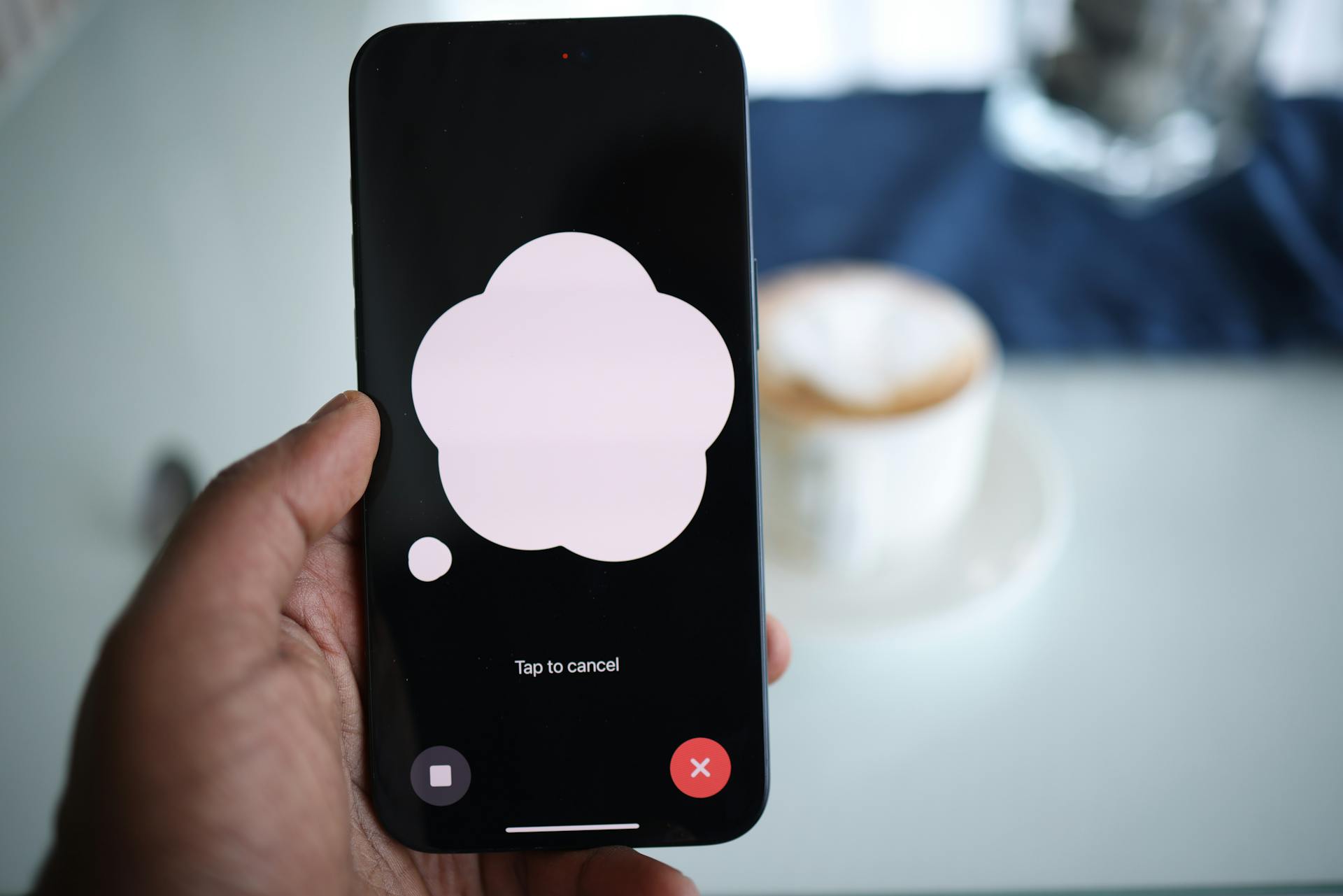
Speech to text generative AI is revolutionizing the way we interact with computers. It's no longer a futuristic concept, but a rapidly evolving technology that's becoming increasingly accessible and user-friendly.
Studies have shown that speech recognition accuracy has improved dramatically over the years, with some systems now boasting accuracy rates of up to 95%. This is largely due to advancements in deep learning algorithms and large-scale data training.
The benefits of speech to text generative AI are numerous, including increased accessibility for people with disabilities, improved productivity, and enhanced user experience. For instance, a study found that users who used speech recognition software were able to complete tasks up to 30% faster than those who used traditional typing methods.
As the technology continues to advance, we can expect to see even more innovative applications of speech to text generative AI, from virtual assistants to smart home devices.
Additional reading: How Multimodal Used in Generative Ai
What is Speech to Text
Speech to Text is a technology that allows you to convert spoken words into written text. It's a game-changer for people who need to take notes quickly or for those who prefer typing with their voice.
Intriguing read: Text to Speech Ai Training
The first speech recognition system, Audrey, was developed in 1952 by three Bell Labs researchers. It could only recognize digits.
Speech to Text has come a long way since then. With the advancement of technology, we now have various tools that can help us achieve this. For example, Codio offers courses on Speech to Text, including Intro to Whisper, Advance Whisper, and Meeting Transcription and Action Item Extraction, all of which are 60-minute courses.
These tools can be useful for people who need to transcribe audio or video files, such as podcasters, reporters, or students. They can also be used for people who have difficulty typing or writing, such as those with disabilities.
Here are some examples of Speech to Text courses offered by Codio:
- Codio: Intro to Whisper•60 minutes
- Codio: Advance Whisper•60 minutes
- Codio: Meeting Transcription and Action Item Extraction•60 minutes
Applications and Uses
Speech to text generative AI has a wide range of applications and uses.
It can be used to transcribe interviews, lectures, and meetings, making it easier to review and reference important information.
For instance, journalists can use it to transcribe interviews with sources, while students can use it to transcribe lectures and study sessions.
It's also being used in customer service to help resolve issues more quickly and efficiently.
With speech to text generative AI, users can dictate emails, messages, and other written content, freeing up their hands and eyes for other tasks.
It can even be used to create written content, such as articles and blog posts, by dictating text and then editing it as needed.
This technology is also being explored for use in healthcare, where it can be used to transcribe medical notes and other important documents.
By automating the transcription process, speech to text generative AI can help reduce errors and save time for healthcare professionals.
It's also being used in education to help students with disabilities, such as those who are deaf or hard of hearing, by providing a way for them to participate in class and complete assignments.
Speech to text generative AI can also be used to create audio descriptions for visually impaired individuals, making it easier for them to access and understand visual content.
Recommended read: Chatgpt Openai Generative Ai Chatbot Can Be Used for
Technical Aspects
Automatic Speech Recognition (ASR) technology is at the core of speech to text generative AI. It's what enables us to convert spoken words into written text.
ASR systems use complex algorithms to analyze audio data and transcribe it into text. This process involves several key steps, including the Acoustic Model, which handles the initial conversion of audio signals into phonemes, the smallest units of sound in a language.
The Acoustic Model is a crucial component of ASR, and it's what allows us to accurately transcribe spoken words into text. Here's a breakdown of the key components involved in ASR:
The Language Model and Dictionary work together to ensure that the transcribed text is accurate and makes sense in context.
A History of Speech Recognition
Speech recognition has been around for over 50 years, with the first system, Audrey, developed in 1952 by three Bell Labs researchers. Audrey was designed to recognize only digits.
The first speech recognition system capable of recognizing more than just digits was IBM's Shoebox, introduced in the early 1960s. It could recognize 16 words, including digits, and perform basic math operations.
In the 1970s, the Defense Advanced Research Projects Agency (DARPA) funded a program called Speech Understanding Research, which led to the development of Harpy in 1976. Harpy was able to recognize 1,011 words, a significant achievement at the time.
The Hidden Markov Model (HMM) was applied to speech recognition systems in the 1980s, providing a statistical model for sequential information. This marked a significant improvement in speech recognition technology.
In 2001, Google introduced Voice Search, allowing users to search for queries by speaking to the machine. This was a major breakthrough in voice-enabled applications.
By 2011, Apple had launched Siri, a real-time, faster, and easier way to interact with Apple devices using voice commands. Today, Amazon's Alexa and Google's Home are among the most popular voice command-based virtual assistants.
Here's a brief timeline of the key milestones in speech recognition development:
- 1952: Audrey, the first speech recognition system, is developed by Bell Labs researchers.
- Early 1960s: IBM introduces Shoebox, the first speech recognition system capable of recognizing more than just digits.
- 1976: Harpy is developed, able to recognize 1,011 words.
- 2001: Google introduces Voice Search.
- 2011: Apple launches Siri.
Deep Learning
Deep learning is a key component of AI speech to text systems, particularly in terms of accuracy and adaptability. It uses multiple layers to process audio data, making it highly effective in transcribing spoken language.
Deep learning models can adapt to different accents, dialects, and languages, making them versatile and reliable. This is particularly useful in real-world applications, where speech patterns can vary greatly.
One of the benefits of deep learning in speech to text is its ability to handle complex audio data. By using multiple layers to process this data, deep learning models can identify patterns and relationships that might be missed by simpler models.
Deep learning models are also highly accurate, with some models achieving accuracy rates of over 90%. This is particularly impressive when you consider the complexity of speech data.
Here are some key features of deep learning models used in speech to text:
Overall, deep learning is a powerful tool in the field of speech to text, offering high accuracy and adaptability in a wide range of applications.
Limitations and Challenges
Speech to text generative AI is a powerful tool, but like any technology, it has its limitations and challenges. Background noise can significantly impact its accuracy, making it difficult for the AI to transcribe speech clearly.
Our research has shown that the scope of hallucinations in speech to text AI can be widened if a broader set of topics are discussed. This is because the AI may struggle to understand the context and provide accurate transcriptions.
In some cases, the AI may even generate non-target language transcriptions, which can be both beneficial and detrimental. For example, code switching can be accurately transcribed, but in other cases, the AI may simply hallucinate a different language.
The accuracy of speech to text AI is highly dependent on the quality and diversity of the training data. This is a crucial factor to consider when evaluating the effectiveness of this technology.
Here are some of the key challenges associated with speech to text AI:
- Background Noise: Noise and multiple speakers can challenge the accuracy of AI transcription.
- Context Understanding: AI systems may struggle with understanding context, leading to errors in transcriptions.
- Training Data: The accuracy of AI speech to text systems is highly dependent on the quality and diversity of the training data.
Detecting Hallucinations
Detecting hallucinations is a crucial step in identifying the limitations of AI models like Whisper. Hallucinations are often non-deterministic, yielding different random text on each run of the API.
Programmatically detecting potential hallucinations can be done by comparing the same audio segments when run through Whisper twice in close succession. This was done in April 2023 and May 2023, with the December 2023 Whisper run used for longitudinal validation.
Multi-token differences between the two transcriptions over time can indicate hallucinations. For example, an audio segment with the words "pick the bread and peanut butter" yielded transcriptions with hallucinated sentences like "Take the bread and add butter. In a large mixing bowl, combine the softened butter."
Hallucinations are consistently different from truly transcribed sentences, making re-running the same audio through the API multiple times a useful way to identify them. Manual review confirmed that 187 audio segments reliably result in Whisper hallucinations.
The portions of the Whisper transcription that mirror the ground truth are often highly accurate, but hallucinations appear as lengthy appended text that are never uttered in the audio.
A different take: Generative Ai Text Analysis
Limitations and Challenges
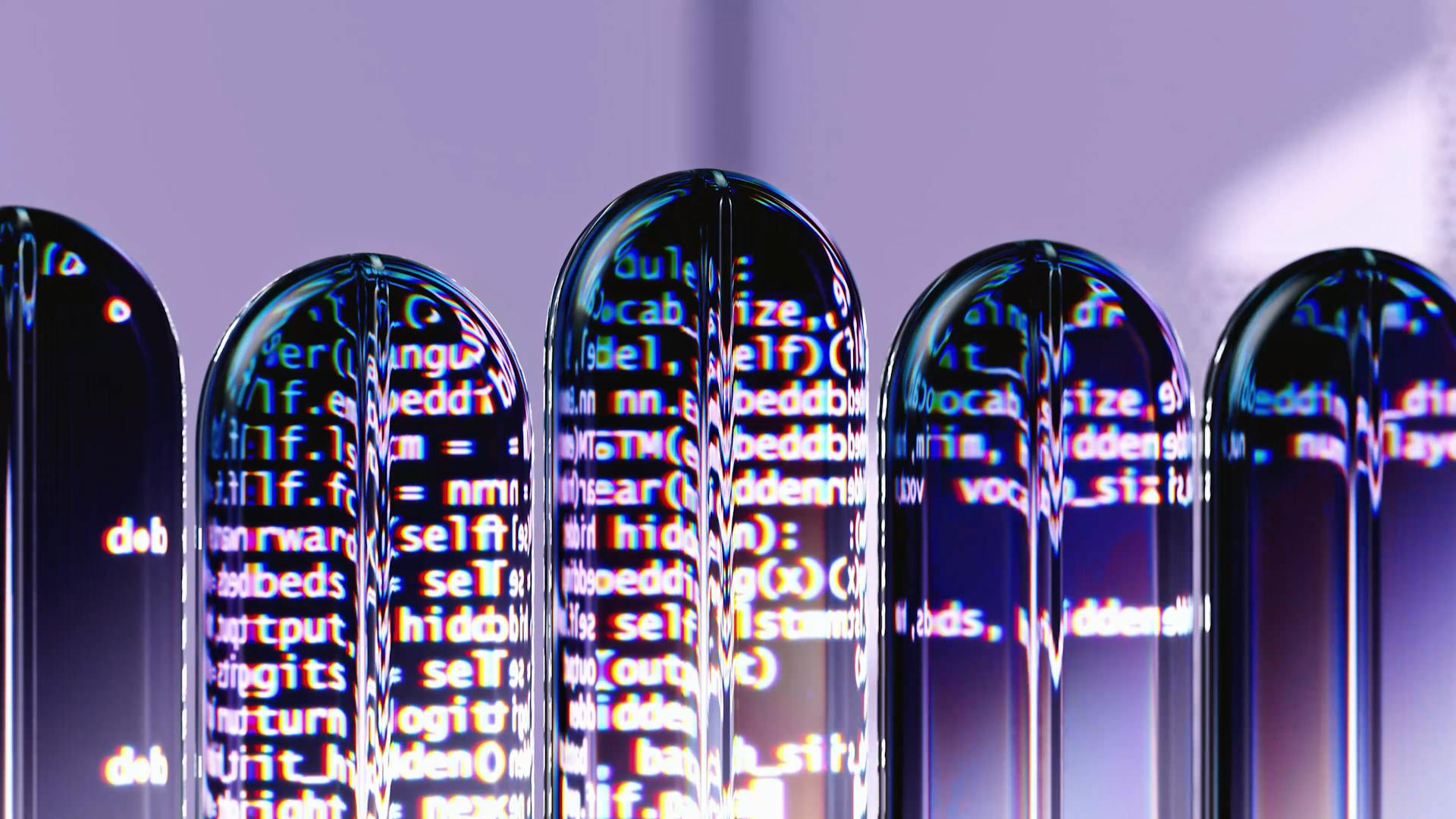
Our research has its limitations, and one of them is that we compared a relatively small set of aphasia speakers to control group speakers in a standard interview setting. This might not be representative of a broader set of topics or discussions.
Background noise can challenge the accuracy of AI transcription. Noise and multiple speakers can make it difficult for AI systems to accurately transcribe speech. I've seen this happen in noisy environments like coffee shops or busy streets.
The accuracy of AI speech to text systems is highly dependent on the quality and diversity of the training data. If the training data is limited or biased, the AI system will likely struggle to accurately transcribe speech.
Context understanding is another challenge that AI systems face. They may struggle to understand the context of a conversation, leading to errors in transcriptions. This can be frustrating, especially in situations where context is crucial.
Related reading: Why Is Controlling the Output of Generative Ai
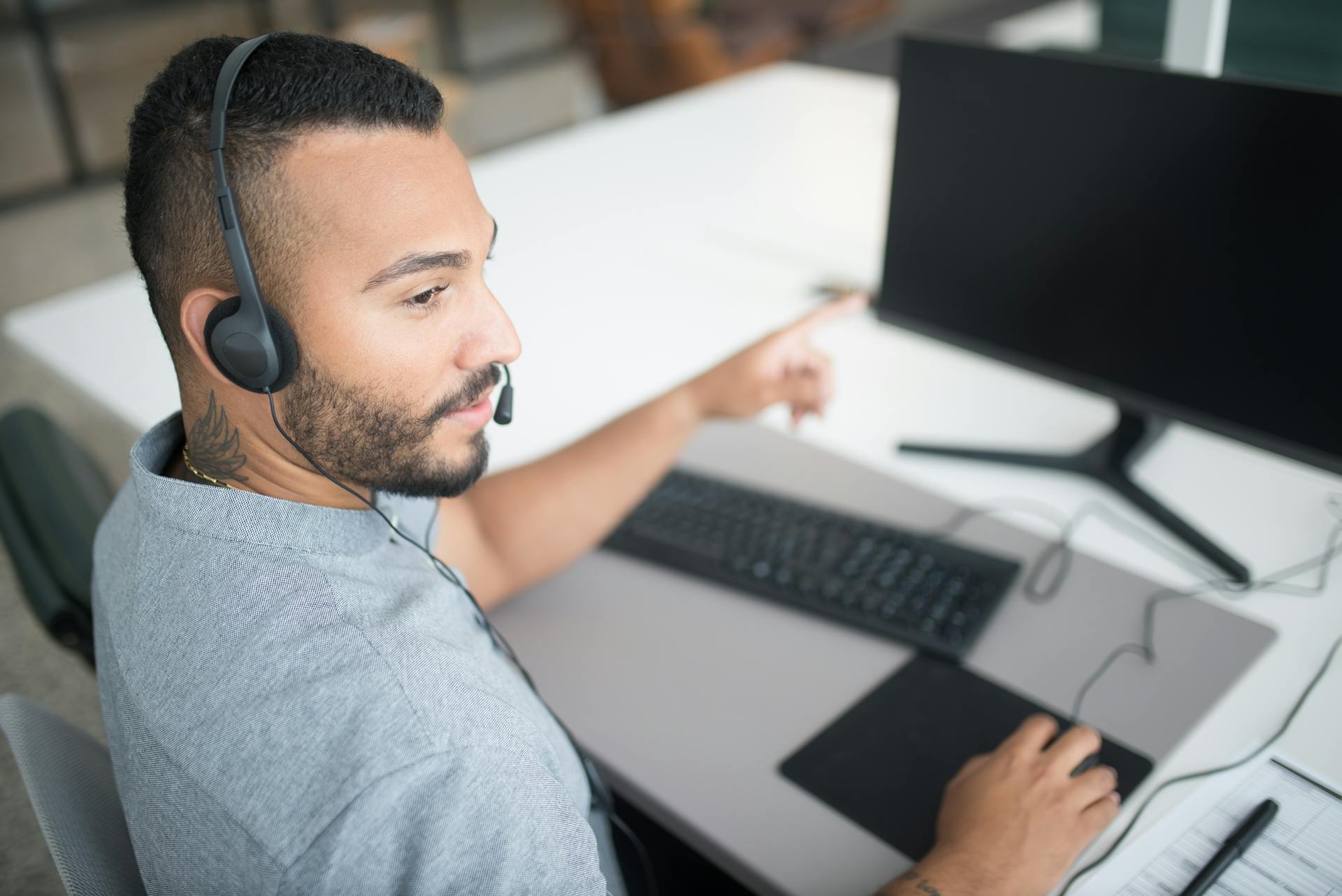
AI speech to text technology has its pros and cons. On the one hand, it can achieve high levels of accuracy and transcribe large volumes of audio data quickly and efficiently. On the other hand, it can struggle with background noise, context understanding, and training data quality.
Here are some of the challenges that AI speech to text technology faces:
- Background Noise: Noise and multiple speakers can challenge the accuracy of AI transcription.
- Context Understanding: AI systems may struggle with understanding context, leading to errors in transcriptions.
- Training Data: The accuracy of AI speech to text systems is highly dependent on the quality and diversity of the training data.
Frequently Asked Questions
Does voice to text count as AI?
Yes, voice-to-text technology utilizes AI-powered speech recognition and transcription capabilities to improve accuracy and vocabulary. This AI-driven approach enables more precise transcriptions from audio inputs.
Sources
- https://www.coursera.org/learn/codio-multimodal-generative-ai-vision-speech-and-assistants
- https://medium.com/@must.ai.generator/the-science-of-ai-speech-to-text-how-it-works-fd06f26d1f1b
- https://viqsolutions.com/media-center/the-brave-new-world-of-ai-generated-speech-to-text-and-language-models/
- https://arxiv.org/html/2402.08021v2
- https://www.analyticsvidhya.com/blog/2019/07/learn-build-first-speech-to-text-model-python/
Featured Images: pexels.com