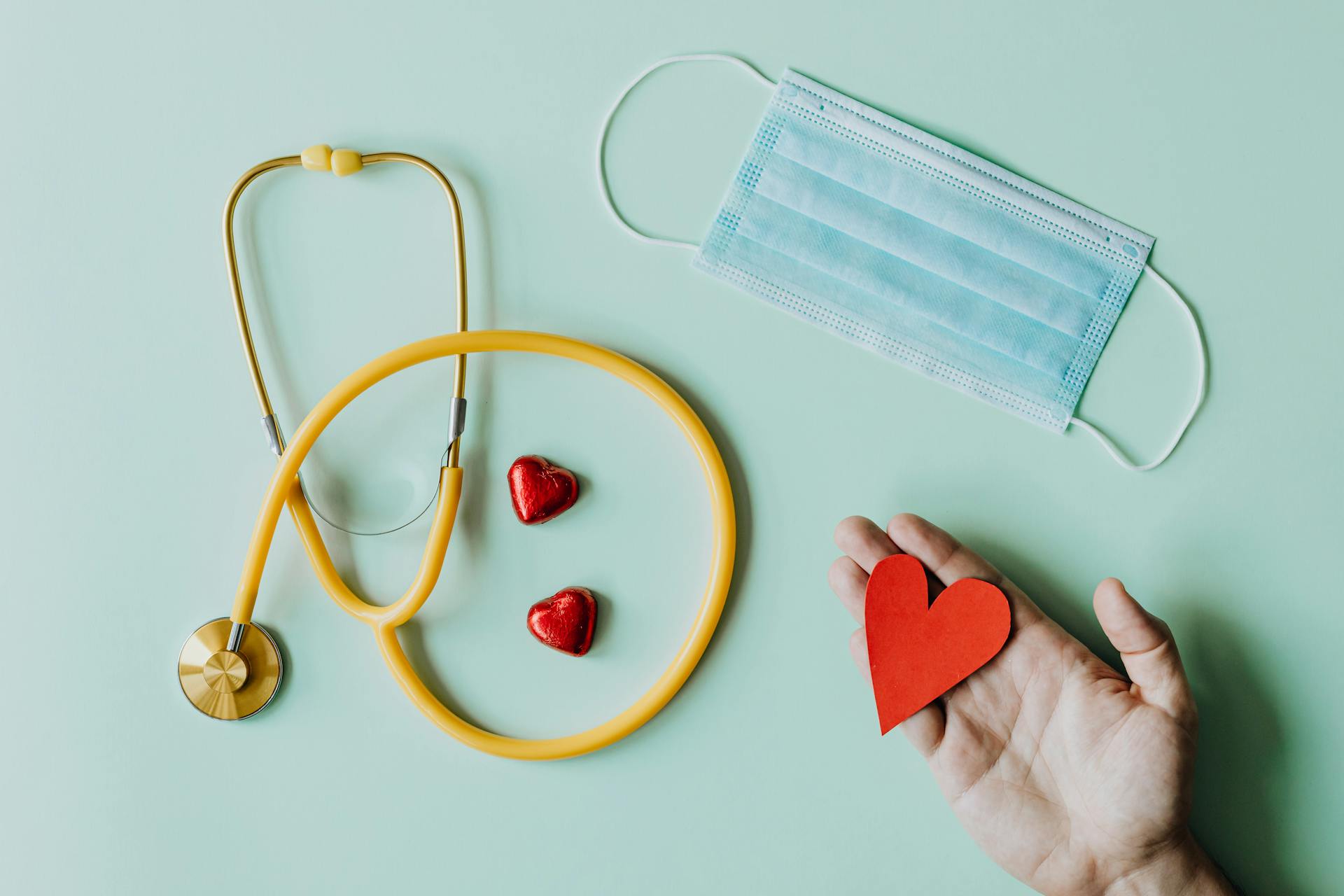
Machine learning is revolutionizing the healthcare industry by enhancing operational efficiency and patient outcomes.
Predictive analytics in healthcare can identify high-risk patients and prevent hospital readmissions by analyzing medical records and patient data. This can lead to significant cost savings and improved patient care.
Machine learning algorithms can also help doctors diagnose diseases more accurately and quickly. For example, a study found that a machine learning algorithm was able to detect breast cancer more accurately than human doctors in some cases.
By automating routine tasks and streamlining administrative processes, machine learning can free up healthcare professionals to focus on more complex and high-value tasks.
Readers also liked: Healthcare Informatic
Applications and Use Cases
Machine learning is revolutionizing the healthcare industry with its numerous applications and use cases. It's helping doctors and patients alike, and I'm excited to share some of the most impressive examples with you.
Machine learning algorithms are being used to detect diseases in early stages by comparing new data with old data on a particular ailment. This enables doctors to make informed decisions and design the best treatment plans accordingly.
A unique perspective: Google Announces New Generative Ai Search Capabilities for Doctors
One of the most significant applications of machine learning in healthcare is in medical imaging interpretation and diagnostic support. This technology enables clinicians to perform and interpret ultrasound exams more accurately and efficiently.
Machine learning is also being used to automate image diagnosis, recognizing abnormalities in medical images such as MRI or radiology scans. This helps doctors diagnose liver and kidney infections, identify tumors, and improve cancer prognosis.
Remote patient monitoring and telehealth are also being enhanced by machine learning, which processes real-time data from wearables and mobile applications to detect anomalies in patient health. This approach improves chronic disease management, reduces hospital readmissions, and offers proactive care.
Here are some of the top applications and use cases of machine learning in healthcare:
- Detecting diseases in early stages
- Medical imaging interpretation and diagnostic support
- Automating image diagnosis
- Remote patient monitoring and telehealth
- Computer vision for analyzing medical images, improving surgical assistance, and providing health monitoring
These applications are not only improving patient outcomes but also reducing costs and increasing efficiency in healthcare. As machine learning continues to evolve, we can expect even more innovative applications in the healthcare sector.
For your interest: Ai and Ml in Healthcare
Predictive Analytics and Diagnostic
Predictive analytics in healthcare is revolutionizing the way doctors diagnose and treat patients. Machine learning algorithms can analyze vast amounts of data, including electronic health records, genetic information, and wearable device data, to predict patient outcomes and identify potential health risks.
Prognos Health is a great example of this, using AI and Big Data analysis to generate insights from clinical laboratory data, supporting early diagnosis, risk stratification, and treatment optimization.
Machine learning can analyze medical images, such as X-rays, MRIs, and CT scans, to detect anomalies like tumors or lesions with remarkable precision. This is particularly useful for early-stage diagnosis of diseases like cancer or cardiovascular conditions.
Predictive analytics can also model the chances of disease progression, enabling healthcare providers to proactively address potential health issues before they become critical. The most common applications of predictive analytics in healthcare include chronic disease management, treatment response, post-surgical care, medication management, and preventive care.
Expand your knowledge: Predictive Learning
Here are some examples of how predictive analytics is being used in healthcare:
- Chronic disease management: Models flag when a patient's condition shows early signs of deterioration, prompting healthcare providers to adjust medications or recommend lifestyle changes.
- Treatment response: Machine learning algorithms can predict which chemotherapy regimens or targeted therapies are the most likely to be effective.
- Post-surgical care: Analysis of pre-existing conditions, surgery complexity, and recovery data models can identify patients likely to have longer recovery times or be at risk of post-surgery complications.
By analyzing large datasets, machine learning algorithms can identify patterns and correlations that human observers may overlook, leading to earlier and more accurate diagnoses. This is especially useful for rare and complex diseases, where early detection is critical for successful treatment outcomes and patient survival.
Care and Treatment
Machine learning is revolutionizing the way healthcare professionals approach patient care and treatment. By analyzing individual patient data, including genetic information, medical history, and responses to medications, machine learning models can identify patterns that correlate with specific outcomes.
This personalized approach allows doctors to design highly effective treatment plans, optimizing therapy effectiveness and minimizing side effects. For instance, in oncology, machine learning can predict how different patients with the same cancer type may respond to various treatments based on their unique profiles.
Machine learning can also streamline healthcare operations by optimizing workflows and automating repetitive administrative tasks. By analyzing hospital operational data, including patient admission trends, staff schedules, and resource usage, machine learning models can predict patient inflow and help hospitals manage staffing levels and reduce wait times.
Here's a summary of the benefits of machine learning in patient care and treatment:
- Personalized treatment plans based on individual patient data
- Improved treatment effectiveness and reduced side effects
- Optimized therapy effectiveness in oncology and other fields
- Streamlined healthcare operations and reduced wait times
Improving Operational Efficiency
Improving Operational Efficiency is crucial in healthcare, and machine learning is a key player in achieving this goal. By analyzing historical data, machine learning models can predict future demand for services, helping hospitals avoid overstaffing or understaffing.
Machine learning can automate administrative tasks, such as appointment scheduling, billing, and patient management, reducing errors and saving time. This frees up healthcare providers to focus on patient care, improving overall efficiency in healthcare delivery.
Predicting demand for supplies is another way machine learning can help reduce costs in healthcare. By optimizing inventory levels and reducing waste, hospitals can identify the best sources for high-quality, cost-effective supplies.
Machine learning can also predict patient flow, helping hospitals manage bed availability, optimize surgery scheduling, and triage patients in Accident and Emergency. This ensures that patients receive timely care, reducing bottlenecks and improving patient outcomes.
Here's a breakdown of how machine learning can improve operational efficiency in healthcare:
By implementing machine learning in healthcare, hospitals can streamline operations, reduce costs, and improve patient outcomes.
Care
Personalized medicine is revolutionizing the way healthcare is delivered, and machine learning is at the forefront of this transformation. Machine learning models can analyze patient data, including genetic information, past treatments, and responses to medications, to identify patterns that correlate with specific outcomes.
By leveraging machine learning, doctors can develop customized treatment plans that target specific diseases in each patient. This approach is most valuable in fields like oncology, where treatment effectiveness varies enormously between patients. In oncology, ML can predict how different patients with the same cancer type may respond to various treatments based on their unique profiles.
Machine learning can also help identify genetic mutations associated with various diseases and develop targeted therapies. For example, Deep Genomics applies machine learning to identify genetic mutations and develop personalized treatment options. This approach can predict disease progression and support personalized treatment plans.
Machine learning-driven personalized treatment plans can predict when a patient is most likely to stray from their treatment plan and put into motion interventions such as reminders or follow-up appointments to help patients stick to the plan. This dynamic approach to treatment plans ensures that care continuously adapts to the patient's evolving condition.
For your interest: Genetic Algorithm Machine Learning
Here are some key benefits of machine learning in personalized medicine:
• Improved patient outcomes
• Reduced side effects
• Enhanced patient experience
• Increased treatment effectiveness
• Reduced healthcare costs
Machine learning can also help streamline healthcare operations by analyzing hospital operational data, including patient admission trends, staff schedules, and resource usage, to optimize workflows. This can help hospitals better manage staffing levels and reduce wait times.
Technology and Tools
The Global Healthcare IT market is expected to reach USD 974.5 billion by 2027, driven in part by AI technology.
AI technology is a key driver behind the expansion of the Global Healthcare IT market.
AI-enabled technology in healthcare is expected to continue its exponential growth, with applications in areas such as diagnosis, treatment, and patient care.
The use of AI in healthcare can help improve patient outcomes, reduce costs, and enhance the overall quality of care.
AI technology can analyze large amounts of medical data to identify patterns and make predictions, enabling healthcare professionals to make more informed decisions.
How AI Works
AI works by aggregating and managing vast amounts of patient data from diverse sources, including Electronic Health Records (EHRs), wearable devices, and other medical records systems.
This comprehensive dataset encompasses critical information such as the patient's medical history, symptoms, lab results, imaging scans, and treatment outcomes.
AI can collect voice data and convert it into text with the help of Natural Language Processing.
To ensure data quality and compatibility, AI algorithms clean noisy data, normalize values, and perform feature engineering to extract relevant information.
Machine learning algorithms can operate in real-time, continuously analyzing incoming patient data to make predictions or classifications.
Here are the five main stages of how AI works in healthcare:
- Data Collection
- Preprocessing
- Real-time Prediction
- Decision Support
- Monitoring and Evaluation
Continuous monitoring and evaluation of machine learning algorithms are crucial to ensuring their accuracy and effectiveness over time.
How Appinventiv Can Help
Appinventiv's expertise in healthcare IT consulting services and machine learning development solutions can speed up time-to-value for your medical operations.
With a team of 1200+ software developers and a proven track record of delivering 3000+ successful projects, Appinventiv can be your trusted tech partner to bring your AI/ML in healthcare vision to life.
Appinventiv has worked on a multitude of healthcare projects over the past, integrating modern technologies including machine learning, IoT, blockchain, and more.
The company developed Soniphi, a personal wellness system that provides a complete well-being analysis report on a personal healthcare app.
Health-e-People, an integrated platform for connecting various health devices and records on one interface for comprehensive healthcare monitoring, is another notable project.
This solution also lets users draw predictive analysis from the records and connects the users with caregivers.
Ethics and Challenges
Machine learning in healthcare is a rapidly evolving field, but it's not without its challenges. From an ethical standpoint, there's a lot to consider.
Bias and fairness are major concerns in healthcare data, which often reflects historical disparities and inequalities. This can lead to biased representations of information on certain patients, amplifying disparities and resulting in unequal healthcare outcomes for different groups.
Patient autonomy and informed consent are also critical issues. Patients may be unaware of how their health data is being used to train AI models or influence clinical decision-making, undermining patient autonomy and decision-making agency.
To address bias in AI algorithms, it's essential to carefully evaluate training data for biases, implement bias detection techniques during model development, and employ fairness-aware algorithms that explicitly account for fairness constraints during training.
Take a look at this: Machine Learning Supervised Learning Algorithms
Success Stories and Benefits
Our track record speaks for itself, with many successful projects completed that have applied machine learning to healthcare with tangible benefits for clients. Some of our most recent case studies have shown practical applications of machine learning in healthcare.
Machine learning has assisted doctors in detecting diseases even before they occur, and has been used to research medicines, design personalized treatment plans, and save lives. It's also been used to reduce costs.
Healthcare companies are expected to allocate 10.5% of their budgets to AI and machine learning next year, up from 5.5% in 2022, as reported by Morgan Stanley.
Our Success Stories
Our success stories are a testament to the power of machine learning in healthcare. We've completed numerous projects that have resulted in tangible benefits for our clients.
Our track record speaks for itself, with projects like the ones mentioned in our case studies that have made a real difference. We've applied machine learning to healthcare in ways that have improved patient outcomes and reduced costs.
These practical applications of machine learning have led to significant benefits for our clients. By leveraging machine learning, we've helped them streamline processes and make more informed decisions.
One notable example is the project we completed that resulted in tangible benefits for our client. We've successfully completed numerous projects like this one, each with its own unique set of challenges and solutions.
Our experience has shown that machine learning can be a game-changer in healthcare, and we're proud to have been a part of it.
Benefits of
Our success stories in machine learning for healthcare are truly impressive, with tangible benefits for our clients.
We've successfully completed projects that showcase the practical applications of machine learning in healthcare, resulting in real-world benefits.
From detecting diseases even before they occur to designing personalized treatment plans, the advantages of machine learning in healthcare are widespread.
Machine learning in healthcare has been on the rise, with healthcare companies expected to allocate 10.5% of their budgets to AI and machine learning next year.
This growth in investment is a testament to the potential of machine learning in healthcare operations, both from a medical and business perspective.
Reducing costs is just one of the many benefits of machine learning in healthcare, making it a valuable tool for medical businesses and patients alike.
The importance of machine learning in healthcare cannot be overstated, with a wide range of use cases that are making a tangible difference in people's lives.
For your interest: Medical Imaging Ai Software Developer
Real-Life Use Cases of Projects
Machine learning in healthcare is already making a significant impact, with 94% of healthcare companies using AI or ML in 2023. This widespread adoption is a testament to the effectiveness of machine learning in healthcare.
Machine learning is helping research institutions, companies, and start-ups innovate and create transformative solutions across the healthcare sector. From diagnostics to personalized medicine, machine learning is driving massive improvement in the industry.
Machine learning is expediting advancements in areas including clinical operations, drug discovery, and surgery. It can detect early indicators of a disease more accurately, helping reduce the number of admissions and readmissions in hospitals and clinics.
Machine learning for healthcare examines satellite data, social media reports, news, and even video sources to determine whether the ailment is controllable or will become lethal. This technology opens a world of endless possibilities for medical professionals to find the best treatment plan and patient care.
The role of machine learning in medicine and healthcare has proven to be a boon for medical professionals and patients alike, redefining the industry and creating a better future for patients.
Explore further: Ai Medical Coding Software
Future of Machine Learning in Healthcare
The future of machine learning in healthcare is incredibly promising. Despite some challenges, ML can help resolve current healthcare problems and anticipate future hardships, preventing outbreaks worldwide.
Machine learning can analyze hundreds of distinct data points, personalizing treatment plans and predicting risks and results. This technology also provides earlier warnings for conditions like seizures or sepsis, which require intensive analysis of highly complex datasets.
In the coming years, professionals can use ML in conjunction with nanotechnology to improve medicine delivery. This breakthrough could revolutionize the way we administer medication.
Machine learning can also enable "virtual biopsies" and advance the innovative field of radiomics. This technology can help doctors make more accurate diagnoses and develop targeted treatments.
Programmed robots could soon assist doctors in the surgery room, helping to minimize the risk during operations by providing detailed treatment information.
Sources
- https://spd.tech/machine-learning/machine-learning-in-healthcare/
- https://eithealth.eu/news-article/machine-learning-in-healthcare-uses-benefits-and-pioneers-in-the-field/
- https://appinventiv.com/blog/machine-learning-in-healthcare/
- https://acropolium.com/blog/machine-learning-in-healthcare-use-cases-benefits-and-success-stories/
- https://www.geeksforgeeks.org/7-applications-of-machine-learning-in-healthcare-industry/
Featured Images: pexels.com