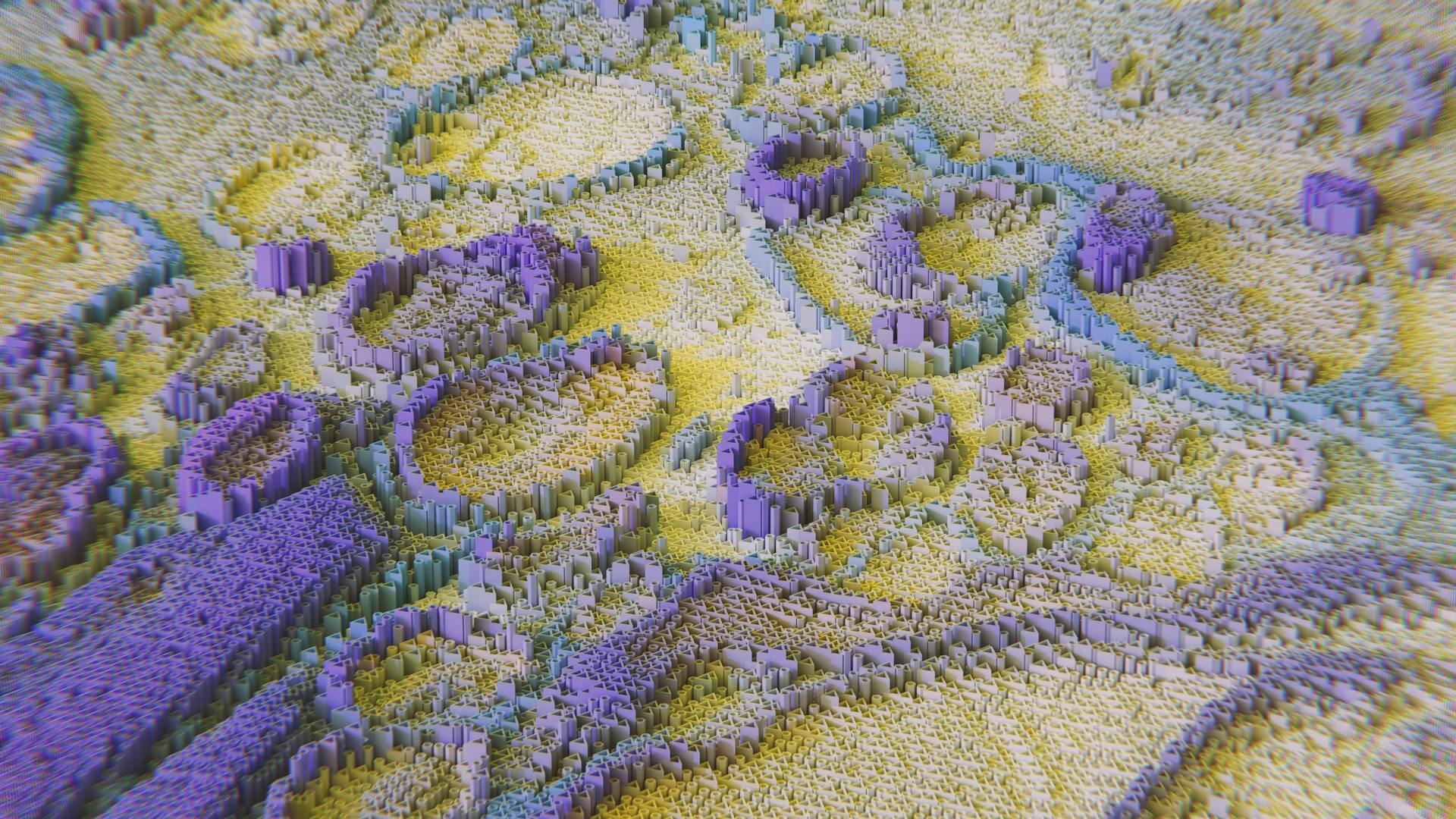
Generative AI is transforming industries by leveraging multimodal technology, which enables machines to understand and process multiple forms of data, such as text, images, and audio. This fusion of modalities is revolutionizing the way we approach tasks.
Multimodal generative AI can analyze and synthesize vast amounts of data, creating new possibilities for applications like content creation, recommendation systems, and even healthcare. By combining different types of data, these models can generate more accurate and informative results.
For instance, a multimodal generative AI model can analyze a medical image and generate a report, while also suggesting potential diagnoses and treatment options. This level of integration and automation is transforming industries and improving lives.
You might enjoy: Can I Generate Code Using Generative Ai
What is Multimodal AI?
Multimodal AI is a cutting-edge technology that mirrors human perception by leveraging multiple sensory inputs, such as text, images, videos, and audio, to understand and interpret the world. This technology enables a range of capabilities, from generating images based on text descriptions to summarizing video content and facilitating natural interaction with AI through voice commands.
Intriguing read: Getty Images Nvidia Generative Ai Istock
At the core of multimodal AI are models that can process different data types or modalities, such as natural language processing (NLP) models for text data, computer vision models for images and videos, and speech recognition models for audio.
There are three main components to multimodal AI: input modules, fusion modules, and output modules. Input modules receive and process different data types individually, fusion modules capture relevant data from each modality and integrate and align the information, and output modules produce the final results or prediction for the user.
The central multimodal fusion component integrates the information from the different inputs, resolving any conflicts or ambiguities before generating a unified multimodal response. This fusion component can combine information from different modalities using techniques such as deep learning neural networks or rule-based systems.
Multimodal AI can unlock innovative opportunities for AI applications that are more intuitive and all-encompassing, narrowing the divide in the interaction and comprehension between humans and machines.
Readers also liked: What Are the Two Main Types of Generative Ai Models
Benefits and Advantages
Multimodal generative AI has numerous benefits and advantages that make it a powerful tool for various applications and industries.
One of the key advantages of multimodal generative AI is its potential to provide more natural and intuitive interactions between humans and large language models (LLMs). This can lead to better customer experiences.
Higher accuracy is another significant benefit of multimodal generative AI. By taking information inputs in several formats, it can achieve higher accuracy compared to unimodal LLMs.
Multimodal AI can also focus on the most relevant information from each input, making it more resource-efficient. This allows models to learn how to limit the amount of irrelevant data to process.
Another advantage of multimodal AI is its ability to provide different sources of information to explain its system's output, making the outputs easier to interpret and increasing accountability.
Here are some of the notable benefits of multimodal AI:
- Better context: Multimodal AI analyzes different inputs and recognizes patterns, leading to natural and human-like accurate outputs.
- Accuracy: Since multimodal AI combines different data streams, it can result in more reliable and precise outcomes.
- Enhanced problem solving: Multimodal AI can process diverse inputs, making it possible to tackle more complex challenges.
- Cross-domain learning: It can efficiently transfer knowledge between different modalities, enhancing data adaptability to perform various tasks.
- Creativity: Multimodal AI can blend data and open up new possibilities to create innovative outputs.
- Rich interactions: Augmented reality, chatbots, and virtual assistants can use multimodal AI to provide a more intuitive user experience.
In addition to these benefits, multimodal generative AI has the potential to reshape the user experience for both end-users and business users by creating new avenues for machine interaction.
Systems and Tools
Multimodal generative AI systems use a structured approach to process inputs from various data types, including images, videos, audio, and textual prompts. This approach involves rigorous safety mechanisms to screen for inappropriate content.
The safety mechanism is a crucial step in ensuring that the generated outputs are coherent and relevant. Once cleared, the advanced AI model processes the inputs, leveraging patterns and associations learned from extensive datasets.
The final step involves refining generated outputs to enhance quality and appropriateness before delivering them to the user. This process is essential in providing users with improved experiences and intuitive interactions with technology.
Several advanced tools are already paving the way for enhancing multimodal artificial intelligence. These tools include Google Gemini, Vertex AI, OpenAI's CLIP, and Hugging Face's Transformers.
Here are some of the key features of these tools:
- Google Gemini can integrate images, texts, and other modalities to create, understand, and enhance content.
- Vertex AI is the machine learning platform of Google Cloud, which can process different data and perform tasks like image recognition, analyzing video, and more.
- OpenAI's CLIP can process text and images to perform tasks like visual search and image captioning.
- Hugging Face's Transformers can support multimodal learning and build versatile AI systems by processing audio, text, and images.
These systems demonstrate the practical application of multimodal generative AI in everyday situations, highlighting how different data types seamlessly merge with AI processing techniques.
Use Cases and Applications
Multimodal generative AI has a wide range of use cases, but each business and its sector will have a different specific area with the highest likely return on investment.
According to Neurons Lab, a GenAI Workshop and Proof of Concept service can help businesses turn their ideas into detailed strategies and tactics, with 75-100% funded by AWS. This service can validate assumptions and reduce risk when investing in GenAI technology.
Here are just a few example use cases for enterprises, including supply chain optimization, customer-facing operations, and marketing and advertising. Supply chain optimization can be achieved by analyzing multimodal data to provide real-time insights into inventory management, demand forecasting, and quality control. This can lead to optimized inventory levels, predictive maintenance schedules, and more context-aware and personalized responses, improving the customer experience.
For more insights, see: Generative Ai Inventory Management
Improved Healthcare
Improved healthcare is a promising area for AI integration, with the potential to enable more accurate diagnostics and personalized patient care. Taylor Dolezal, head of ecosystem at the Cloud Native Computing Foundation, sees considerable promise in this sector.
Multimodal generative AI is particularly useful for diagnostic tools, surgical robots, and remote monitoring devices. These advancements promise improved patient outcomes and accelerated medical research.
However, they pose challenges in data integration, accuracy, and patient privacy. This is a significant concern that needs to be addressed.
Data integration is a major challenge in healthcare AI, as it requires combining various data types to enable accurate diagnostics. For example, medical images, patient records, and lab results need to be integrated to provide a complete picture of a patient's condition.
To overcome these challenges, healthcare professionals and AI developers need to work together to design and implement effective solutions. This requires a multidisciplinary approach that combines medical expertise with AI knowledge.
Some potential benefits of AI in healthcare include:
- Improved diagnostic accuracy
- Personalized patient care
- Accelerated medical research
However, as with any new technology, there are also potential drawbacks to consider, such as the cost of implementing and maintaining AI systems, as well as the need for ongoing training and education for healthcare professionals.
8 Use Cases
Here are eight practical use cases for multimodal generative AI:
Multimodal generative AI can help create dynamic content, such as personalized videos and images, improving engagement on social media platforms and personal blogs.
Personalized recommendations can be provided through the analysis of diverse data sources, encompassing text, images, and audio, enriching convenience and satisfaction for users.
Multimodal AI can track various health metrics, provide personalized fitness advice, and alert users to potential health issues, promoting overall well-being.
Multimodal AI systems can control smart home devices using voice commands, gestures, or images, allowing individuals to automate tasks and improve energy efficiency.
AI-powered tutoring platforms can offer personalized learning experiences by adapting content to individual preferences and providing interactive feedback through text, audio, and video.
Multimodal AI applications in assistive technologies can empower individuals with disabilities by enabling hands-free communication, object recognition, and navigation support.
Multimodal generative AI can optimize supply chain processes by analyzing multimodal data to provide real-time insights into inventory management, demand forecasting, and quality control.
See what others are reading: Generative Ai Text Analysis
Here are some specific use cases for multimodal generative AI in different industries:
Key Concepts and Techniques
Multimodal generative AI combines capabilities across text, images, audio, and video for richer interactions. This technology is on track to redefine digital experiences, offering unprecedented levels of personalization and engagement.
Blending multiple modalities introduces complex, meaningful engagement between humans and machines, mirroring human communication more closely. This shift marks a significant advancement in artificial intelligence.
The evolution from single-modal to multimodal AI requires careful navigation of challenges like data privacy, computational demands, and ethical concerns.
The Many Paths
Multimodal AI has the potential to revolutionize various domains, including self-driving technology and healthcare. With ongoing advancements, it's expected to become more advanced in solving complex problems in a human-like manner.
The challenges of multimodal AI, such as biases and data requirements, need to be addressed to unlock its full potential. Despite these challenges, multimodal AI is on track to redefine digital experiences.
For another approach, see: The Economic Potential of Generative Ai
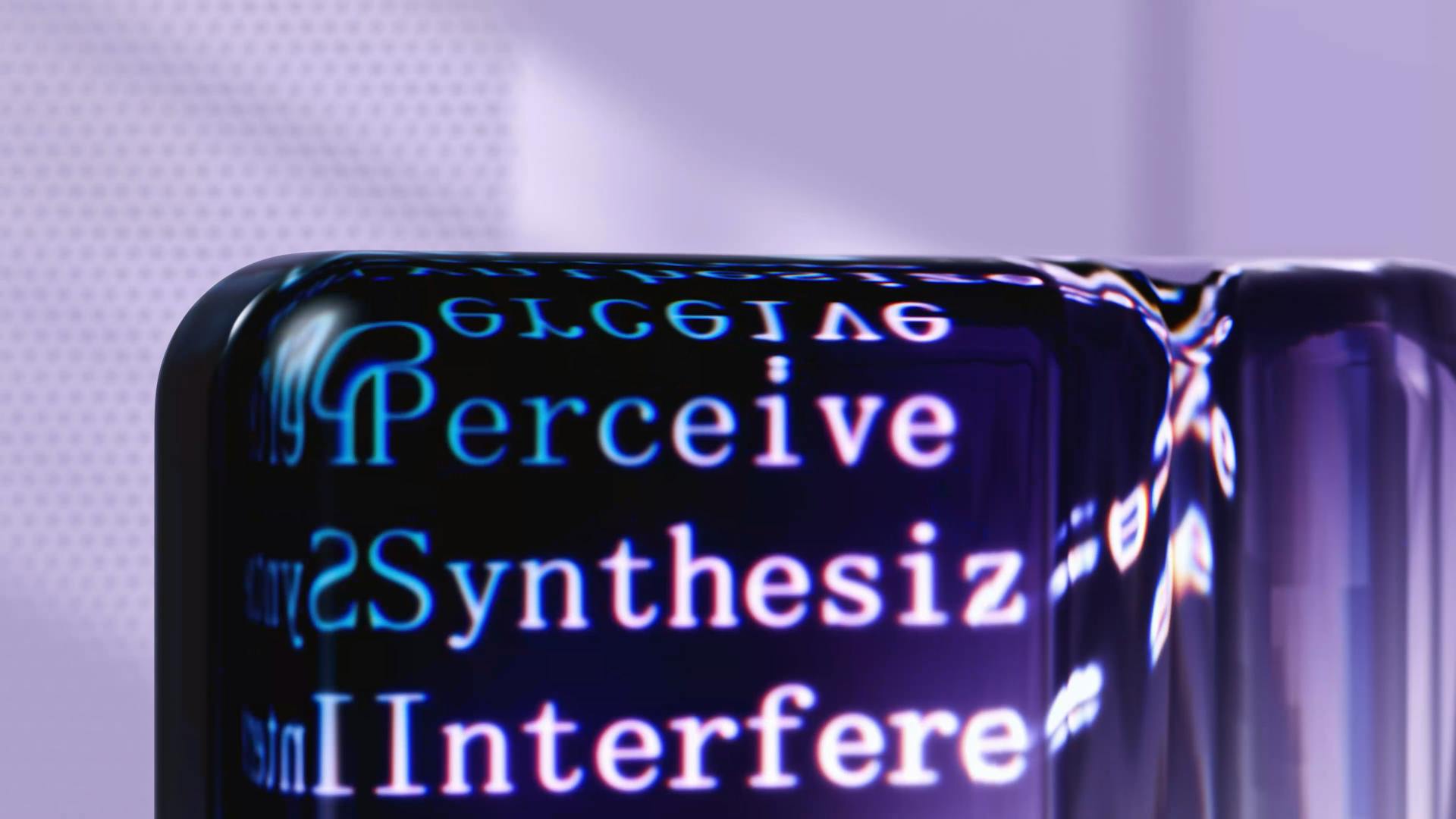
Data fusion is a significant aspect of multimodal AI, but it's also a complex task that requires careful navigation. It's challenging to align relevant data representing the same time and space when diverse data types are involved.
Multimodal AI has the potential to offer unprecedented levels of personalization and engagement, but it requires careful handling of data privacy and computational demands.
Representation
Representation is a crucial aspect of multimodal translation, where a model must effectively capture semantic information and connections between text, audio, and images.
Managing various noise levels, missing data, and merging data from many modalities are some of the difficulties that come with multimodal representation.
Creating representations that effectively capture multimodal data is a complex task, as it requires the model to comprehend the semantic information and connections between different modalities.
Abby, a writer and SEO content strategist, would agree that effectively capturing semantic information is key to successful representation, as it enables the model to understand the context and meaning of the data.
Managing noise levels and missing data is a significant challenge in multimodal representation, as it can lead to inaccurate or incomplete representations.
By effectively managing noise levels and missing data, a model can create more accurate and robust representations, which is essential for successful multimodal translation.
Getting Started and Next Steps
Getting started with multimodal models is exciting, and you can begin by exploring consumer-available chatbots like ChatGPT and Gemini, formerly called Bard. These platforms offer an excellent opportunity to enhance productivity in various areas.
Businesses can automate routine customer interactions, assist in creative content generation, simplify complex data analysis, and interpret visual data in conjunction with text queries using these chatbots.
To get started, consider experimenting with these chatbots to understand their capabilities and limitations. According to Samuel Hamway, research analyst at Nucleus Research, this hands-on approach will help you identify potential use cases for your business.
Here's an interesting read: How to Get Started with Generative Ai
Getting Started
To get started with multimodal models, explore consumer-available chatbots like ChatGPT and Gemini, formerly called Bard. These platforms offer a great opportunity to enhance productivity by automating routine customer interactions and assisting in creative content generation.
ChatGPT and Gemini can simplify complex data analysis and interpret visual data in conjunction with text queries. This is particularly useful for businesses looking to streamline their operations.
Major AI services, including OpenAI's GPT-4 and Google's Gemini, are starting to support multimodal capabilities. These models can understand and generate content across multiple formats, including text, images, and audio.
Businesses should start by experimenting with these chatbots to see how they can be applied to their specific needs.
For more insights, see: Is Speech to Text Generative Ai
Final Thoughts
As you start exploring multimodal generative AI, it's essential to remember its vast potential to transform businesses across industries.
Companies with the best customer experience bring in 5.7 times more revenue than others, according to Forbes, making it a direct link to revenue.
Optimizing front-office services through improved customer experiences and unprecedented personalization is a key benefit of multimodal generative AI.
Greater operational efficiency and optimized costs in back-office functions are also significant advantages.
These benefits align closely with the main focuses of enterprises' GenAI initiatives, based on a recent Gartner survey.
If this caught your attention, see: Generative Ai Customer Support
Sources
- https://www.spiceworks.com/tech/artificial-intelligence/articles/multimodal-generative-ai-adoption/
- https://www.neilsahota.com/multimodal-generative-ai-next-big-leap-in-generative-intelligence/
- https://www.techtarget.com/searchenterpriseai/feature/Explore-real-world-use-cases-for-multimodal-generative-AI
- https://www.neurons-lab.com/article/multimodal-ai-use-cases-the-next-opportunity-in-enterprise-ai/
- https://www.splunk.com/en_us/blog/learn/multimodal-ai.html
Featured Images: pexels.com