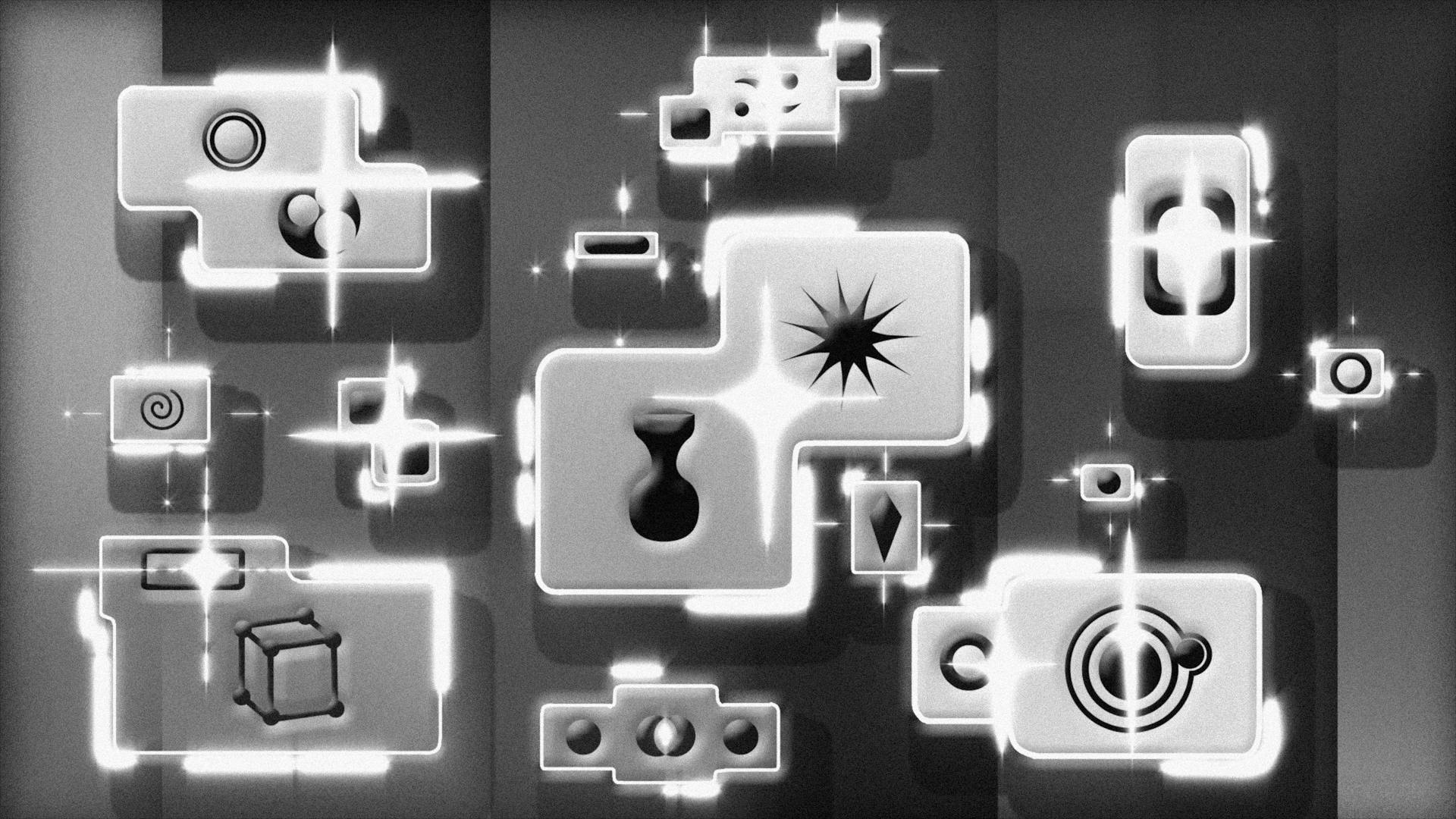
Generative AI in healthcare is revolutionizing the way we approach research and treatment. By analyzing vast amounts of medical data, generative AI can identify patterns and connections that humans might miss.
Generative AI models can generate new medical images, such as MRIs and CT scans, that are indistinguishable from real ones. This technology has the potential to improve diagnosis accuracy and reduce the risk of human error.
Generative AI is also being used to develop personalized treatment plans for patients. By analyzing a patient's unique genetic profile and medical history, generative AI can identify the most effective treatment options.
For example, generative AI has been used to develop personalized cancer treatment plans that have shown significant improvement in patient outcomes.
On a similar theme: Generative Ai and Cyber Security
Generative AI in Healthcare
Generative AI in Healthcare has the potential to revolutionize the way we approach patient care and medical research.
Synthetic data generation and data augmentation can create realistic and anonymized patient data for research and training purposes, addressing data privacy concerns and improving the accuracy and robustness of machine learning models.
By leveraging generative AI models, clinicians can automate and accelerate tasks such as data analysis, report generation, and medical diagnosis, freeing up time for more complex and high-value tasks.
Generative AI can analyze medical images like CT scans, MRIs, and X-rays with high accuracy, even outperforming human radiologists in some cases.
Post-Treatment Monitoring
Post-Treatment Monitoring is a crucial aspect of healthcare where generative AI can make a significant impact. By leveraging wearable technology, smart devices, and smartphones with sensors, AI can monitor patient behavior outside clinical settings.
With access to this extensive data, AI can provide real-time monitoring and personalized recommendations and interventions. This can help medical providers proactively address patient health deterioration by alerting them when immediate medical attention is necessary.
Non-adherence to post-treatment advice can diminish treatment effectiveness, negatively affecting patient health and potentially resulting in financial repercussions for providers. AI can help bridge this gap by ensuring patient compliance.
A key application of generative AI in post-treatment monitoring is the use of AI-generated reports for medical data, which have been shown to have the same level of quality and accuracy as those produced by human radiologists.
Revolutionizing Research
Generative AI is revolutionizing medical research by enabling the creation of synthetic data that mirrors real patient data. This capability is invaluable for research areas where data may be scarce or difficult to obtain, such as rare diseases.
Synthetic data can be used to simulate clinical trials, test hypotheses, and model disease progression without the ethical and privacy concerns associated with using actual patient data. This accelerates research and reduces costs.
Generative AI models like GANs can synthesise electronic health record (EHR) data by learning the underlying data distributions, allowing for excellent performance and addressing challenges such as data privacy concerns.
With synthetic data, researchers can investigate and test various hypotheses, leading to new insights and discoveries. This is particularly useful in situations where there is a limited amount of real-world patient data available.
John Snow Labs provides AI-driven platforms that accelerate the drug discovery process by leveraging their vast datasets and AI models. By using these platforms, pharmaceutical companies can identify promising compounds faster and more efficiently.
Consider reading: Generative Ai in Real Estate
The use of synthetic data can improve the accuracy and robustness of machine learning models, as it allows for a more diverse and representative range of data to be used in the training process. This is essential for developing effective treatments and therapies.
Generative AI models can also be used to generate synthetic medical images that can be used to train machine learning models for image-based diagnosis or augment medical datasets. This can create a more user-friendly and understandable system for patients compared to conventional CAD systems.
On a similar theme: How Multimodal Used in Generative Ai
Methods and Techniques
To integrate generative AI in healthcare, careful planning is essential. This involves considering factors such as data privacy and security.
The Technology Acceptance Model (TAM) and the Non-Adoption, Abandonment, Scale-up, Spread and Sustainability (NASSS) model are frameworks that can be used to promote responsible integration. These frameworks help anticipate and address barriers to adoption.
By using these frameworks, healthcare professionals can facilitate stakeholder participation and responsibly transition care systems to harness generative AI's potential.
Additional reading: Ai and Ml in Healthcare
Synthetic Generation and Augmentation
Synthetic data generation and augmentation are powerful tools in the field of artificial intelligence. They allow for the creation of realistic and anonymized patient data, which can be used for research and training purposes without compromising patient privacy.
Generative AI models like GANs are increasingly being used for this purpose, as they can learn the underlying data distributions and generate high-quality synthetic data. This approach is particularly useful when real-world patient data is limited or restricted due to privacy concerns.
Synthetic data can be used to improve the accuracy and robustness of machine learning models by providing a more diverse and representative range of data for training. It can also enable researchers to investigate and test various hypotheses, leading to new insights and discoveries.
GANs can synthesize electronic health record (EHR) data, allowing for excellent performance and addressing data privacy concerns. This is achieved through the adversarial training process, where the generator creates fake data to pass to the discriminator, which decides if the data is like the known, real data.
For more insights, see: Learn Generative Ai
The ability to generate synthetic data with different characteristics and parameters can be used to augment training data, helping in cases where the original dataset is small or imbalanced. This can improve the performance of machine learning models and enable the creation of more realistic and diverse samples.
Synthetic data retains the statistical relationships of real data while offering privacy protection, making it a valuable tool for medical research and development.
Suggestion: Synthetic Data Generative Ai
Workflow
Workflow is a crucial aspect of healthcare, and generative AI can significantly enhance it. Generative AI can tap into health information exchanges (HIEs) to retrieve patient medical records, analyze them, and formulate pertinent inquiries based on the patient’s medical background.
AI systems can assist healthcare providers with collecting medical histories of patients by posing specific questions in a conversational manner. This approach can aid in assembling a more comprehensive medical history for the patient, which can then be used by physicians to provide better care.
For another approach, see: Generative Ai Healthcare Use Cases
Generative AI can also help streamline administrative work by handling basic healthcare operations like booking appointments and reminding patients about their scheduled visits. This can save the hours human operators have to give in for handling an ever-increasing number of calls and messages in healthcare systems.
AI assistants can automate tasks like patient registration, routing, scheduling, FAQs, IT helpdesk ticketing, and prescription refills. For instance, Mercy Health, Baptist Health, and Intermountain Healthcare have launched bots for automating these tasks.
Generative AI can also be used to create personalized treatment and therapy plans based on the current conditions as well as previously recorded parameters, including genetic makeup, health history, and lifestyle.
Benefits and Use Cases
Generative AI has the potential to revolutionize medicine and healthcare by enhancing disease diagnosis, treatment personalization, and medical research. It can learn from complex datasets and produce novel, actionable intelligence.
Generative AI models can generate text and images, which are being used in various healthcare scenarios, including drug discovery, medical diagnosis, clinical documentation, patient education, and personalized medicine. These models have been used to create synthetic medical images for training purposes, helping to overcome data privacy and availability challenges.
Generative AI can also be used to analyze a patient's genetic makeup, lifestyle, and medical history to predict how they might respond to different treatments. This can lead to more effective treatment and improved patient outcomes.
Discover more: Chatgpt Openai Generative Ai Chatbot Can Be Used for
Real Use Cases
Generative AI is revolutionizing the healthcare industry, and its real-world use cases are impressive. Generative AI can transform healthcare by enabling improvements in diagnosis, reducing the cost and time required to deliver healthcare, and improving patient outcomes.
One prominent use case of Gen AI in healthcare is the implementation of Retrieval-Augmented Generation (RAG) on FHIR, which makes healthcare data more accessible by pairing RAG with FHIR. This approach, developed using John Snow Labs' language models, allows real-time retrieval of data based on specific questions.
Healthcare providers can use Generative AI models to segment high-risk patient groups using free-text prompts, improving the accuracy of patient treatment strategies. For instance, healthcare teams can retrieve information about patients at high risk of unplanned admissions based on medical conditions like chronic kidney disease.
Generative AI models can create synthetic medical images for training purposes, helping to overcome the challenges of data privacy and availability. This is particularly important for medical imaging, which is crucial for diagnosis and treatment planning.
For more insights, see: What Is Rag in Generative Ai
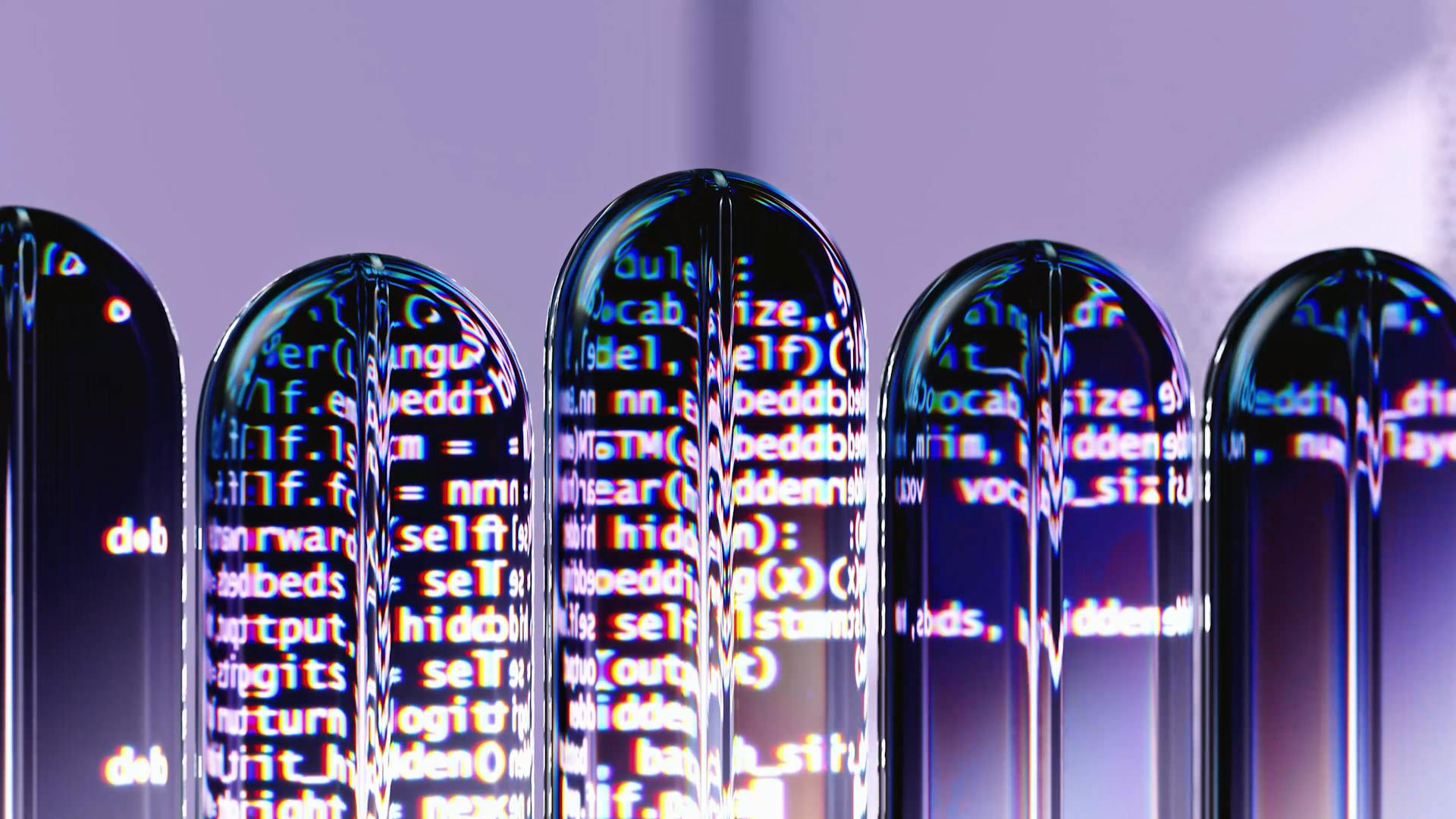
Generative AI can also be used for patient education, creating personalized educational content based on a patient's specific condition, symptoms, or questions. For example, if a patient has diabetes, the AI can generate information about managing blood sugar levels, diet, exercise, and medication.
John Snow Labs' AI solutions analyze vast amounts of data to create personalized treatment plans, ensuring that patients receive the most effective care tailored to their specific needs. This approach can lead to more effective treatment and improved patient outcomes.
By integrating insights from John Snow Labs and aligning with the recommendations of BCG and Brookings, healthcare providers can better navigate the challenges of deploying Gen AI in healthcare. These real-world use cases demonstrate the vast potential of Generative AI to revolutionize patient care, data management, and operational efficiency on a global scale.
A different take: Google Labs Generative Ai
Informed Consent
Informed consent is a crucial aspect of using AI in healthcare. Patients must be thoroughly informed about the role AI plays in their healthcare journey.
Openness in patient communications is vital, and patients need to understand the privacy implications of using AI-driven tools. This includes data collection from wearable devices and smart technology.
Patients should be educated on the utilization of their data, the privacy safeguards in place, and the benefits and risks associated with AI in healthcare. This education is a foundational element in fortifying trust between patients and the evolving healthcare system.
Challenges and Limitations
Deploying generative AI in healthcare is not without its challenges, which include issues related to interpretability, data requirements, transparency, ethics, risk, and bias.
Risk concerns and considerations top the list of scale-up challenges faced by healthcare organizations, largely due to the untested nature of the technology and uncertainty around regulations.
Healthcare leaders have begun pursuing plans to more broadly adopt generative AI, but they face challenges such as insufficient capability, data and tech infrastructure, and proof of value.
See what others are reading: What Challenges Does Generative Ai Face
Bias and Fairness
Bias and Fairness is a critical challenge in deploying Generative AI in healthcare.
AI systems can inadvertently learn and perpetuate biases present in their training data, leading to unfair or biased outcomes in patient care. This is a major concern, as it can affect the quality of care and trust in AI-driven decisions.
John Snow Labs addresses this challenge by using diverse and comprehensive datasets, along with bias detection and mitigation tools. Their commitment to diversity in data collection and algorithmic transparency is key to minimizing bias.
Bias can stem from various sources, including data collection methods and algorithmic design. It's essential to be aware of these potential sources and take steps to mitigate them.
John Snow Labs Solution: Through diverse and comprehensive datasets, along with bias detection and mitigation tools, John Snow Labs helps healthcare organizations develop AI models that are fair and equitable.
Consider reading: One Challenge in Ensuring Fairness in Generative Ai
Privacy and Security
Data privacy and security are significant concerns in healthcare due to the sensitive nature of the data and the need for large datasets to train AI models.
Healthcare data must comply with strict regulations like HIPAA in the United States, which adds to the complexity of handling and managing risk.
Gen AI addresses data scarcity and privacy concerns by generating high-quality, synthetic datasets that can supplement real data.
This approach not only expands the scope of research but also enhances the training of AI models, leading to more robust and accurate healthcare solutions.
John Snow Labs ensures that data platforms and AI models are built with privacy-by-design principles, offering robust encryption and anonymization techniques.
Their secure data handling and compliance with healthcare data regulations make it easier for enterprises to manage risk and provide peace of mind in a sensitive field.
A fresh viewpoint: Generative Ai Regulations
Scaling Hurdles
Scaling hurdles in healthcare technology adoption can be a significant challenge. Risk concerns and considerations top the list of scale-up challenges faced by surveyed leaders, regardless of whether they work at a payer, provider, or HST company.
Investing in the untested technology of gen AI requires significant investment to build capabilities. Uncertainty around regulations also plays a major role in the hesitation to adopt gen AI.
See what others are reading: Roundhill Generative Ai & Technology Etf
Insufficient capability, data, and tech infrastructure are also major roadblocks to deploying gen AI solutions. This highlights the limited tech readiness of healthcare organizations to deploy and validate gen AI capabilities.
Strategic partnerships have enabled healthcare leaders to pursue plans to more broadly adopt gen AI technology. Cross-functional collaborations allow organizations to bring in outside talent and build flexible and customizable gen AI solutions.
Buying publicly available gen AI products may offer a viable alternative to tapping into the technology's value proposition, particularly for functional uses that have matured faster, such as customer service applications.
You might enjoy: Generative Ai Solutions Stock
Deployment and Adoption
To successfully deploy generative AI in healthcare, enterprises must start with a clear strategic plan that outlines the objectives for incorporating Gen-AI into their operations. This includes identifying specific healthcare challenges to address, such as improving diagnosis accuracy, personalizing patient care, or streamlining drug discovery.
A solid grasp of AI promotes continuous learning and adaptation to the evolving landscape of AI technology, making it crucial to invest in improving awareness for all partners. Healthcare professionals need to understand and accept the applications of AI to foster trust in AI systems and enable their effective use.
Broaden your view: How Are Modern Generative Ai Systems Improving User Interaction
Perceived usefulness and ease of use are key factors in determining whether healthcare professionals will adopt generative AI. If they perceive the AI system as useful and easy to use, they are likely to develop a positive attitude towards using it.
The value proposition of generative AI in healthcare is compelling, offering benefits like cost-effectiveness, speed, and personalized treatment options. If healthcare professionals perceive the AI system as useful and easy to use, they are likely to develop a positive attitude towards using it.
To encourage actual use of the AI system, opportunities to use it in a safe and supportive environment should be provided, and the AI system should be integrated into existing workflows.
Here are the key factors to consider for successful deployment and adoption:
- Strategic planning and investment
- Successful integration into existing healthcare systems and workflows
- Leveraging big data for analysis and insights
- Scaling Gen AI solutions across different departments, healthcare settings, and patient populations
By following these key factors, enterprises can ensure successful deployment and adoption of generative AI in healthcare.
Frequently Asked Questions
What are generative AI examples?
Generative AI examples include creating new text, images, music, audio, and videos. These can range from generating art and music to producing personalized content like chatbots and virtual assistants.
What is generative AI in drug discovery?
Gen AI in drug discovery uses algorithms to identify new uses for existing drugs, accelerating the development of treatments for new indications
Sources
- https://implementationscience.biomedcentral.com/articles/10.1186/s13012-024-01357-9
- https://www.brookings.edu/articles/generative-ai-in-health-care-opportunities-challenges-and-policy/
- https://www.mckinsey.com/industries/healthcare/our-insights/generative-ai-in-healthcare-adoption-trends-and-whats-next
- https://www.forbes.com/sites/shashankagarwal/2024/05/25/generative-ai-the-new-lifeline-to-overwhelmed-healthcare-systems/
- https://www.johnsnowlabs.com/generative-ai-healthcare/
Featured Images: pexels.com