizing GenAI technology.
Amazon launched GenAI tools in 2023 to help sellers write product descriptions and enable advertisers to create custom product images. This is a huge time-saver for businesses and allows for more personalized marketing.
L’Oréal is using GenAI to allow customers to virtually try on makeup, making the shopping experience more interactive and fun. Wayfair released the Decorify app in 2023, enabling customers to view realistic images of furniture in their own home.
Expand your knowledge: Generative Ai Product Design
Retailers are increasingly using GenAI to personalize their marketing, product recommendations, and user experience based on customer profiles. This means customers are more likely to see products they're interested in, making their shopping experience more enjoyable.
Here are some ways GenAI is being used in retail:
- Personalized marketing
- Product recommendations
- User experience based on customer profiles
- Virtual customer support agents and chatbots
GenAI-powered virtual customer support agents and chatbots are also becoming more common, helping to streamline customer service and free up human resources for more strategic tasks.
On a similar theme: Generative Ai Customer Support
Gen in Manufacturing
Gen in Manufacturing is revolutionizing the industry in amazing ways. Deloitte estimates that Predictive Maintenance increases productivity by 25%, reduces breakdowns by 70%, and lowers maintenance costs by 25%.
Leading companies like IBM, General Electric, SAP, and Siemens are already using GenAI to anticipate and prevent equipment failures. This technology is so powerful that it's changing the manufacturing game.
Boeing uses GenAI-powered technology to generate and test virtual prototypes of their airplanes, simulating the production process. This helps them identify and fix issues before they become major problems.
Suggestion: Generative Ai for Manufacturing
General Motors uses generative models to optimize and test lightweight automotive parts, resulting in significant weight reductions on certain vehicles. By reducing weight, they're improving fuel efficiency and performance.
By analyzing data on product performance, generative AI can help manufacturers optimize product design, improving functionality and reducing costs. This is a major win for companies looking to stay competitive in the market.
GenAI can also help manufacturers predict equipment failures, enabling them to perform maintenance before a breakdown occurs. This reduces downtime and keeps production running smoothly.
Recommended read: How Are Modern Generative Ai Systems Improving User Interaction
Travel & Hospitality
Travel & Hospitality is where GenAI is truly making a splash. GenAI tools are enhancing customer support and customizing travel plans, with travel booking platforms like Expedia, Booking.com, and Marriott International using GenAI-powered chatbots since 2017.
These advanced chatbots are helping businesses offer their customers even more travel support and personalization. Kayak and Expedia have integrated with ChatGPT, creating virtual travel assistants that can answer questions like "Where can I fly to from NYC for under $500 in April?" and provide personalized recommendations.
Airbnb's CEO has announced plans to build the "ultimate AI concierge" that learns about a user over time to create a tailored customer experience. This is a game-changer for travelers who want a seamless and personalized experience.
Curious to learn more? Check out: Generative Ai in Tourism
Advantages of Public Cloud
Deploying generative AI on public cloud platforms offers numerous advantages. You can immediately remove the costs and time associated with developing your own models by leveraging foundational models available on public cloud providers.
Using public cloud for generative AI can save you a significant amount of time and resources. According to recent trends, hiring a data scientist and developing models from scratch is no longer necessary.
Public cloud providers continuously develop and update their models, allowing you to stay up-to-date with the latest advancements in generative AI. This means you can focus on building applications and solutions rather than investing time and resources in model development.
Developing your own models can be a complex and time-consuming process. By using public cloud providers, you can tap into their expertise and knowledge of how models behave, which can significantly reduce the time-to-market.
Here are some of the key advantages of using public cloud for generative AI:
Overall, using public cloud for generative AI can save you time, money, and resources, allowing you to focus on building innovative applications and solutions.
Fostering Creativity
Generative AI has the power to foster creativity in various ways, including style transfer, image manipulation, and art creation. This technology allows users to enter a text description of a desired artistic image and receive an original image that brings their idea to life.
DeepDream models, for example, enable users to specify stylistic features and receive an original image that matches their vision. GenAI can also be used to generate music, poetry, architectural designs, and virtual gaming worlds, all in a matter of seconds.
One of the most impressive applications of GenAI in creativity is music generation. By analyzing existing musical patterns and structures, these models can generate new melodies, harmonies, and rhythms. This finds applications in various domains, including background music for videos, video games, and other multimedia projects.
Musicians and producers can even use generative AI as a tool for inspiration, generating musical ideas that they can further develop into complete compositions. This can be a game-changer for creatives, freeing them up to focus on the creative process rather than getting bogged down in tedious tasks.
Curious to learn more? Check out: Generative Ai Human Creativity and Art Google Scholar
Here are some examples of GenAI tools that can help foster creativity:
- DeepDream: A model that allows users to enter a text description of a desired artistic image and receive an original image that matches their vision.
- Style transfer: A technique that enables users to transfer the style of one image to another, creating unique and captivating visuals.
- Music generation: A tool that analyzes existing musical patterns and structures to generate new melodies, harmonies, and rhythms.
What Are the Limitations of?
As you start exploring generative AI, it's essential to understand its limitations. Generative AI technology is progressing quickly, but it's not without its challenges.
One of the significant limitations is the need for large training datasets. This means compiling and structuring terabytes of data, or billions of data points, to capture the nuance of natural language.
Large Language Models (LLMs), like OpenAI's GPT architecture, require extensive and diverse datasets for training. This can be a significant hurdle for smaller organizations or developers who may not have access to the necessary resources.
Generative models need tremendous processing power, memory, technical infrastructure, and expertise to facilitate the Machine Learning process. This can be a barrier for those who don't have the necessary technical capabilities.
Another limitation is the potential for generating misleading information. Generative models are trained on past data and often lack up-to-date information. They may replicate inaccuracies from biased or incorrect training data, which can lead to factually incorrect answers.
Here are the main limitations of generative AI in a nutshell:
- Large training datasets: Requires extensive and diverse datasets for training.
- Massive computational requirements: Needs tremendous processing power, memory, and technical infrastructure.
- Generating misleading information: May replicate inaccuracies from biased or incorrect training data.
Future and Trends
The future of Generative AI is looking bright, with predictions that it will perform at a median human level by 2030. McKinsey's August 2023 report suggests that GenAI will make significant strides in capabilities like social, emotional, and logical reasoning, natural language understanding, and problem solving.
Improved realism and customization are expected to be key features of future generative models. These models will be able to produce even more realistic and personalized outputs that are indistinguishable from human-created content. Users will have more control over the style, tone, and other specific characteristics of generated content.
Here are some specific possibilities for the future of GenAI:
- Improved realism and customization: GenAI models will produce more realistic and personalized outputs.
- Multimodal capabilities: GenAI technology will handle multiple modalities simultaneously, such as generating an image and corresponding text description.
- Integration with emerging technologies: GenAI will integrate with AR and VR to create immersive and interactive user experiences.
- Expanded industry applications: Generative models will be tailored and refined for specific industries like healthcare, finance, or entertainment.
- Ethical and responsible use: Future advancements in Generative AI will prioritize responsible practices and regulations.
The Future of
By 2030, McKinsey predicts that Generative AI (GenAI) will perform at a median human level for various capabilities, including social, emotional, and logical reasoning, natural language understanding, and problem solving.
Improved realism and customization are expected to be key features of future GenAI models, allowing users to create highly personalized and realistic content that's indistinguishable from human-created content.
Worth a look: Generative Ai Human Creativity and Art
In fact, future GenAI models will likely produce even more realistic and personalized outputs, with users having more control over the style, tone, and other specific characteristics of generated content.
Here are some potential applications of improved realism and customization in GenAI:
- Personalized marketing content that resonates with specific audiences
- Realistic virtual environments for immersive gaming experiences
- Customized educational content that adapts to individual learning styles
Multimodal capabilities will also become increasingly important, allowing GenAI models to handle multiple modalities simultaneously, such as generating an image and corresponding text description simultaneously.
This will enable the creation of more immersive and interactive user experiences, such as virtual reality (VR) and augmented reality (AR) applications that integrate GenAI with emerging technologies.
In the near future, we can expect to see GenAI models that integrate seamlessly with AR and VR to create realistic virtual environments and character interactions in real-time.
As GenAI continues to advance, we can also expect to see expanded industry applications, with customized models being tailored and refined for specific industries like healthcare, finance, and entertainment.
These industry-specific models will be able to better understand and generate content specific to the nuances and use cases of each industry, leading to more accurate and effective applications.
Check this out: Generative Ai in Chemical Industry
Trends in Organisational Cases
Organisations are pursuing various use cases with generative AI, which can be grouped into five main categories: summarisation, inferring and classifying, extraction and transformation, Q&A, and content generation.
These use cases aim to provide decision support, boost efficiency, and extract the hidden potential of an organisation's own data, such as customer feedback, product data, contracts, or employee resources.
Generative AI adoption involves seven basic phases, which are designed to address the various challenges and opportunities that arise as you scale adoption.
The first phase is awareness and education, where you learn about generative AI technologies, their capabilities, and potential use cases.
You should also identify specific problems or opportunities where generative AI can add value in the organisation, evaluate generative AI tools, platforms, and models, and experiment with generative AI technologies through proof-of-concept (POC) projects and pilot programmes.
The third phase involves outlining goals, timelines, and resource requirements for generative AI adoption, identifying and evaluating the infrastructure needed, and establishing guidelines and processes for ethical considerations like data privacy, fairness, and potential misuse.
Here are the seven phases of generative AI adoption:
- Awareness and education
- Assessment and experimentation
- Planning and preparation
- Implementation and integration
- Monitoring and evaluation
- Scaling and expansion
- Continuous improvement and innovation
Industry leaders are applying generative AI in various sectors, including retail, consumer packaged goods, manufacturing, banking, finance, telecommunications, healthcare, travel, and hospitality.
Cloud and Infrastructure
All three major hyperscalers - Azure, AWS, and Google Cloud - offer access to generative AI tools via APIs. These tools enable text and image generation.
You'll need to consider security, scalability, performance, data quality, and ethics when deploying OpenAI, as outlined in the article "Deploying OpenAI with Public Cloud: Dos & Don'ts".
Azure, AWS, and Google Cloud each have their own approach to generative AI, but they all share the common goal of making AI more accessible and user-friendly.
If you're planning to deploy OpenAI, be sure to check out the article "OpenAI & Cloud: 2 Use Cases We Built & What We Learned" for some valuable insights and lessons learned.
Here are some key considerations to keep in mind when deploying generative AI in the cloud:
Generative AI Techniques
Generative AI models rely on Machine Learning techniques to process and learn from vast datasets, enabling them to generate new content based on their input.
Machine Learning is a subfield of AI that enables GenAI systems to learn from data, and it's a key component of GenAI and Large Language Models (LLMs). This is why enterprises are setting their focus on Large Language Model operations (LLMOps) to develop, deploy, monitor, and maintain LLMs that meet their specific use cases.
Neural Networks (NNs) have enabled Generative AI to produce more creative, detailed, and diverse outputs. By adding deeper, more complex layers of neurons to NNs, GenAI models are able to learn from complicated data distributions and generate increasingly realistic content.
Deep Learning, a type of Machine Learning, involves layering neural networks to understand complex data. This is why many enterprises are developing and scaling ML models through ML operations (MLOps) to improve the efficiency of their organizations.
Here are some key techniques used in Generative AI:
- Text-based generative AI models generate new content based on input, such as a sentence or paragraph.
- Image-based generative AI models generate new similar images based on input.
- Large Language Models (LLMs) use Machine Learning, especially Deep Learning, to power their Natural Language Processing (NLP) abilities.
Neural Networks have transformed the fields of Machine Learning and Natural Language Processing, and have enabled GenAI models to learn from complicated data distributions and generate increasingly realistic content.
Training and Architecture
To create a generative AI model, you need a large dataset to train on, which can be text, images, or any other data type. The more data the model has, the better it will be at generating new content.
The most common type of generative AI model is a Generative Adversarial Network (GAN), which consists of two neural networks: a generator and a discriminator. The generator creates new content based on the input it receives, while the discriminator evaluates the content and provides feedback to the generator.
Here's a breakdown of the key components of a GAN:
- Generator: creates new content based on input
- Discriminator: evaluates content and provides feedback
Model Architecture
Generative AI models come in various architectures, each with its unique approach to generating content. One of the most common types of generative AI models is a Generative Adversarial Network (GAN), which consists of two neural networks: a generator and a discriminator. The generator creates new content based on the input it receives.
Recommended read: Generative Ai Content Creation
Autoencoders are another type of generative AI model, which are trained to encode input data into a lower-dimensional representation and then decode it back to the original input. They consist of two main parts: an encoder and a decoder.
Deep learning architectures, an advanced system of neural networks, have revolutionized generative AI by enabling models to learn from complicated data distributions and generate increasingly realistic content. This has led to the development of more sophisticated and powerful generative AI models.
Variational Autoencoders (VAEs) are a type of autoencoder that uses probabilistic models to encode the input data and generate new samples from the encoded data distribution. This allows VAEs to generate more realistic and diverse data, making them a powerful tool for generative AI.
A unique perspective: Can I Generate Code Using Generative Ai Models
Training
Training is a crucial step in creating a generative AI model. A large dataset is needed for the model to learn from, and the more data it has, the better it will be at generating new content.
This dataset can be text, images, or any other data type the model will generate. The quality and quantity of the data will directly impact the model's ability to learn and generate content.
Before the model can start training, the data must be preprocessed to make it easier for the model to understand. This can involve converting the data into a format that the model can work with, such as converting images into pixels or text into numerical vectors.
The model architecture is defined, and the model is trained using the preprocessed data. During training, the generator creates new content based on random inputs, and the discriminator evaluates the content to determine if it is real or fake.
Here's a breakdown of the training process:
The more complex the data distribution, the more layers of neurons are needed in the model to learn from it. This is why deep learning architectures have revolutionized generative AI by enabling models to learn from complicated data distributions.
Comparison and Assessment
Generative AI is a type of AI that can create new content, such as images, music, or text, based on patterns and structures it has learned from existing data. This is a key distinction from traditional AI, which is typically focused on processing and analyzing existing data.
The three main types of generative AI are Generative Adversarial Networks (GANs), Variational Autoencoders (VAEs), and Recurrent Neural Networks (RNNs). GANs are particularly well-suited for generating images and videos, while VAEs are often used for generating text and music.
One of the most significant advantages of generative AI is its ability to create unique and diverse content. For example, a generative AI model can generate a new image that is similar to a given image, but with its own distinct characteristics. This can be useful for applications such as art and design.
However, generative AI can also be limited by its reliance on existing data. If the training data is biased or incomplete, the generated content may reflect these flaws. For instance, a generative AI model trained on a dataset of images of people may produce images of people that are predominantly of one racial or ethnic group.
You might like: Getty Images Nvidia Generative Ai Istock
In terms of practical applications, generative AI has the potential to revolutionize fields such as art, music, and design. For example, a generative AI model can be used to generate new music that is similar to a given style or genre. This can be useful for musicians who want to create new music, but may not have the time or expertise to do so themselves.
A different take: Google Announces New Generative Ai Search Capabilities for Doctors
Examples and Use Cases
Generative AI is being applied in various industries, including retail, consumer packaged goods, manufacturing, banking, finance, telecommunications, healthcare, and travel. It's used in diverse use cases, such as generating realistic images and videos, creating natural language responses in chatbots, and summarizing large amounts of data.
Some examples of generative AI tools include Dall-E, ChatGPT, Bard, and MosaicML, which are popular models for generating images, text, and code. StyleGAN, developed by NVIDIA, specializes in generating high-quality, photorealistic images, particularly portraits of people.
A fresh viewpoint: Generative Ai by Getty
Generative AI has many potential use cases, from generating realistic images and videos to creating natural language responses in chatbots. These use cases include summarization, inferring and classifying, extraction and transformation, Q&A, and content generation.
There are 5 main categories of use cases for generative AI: summarization, inferring and classifying, extraction and transformation, Q&A, and content generation. These use cases aim to provide decision support, boost efficiency, and extract the hidden potential of an organization's own data.
Here are some specific examples of generative AI use cases:
- Summarization: condensing large amounts of text into a shorter summary
- Inferring and classifying: identifying patterns and making predictions based on data
- Extraction and transformation: extracting data from one format and transforming it into another
- Q&A: generating natural language responses to user queries
- Content generation: creating new content, such as text, images, or videos
These use cases are being pursued by organizations across various industries, and are aimed at providing decision support, boosting efficiency, and extracting the hidden potential of an organization's own data.
Frequently Asked Questions
What is the difference between ChatGPT and generative AI?
ChatGPT is a specialized text generation tool, whereas generative AI is a broader category that encompasses all AI systems capable of creating new content. Understanding this difference helps choose the right AI tool for your specific needs.
Featured Images: pexels.com