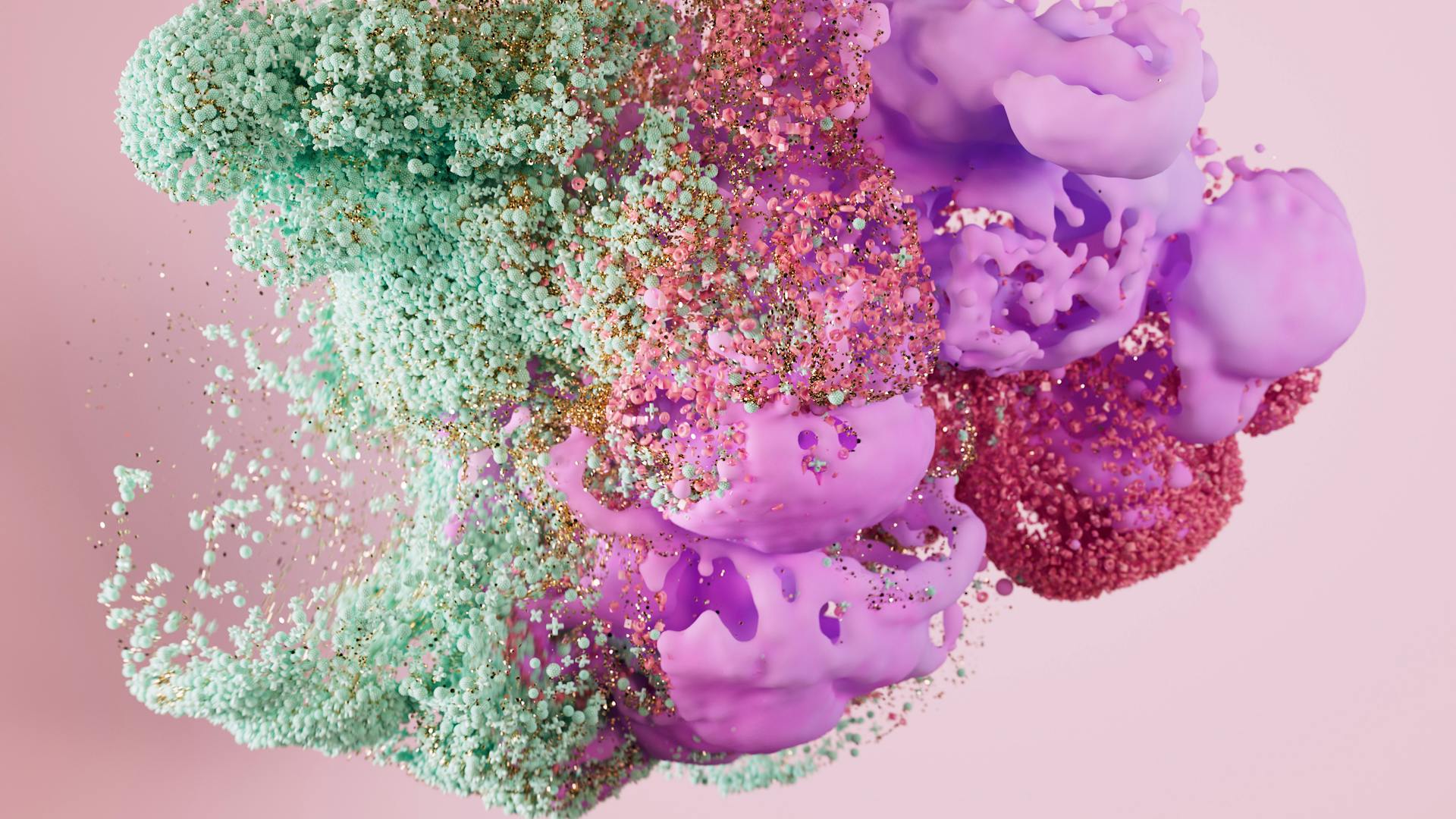
Synthetic data generation with generative AI is a game-changer for businesses and organizations. It allows them to create realistic and varied data without the need for manual input or expensive data collection.
Generative AI models can generate synthetic data that mimics real-world data, making it an ideal solution for testing and training machine learning models. This approach can reduce costs and increase efficiency.
One of the key benefits of synthetic data generation is that it can be used to augment existing datasets, making them more diverse and representative. This can lead to more accurate and reliable machine learning models.
With generative AI, businesses can create synthetic data that meets specific requirements, such as data quality, quantity, and format. This level of customization is not possible with traditional data collection methods.
You might like: Gen Ai vs Ml
What Is Synthetic Data Generation?
Synthetic data generation is a powerful tool that uses deep learning algorithms to create fake data that closely mirrors the patterns and distributions of real data. This approach is especially useful when real data is inaccessible due to compliance and privacy requirements.
You can generate synthetic data to replace real data, and it's often used in enterprises where the real data can't be changed or accessed. Synthetic data aims to replicate authentic data by reconstructing its statistical characteristics.
Synthetic data generators can produce any amount of data that closely mirrors the patterns, distributions, and interconnections observed in the real dataset. This is made possible by training the generator on genuine data.
Synthetic data not only allows the generation of analogous data but also enables the imposing of specific constraints on the data as necessary.
Types of Synthetic Data
When generating synthetic data, it's essential to understand the types of synthetic data available to address a business issue.
There are two primary types of synthetic data: fully synthetic and partially synthetic.
Fully synthetic data is unrelated to actual or real-world data, making it unidentifiable.
Partially synthetic data retains all the original data except for sensitive information, which is removed to ensure confidentiality.
Use Cases
Synthetic data is revolutionizing industries with its numerous benefits. It's customizable to meet a business's unique requirements.
In the automotive industry, synthetic data can be produced to satisfy specific needs, such as creating datasets for vehicle collision analysis. This is more cost-effective than collecting actual data, which can be expensive and time-consuming.
With the right hardware and tools, synthetic data can be created and assembled much faster than real-world data. This means a vast amount of synthetic data can be made available quickly.
Synthetic data preserves data privacy by not containing any identifiable information about the original data. This makes it anonymous and suitable for distribution.
Synthetic data can be used in various concepts like data augmentation, diffusion models, and many others. It's being explored in different industries, from technology to healthcare.
In the future, we can expect even more exciting applications of synthetic data and Generative AI.
Security and Ethics
Synthetic data generation with generative AI raises important questions about security and ethics.
Security concerns are a major obstacle to data flow within a company, particularly when dealing with personally identifiable information (PII). This is why synthetic data generation is necessary to protect sensitive details.
One of the primary challenges with synthetic data is ensuring its accuracy and resemblance to real-world data, which is crucial in healthcare where data quality can be a matter of life and death.
Synthetic data can be misused for malicious purposes, such as creating deepfakes or spreading misinformation, which is a serious concern in sensitive domains.
Here are some key ethical implications of using synthetic data:
- Privacy: Synthetic data can protect individuals’ privacy by avoiding using accurate personal data.
- Bias: Ensuring that synthetic data is generated without biases that could perpetuate discrimination or inequality.
- Misuse: Synthetic data can be misused for malicious purposes, such as creating deepfakes or spreading misinformation.
Security Concerns
Security Concerns can be a major hurdle when it comes to data sharing and collaboration.
Data flow within a company may be impeded by security concerns, such as sensitive information that can't be moved to a cloud infrastructure. This is especially true for personally identifiable information (PII), which requires extra protection.
Organizations protect sensitive details, so such information is not disclosed when data is collected. This creates a need for synthetic data generation.
Synthetic data can be generated using statistical models and algorithms, but the first question is – “Can we trust this synthetically generated data?”
Here's an interesting read: What Is a Best Practice When Using Generative Ai
Ethical Implications
Synthetic data can protect individuals' privacy by avoiding using accurate personal data. This is a significant advantage, especially in sensitive domains like healthcare, where data quality is paramount and privacy concerns are high.
The World Health Organization (WHO) has even issued caution against using AI to make healthcare decisions due to concerns about biased data leading to skewed models and algorithms. However, introducing synthetic data in these contexts can help alleviate these concerns.
Ensuring that synthetic data is generated without biases that could perpetuate discrimination or inequality is crucial. Research has shown that it's possible to extract specific information from the datasets used in training Large Language Models (LLMs), which raises questions about privacy and consent.
Synthetic data can be misused for malicious purposes, such as creating deepfakes or spreading misinformation. A report by McKinsey & Company highlighted the ethical concerns surrounding using synthetic data, emphasizing the need for responsible development and deployment.
Take a look at this: Applications of Generative Ai in Healthcare
Here are some key ethical implications of using synthetic data:
- Privacy: Synthetic data can protect individuals' privacy by avoiding using accurate personal data.
- Bias: Ensuring that synthetic data is generated without biases that could perpetuate discrimination or inequality.
- Misuse: Synthetic data can be misused for malicious purposes, such as creating deepfakes or spreading misinformation.
Ultimately, addressing the ethical concerns surrounding synthetic data requires a careful balance between leveraging the benefits of AI and respecting the confidentiality and privacy of individuals.
Industry-Specific Applications
Synthetic data generation with generative AI has numerous industry-specific applications. In the healthcare sector, synthetic data is used to build models and test datasets for illnesses with limited real data, while maintaining patient privacy. This approach enables the creation of extensive datasets for research.
Healthcare organizations can generate synthetic medical records or claims to support research without breaching sensitive patient confidentiality. Researchers can also use Generative AI to create synthetic medical images, such as CT/MRI scans, that are essential for training AI algorithms.
In the financial sector, synthetic data is used to identify and prevent financial fraud through predictive analysis. Companies like J.P. Morgan carry out research and build algorithms to provide realistic synthetic datasets to expedite the development of financial AI research.
Recommended read: Generative Ai Use Cases in Healthcare
Here are some key applications of synthetic data in various industries:
- Healthcare: Generating synthetic medical records, images, and claims to support research.
- Financial Services: Anonymizing sensitive client information and augmenting limited fraud detection datasets.
- Gaming: Crafting lifelike environments and characters to enhance the gaming experience.
Synthetic data has also shown potential in improving the accuracy of drug discovery models by 15% and fraud detection models by 10-15%.
Media
Synthetic media is a game-changer in the industry, allowing for the creation of realistic images, audio, and videos that can be used in place of the original data without any issues. This technique can be used to expand the databases used to train machine learning algorithms, making it easier to generate synthetic videos and images.
To create realistic human faces, algorithms acquire characteristics from photos of actual people. This is particularly useful when video data is unavailable due to privacy concerns. Synthetic tools can help generate synthetic videos in these situations.
Synthetic data is also useful for training image recognition systems, allowing for the expansion of the quantity and diversity of datasets. Concepts like diffusion models, data augmentation, and others have been implemented using synthetic data.
Worth a look: How Multimodal Used in Generative Ai
Synthetic media has numerous applications, including gaming and financial services. In gaming, generative AI is used to craft lifelike environments and characters, enhancing the gaming experience. This innovation allows for the creation of immersive gaming worlds without requiring large teams of artists and designers.
In financial services, synthetic data is used for fraud detection and risk assessment. For example, synthetic financial transactions can be generated to train fraud detection models in broader scenarios, improving accuracy by 10-15%.
Intriguing read: Chatgpt Openai's Generative Ai Chatbot Can Be Used for
Applications
Synthetic data has numerous applications across various industries, including healthcare, finance, and product design. In healthcare, synthetic data is used to build models and test datasets for illnesses with limited real data, ensuring patient confidentiality.
Medical imaging AI models are trained with fake data to maintain patient privacy, and Generative AI is used to create synthetic medical images for training AI algorithms. This eliminates the need for real patient data, making it easier to create extensive datasets for research.
You might like: Generative Ai in Cyber Security
The banking sector uses synthetic data to identify and prevent financial fraud through predictive analysis. Companies like J.P. Morgan create realistic synthetic datasets to expedite financial AI research.
Synthetic data can also be used in product design to create standard benchmarks, allowing businesses to assess product performance in a controlled landscape.
Here are some examples of industry-specific applications of synthetic data:
* Healthcare:
+ Generating synthetic medical records or claims to support research
+ Creating synthetic medical images for training AI algorithms
+ Improving the accuracy of drug discovery models by 15% (Nature Communications study)
* Finance:
+ Identifying and preventing financial fraud through predictive analysis
+ Anonymizing sensitive client information for secure development and testing
+ Improving the accuracy of fraud detection models by 10-15% (JPMorgan Chase study)
* Product design:
+ Creating standard benchmarks for product performance
+ Assessing product performance in a controlled landscape
* Autonomous vehicles:
+ Training perception models with diverse driving scenarios
+ Testing autonomous systems with simulated rare or dangerous driving conditions
+ Demonstrating comparable performance to real-world data (Waymo study)
These examples illustrate the potential of synthetic data to transform industries and improve outcomes. By leveraging synthetic data, organizations can create more accurate models, improve decision-making, and reduce costs.
A unique perspective: Use Cases of Generative Ai
How It Works
Synthetic data generation with generative AI is a powerful tool that can create realistic data from scratch. It uses various techniques such as Generative Pre-trained Transformer (GPT) methodology, Generative Adversarial Networks (GANs), and Variational Auto-Encoders (VAEs) to generate synthetic data.
These models work by understanding and replicating patterns from the training data, making them valuable for augmenting tabular datasets and creating realistic tabular data for machine learning tasks. GANs, for instance, function on the interplay between "generator" and "discriminator" neural networks, where the generator produces synthetic data that mimics reality, while the discriminator distinguishes real data from synthetic data.
Here are the three main models used for synthetic data generation:
Synthetic data can be generated from scratch or built from an existing dataset, and it can be used for various purposes such as data augmentation and training machine learning models.
How Generative AI Works
Generative AI creates synthetic data using deep machine learning (ML) generative models such as Generative Pre-trained Transformer (GPT), Generative Adversarial Networks (GANs), and Variational Auto-Encoders (VAEs).
These models can generate synthetic data that mimics real-world data, making them valuable for augmenting tabular datasets. GPT-based synthetic data generation tools can replicate patterns from the training data, creating realistic tabular data for ML tasks.
GANs, on the other hand, function on the interplay between a "generator" and a "discriminator" neural network. The generator produces synthetic data that attempts to deceive the discriminator, resulting in a high-quality synthetic dataset.
VAEs employ an "encoder" and a "decoder" to summarize patterns and characteristics present in real-world data and transform that summary into a lifelike synthetic dataset.
Synthetic data can be generated using computer simulations or algorithms, and it can be built from an existing dataset. The newly created data and the original data are almost exactly the same, making it possible to produce synthetic data of any size at any time and anywhere.
Generative AI can also create synthetic text by training a model on a large volume of text data. This technique is known as large language models, and it has become increasingly effective in generating realistic-looking synthetic writing.
One type of neural network used for synthetic text generation is the GPT-3 technique, which was trained on an enormous volume of text data. This technique has been used to create extraordinarily effective natural language generation systems.
See what others are reading: Telltale Words Identify Generative Ai Text
Here are some of the key methods for generating synthetic data from large language models (LLMs):
Synthetic data can be used to support existing datasets, especially in cases where the data does not exist or is limited. By relying on synthetic data, data scientists can augment their existing datasets and improve the performance of their ML models.
Synthetic data can also be used in computer vision applications, such as image and video generation, object detection and tracking, and training AI models. A study by NVIDIA demonstrated that synthetic data can train computer vision models with comparable performance to real-world data.
Training with synthetic data generated from LLMs can be challenging due to inherent biases and hallucinations in the LLMs. To mitigate these issues, regularization techniques can be implemented to stabilize training with noisy datasets.
If this caught your attention, see: How Is Generative Ai Trained
Measuring Quality
Measuring the quality of synthetic data is crucial for its effectiveness in model training. It's often done through quantitative metrics, which provide a numerical score to evaluate the data.
There are three main perspectives to measure data quality: diversity, correctness, and naturalness. Diversity measures the difference between chunks of text in the generated instances, while correctness measures whether the data instance is related to the given label.
Automatic evaluation methods can be used to measure correctness, where a model is trained on the oracle training dataset and then applied to calculate the percentage of correctly predicted samples on the synthetic dataset.
Human evaluation is also used to measure naturalness, where human evaluators assess whether the generated text is fluent and similar to human-written texts by selecting a score from a given range.
A quality estimation module can be incorporated into the data generation pipeline to obtain high-quality synthetic data. This involves evaluating the first generated synthetic data using a task-specific model trained on oracle data, and then selecting the most influential samples as in-context examples to prompt GPT2-XL to generate new data.
Here are some key metrics to evaluate data quality:
- Diversity: measures the difference between chunks of text in the generated instances.
- Correctness: measures whether the data instance is related to the given label.
- Naturalness: measures whether the generated text is fluent and similar to human-written texts.
Using high-quality synthetic data can improve the accuracy of machine-learning models by 10-15%, as found in a Stanford University study.
The UI Guide
The UI Guide makes it easy to generate synthetic data. With a user-friendly interface, you can navigate through the steps and inputs required for structure data generation.
You can experiment with YData Fabric's UI interface by registering for the Community version, which is available today.
Sources
- Synthetic Data | Benefits, Types, Use Cases (solguruz.com)
- Unlocking the Potential of Generative AI in Synthetic Data ... (saxon.ai)
- Generative AI for Synthetic Data Generation: Methods ... (arxiv.org)
- YData Fabric (ydata.ai)
- TimeGAN (nips.cc)
- CWGAN-GP (cameronfabbri.github.io)
- synthetic data generation with Python see the documentation (ydata.ai)
- Synthetic Data Generation Using Generative AI (xcubelabs.com)
Featured Images: pexels.com