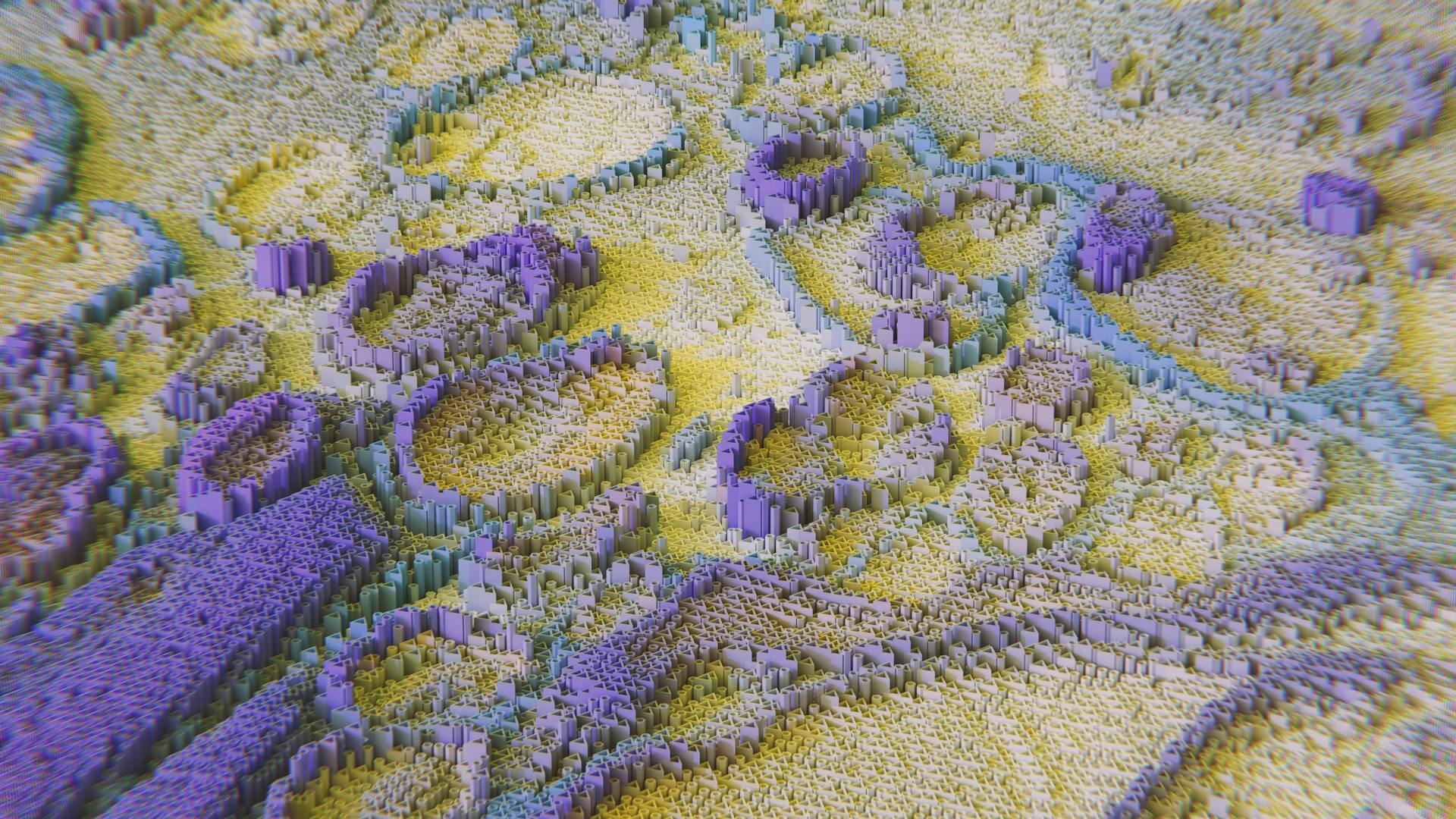
The generative AI ecosystem is a complex web of technologies and applications that are transforming the way we live and work. At its core, it's a system that uses algorithms to generate new content, such as images, music, and text, based on patterns and structures learned from existing data.
Generative AI models are trained on vast amounts of data, which allows them to learn and improve over time. This training process is often referred to as "deep learning." For instance, a generative AI model trained on a dataset of images can learn to generate new images that are similar in style and composition.
One of the key benefits of the generative AI ecosystem is its ability to automate tasks and processes that were previously time-consuming and labor-intensive. This can lead to significant productivity gains and cost savings for businesses and organizations that adopt these technologies.
If this caught your attention, see: What Does the Generative Ai Ecosystem Refer to
Generative AI Tools
Elasticsearch provides a suite of powerful development tools for building relevant, enterprise search experiences and AI apps with its Elasticsearch Relevance Engine. This production-ready, highly scalable, and trusted platform makes use of a vector database, semantic search, and transformer models.
Readers also liked: Sge Generative Ai in Search
You can create, store, and search embeddings for dense retrieval and capture your unstructured data's meaning and context across various data types, including text, images, videos, audio, and geo-location. Elasticsearch goes further than other vector databases with a full suite of search capabilities, including filters and faceting, document level security, and on-prem or cloud deployment.
To get started, you can use the Elastic Learned Sparse Encoder model, which is easily implementable with a single click when setting up your new search application. This model provides query expansions with related keywords and relevance scores, making it easily understood and ready for prime time on any dataset – no fine-tuning required.
On a similar theme: Google Generative Ai Search
A New Generation of Tools
Elasticsearch Relevance Engine is a suite of powerful development tools that make use of a vector database, semantic search, and transformer models. It's production-ready, highly scalable, and trusted by developers worldwide.
You can get the foundation for a full vector search experience and generative AI integration using a single platform to create, store, and search embeddings for dense retrieval and capture your unstructured data's meaning and context.
On a similar theme: Vector Database for Generative Ai
Elasticsearch offers a full suite of search capabilities, including filters and faceting, document level security, on-prem or cloud deployment, and more. This makes it a robust choice for developers.
Here are some key features of Elasticsearch's vector database:
- Support for dense retrieval and capturing unstructured data's meaning and context
- On-prem or cloud deployment options
- Document level security for sensitive data
- Filters and faceting for precise search results
The Elastic Learned Sparse Encoder model provides relevant semantic search out of the box across domains with query expansions and relevance scores. This model is easily implemented with a single click when setting up your new search application.
By incorporating proprietary, business-specific information with LLMs, you can enhance the quality of LLM output via context window. This is especially useful when working with generative AI applications that rely on publicly trained data.
Discover more: What Is a Best Practice When Using Generative Ai
Supervised Fine-Tuning
Supervised fine-tuning is a great way to improve your Generative AI model's performance. It's especially useful when working with midsize datasets that range from 1 to 5 GB in size.
Typically, conducting five training runs is sufficient to achieve the desired model performance. This approach helps you identify specific areas for enhancement and allows for more tailored adjustments to the model.
Additional reading: Geophysics Velocity Model Prediciton Using Generative Ai
Intellectual Property Ownership is another benefit of supervised fine-tuning. By fine-tuning your own models, you retain ownership of the intellectual property (IP) and maintain full control over the data flow.
Fine-tuning internally also enhances security for your customers by minimizing data exposure to third-party providers. This is a big deal, especially in industries like healthcare where data security is paramount.
Fine-tuning enables you to reduce reliance on external vendors, allowing for greater flexibility in model adjustments and improvements. This is a huge advantage for organizations that want to stay in control of their data and models.
Here are some key benefits of supervised fine-tuning at a glance:
Evolving Role and Impact
The Generative AI ecosystem has been rapidly evolving since 2020, driven by the advent of large Machine Learning Models. This ecosystem comprises various key components, each playing a crucial role in shaping the future of AI-driven innovation.
The journey of Generative AI began in 2017 with the release of transformers, which laid the foundation for the development of more advanced and versatile AI systems. The subsequent emergence of BERT, RoBERTa, and various versions of GPT further accelerated this progress.
The generative AI ecosystem has been rapidly evolving, with significant advancements in language models and their adoption across various industries worldwide. This rapid development is expected to revolutionize the industry, with widespread adoption expected in the near future.
Here's an interesting read: Generative Ai and the Future of Work in America
The Evolving Role
The Generative AI ecosystem has been rapidly evolving since 2020, driven by the advent of large Machine Learning (ML) Models. This ecosystem comprises various key components, each playing a crucial role in shaping the future of AI-driven innovation.
The journey of generative AI began in 2017 with the release of transformers, marking a significant milestone in AI development. These models laid the foundation for the development of more advanced and versatile AI systems.
Major companies are investing significant resources into AI, transforming both technical and software engineering landscapes. This investment is expected to revolutionize the industry, with widespread adoption expected in the near future.
To build a successful career in AI, individuals should follow a well-defined learning path to master technologies like generative AI and LLMs. This includes experimenting with various applications and maintaining perseverance, which will be crucial for achieving long-term success in the AI field.
The global impact and adoption patterns of generative AI are being driven by significant advancements in language models and their adoption across various industries worldwide. This has led to a rapid evolution of the generative AI ecosystem, with costs decreasing and organizations focusing on high integration and comprehensive AI ecosystems.
Discover more: Generative Ai for Software Development
Manuscript Assessment
Manuscript Assessment plays a crucial role in the publishing industry, where AI-powered tools are used to evaluate the quality and potential of submitted manuscripts. These tools leverage Large Language Models (LLMs) to analyze various aspects of the manuscript, such as writing style, plot development, and character depth.
Ensuring the accuracy and reliability of these assessments is critical, as it directly impacts authors' careers and the publishing industry's reputation. The accuracy of the LLM is paramount, providing precise and insightful feedback on the manuscript's strengths and weaknesses.
In manuscript assessment, observability is crucial to understand the reasoning behind the AI's assessments and ensure that it is not making biased or unfair judgments. This is achieved through effective guardrails, such as human oversight and clear guidelines for the AI's decision-making process.
The client's needs vary, ranging from minimal assessment to in-depth analysis. For instance, if the client wants to figure out if the manuscript is grammatically correct or not, a minimal assessment is sufficient. However, if the client wants the entire manuscript rewritten, an in-depth analysis of the language and content is required.
Here are some scenarios where different approaches are needed:
Accuracy vs Observability
Accuracy vs Observability is a crucial tradeoff in the generative AI ecosystem. Companies face a significant challenge in managing this tradeoff, especially when it comes to fine-tuning and pre-training their models.
LLMs provide a significant boost in accuracy, but this comes with the disadvantage of reduced observability. This means that companies have less control over the model's behavior.
Implementing proper guardrails is essential to mitigate this issue. Guardrails are essential to ensure reliable and safe deployment of LLMs. Companies need to implement effective guardrails to manage the accuracy and observability tradeoff.
Properly managing this tradeoff is essential to leverage the benefits of LLMs. Companies need to manage these aspects independently to ensure reliable and safe deployment.
Additional reading: Generative Ai Companies
Frequently Asked Questions
What does generative AI ecosystem mean?
The generative AI ecosystem is a network of technologies and tools that enable the development and application of generative AI. It's a complex system that brings together various components to power the creation of AI models and applications.
Where is the generative AI ecosystem right now?
The generative AI ecosystem is already established with hundreds of online platforms available for hands-on experience. These platforms are just the tip of the iceberg, with technology coming from a diverse range of sources.
What are generative AI systems?
Generative AI systems are advanced models that can create new content from various inputs, such as text, images, or audio, by generating outputs in different formats. They can transform one type of data into another, like turning text into an image or a video into a song.
Sources
- https://www.elastic.co/generative-ai
- https://www.projectpro.io/podcast/title/generative-ai-ecosystem
- https://aithority.com/machine-learning/elastic-announces-ai-ecosystem-to-accelerate-genai-application-development/
- https://draup.com/sales/whitepapers/the-generative-ai-landscape-a-comprehensive-ecosystem-overview/
- https://speakerdeck.com/draup/the-generative-ai-landscape-an-ecosystem-overview
Featured Images: pexels.com