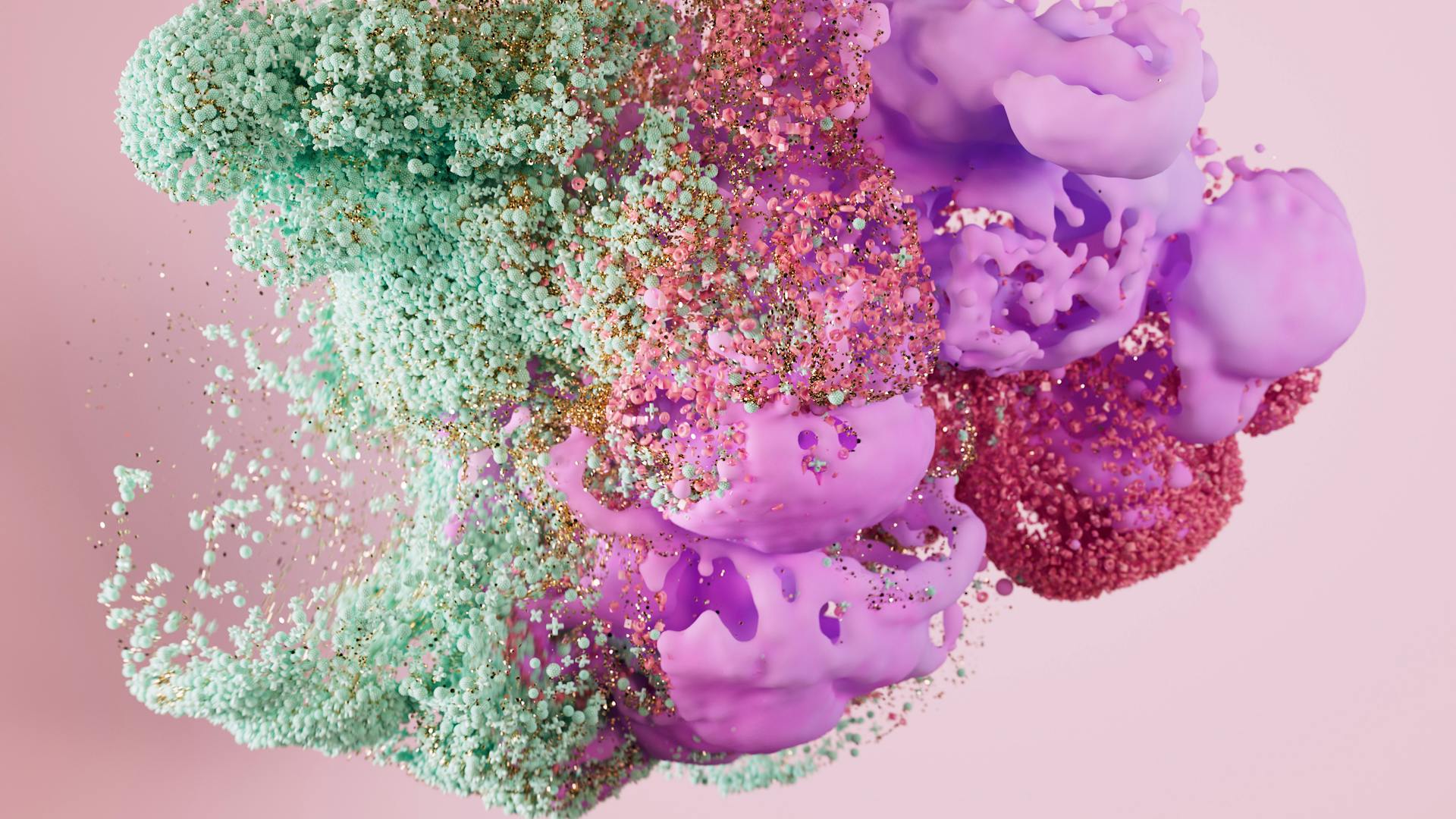
Generative AI has revolutionized the way we approach creative tasks, enabling machines to produce original content that's often indistinguishable from human-created work.
With the ability to generate images, music, and even entire stories, generative AI is pushing the boundaries of what's possible in various industries.
One of the key benefits of generative AI is its speed and efficiency. For example, a generative AI model can produce hundreds of images in a matter of seconds, compared to a human artist who might spend hours or even days working on a single piece.
This technology has the potential to democratize access to creative tools, making it possible for anyone to create high-quality content without needing extensive training or experience.
On a similar theme: Generative Ai by Getty Images
What Is Generative AI?
Generative AI is an exciting field that has the potential to revolutionize the way we create and consume content.
It can generate new art, music, and even realistic human faces that never existed before. One of the most promising aspects of Generative AI is its ability to create unique and customized products for various industries.
Additional reading: Create with Confidence Using Generative Ai
In the fashion industry, Generative AI can be used to create new and unique clothing designs. In contrast, in interior design, it can help generate new and innovative home decor ideas.
Generative AI is a type of artificial intelligence that creates content like pictures, text, or music.
You've probably used or heard of systems like ChatGPT, Bing, Bard, YouChat, DALL-E, or Jasper, which use generative AI. These systems learn from data and generate original content that looks or sounds similar.
Generative AI has applications in entertainment, healthcare, and even finance, making it a versatile tool.
On a similar theme: Generative Ai Content
How Does Generative AI Work?
Generative AI starts with a prompt that could be in the form of a text, an image, a video, a design, musical notes, or any input that the AI system can process.
Early versions of generative AI required submitting data via an API or a complicated process, but now developers are creating better user experiences that let you describe a request in plain language.
Various AI algorithms then return new content in response to the prompt, which can include essays, solutions to problems, or realistic fakes created from pictures or audio of a person.
Generative AI works by using algorithms to analyze the patterns and relationships within existing data, such as text, images, or audio.
Generative AI models can generate new data through two main methods: Generative Adversarial Networks (GANs) and Variational Autoencoders (VAEs).
GANs consist of two competing neural networks: a generator and a discriminator, which forces the generator to improve its ability to generate realistic data.
VAEs are neural networks that encode input data into a compressed representation called the latent space and then decode it to generate similar data.
Generative AI models learn from existing data to create new data through GANs’ competitive process or VAEs’ encoding and decoding.
A different take: Neural Network vs Generative Ai
Types of Generative AI Models
Generative AI models combine various AI algorithms to represent and process content. These models use techniques like GANs and variational autoencoders (VAEs) to generate new content in response to a query or prompt.
Broaden your view: Generative Ai Content Creation
One type of generative AI model is the transformer, which has led to significant advancements in AI-generated content. Techniques like Google's Bidirectional Encoder Representations from Transformers (BERT) and OpenAI's GPT have enabled neural networks to not only encode language but also generate new content.
These models can generate realistic human faces, synthetic data for AI training, and even facsimiles of particular humans. They work by applying a particular neural network to generate new content, using techniques like GANs and VAEs to transform raw data into vectors.
Check this out: Can I Generate Code Using Generative Ai Models
What Are the Types of Models?
Generative AI models can be quite complex, but they're essentially a combination of various AI algorithms that help represent and process content. Generative AI models use techniques such as natural language processing to transform raw characters into vectors, which are then used to generate new content.
One of the key techniques used in generative AI is Generative Adversarial Networks, or GANs for short. GANs are a type of deep learning technique that involves two competing neural networks: a generator and a discriminator. The generator produces novel content similar to the original input, while the discriminator sets apart the initial data from the produced data.
Some other notable models include transformers like BERT and GPT, which can encode language and generate new content. These models have been trained on vast amounts of data and can produce highly realistic results.
Here are some of the key types of generative AI models mentioned in the article:
These models are capable of generating a wide range of content, from text and images to synthetic data and even facsimiles of particular humans.
VAE
VAEs, or Variational Autoencoders, are a type of neural network that combines encoders and decoders to create sophisticated generative models. They're particularly useful when dealing with enormous datasets.
VAEs work by having the decoder network improve its ability to recreate the original information, which in turn allows the encoder network to gain abilities to represent data. This process is a key part of what makes VAEs so effective.
VAEs can produce potent generative models by maximizing accurate depictions and regenerating data. They're a great option for individuals looking to produce novel content using AI-generated models.
Worth a look: Generative Adversarial Network Ai
Here are some key characteristics of VAEs:
- Use encoders and decoders, two neural networks that work together to provide the best generative models.
- Can create sophisticated generative models of material.
- Are particularly useful when dealing with enormous datasets.
- Can produce potent generative models by maximizing accurate depictions and regenerating data.
- Are a great option for individuals looking to produce novel content using AI-generated models.
Gaussian Mixture Model
A Gaussian Mixture Model is a type of generative probabilistic framework that combines multiple Gaussian distributions to produce information sets.
GMMs are a parametric framework for the likelihood distribution of parameters in a biometric system, which means they help determine the probability of certain parameters being present.
They're particularly useful in speaker identification technology, analyzing vocal-tract-associated spectral characteristics to identify unique speaker patterns.
In essence, GMMs are a powerful tool for generating and analyzing data in various applications, including biometrics and speaker recognition.
Applications and Use Cases
Generative AI has a wide range of applications across various industries, including finance, legal, manufacturing, film and media, medical, architecture, gaming, art and design, music composition, content creation, fashion, and product design.
Generative AI can be used to design and interpret contracts, analyze evidence, and suggest arguments in legal firms, or to combine data from cameras, X-ray, and other metrics to identify defective parts and the root causes in manufacturing. It can also produce content more economically and translate it into other languages with the actors' own voices in film and media companies.
A different take: Legal Implications of Ai
Some of the key use cases for generative AI include:
- Implementing chatbots for customer service and technical support.
- Deploying deepfakes for mimicking people or even specific individuals.
- Improving dubbing for movies and educational content in different languages.
- Writing email responses, dating profiles, resumes, and term papers.
- Creating photorealistic art in a particular style.
- Improving product demonstration videos.
- Suggesting new drug compounds to test.
- Designing physical products and buildings.
- Optimizing new chip designs.
- Writing music in a specific style or tone.
Applications and Use Cases
Generative AI is a powerful tool with a wide range of applications and use cases. It can be applied in various industries to generate virtually any kind of content.
Generative AI can be used to implement chatbots for customer service and technical support, deploy deepfakes for mimicking people or specific individuals, and improve dubbing for movies and educational content in different languages.
One of the most exciting applications of generative AI is in the field of art and design. It can enable artists and designers to create unique artwork, generate new design concepts, and explore novel styles and compositions.
Some of the use cases for generative AI include improving product demonstration videos, suggesting new drug compounds to test, designing physical products and buildings, and optimizing new chip designs.
Here are some of the ways generative AI can impact different industries:
Generative AI can also be used to speed up tedious or repetitive operations like coding, email writing, and documentation summary. It can generate data and content customized for an intended audience, like creating chatbots for customized consumer experiences or targeted marketing that follows a specific consumer’s behavioral patterns.
Improving Search
Improving Search is a game-changer for anyone who's ever struggled to find the right information online. With generative AI capabilities in Search, you'll be able to understand a topic faster and get things done more easily.
You can ask a question like "what's better for a family with kids under 3 and a dog, bryce canyon or arches" and get an AI-powered snapshot of key information to consider, with links to dig deeper.
This snapshot is a huge time-saver, as it takes the heavy lifting out of searching and helps you identify the most important factors to consider. You'll see suggested next steps, including the ability to ask follow-up questions like "How long to spend at Bryce Canyon with kids?".
These follow-up questions allow you to continue exploring your topic naturally, with context carried over from question to question. This means you can dive deeper into your topic without having to start from scratch each time.
You'll also find helpful jumping-off points to web content and a range of perspectives that you can dig into. This is especially useful for topics that require multiple viewpoints, like travel recommendations for families with kids and dogs.
Check this out: Generative Ai Interview Questions
Sources
- https://www.techtarget.com/searchenterpriseai/definition/generative-AI
- https://neebal.com/generative-ai-vs-predictive-ai-unraveling-the-distinctions-and-applications/
- https://www.analyticsvidhya.com/blog/2023/04/what-is-generative-ai/
- https://blog.google/products/search/generative-ai-search/
- https://www.couchbase.com/blog/generative-ai-development/
Featured Images: pexels.com