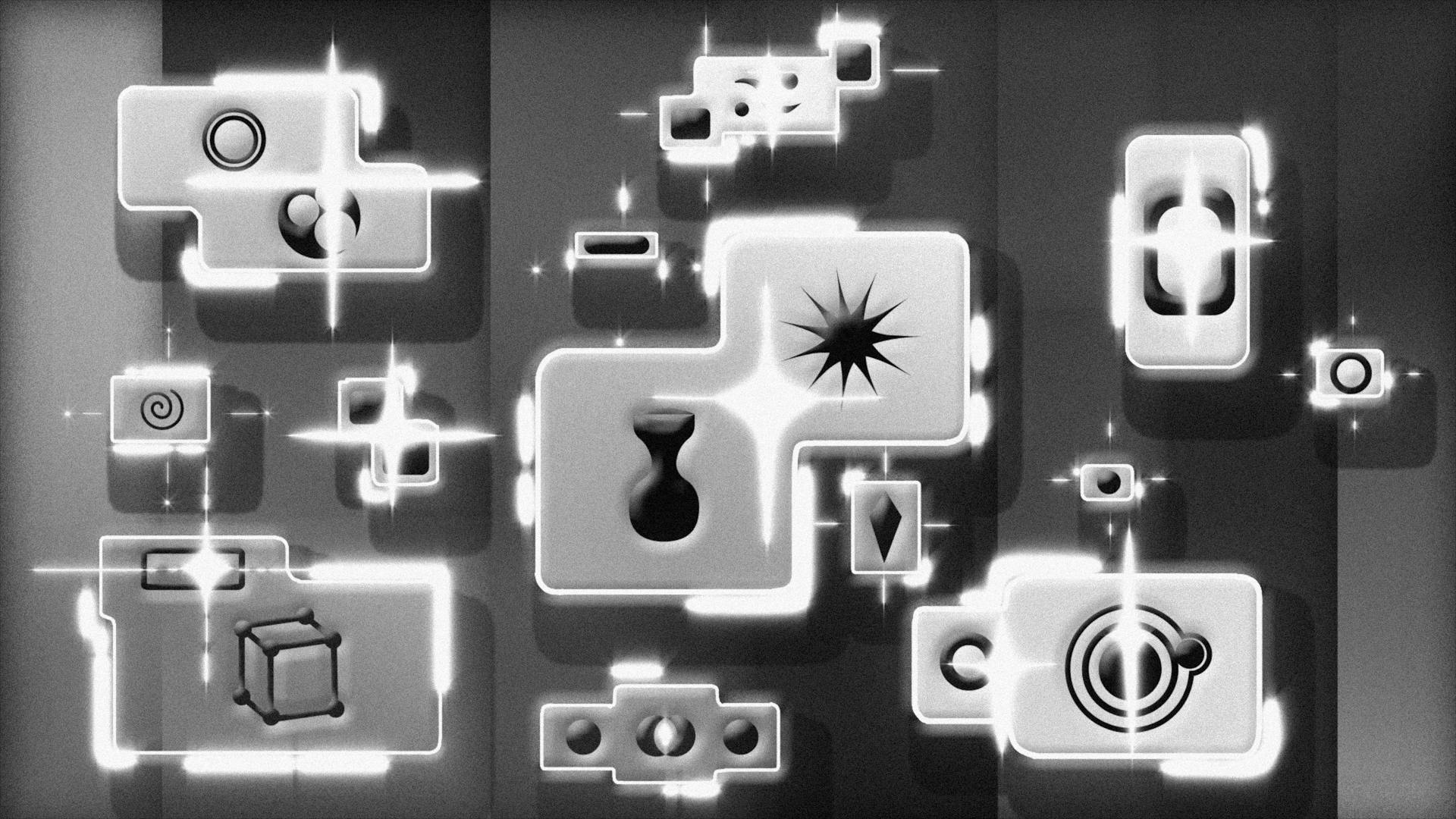
Generative AI is rapidly evolving, and its future looks bright. According to recent advancements, generative AI models can now create realistic images, videos, and music that are almost indistinguishable from human-made content.
One of the most significant opportunities in generative AI is its potential to revolutionize content creation. By automating tasks such as image and video editing, generative AI can free up human creators to focus on high-level tasks that require creativity and originality.
However, generative AI also raises important questions about authorship and ownership. As AI-generated content becomes increasingly prevalent, it's unclear who should be credited as the creator.
For instance, a recent study found that 75% of AI-generated content is indistinguishable from human-generated content. This raises questions about the role of human creators in the content creation process.
If this caught your attention, see: How Generative Ai Can Augment Human Creativity
Types of Generative AI Models
Generative AI models come in different types, each designed for specific tasks and purposes. These types can be broadly categorized into several groups.
Transformer-based models are adept at natural language processing and understanding the structure and context of language. They're well suited for text-generation tasks.
Some examples of transformer-based generative AI models include ChatGPT-4 and Google Gemini, which are trained on large sets of data to understand relationships between sequential information.
Curious to learn more? Check out: What Are the Types of Data in Generative Ai
Variational Autoencoders
Variational autoencoders are a type of generative AI model that use two networks to interpret and generate data. This neural network architecture was first described in 2013.
The encoder in a variational autoencoder takes the input data and compresses it into a simplified format. This is done by identifying the most important characteristics of the data.
For example, variational autoencoders can be used to generate human faces using photos as training data. The program learns how to simplify the photos of people's faces into a few important characteristics.
The decoder then takes this compressed information and reconstructs it into something new that resembles the original data but isn't entirely the same. This process can be repeated multiple times to create a wide range of new faces.
Variational autoencoders can be used to increase the diversity and accuracy of facial recognition systems. By generating new faces, facial recognition systems can be trained to recognize more diverse, less common facial features.
Consider reading: New Generative Ai
Multimodal Models
Multimodal models can understand and process multiple types of data simultaneously, allowing them to create more sophisticated outputs. An example of this is an AI model capable of generating an image based on a text prompt.
DALL-E 3 is a notable example of a multimodal model that can create images based on text prompts. It's a powerful tool that can generate highly realistic images.
OpenAI’s GPT-4 is another example of a multimodal model, capable of processing and understanding multiple types of data.
Check this out: What Are the Two Main Types of Generative Ai Models
Discriminative vs. Generative
Discriminative AI is used for classifying or categorizing data into predefined groups or classes, making it ideal for tasks like sorting emails into spam or non-spam folders.
It learns to distinguish between different types of data by analyzing known data, which allows it to classify new data correctly.
Discriminative AI is particularly useful for identifying patterns in data, such as recognizing objects in an image or diagnosing diseases from medical scans.
A unique perspective: Difference between Generative Ai and Discriminative Ai
In contrast, generative AI is designed to create original content or data based on training data not necessarily directly related to the input it receives.
Generative AI can generate new content, but discriminative AI is used for analyzing and sorting it, making each type of AI useful for different applications.
Most current AI systems are examples of "narrow AI" compared to the concept of general AI, which refers to computer systems and robotics that possess human-like intelligence and autonomy.
You might enjoy: How Are Modern Generative Ai Systems Improving User Interaction
Technical Challenges
Training generative AI models requires large amounts of data and considerable computing power.
This raises questions about accessibility and equality in technology access.
Use Cases
Generative AI is being explored in a number of industry sectors, with varying levels of adoption. McKinsey estimates that activities currently accounting for around 30% of U.S. work hours could be automated by 2030, prompted by the acceleration of generative AI.
Generative AI has found a foothold in healthcare, where it's being used to help accelerate drug discovery and transcribe patient consultations. In fact, tools like AWS HealthScribe allow clinicians to upload important information into their electronic health record.
In digital marketing, generative AI is being used to craft personalized campaigns and adapt content to consumers' preferences. This is especially effective when combined with customer relationship management data.
Some educational tools are beginning to incorporate generative AI to develop customized learning materials. This allows students to learn in a way that suits their individual learning style.
Generative AI is also being used in finance to analyze market patterns and anticipate stock market trends. It's often used alongside other forecasting methods to assist financial analysts.
In terms of specific use cases, generative AI is being used in customer support to help businesses reduce response times and quickly deal with common customer queries. This reduces the burden on staff and allows them to focus on more complex issues.
Generative AI tools are also being used in software development to help developers code more cleanly and efficiently. These tools review code, highlight bugs, and suggest potential fixes before they become bigger issues.
Here are some specific examples of generative AI use cases across different industries:
Generative AI is also being used in customer support, where AI-driven chatbots and virtual assistants can help businesses reduce response times and quickly deal with common customer queries.
Related reading: Generative Ai Customer Experience
Regulation and Ethics
Regulation and Ethics are crucial in the future of generative AI. We need regulation to protect us from the potential misuse of generative AI, such as spreading misinformation or creating autonomous weapons.
Regulators are starting to take notice, with Meta announcing that political ads on Facebook and Instagram will require disclosure if they contain AI-generated content and images. This is a step in the right direction, but we need formal regulations in place.
Generative AI companies may clash with media companies over the use of published work, as their models are often trained on internet-sourced information. IT and cybersecurity professionals need to carefully delineate where the model can and cannot access data.
To ensure responsible use, we need transparency around how generative AI systems are used, as well as ethical guidelines and frameworks in place. This will help prevent the misuse of generative AI and ensure it's used for positive transformation.
Take a look at this: How Generative Ai Will Transform Knowledge Work
Future of Generative AI
Generative AI companies are pushing the envelope by creating higher-parameter models and photorealistic AI video. This is a significant development, as it shows how far the technology has come in a relatively short period.
One potential change generative AI might bring to computing is the use of natural language commands to both find information and command the system. This could revolutionize the way we interact with technology.
OpenAI's release of the o1 model in 2024 is a great example of this trend. The o1 model traded speed for complex coding and math processes, demonstrating the potential of generative AI to tackle complex problems.
For more insights, see: The Economic Potential of Generative Ai the Next Productivity Frontier
Introduction to Technology
Generative AI is a type of technology that uses deep learning to analyze patterns in large datasets and create new data that mimics human-generated content.
At its core, generative AI has a unique ability to learn from vast datasets and produce new and original content. This is made possible by advanced algorithms and deep learning models that allow it to analyze patterns, infer structures, and generate creative or technical outputs.
Curious to learn more? Check out: Generative Ai Content Creation
Generative AI models, such as ChatGPT-4 and Google Gemini, are trained on large sets of data to understand the relationships between sequential information, making them well-suited for text-generation tasks.
These models use neural networks, a type of machine learning process inspired by the human brain, to analyze patterns and replicate them to create new data.
Generative AI is transforming various sectors, including art and design, product development, entertainment and media, and research and development.
Here are some examples of how generative AI is being used in these sectors:
As generative AI gets more sophisticated over time, its outputs become more convincing and human-like, making it an exciting and rapidly evolving field.
Environmental Concerns
Generative AI can increase emissions due to the high energy needs of data centers.
The data centers required to run generative AI have become a key conversation in debates over the Earth's future energy needs.
These data centers rapidly deplete water sources, contributing to environmental concerns.
Generative AI's environmental impact is a pressing issue that needs to be addressed.
Expand your knowledge: Generative Ai Energy Consumption
Future of
Generative AI is on the cusp of a major breakthrough with the potential to revolutionize computing. One potential change is the use of natural language commands to both find information and command the system.
The concept of "agentic" AI, where teams of generative AI "agents" work together to solve complex problems, is often cited as the future of the technology. This is already being explored with models like OpenAI's o1.
Generative AI's capabilities are set to upend decades of "skill-biased" technologies that substituted for routine skills in middle- and low-wage jobs. Instead, generative AI excels at mimicking non-routine skills like programming, prediction, and creativity.
Already, generative AI technologies are capable of performing sophisticated tasks autonomously without human oversight. This includes tasks like programming, writing, and analysis.
The industries that face the greatest exposure to generative AI today are those that were previously ranked at the bottom of automation risk. This is a stark break from previous technologies that mainly disrupted physical, routine, blue-collar work.
Curious to learn more? Check out: Generative Ai Skills
What's at Stake
The future of generative AI is a complex and multifaceted issue, with far-reaching implications for various industries and aspects of our lives.
As we've seen in the article, the rise of generative AI has the potential to automate many tasks, freeing up human time and resources for more creative and strategic pursuits. Generative AI is projected to save businesses $1.5 trillion by 2030, with 70% of organizations planning to adopt AI-powered automation.
The stakes are high, with the potential for significant job displacement and disruption to traditional industries. According to a study, 30% of jobs are at high risk of being automated, with AI expected to replace 20 million jobs by 2030.
However, generative AI also holds the key to solving some of the world's most pressing problems, such as climate change and healthcare disparities. For instance, AI can help optimize energy consumption and reduce waste, leading to a more sustainable future.
Additional reading: Generative Ai and the Future of Work in America
The future of work will be shaped by the adoption of generative AI, with a focus on upskilling and reskilling to remain relevant in a rapidly changing job market. As we've discussed, 85% of executives believe AI will have a significant impact on their organizations, with 60% planning to invest in AI training for their employees.
Ultimately, the future of generative AI will depend on our ability to balance the benefits and risks, ensuring that the technology is developed and used in a responsible and equitable manner.
Capabilities Portend a Break
Generative AI's capabilities are a stark departure from previous workplace technologies. For decades, technology has been "skill-biased", substituting for routine skills in middle- and low-wage jobs, while complementing non-routine skills in higher-paid jobs.
Generative AI upends this paradigm, exceling at mimicking non-routine skills like programming, prediction, writing, creativity, and analysis. This technology is not likely to disrupt physical, routine, blue-collar work, unless there's a breakthrough in robotics.
Worth a look: Roundhill Generative Ai & Technology Etf
Generative AI is capable of performing a wide range of tasks, often quite sophisticated, and at times even without human oversight. Some of the autonomous capabilities include tasks in the following areas:
Generative AI is transforming various sectors, including art and design, product development, entertainment and media, and research and development.
If this caught your attention, see: Generative Ai in Software Development
What Is the Difference Between
Generative AI and regenerative AI serve different purposes. Generative AI is designed for creativity and originality, while regenerative AI focuses on durability and sustainability within AI systems.
Regenerative AI aims to build AI systems that can fix themselves or improve over time without human help. This concept is centered around creating AI systems that can last longer and work more efficiently.
Like generative AI, regenerative AI is still a theoretical concept. It has the potential to help the environment by making smarter decisions that result in less waste.
AI systems that can regenerate themselves are less commonly discussed than generative AI.
Here's an interesting read: Why Is Controlling the Output of Generative Ai Important
Frequently Asked Questions
What is generative AI predictions 2024?
Generative AI and Large Language Models (LLMs) are expected to revolutionize the enterprise in 2024, accelerating data strategies and driving innovation through AI-powered apps. According to Snowflake's 2024 predictions report, this transformation will unlock new revenue streams through data monetization.
Sources
- https://www.forbes.com/sites/bernardmarr/2024/03/05/the-future-of-generative-ai-6-predictions-everyone-should-know-about/
- https://www2.deloitte.com/us/en/pages/consulting/articles/ai-and-the-future-how-ai-is-changing-the-world.html
- https://kaila.eu/blog/the-future-of-generative-ai-pioneering-technological-innovation/
- https://www.techrepublic.com/article/what-is-generative-ai/
- https://www.brookings.edu/articles/generative-ai-the-american-worker-and-the-future-of-work/
Featured Images: pexels.com