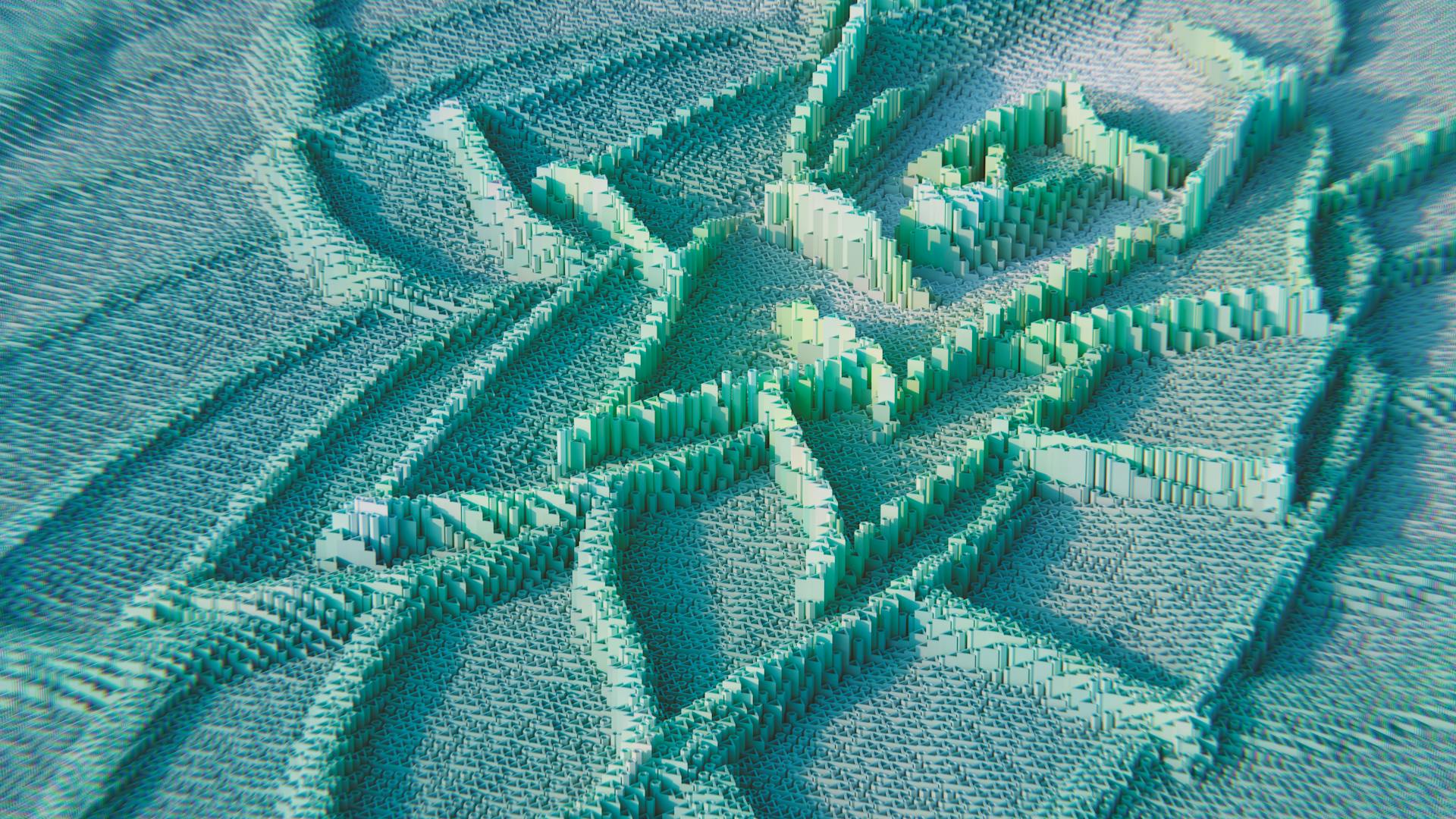
Generative AI is revolutionizing the chemical industry, enabling companies to streamline processes, reduce costs, and increase innovation.
By applying generative AI to molecule design, researchers have discovered new compounds with improved properties, such as increased potency or reduced toxicity.
One notable example is the discovery of a new cancer-fighting compound that was 100 times more effective than existing treatments.
Generative AI is also being used to optimize chemical reactions, reducing energy consumption and waste production.
Consider reading: New Generative Ai
Generative AI in Chemical Industry
Generative AI in the chemical industry is revolutionizing the way companies approach sales, research, and production. Generative AI can turn every member of a team into an SME, opening the door to better conversations, deeper trust, and more consistent sales results.
In the chemical manufacturing industry, generative AI use cases are transforming organizations. At Nesh, we provide GenAI tools that drive sales in complex industries, enhancing knowledge across the entire organization.
Generative AI can identify new molecular structures that fulfill property requirements like binding affinity, solubility, and synthesizability. This can be a game-changer for the chemical industry, especially considering the length and costs of the drug discovery and chemical compound design process.
Additional reading: Generative Ai in Sales
Machine learning tools can provide scientists with efficient ways to screen numerous chemical combinations or reactions and their outcomes. This can bring us closer to a greener future, making it easier for chemical companies to produce substances with matching properties to plastic or petroleum products but break down easier and without waste or pollution.
Generative AI can also help design new production lines, both small-scale and large-scale, by advanced modeling of various parameters of a newly developed substance. This can be achieved through deep learning techniques, such as generative modeling (GANs, RL, AE).
Challenges and Opportunities
Despite the promising potential of GenAI in chemical engineering, its use and implementation come with significant challenges and limitations. Successfully addressing these challenges will require international collaboration among all stakeholders.
One of the foremost issues is the quality and availability of data. GenAI models, such as LLMs, need vast amounts of high-quality, domain-specific data to train effectively. In chemical engineering, such data is often proprietary, sparse, or inconsistent.
Many large GenAI models often hallucinate, and provide little to no insight into how they arrive at specific solutions. This lack of transparency can be a significant barrier to adoption in the safety-critical applications often encountered in chemical engineering.
Worth a look: Generative Ai Photoshop Increase Quality
Challenges in Chemical Manufacturing
The chemical manufacturing process can be a long and costly endeavor, with the drug discovery and chemical compound design process taking a significant amount of time and resources.
One of the main challenges is the difficulty in predicting the properties of new chemical compounds. This is where AI comes in, enabling advanced modeling of various parameters of a newly developed substance.
The process of chemical property prediction involves feeding the components of a molecule to a neural network, which is then trained to recognize particular properties based on a dataset of already applied molecules.
The length and costs of the drug discovery and chemical compound design process can be a real challenge for the chemical industry, but AI and machine learning offer a potential solution.
Generative modeling, such as GANs, RL, and AE, can identify new molecular structures that fulfill specific property requirements, like binding affinity, solubility, and synthesizability.
This can be a game-changer for the chemical industry, enabling manufacturers to design new production lines and create compounds that are less expensive or easier to process.
You might enjoy: What Is the Role of Generative Ai in Drug Discovery
Opportunities for AI Adoption
The opportunities for AI adoption in chemical engineering are vast and exciting. Generative AI models, such as LLMs, can be trained to recognize patterns and make predictions in chemical engineering, but they require vast amounts of high-quality, domain-specific data to train effectively.
One of the most promising applications of AI in chemical engineering is chemical property prediction. By feeding molecular components into a neural network, manufacturers can detect compounds with desirable features, such as lower costs or easier breakdown.
Generative modeling, particularly GANs, RL, and AE, is the most powerful technique in identifying new molecular structures that fulfill specific property requirements, like binding affinity or solubility. This can be a game-changer for the chemical industry, especially in the expensive and time-consuming process of drug discovery and chemical compound design.
AI can also enhance the interpretability and trustworthiness of GenAI models by integrating well-established first principles modeling in chemical engineering. This can help address the lack of transparency and trust in AI decision-making in safety-critical applications.
The potential of machine learning for the chemical manufacturing industry is vast, and companies can take advantage of AI to produce environmentally friendly alternatives to substances with negative impacts on the environment.
A different take: Travel Industry and the Use of Generative Ai
The Future of Chemicals
Generative AI is transforming the chemical manufacturing industry by turning every member of the team into a subject matter expert, enhancing knowledge across the organization.
Driving sales results in the chemical industry now relies on generative AI, which opens the door to better conversations, deeper trust, and more consistent sales results.
The chemical industry is shifting towards sustainability, with generative AI playing a crucial role in developing environmentally friendly alternatives to substances with negative impacts.
Machine learning tools can efficiently screen numerous chemical combinations or reactions and their outcomes, making it easier for companies to produce greener substances.
Generative AI can help chemists search for new molecules with healing properties or alternatives to substances in wide use that harm the environment.
AI can bring us closer to a greener future by making it easier for chemical companies to produce substances that break down easily and without waste or pollution.
The future of chemicals production is looking bright, with AI technology enabling companies to optimize manufacturing processes and research environmentally friendly alternatives.
By leveraging machine learning, chemical companies can unlock new possibilities and create a more sustainable future.
For your interest: How Are Companies Using Generative Ai
Chemical Industry and AI
The chemical industry is on the cusp of a revolution, thanks to the power of AI. Generative AI is being used to transform the industry, and it's already showing promising results.
Generative AI can predict chemical properties, allowing manufacturers to detect compounds with desirable features that are less expensive or easier to process. This is done by feeding the components of molecules to a neural network, which is trained to recognize specific properties based on a dataset of already applied molecules.
The application of generative AI in the chemical industry can be a game-changer, particularly in the area of drug discovery and chemical compound design. It's estimated that generative modeling, a type of generative AI, can identify new molecular structures that fulfill specific property requirements.
AI can also help design new production lines, both small-scale and large-scale, by modeling various parameters of a newly developed substance. This can lead to increased efficiency and reduced costs in the manufacturing process.
Intriguing read: Which Term Describes the Process of Using Generative Ai
Machine learning tools can provide scientists with efficient ways to screen numerous chemical combinations or reactions and their outcomes. This can help bring us closer to a greener future, where chemical companies can produce substances that have matching properties to plastic or petroleum products but break down easier and without waste or pollution.
Sources
- https://www.infotech.com/research/ss/generative-ai-use-case-library-for-the-chemical-pharmaceutical-manufacturing-industry
- https://www.frontiersin.org/journals/chemical-engineering/articles/10.3389/fceng.2024.1458156/full
- https://www.hellonesh.io/blog/generative-ai-in-chemical-manufacturing-use-cases-for-technical-sales-teams
- https://www.chemicalprocessing.com/automation-it/article/33008789/ai-in-the-chemical-industry-from-molecule-discovery-to-contract-compliance
- https://nexocode.com/blog/posts/ai-in-chemical-manufacturing/
Featured Images: pexels.com