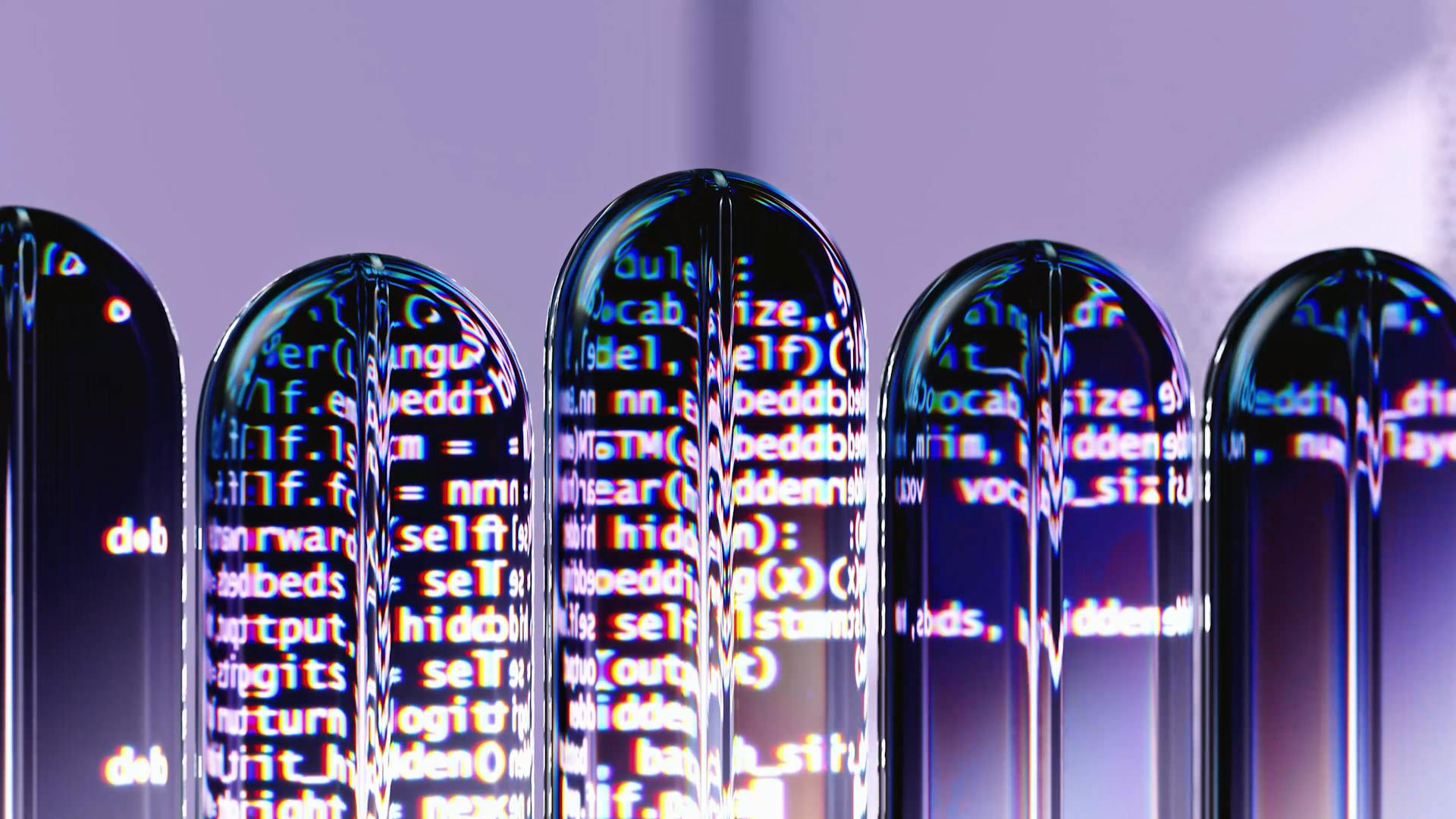
Generative AI is revolutionizing the healthcare industry with its vast potential to improve patient outcomes and streamline clinical workflows.
One notable application of generative AI in healthcare is medical imaging analysis.
Generative AI models can analyze medical images, such as X-rays and MRIs, to detect abnormalities and diagnose conditions more accurately than human radiologists.
This technology can help reduce the risk of human error and improve patient care.
Worth a look: Generative Ai Human Creativity and Art
Methods
To effectively integrate generative AI in healthcare, careful planning and execution are crucial. This involves considering factors such as data privacy and security.
The Technology Acceptance Model (TAM) and the Non-Adoption, Abandonment, Scale-up, Spread and Sustainability (NASSS) model are frameworks that can help promote responsible integration. These frameworks allow for anticipating and addressing barriers to adoption.
Structured adoption programs grounded in implementation science are necessary for shifting complex care ecosystems. Technological capabilities alone cannot accomplish this overnight.
Integration of generative AI necessitates meticulous change management and risk mitigation strategies.
You might like: Generative Ai Adoption
Conclusions
Generative AI can usher in tremendous healthcare progress if introduced responsibly.
Strategic adoption based on implementation science is key to promoting safe and ethical generative AI integration.
Extensive real-world piloting and iteration aligned to clinical priorities should drive development.
With conscientious governance centred on human wellbeing over technological novelty, generative AI can enhance accessibility, affordability and quality of care.
Ongoing reassessment and transparent communication around generative AI's strengths and weaknesses remain vital to restoring trust, realizing positive potential and improving patient outcomes.
Generative AI in Healthcare
Generative AI has the potential to revolutionize the healthcare industry by providing a wide range of benefits, including improved diagnosis, treatment, and patient care. By analyzing vast amounts of medical data, Generative AI can help identify patterns and make predictions, leading to more accurate diagnoses and personalized treatment plans.
Generative AI can also generate synthetic medical images that can be used to train machine learning models for image-based diagnosis, and can even reconstruct images to help doctors diagnose conditions more accurately. This can be particularly useful in situations where there is a limited amount of real-world patient data available.
Generative AI can also help improve the operational aspects of healthcare facilities by analyzing data to predict patient demand, forecasting medical supply needs, and optimizing bed allocation. This can help reduce wait times, improve patient outcomes, and make healthcare more efficient.
For another approach, see: Getty Images Nvidia Generative Ai Istock
Synthetic Generation and Augmentation
Synthetic data generation and augmentation are key applications of generative AI in healthcare. Generative AI models like GANs create realistic and anonymized patient data for research and training purposes, addressing data privacy concerns.
Synthetic data can be used to improve the accuracy and robustness of machine learning models by providing a more diverse and representative range of data.
Generative AI can also generate synthetic electronic health record (EHR) data, learning the underlying data distributions to achieve excellent performance.
Synthetic data generation capabilities are valuable for research and training purposes, protecting real patient data and adhering to privacy regulations.
Here are some potential benefits of synthetic data generation and augmentation:
• Improved accuracy and robustness of machine learning models
• Enhanced data privacy and protection
• Increased availability of diverse and representative data
• Improved research and training outcomes
By leveraging synthetic data generation and augmentation, researchers and clinicians can investigate and test various hypotheses, leading to new insights and discoveries.
Recommended read: Gen Ai vs Ml
Administrative Automation
Generative AI in healthcare is revolutionizing the way administrative tasks are handled. It automates duties such as scheduling, allowing healthcare professionals to focus on patient care.
Administrative tasks like billing and inventory management are also being taken care of by generative AI, freeing up staff to concentrate on more important tasks.
Automated medical coding is another area where generative AI is making a significant impact, accurately translating patient diagnoses and procedures into standardized codes for billing and documentation.
You might enjoy: Ai Ml in Healthcare
Use Cases
Generative AI is transforming the healthcare industry in numerous ways, and we'll explore some of the most exciting use cases below.
Generative AI can help healthcare professionals make informed decisions by analyzing vast amounts of medical data, including patient records, diagnostic images, and lab results. This can lead to improved clinical decision-making and better patient outcomes.
One of the most promising use cases is early disease detection. Generative AI can analyze electronic health records, medical imaging, and genetic information to identify subtle patterns and anomalies that might signal the presence of diseases in their early stages.
Recommended read: Ai Ml Use Cases
Generative AI can also assist surgeons in preoperative planning by generating detailed 3D models of patient anatomy and simulating surgical procedures. This can minimize risks and optimize outcomes.
In addition to these use cases, generative AI can also help automate administrative tasks, such as scheduling, billing, and inventory management. This can free up healthcare professionals to focus on patient care.
Generative AI can also be used to improve operational works in healthcare facilities. It can analyze data to predict patient demand and allocate healthcare staff and resources more effectively.
Here are some specific examples of how generative AI is being used in healthcare:
- PathAI uses generative AI to automate and improve the accuracy of diagnostic processes in pathology services.
- Zebra Medical Vision employs generative AI to analyze medical imaging data and assist radiologists in detecting and diagnosing diseases.
- January.ai uses generative AI to monitor glucose levels and provide personalized health recommendations to individuals.
Generative AI has the potential to revolutionize patient care and improve health outcomes. By leveraging its capabilities, healthcare professionals can make more informed decisions, improve diagnostic accuracy, and provide more personalized care to patients.
For your interest: Can Generative Ai Improve Social Science
Benefits and Impact
Studies have already shown that generative AI can deliver tremendous value and hundreds of billions of dollars in cost savings for the US healthcare industry.
Generative AI has the potential to eliminate nearly a billion manual interventions in Eligibility & Benefits Verification (EBV) and make the process faster and more efficient.
With its ability to process both structured and unstructured data, Gen AI can analyze vast and complex healthcare information to provide decision support to healthcare providers, improving efficiency, accuracy, and patient experience.
By automating the EBV process, Gen AI can reduce the number of calls healthcare providers have to field, making the modern healthcare experience smoother and more convenient for patients.
For another approach, see: Generative Ai Customer Experience
Challenges and Considerations
Lack of transparency in Generative AI models makes it difficult for healthcare professionals to understand how AI arrives at specific recommendations or diagnoses. This can lead to a lack of trust among healthcare professionals, who might be hesitant to rely on AI-generated insights.
Complex algorithms and bias in training data can contribute to transparency issues. Implementing explainability and interpretability features can help healthcare professionals grasp the rationale behind AI-generated decisions.
Poor quality data used for training GenAI models can produce inaccurate or misleading outputs, which can have a huge impact on patient health and well-being. A false diagnosis generated by GenAI could lead to unnecessary treatments or delayed diagnosis of serious conditions.
Broaden your view: Generative Ai in Healthcare Use Cases
Privacy & Security
Healthcare data is highly sensitive and regulated, making it a prime target for cyber-attacks. This data includes personal medical records, patient histories, and genomic information.
To address the risk of breaches or unauthorized access, healthcare organizations must implement stringent security measures. These include robust encryption, access controls, and regular security audits.
In the event of a cyber-attack, there's a chance of unauthorized modifications to AI models that could produce incorrect or even life-threatening results. This could occur if treatment recommendations or diagnoses are tampered with.
Ethical guidelines and regulatory frameworks are necessary to ensure the responsible and secure deployment of Generative AI in healthcare. This will help safeguard patient privacy and data integrity.
Healthcare organizations must take a proactive approach to security, rather than simply reacting to incidents. Regular security audits and updates to security measures can help prevent breaches and unauthorized access.
You might enjoy: How Has Generative Ai Affected Security
Existing System Interoperability
Existing system interoperability is crucial for the practical application of AI in healthcare.
Seamless integration with existing healthcare workflows and systems used by hospitals and clinics is necessary for successful implementation.
Hospitals and clinics already have established systems in place, and AI solutions need to be compatible with these systems to be effective.
Integration with Electronic Health Records (EHR) and other relevant tools is a key aspect of interoperability.
Ensuring compatibility with existing systems requires careful consideration and planning to avoid disruptions to hospital workflows.
Additional reading: Why Is Controlling the Output of Generative Ai
Implementation and Integration
To implement generative AI in healthcare, integration with existing systems is key. This involves ensuring compatibility with Electronic Health Records (EHR) and other relevant tools.
Seamless integration with existing healthcare workflows and systems used by hospitals and clinics is crucial for practical application. Interoperability is essential to avoid duplicating efforts and to ensure that patient data is up-to-date and accurate.
Integrating the validated AI model with existing healthcare systems requires careful planning and expertise. It's a complex process that needs to be done right to avoid any disruptions or errors.
To achieve seamless integration, you'll need to consider the specific requirements of your healthcare organization, including the systems and tools already in use. This might involve working with IT staff to ensure that the AI model is compatible with existing infrastructure.
Intriguing read: What Are the Generative Ai Tools
Sources
- https://implementationscience.biomedcentral.com/articles/10.1186/s13012-024-01357-9
- https://appinventiv.com/blog/generative-ai-in-healthcare/
- https://www.forbes.com/councils/forbesbusinesscouncil/2023/12/11/five-game-changing-use-cases-for-gen-ai-in-healthcare/
- https://www.a.team/mission/top-10-use-cases-for-generative-ai-in-healthcare
- https://www.mindbowser.com/generative-ai-healthcare-use-cases/
Featured Images: pexels.com