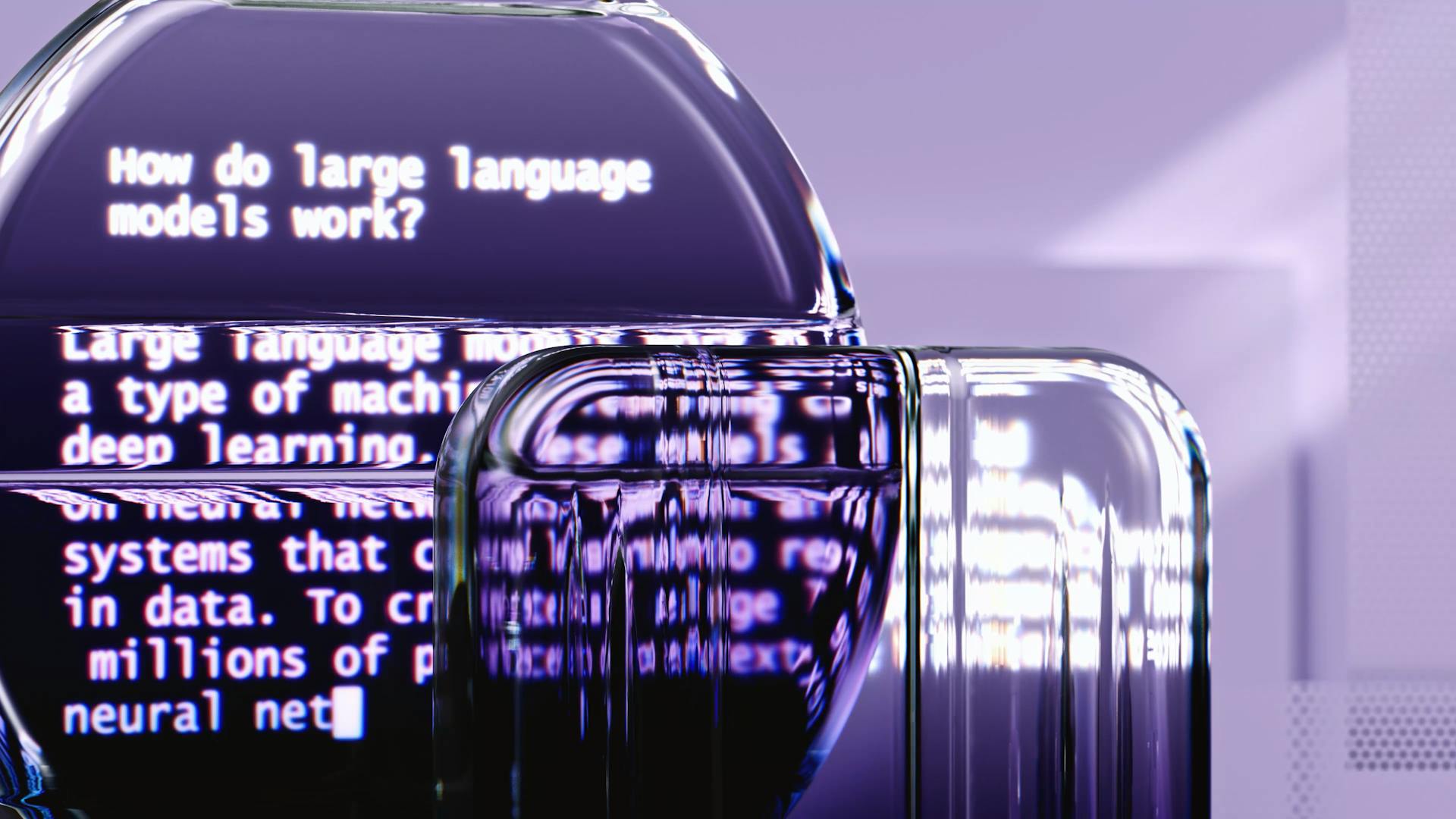
Generative AI is revolutionizing the field of drug discovery by enabling the rapid design and optimization of new compounds.
Researchers are using generative AI to generate thousands of potential lead compounds in a matter of hours, a process that would take years by traditional methods.
One of the key benefits of generative AI in drug discovery is its ability to identify novel chemical structures that would be difficult or impossible for humans to design.
This is achieved through the use of machine learning algorithms that can analyze vast amounts of data and identify patterns that are not immediately apparent to humans.
A different take: Can Generative Ai Replace Humans
What is Generative AI in Drug Discovery?
Generative AI in drug discovery involves training models on large datasets of known molecular structures and their associated properties.
This process can generate new molecular structures with similar or improved properties, which can then be optimized and tested for efficacy and safety.
Traditional drug discovery methods are time-consuming and expensive, often taking years and billions of dollars to bring a new drug to market.
Generative AI techniques, such as deep learning and reinforcement learning, have the potential to revolutionize this process by automating the generation of novel molecular structures.
How Generative AI Works in Drug Discovery
Generative AI in drug discovery leverages machine learning models, particularly generative adversarial networks (GANs) and transformer models, to create new drug candidates. These AI models are trained on vast chemical compounds and biological data datasets, enabling them to generate novel molecules that meet specific therapeutic criteria.
Generative AI can design new molecules by predicting the components in a molecule's structure, which might not be easily discovered through conventional methods. It can also optimize existing compounds by suggesting modifications to enhance their efficacy, reduce side effects, and improve their drug-like properties. This capability is crucial for refining drug candidates before they enter clinical trials.
Generative AI can predict biological activity, forecasting how a new drug will interact with biological targets, and help prioritize the most promising candidates for further development. By automating much of the molecule generation process, AI can rapidly produce new chemical structures that could become the next big drug.
For another approach, see: Generative Ai in Chemical Industry
Use Cases
Generative AI is leading a revolution in the pharmaceuticals industry by providing innovative solutions to address some of the most complex challenges in drug discovery.
De Novo Drug Design involves creating entirely new molecules from scratch, which can be achieved through generative AI predicting and designing molecular structures with desirable properties.
A leading biotech firm used generative AI to design a new antiviral drug that demonstrated high efficacy against various viruses, moving quickly from concept to preclinical trials.
Generative AI helps optimize existing drug compounds by suggesting modifications to enhance their efficacy, reduce side effects, and improve their overall drug-like properties.
A pharmaceutical company leveraged generative AI to improve the binding affinity of a cancer drug, resulting in a more potent and safer treatment option that advanced to clinical trials.
Virtual Screening utilizes generative AI to evaluate how millions of compounds interact with specific biological targets, dramatically reducing the time and cost associated with physical screening.
On a similar theme: New Generative Ai
A research institution used generative AI to screen for inhibitors of a novel bacterial enzyme, quickly identifying several potent candidates that were further validated in laboratory tests.
Drug repurposing entails identifying new therapeutic applications for existing drugs, which generative AI can speed up by analyzing extensive datasets.
An AI-driven drug discovery company repurposed an existing cardiovascular drug for treating a rare autoimmune disorder, significantly shortening the time to clinical testing.
Generative AI aids in the development of personalized medicine by analyzing individual genetic and clinical data to create tailored treatment plans.
A healthcare startup used generative AI to design personalized cancer treatments based on patient’s genetic profiles, resulting in better outcomes and reduced side effects.
Generative AI excels in biomarker discovery, identifying biomarkers associated with specific diseases by analyzing large datasets.
A biotech firm utilized generative AI to discover new biomarkers for early detection of Alzheimer’s disease, leading to more accurate diagnostic tests.
Generative AI provides new approaches to drug optimization, enhancing therapeutic agents’ efficacy and binding affinity.
A pharmaceutical company employed generative AI to optimize a potential diabetes treatment, improving its effectiveness and safety profile before clinical trials.
You might enjoy: Generative Ai and Cyber Security
Techniques
Generative AI in drug discovery leverages machine learning models, particularly generative adversarial networks (GANs) and transformer models, to create new drug candidates.
GANs consist of two neural networks: the generator and the discriminator. The generator creates new synthetic data, while the discriminator evaluates this data against actual data to distinguish authenticity.
Reinforcement learning optimizes molecular structures by adjusting their properties to meet desired criteria. This approach enables the precise adjustment of molecules to boost their effectiveness and minimize potential side effects.
Natural Language Processing (NLP) empowers computers to comprehend and extract valuable insights from textual data. NLP is essential in target identification, drug repurposing, and knowledge extraction in drug discovery.
Variational Autoencoders (VAEs) are another type of deep learning model used in generative AI for drug discovery. VAEs consist of an encoder and a decoder network, allowing for the generation of diverse and novel structures with desired properties.
Generative AI techniques like GANs, reinforcement learning, NLP, and VAEs are revolutionizing the pharmaceutical and biotech industries by enabling the creation of new molecular structures with remarkable precision and speed.
These advanced methods leverage AI to enhance drug discovery, increasing efficiency and cost-effectiveness. By automating much of the effort, generative AI can rapidly produce new chemical structures that could become the next big drug.
Rare Diseases
Rare diseases are a significant challenge for the medical community, affecting millions of people worldwide. Generative AI offers a promising solution by providing a cost-effective and rapid approach to identifying therapeutic leads.
Traditional drug discovery efforts for rare diseases are often limited by resource constraints. Generative AI can help bridge this gap by streamlining the process and reducing costs.
For rare and neglected diseases, generative AI can be a game-changer. It enables researchers to identify potential therapeutic leads quickly and efficiently, which can lead to new treatments and improved patient outcomes.
Benefits and Advantages of Generative AI in Drug Discovery
Generative AI is transforming the field of drug discovery, offering numerous benefits that enhance efficiency, reduce costs, and improve outcomes. It significantly speeds up the drug discovery timeline by rapidly generating novel compounds and optimizing existing ones.
Developing a new drug typically costs around USD 2.6 billion, but generative AI reduces these costs by streamlining the discovery phase and minimizing the need for extensive lab work.
Generative AI utilizes advanced algorithms to design drug molecules with high precision, ensuring that the compounds created possess the intended properties.
Integrating generative AI in drug discovery leads to higher success rates, with AI helping to identify the most promising drug candidates.
Generative AI facilitates the development of personalized treatments by analyzing individual patient data, such as genetic profiles.
Virtual screening powered by generative AI allows researchers to quickly analyze how millions of compounds interact with specific biological targets.
Generative AI excels in drug repurposing by analyzing existing medications and identifying new therapeutic uses.
By accelerating the identification and optimization of drug candidates, generative AI has the potential to significantly reduce the time and financial resources required to bring new drugs to market.
Generative AI can predict how likely a drug is to succeed in clinical trials, helping researchers to concentrate on the most promising medications.
The ability to explore a broader chemical space and predict potential issues early on can significantly increase the success rate of drug discovery projects.
Here are some of the key benefits of generative AI in drug discovery:
- Accelerated drug development
- Reduced costs
- Enhanced precision and accuracy
- Improved success rates
- Personalized medicine
- Efficient virtual screening
- Innovative drug repurposing
- Robust compound library expansion
- Biomarker discovery
Challenges and Considerations of Generative AI in Drug Discovery
Generative AI in drug discovery comes with its own set of challenges. These difficulties include poor data quality, which can result in poor models and inaccurate predictions.
Data quality is a major concern because generative AI relies on existing data for training. If the input data is poor, the output will also be poor. This is a critical issue because it can lead to the development of ineffective or even harmful medications.
Accessing a Generative AI system with the right data can be costly, but companies are now creating solutions tailored specifically for drug discovery, making this advanced technology more accessible.
Consider reading: Generative Ai Photoshop Increase Quality
Data Constraints
Data Constraints can be a significant hurdle in drug discovery, but Generative AI offers a solution by producing synthetic data sets that resemble real data. This is particularly advantageous when actual data is limited or difficult to get.
Generative AI can help circumvent the lack of adequate data by generating synthetic data sets. This allows research to continue without delay, which is crucial in the drug discovery process.
For your interest: Synthetic Data Generation with Generative Ai
The absence of quality data can lead to poor models and inaccurate predictions, which can be detrimental to the entire drug development process. Poor data quality is a major challenge in Generative AI.
Generative AI can help minimize the impact of poor data quality by producing high-quality synthetic data sets. This can ensure that the models developed are accurate and reliable.
However, it's essential to note that Generative AI models are only as good as the data from which they are trained and developed. Inaccurate or biased data can lead to model distortion and inaccuracy.
Addressing data constraints is critical in realizing the full potential of Generative AI in drug discovery. By producing synthetic data sets, Generative AI can help overcome data limitations and accelerate the drug development process.
Recommended read: Generative Ai Software Development
Limited Model Interpretability
Limited Model Interpretability is a significant challenge in Generative AI for drug discovery. It's difficult to understand how AI models make decisions, especially in medical fields where knowing the 'why' behind a choice is crucial.
The lack of transparency can make it hard to trust and deploy AI in healthcare settings. This is a major concern in drug discovery where accuracy and reliability are paramount.
Generative AI models are often complex and hard to decipher, making it challenging to understand the rationale behind AI-generated molecules. This is a pressing issue in the field.
Understanding the decision-making process of AI models is essential for guiding experimental validation and decision-making. However, this is a significant hurdle that needs to be overcome.
Fortunately, researchers are actively working on enhancing the interpretability and explainability of generative models. This will be a crucial step in realizing the full potential of Generative AI in drug discovery.
Future Prospects and Directions of Generative AI in Drug Discovery
The future of generative AI in drug discovery is looking incredibly promising. With continual advancements in AI technology, the potential for developing novel medications is growing.
Continuous research and collaboration between AI specialists and biochemists are essential to overcoming the hurdles that generative AI currently confronts. This partnership is critical for realizing its full promise in drug discovery.
As Generative AI continues to advance, several exciting opportunities emerge on the horizon. The significance of generative AI in drug discovery is expected to grow even more crucial in the future.
Additional reading: Generative Ai and the Future of Work in America
Sources
- https://www.veritis.com/blog/from-concept-to-cure-generative-ai-in-drug-discovery/
- https://saturncloud.io/glossary/generative-ai-in-drug-discovery/
- https://data-science-ua.com/blog/what-is-the-role-of-generative-ai-in-drug-discovery/
- https://www.ditstek.com/blog/role-of-generative-ai-in-drug-discovery
- https://www.zealousys.com/blog/role-of-generative-ai-in-drug-discovery/
Featured Images: pexels.com