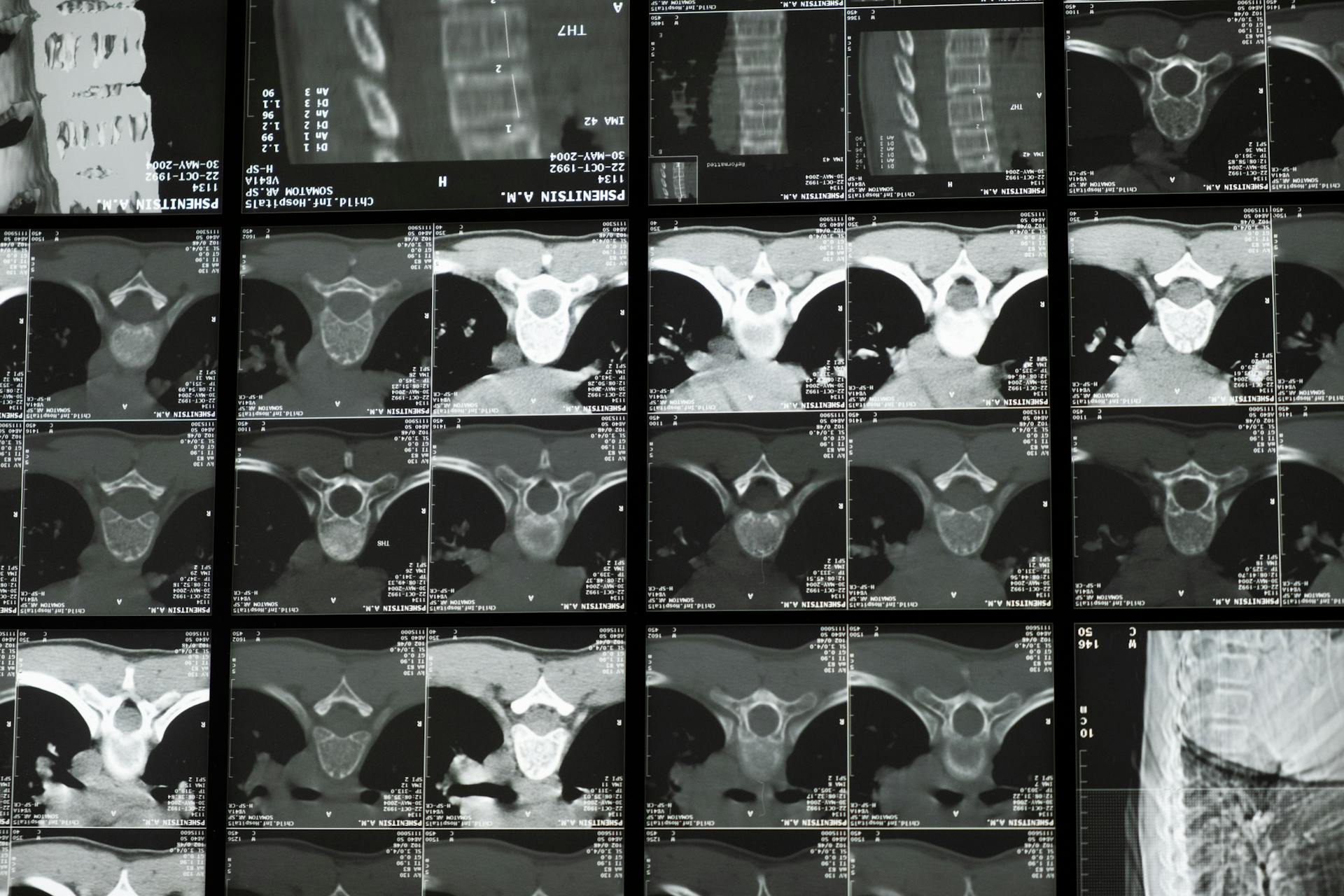
AI and ML in Healthcare are revolutionizing the way medical professionals diagnose and treat patients. They're helping to improve patient outcomes, reduce costs, and enhance the overall quality of care.
With AI-powered tools, doctors can analyze large amounts of medical data to identify patterns and make more accurate diagnoses. For example, AI-assisted diagnosis can detect breast cancer from mammography images with a high degree of accuracy.
The benefits of AI and ML in healthcare are numerous, but one of the most significant advantages is the ability to personalize treatment plans for individual patients. By analyzing a patient's unique medical history and genetic profile, doctors can create tailored treatment plans that are more effective and less likely to cause side effects.
AI and ML can also help reduce medical errors by automating routine tasks and providing real-time alerts to doctors when a patient's condition is deteriorating. This can lead to faster and more effective treatment, and ultimately, better patient outcomes.
Intriguing read: Google Announces New Generative Ai Search Capabilities for Doctors
Current State
Currently, AI systems in healthcare are not yet able to reason like human physicians, who can draw upon 'common sense' or 'clinical intuition and experience'.
They can, however, translate patterns from datasets, which is a key area of progress in AI adoption.
AI systems are being adopted by healthcare organisations to automate time-consuming, high-volume repetitive tasks.
Precision diagnostics, such as diabetic retinopathy and radiotherapy planning, are also areas where AI is making significant strides.
Additional reading: Machine Learning Facial Recognition Security and Surveillance Systems
Use Cases
AI in healthcare has the potential to revolutionize the industry, and its use cases are numerous. AI can enable healthcare systems to achieve the 'quadruple aim' by democratising and standardising connected and AI-augmented care.
AI is being used in various areas, including improving medical diagnosis, speeding up drug discovery, transforming patient experience, managing healthcare data, and performing robotic surgery. These applications have the potential to significantly improve healthcare outcomes and patient care.
Some specific examples of AI use in healthcare include:
- Improving medical diagnosis
- Speeding up drug discovery
- Transforming patient experience
- Managing healthcare data
- Performing robotic surgery
In the area of infectious diseases, AI has shown potential in early detection, tracking virus spread, and analysing virus behaviour, among other things. AI has also been used to identify antimicrobial resistance, detect malaria, and improve point-of-care testing of Lyme disease.
What Are the Use Cases?
In healthcare, AI has numerous use cases that can improve patient outcomes and streamline clinical workflows. AI can assist clinicians with data processing capabilities to save time and improve accuracy in medical diagnosis.
AI is being explored for its potential in improving medical diagnostic approaches, particularly in areas such as disease diagnosis and early prediction of diseases like Alzheimer's and dementias. Recent developments in statistical physics, machine learning, and inference algorithms are also being explored for their potential in improving medical diagnostic approaches.
AI can aid doctors in patient diagnosis through the analysis of mass electronic health records (EHRs) and help early prediction of diseases. AI can also support doctors' decision-making in urgent situations, such as in the emergency department, by prioritizing more serious cases and reducing waiting time.
One notable example of AI's potential in healthcare is its ability to assist with dermatology. Machine learning has been shown to be effective in processing images for better diagnoses, with AI algorithms achieving high accuracy in identifying skin cancer from lesion images.
A fresh viewpoint: Applications of Machine Learning in Manufacturing
AI can also be used to automatically evaluate the outcome of maxillo-facial surgery or cleft palate therapy in regard to facial attractiveness or age appearance. Additionally, AI can be applied to the remote monitoring of patients through wearables and sensors to identify and provide timely care of patients at risk of deterioration.
AI has the potential to transform patient experience, improve patient flow and experience, and enhance caregiver experience and patient safety through the care pathway. AI can also be used to improve medication management, perform robotic surgery, and manage healthcare data.
Here are some specific use cases of AI in healthcare:
- Improving medical diagnosis
- Speeding up drug discovery
- Transforming patient experience
- Managing healthcare data
- Performing robotic surgery
Gastroenterology
In gastroenterology, AI can significantly enhance endoscopic procedures like EGDs and colonoscopies by rapidly identifying abnormal tissue.
Early trials have shown AI detection systems can identify stomach cancer with sensitivity close to expert endoscopists.
AI can also assist doctors treating ulcerative colitis by analyzing digitized bowel samples, known as biopsies.
These AI-powered tools can distinguish between samples showing remission and those with active disease with 80% accuracy.
They can also predict the risk of a flare-up happening with the same accuracy as pathologists.
Obstetrics and Gynaecology
In obstetrics, artificial intelligence is being used in various ways to improve patient care.
Artificial intelligence utilises massive amounts of data to help with predicting illness, prevention, and diagnosis.
In obstetrics, AI is used in magnetic resonance imaging, ultrasound, and foetal cardiotocography.
AI contributes in the resolution of a variety of obstetrical diagnostic issues.
Discover more: Glossary Artificial Intelligence
Infectious Diseases
AI has shown potential in both laboratory and clinical spheres of infectious disease medicine, particularly during the COVID-19 pandemic.
AI was used for early detection, tracking virus spread, and analysing virus behaviour during the pandemic.
In clinical practice, AI was used in a few instances, such as identifying antimicrobial resistance with support-vector machines.
Machine learning analysis of blood smears can detect malaria, improving diagnosis and treatment outcomes.
AI has been investigated for improving diagnosis of meningitis, sepsis, and tuberculosis, as well as predicting treatment complications in hepatitis B and hepatitis C patients.
Point-of-care testing of Lyme disease based on antigen detection has been improved with AI, making it easier to diagnose and treat the disease.
Recommended read: How Does Ai Detection Software Work
Musculoskeletal
Musculoskeletal conditions like knee pain affect underserved populations more severely. They experience higher levels of pain that persists even after controlling for the objective severity of diseases like osteoarthritis.
AI has been used to identify causes of knee pain that doctors miss. These causes disproportionately affect Black patients.
Standard radiographic measures of severity often overlook objective but undiagnosed features. This can lead to disparities in diagnosis and management of underserved populations with knee pain.
A machine-learning algorithm has shown that ALG-P, a new algorithmic measure, could potentially enable expanded access to treatments for underserved patients.
Precision Medicine
Precision medicine is a game-changer in healthcare, and AI is playing a significant role in making it a reality. AI can help improve our understanding of disease by analyzing large amounts of data, including multimodal datasets that can lead to digital and biological biomarkers for diagnosis, severity, and progression.
This can lead to more accurate diagnoses and targeted treatments, which is crucial for patients who need timely and effective care. For example, researchers globally are exploring the cellular and molecular basis of disease, collecting datasets that can help develop biomarkers for diagnosis and progression.
AI can also help alleviate the global shortages of physicians and increase accessibility to modern imaging and diagnostic tools, particularly in areas where they are scarce.
Precision Medicine
Precision medicine is revolutionizing the way we approach healthcare. It's a personalized approach to treatment that takes into account an individual's unique genetic, molecular, and environmental characteristics.
By leveraging AI and machine learning, researchers are making significant strides in understanding the cellular and molecular basis of disease. This is leading to the development of digital and biological biomarkers for diagnosis, severity, and progression.
AI-assisted pathology tools are being developed to assist with the diagnosis of various diseases, including breast cancer, hepatitis B, gastric cancer, and colorectal cancer. These tools have shown accuracy similar to that of human pathologists.
The use of AI in precision medicine is also being explored in the field of oncology. AI has been trialed in cancer diagnostics with the reading of imaging studies and pathology slides. In fact, an AI algorithm developed by the University of Pittsburgh achieved the highest accuracy to date in identifying prostate cancer.
See what others are reading: Informatic Tools
With the help of AI, researchers are able to analyze large-scale screening samples more efficiently, lessening the burden on pathologists and allowing for faster turnaround of sample analysis. This can save millions of dollars in costs, as seen in a university center that implemented digital pathology and saved over $12 million over five years.
Psychiatry
In psychiatry, AI applications are still in a proof-of-concept phase.
Predictive modelling of diagnosis and treatment outcomes is an area where evidence is quickly widening, with chatbots and conversational agents being studied for anxiety and depression.
These applications outside the healthcare system raise various professional, ethical, and regulatory questions, such as the screening for suicidal ideation implemented by Facebook in 2017.
Small training datasets contain bias that is inherited by the models, compromising their generalizability and stability.
The National Eating Disorders Association had to take its chatbot offline in 2023 after users reported receiving harmful advice from it.
AI models may also have the potential to be discriminatory against minority groups that are underrepresented in samples.
For more insights, see: Applications of Ai and Ml
Technologies
The AI healthcare market can be divided into software, hardware, and services, with software having the largest share and fastest growth. This segment includes Machine Learning platforms, Natural Language Processing (NLP) and text analysis tools, Deep Learning Platforms, Computer Vision, Speech and Audio Recognition, Integrated Development environments (IDEs) and AI frameworks.
Machine Learning is currently the leader in AI healthcare technology, but Natural Language Processing (NLP) has the potential to take over as the market leader in the future. The demand for analyzing human language data will soon surpass the demand for images and contextual information.
The top AI technologies in healthcare are Machine Learning, Natural Language Processing (NLP), Computer Vision, and Context-Aware Computing. Here are some key statistics on each:
Virtual Assistants
Virtual assistants and AI chatbots are revolutionizing the way we interact with healthcare. AI chatbots like Babylon and Ada are being used by patients to identify symptoms and recommend further actions.
These chatbots can be integrated with wearable devices such as smartwatches to provide insights to both patients and caregivers. This integration enables patients to track their behavior, sleep, and wellness more effectively.
AI chatbots can help patients identify potential health issues early on, reducing the need for hospital visits and improving overall health outcomes. By leveraging AI technology, patients can take a more proactive approach to their healthcare.
Here are some examples of AI chatbots being used in healthcare:
By incorporating AI chatbots into our healthcare routine, we can improve our overall health and well-being.
Immunomics and Synthetic
Immunomics and synthetic biology are revolutionizing the way we approach healthcare. Through the application of AI tools on multimodal datasets, we can better understand the cellular basis of disease.
This technology has the potential to cluster diseases and patient populations, allowing for more targeted preventive strategies. AI tools can diagnose and predict care and treatment options using immunomics.
The impact will be significant, particularly in the cancer, neurological, and rare disease space. Personalized care will become a reality, tailoring the experience of care to the individual.
Market Segmentation by Technology
The AI healthcare market is segmented by technology into several key areas. Machine Learning is currently the leader, but Natural Language Processing (NLP) has the potential to be the market leader in the future.
The software segment has the largest share and is expected to have the fastest growth. It includes Machine Learning platforms, NLP and text analysis tools, Deep Learning Platforms, Computer Vision, Speech and Audio Recognition, Integrated Development environments (IDEs) and AI frameworks.
The AI in healthcare statistics shows that the demand for analyzing human language data will soon be more than for images and contextual information. This is because NLP has a wide range of applications in healthcare, from medical text analysis to patient communication.
Here's a breakdown of the AI healthcare market segmentation by technology:
This segmentation highlights the importance of NLP in the future of AI healthcare, and the need for companies to invest in this area to stay ahead of the competition.
Robotic Surgery
Robotic surgery is becoming increasingly precise thanks to AI-powered robots. These robots can ensure absolute accuracy in complicated surgeries such as cardiovascular or neurology procedures.
Surgeons can control a robot's mechanical arms while seated at a computer console, giving them a three-dimensional, magnified view of the surgical site. This allows them to lead other team members through the entire operation.
Robot-assisted surgeries have led to fewer surgery-related complications, less pain, and a quicker recovery time. The power of AI in robotic surgery is undeniable.
The FDA has approved 391 devices using AI in radiology, which is 75% of all FDA-cleared devices. This shows the significant role AI is playing in radiological imaging.
AI integrates data from IoT devices and sensors into a single healthcare system, allowing for the gathering, exchange, and analysis of huge amounts of data.
Related reading: Data Science vs Ai vs Ml
Data Management
Data management is a crucial aspect of healthcare, and AI is revolutionizing the way data is handled. AI can break down data silos and connect information in minutes that used to take years to process.
Suggestion: Ai and Ml in Data Analytics
The sheer volume of data in healthcare is staggering, with trillions of data points to sift through. This can lead to valuable information getting lost, slowing down the development of new drugs and treatments.
AI can help reduce the time and costs of healthcare administrative processes, contributing to more efficient daily operations and patient experiences. By connecting the dots between data points, AI can provide insights that would be impossible to uncover manually.
One of the biggest challenges in healthcare data management is patient privacy. A survey in the UK found that 63% of the population is uncomfortable with sharing their personal data to improve AI technology.
The lack of current regulations surrounding AI in the US has raised concerns about mismanagement of patient data. For example, a Swiss healthcare company, Roche, was found to have purchased healthcare data for approximately 2 million cancer patients at an estimated total cost of $1.9 billion.
The potential of AI in healthcare is hindered by concerns about data management, especially in the US. A survey by the Pew Research Center in 2022 found that 37% of Americans were more concerned than excited about the increased presence of AI in their daily lives.
Here are some of the top segments dominating the AI healthcare market by application:
- Robot-Assisted Surgery: AI-powered robots make surgical procedures more precise.
- Clinical Trials: AI algorithms are changing clinical trials processes, making them more accurate, cost-effective, and patient-centric.
- Connected Machines: AI integrates data from IoT devices and sensors into a single healthcare system, giving an opportunity to gather, exchange, and analyze huge amounts of data.
The FDA-approved list of AI-enabled applications shows that radiology has become a promising area, with 75% or 391 FDA-cleared devices. This is a testament to the potential of AI in healthcare data management.
Challenges and Limitations
Data quality and access are significant challenges in adopting AI in healthcare systems.
Inaccurate patient demographics in data sets can lead to AI making biased decisions that discriminate against minorities.
Technical infrastructure, organisational capacity, and ethical and responsible practices also pose challenges.
AI's decisions are a direct reflection of its input data, making it crucial to have accurate representation of patient demographics.
White males are overly represented in medical data sets, which can lead to AI making more accurate predictions for majority populations.
This can result in unintended worse medical outcomes for minority populations.
Careful implementation and methodical collection of representative data can help eliminate biases.
You might like: Automated Decision-making
Challenges
The adoption of AI in healthcare systems is not without its challenges. Significant technical infrastructure issues must be addressed in order for AI to be successfully implemented.
Data quality and access are major concerns, as poor data can lead to inaccurate AI decision-making. This can have serious consequences in healthcare settings.
Organisational capacity is also a significant challenge, as healthcare systems must be able to adapt to the changing needs of AI implementation.
Aspects related to safety and regulation must be carefully considered to ensure the safe and effective use of AI in healthcare.
Bias
Bias is a significant challenge in AI, particularly in healthcare settings. AI makes decisions solely based on the data it receives, which can be problematic if the data is not representative of the patient demographics.
Patient demographics are not always accurately represented in medical data sets, leading to unfair coding of algorithms that can discriminate against minorities. This can result in prioritizing profits over optimal care.
White males are often overrepresented in medical data sets, while minority groups are underrepresented. This can lead to AI making more accurate predictions for majority populations, resulting in worse medical outcomes for minority populations.
Collecting data from minority communities can also lead to medical discrimination, such as using HIV status to discriminate against patients. Different clinical systems and clinician work practices can also introduce biases into AI functionality.
These biases can be eliminated through careful implementation and a methodical collection of representative data. However, biases can also arise from "label choice bias", where proxy measures are used to train algorithms that build in bias against certain groups.
For example, an algorithm predicted healthcare costs as a proxy for healthcare needs, which introduced bias because Black patients have lower costs, even when they are just as unhealthy as White patients.
Consider reading: Ai Medical Coding Software
Error Prevention in Healthcare
Error prevention in healthcare is a pressing concern, with 2% of hospitalized patients experiencing serious preventable medication-related incidents that can be life-threatening, cause permanent harm, or result in death.
These incidents are often the result of human error, but research suggests that machine learning can play a crucial role in preventing them. Our AI model was able to anticipate physician's actual prescribing decisions 75% of the time, based on de-identified electronic health records and the doctor's prescribing records.
This is a significant step towards testing the hypothesis that machine learning can support clinicians in ways that prevent mistakes and help to keep patients safe.
A different take: Machine Learning for Healthcare Applications
Companies and Research
Companies like DeepMind are leading the way in healthcare innovation by applying AI technology. Their breast cancer screening tool is just one example of how AI can be used to improve patient outcomes.
DeepMind, a Google daughter company, is also working on radiological solutions for eye disease detection. This is just one of many companies that are using AI to make a positive impact in healthcare.
Augmedix, another innovative company, has developed an AI-based solution to extract data from doctor-patient conversations, convert it into text notes, and insert it into the EHR system. This helps streamline the documentation process and saves time for healthcare professionals.
Here are some of the top AI healthcare companies:
- DeepMind (US)
- Augmedix (US)
- CloudMedX Health (US)
- Babylon Health (UK)
- Corti (Denmark)
- Butterfly Network (US)
- Enlitic (US)
- Arterys (US)
- Caption Health (US)
- Behold.ai (UK)
Companies
Companies like EliseAI, Cohere Health, Pfizer, Butterfly Network, and Novo Nordisk are already using AI in healthcare to innovate and improve patient care.
Some of these companies are developing tools to help doctors detect diseases earlier, such as EliseAI's breast cancer screening tool, and Butterfly Network's portable ultrasound imaging system.
DeepMind, a US-based company, is a daughter of Google and is famous for its breast cancer screening tool and radiological solutions for eye disease detection.
Augmedix is a medical documentation expert that uses AI to extract data from doctor-patient conversations and convert it into text notes, which are then inserted into the EHR system.
CloudMedX Health uses NLP and Deep Learning to analyze data from EMRs and clinical notes, giving clinicians insights about a patient's health state and new approaches to disease treatment.
Here are some top AI healthcare companies:
- DeepMind (US)
- Augmedix (US)
- CloudMedX Health (US)
- Babylon Health (UK)
- Corti (Denmark)
- Butterfly Network (US)
- Enlitic (US)
- Arterys (US)
- Caption Health (US)
- Behold.ai (UK)
United Nations (WHO/ITU)
The United Nations is playing a significant role in advancing the use of AI in healthcare. The ITU-WHO AI for Health Framework is a platform built by the joint ITU-WHO Focus Group on Artificial Intelligence for Health (FG-AI4H) for testing and benchmarking AI applications in the health domain.
Eight use cases are currently being benchmarked on this platform, including assessing breast cancer risk from histopathological imagery.
Discover more: Generative Ai Healthcare Use Cases
Applications
AI in healthcare is being used in various applications to improve patient care and outcomes. Robot-assisted surgery is one area where AI is making a significant impact, allowing for more precise procedures.
In clinical trials, AI is being used to make them more accurate, cost-effective, and patient-centric through rapid data analysis. This is particularly useful in complicated surgeries like cardiovascular or neurology procedures.
Connected machines in healthcare are also being integrated with AI to gather, exchange, and analyze large amounts of data. This has the potential to improve patient care and outcomes.
Radiology has seen a significant increase in the use of AI, with 75% of FDA-cleared devices being used in this field. This is due to the potential of AI to enhance radiological imaging and improve diagnosis rates.
AI is also being used in primary care to support decision-making, predictive modeling, and business analytics. This has the potential to improve treatment choices and patient outcomes.
Ambient Care
Ambient care is a game-changer in the healthcare industry. It enables remote monitoring of patients without the need for any peripherals.
For instance, the Emerald platform uses wireless, touchless sensors and machine learning to monitor sleep, breathing, and behavior. This can be especially helpful for patients who have difficulty with traditional monitoring methods.
Google Nest is another example of ambient care, claiming to monitor sleep and sleep disturbances like cough using motion and sound sensors. This technology has the potential to improve patient care and outcomes.
Ambient clinical intelligence is also on the rise, with AI systems leveraging natural language processing to automate administrative tasks. This can free up clinicians to focus more time on caring for patients.
Here are some examples of ambient care technologies:
- Emerald (www.emeraldinno.com): a wireless, touchless sensor and machine learning platform for remote monitoring of sleep, breathing and behaviour.
- Google Nest: claiming to monitor sleep (including sleep disturbances like cough) using motion and sound sensors.
- Nuance Dragon Ambient eXperience (www.nuance.com/healthcare/ambient-clinical-intelligence.html): an AI system that automates administrative tasks and optimises clinical workflow.
These technologies have the potential to revolutionize the way we deliver care, making it more efficient, effective, and patient-centered.
Cardiovascular
Artificial intelligence algorithms have shown promising results in accurately diagnosing and risk stratifying patients with concern for coronary artery disease.
AI can potentially be used as an initial triage tool, helping to identify patients who need further evaluation.
Studies have shown that AI can diagnose heart attack better than human physicians in the emergency setting, reducing both low-value testing and missed diagnoses.
AI is being used to analyze microscopy images in cardiovascular tissue engineering and organoid studies.
Wearables, smartphones, and internet-based technologies have also shown the ability to monitor patients' cardiac data points, expanding the amount of data and the various settings AI models can use.
AI has been used to classify heart sounds and diagnose valvular disease, showing its potential in various cardiovascular applications.
However, challenges remain, including the limited data available to train machine learning models, such as limited data on social determinants of health as they pertain to cardiovascular disease.
AI has been shown to be non-inferior to humans in interpretation of cardiac echocardiograms, demonstrating its potential for accurate diagnosis.
Here's an interesting read: Ai Ml Models
Ophthalmology
In ophthalmology, artificial intelligence is being used to improve diagnosis rates and prevent blindness. The FDA authorized the marketing of a medical device in 2018 that uses an AI algorithm to diagnose diabetic retinopathy.
This technology has the potential to decrease detection time for eye diseases. AI-enhanced screening is being used to aid in the prevention of blindness.
The AI algorithm is specifically designed to diagnose diabetic retinopathy, a common cause of blindness.
Primary Care
Primary care has become a key development area for AI technologies. AI in primary care has been used for supporting decision making and predictive modeling.
There are only a few examples of AI decision support systems that were prospectively assessed on clinical efficacy when used in practice by physicians. These systems have shown a positive effect on treatment choice by physicians.
AI in primary care has also been used for business analytics. This has helped physicians make more informed decisions about patient care.
Radiology
AI is being studied in radiology to detect and diagnose diseases through CT and MR imaging. It's particularly useful in settings where human expertise is in short supply or data is too complex to be efficiently interpreted by human readers.
Several deep learning models have shown the capability to be as accurate as healthcare professionals in identifying diseases through medical imaging. However, few of the studies reporting these findings have been externally validated.
A different take: Medical Imaging Ai Software Developer
AI can provide non-interpretive benefits to radiologists, such as reducing noise in images and creating high-quality images from lower doses of radiation. This can be especially helpful in situations where patients may be exposed to excessive radiation.
Convolutional neural network-based analysis is one of the primary techniques used in AI for radiology. This technique has shown promise in analyzing images and identifying diseases.
Radiomics is another technique used in AI for radiology, which involves the analysis of images to identify patterns and characteristics that can be used to diagnose diseases.
You might like: Ai and Ml Images
Market Segmentation by Application
The AI healthcare market is segmented by application, with some areas leading the way in innovation. Robot-Assisted Surgery is one of the top segments, making surgical procedures more precise.
AI-powered robots can ensure absolute accuracy in complicated surgeries, such as those in cardiovascular or neurology. This is crucial for patient outcomes.
Clinical Trials are another area where AI is making a significant impact. AI algorithms are changing the game by providing rapid data analysis at every stage of clinical trials.
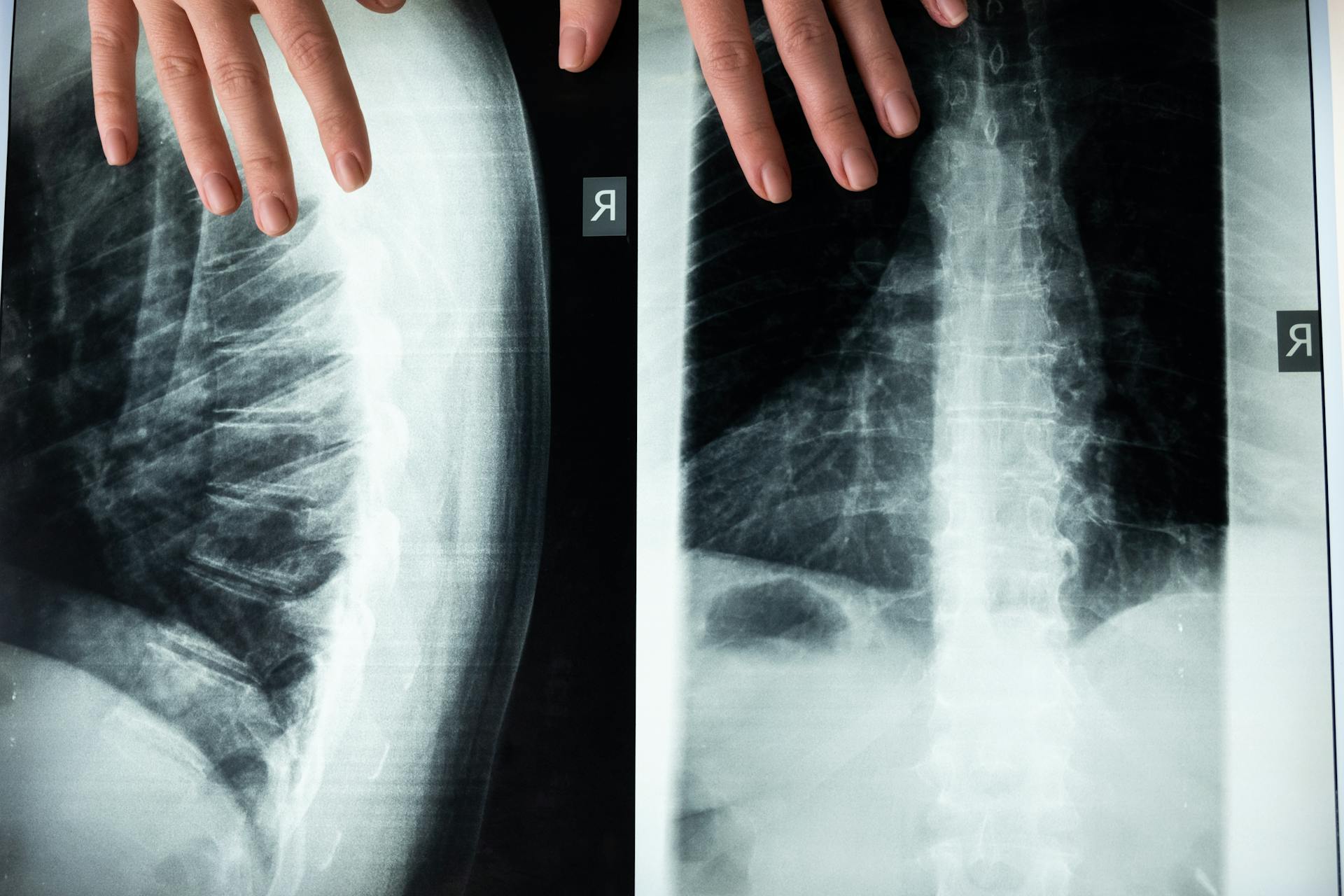
This makes clinical trials more accurate, cost-effective, and patient-centric. The power of AI in clinical trials is undeniable.
Connected Machines are also a key area of focus, with AI integrating data from IoT devices and sensors into a single healthcare system. This allows for the gathering, exchange, and analysis of huge amounts of data.
Interestingly, the FDA has approved 521 devices using AI, with Radiology accounting for 75% of these approvals.
Industry and Regulation
Regulations are being developed to address the unique challenges of AI in healthcare. The Health Insurance Portability and Accountability Act (HIPAA) protects patient data in the United States.
The European General Data Protection Regulation (GDPR) goes a step further, requiring consent from patients within the EU for the use of their healthcare data. HIPAA and GDPR are just two examples of existing regulations.
In the US, HIPAA protects healthcare data from patient records, while in the EU, GDPR requires consent for patient data use.
See what others are reading: Ai Ml Use Cases
Industry
The industry is rapidly adopting AI in healthcare, with companies like EliseAI, Cohere Health, Pfizer, Butterfly Network, and Novo Nordisk already using AI in their operations.
Big tech giants like Google, Microsoft, Amazon, and Apple are also investing heavily in AI healthcare, with Google's DeepMind being a key player in the market.
These companies are using AI for various purposes, including improving medical diagnosis, speeding up drug discovery, transforming patient experience, managing healthcare data, and performing robotic surgery.
Here are some examples of the uses of AI in healthcare:
- Improving medical diagnosis
- Speeding up drug discovery
- Transforming patient experience
- Managing healthcare data
- Performing robotic surgery
Tech giants are also developing AI-powered solutions, collaborating with other companies, and investing in AI startups to stay ahead in the competitive landscape.
Some notable players in the AI healthcare market include Google, Microsoft, Amazon, and Apple, each with their own focus areas such as AI pharmaceutical R&D, radiology, and imaging, health IT services, AI precision medicine, and wearable devices.
Regional Analysis
North America has a dominant position in the healthcare artificial intelligence market due to great support from the government and coordinated collaboration between the public and private sectors.
The USA is a key player in this region, having adopted AI technologies earlier than others.
Europe is the second-largest region in the AI healthcare market, with countries like Germany, the United Kingdom, France, Spain, Ireland, Switzerland, and Belgium focusing on R&D activity and biotechnology drug discovery.
These countries are driving innovation in life sciences, making Europe a hub for AI healthcare advancements.
The Asia-Pacific region is quickly growing, with a CAGR of 8.5% expected by 2028, driven by factors like a rising geriatric population, medical tourism, and growing research activities.
Asian countries are accelerating the adoption of medical AI to address these challenges.
In South America, the focus is on developing Remote Patient Monitoring (RPM) and telehealth, while the Middle East and Africa are growing more slowly, with a focus on AI-based telehealth services and increasing collaborations between healthcare facilities.
Additional reading: Claude Ai Europe
Market and Future
The AI in healthcare market is growing rapidly, with a projected CAGR of 40.2% by 2029. This growth is expected to reach USD 173.55 billion by 2029.
North America currently dominates the market, with a strong presence of key players. This is likely due to the early adoption of AI in healthcare in the region.
Mergers and acquisitions are becoming increasingly common as medical businesses grow and expand. Larger players are acquiring smaller companies to enhance their AI capabilities or extend their market coverage.
The integration of AI with complementary technologies like robotics or blockchain is promoting precision medicine and transforming healthcare delivery. This is leading to improved patient outcomes and more efficient healthcare systems.
Next 5-10 Years
The next 5-10 years will see significant progress in AI development, with powerful algorithms that require less data to train and can use unlabelled data becoming more efficient. This will enable AI to make more accurate predictions and improve patient care.
The healthcare industry will also evolve, with organisations and medical practices becoming co-innovators with technology partners in the development of novel AI systems for precision therapeutics. This will lead to more tailored treatments and better patient outcomes.
Readers also liked: Ai and Ml Development Services
AI-powered medical imaging technologies will continue to be a major trend, with healthtech companies using them to improve diagnosis and treatment. The global artificial intelligence in the healthcare market is expected to grow at a CAGR of 40.2% to reach USD 173.55 billion by 2029.
Here are some key statistics to keep in mind:
Generative AI-powered virtual assistants and chatbots will also become more prevalent, offering 24/7 support opportunities. This will be particularly useful for patients who need immediate assistance or have questions about their treatment.
Long Term (>10 Years)
In the long term, AI systems will become more intelligent, enabling AI healthcare systems achieve a state of precision medicine through AI-augmented healthcare and connected care.
Healthcare will shift from the traditional one-size-fits-all form of medicine to a preventative, personalised, data-driven disease management model that achieves improved patient outcomes in a more cost-effective delivery system.
This new model will lead to improved patient experiences and clinical outcomes, making healthcare more patient-centric and effective.
Sources
- https://pmc.ncbi.nlm.nih.gov/articles/PMC8285156/
- https://builtin.com/artificial-intelligence/artificial-intelligence-healthcare
- https://en.wikipedia.org/wiki/Artificial_intelligence_in_healthcare
- https://binariks.com/blog/artificial-intelligence-ai-healthcare-market/
- https://health.google/health-research/
Featured Images: pexels.com