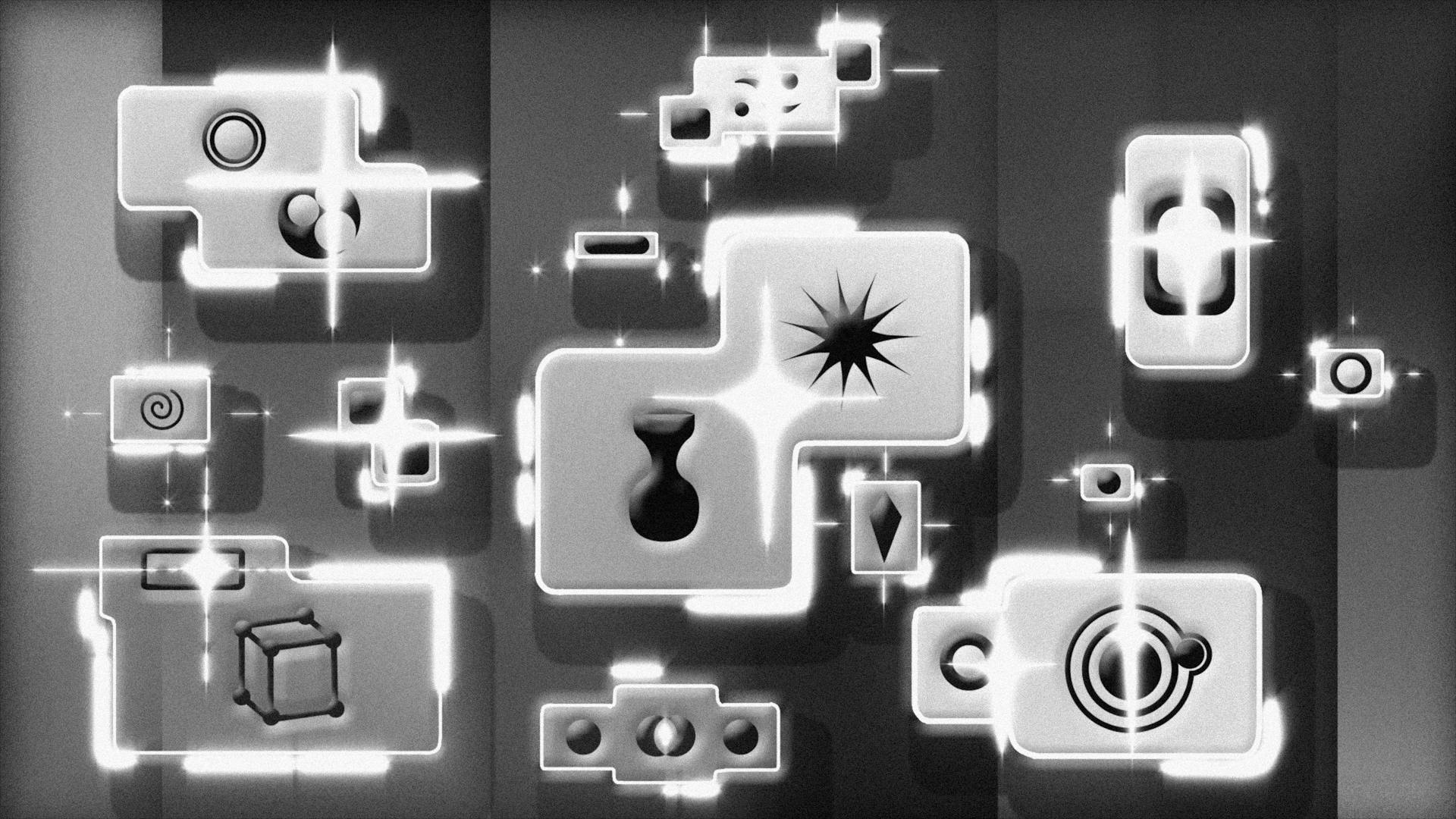
Generative AI is revolutionizing the way we work, and to stay ahead of the curve, it's essential to develop the right skills. The ability to generate high-quality content, such as text, images, and music, is becoming a crucial asset in the job market.
To thrive in the future of work, you'll need to learn how to work with generative AI models, like the ones that can generate realistic human-like faces. These models are being used in various industries, including entertainment and advertising.
Developing essential generative AI skills will not only make you more competitive in the job market but also open up new career opportunities. According to a recent study, 70% of companies plan to use generative AI in the next two years.
Explore further: Generative Artificial Intelligence Market
Top 9 Essential
Generative AI skills are essential for a career in this field. To make a career in generative AI, you must have top skills such as programming and data analysis.
Discover more: Google Cloud Skills Boost Generative Ai
Developing a blend of technical and creative skills is crucial for success in generative AI. Cultivating a blend of these abilities will prepare individuals for the future of work and empower them to innovate and lead.
RAG building, short for Retrieval-Augmented Generation, is a crucial skillset in generative AI. Mastering RAG building is integral for navigating the complexities of AI development.
In the field of generative AI, developers must cultivate a diverse set of skills that encompass both technical and creative aspects. This includes hands-on experience using generative AI tools.
Generative AI skills are not just limited to technical proficiencies, but also include softer skills such as creative thinking and ethical reasoning. These skills are essential for individuals to stay ahead in this dynamic field.
Developers in generative AI must have a detailed overview of essential skills that are crucial for success in this domain. This includes a blend of technical and creative skills.
Generative AI tools can be used to increase efficiency, productivity, and creativity in various industries, including education. This can help save time and make work more engaging for students.
To excel in generative AI, developers must have a diverse set of skills that are practical and applicable to their work. This includes hands-on experience using generative AI tools to solve real-world problems.
A different take: How Generative Ai Is Changing Creative Work
Addressing Challenges
Bias detection and mitigation are crucial skills for generative AI professionals. LLMOps equips you with the tools to detect and mitigate biases in AI outputs, ensuring fairness and ethics.
In the development of machine learning models, TensorFlow and PyTorch are instrumental frameworks that offer a wealth of tools and libraries for model building. These frameworks facilitate collaboration and innovation within the AI community, providing a common platform for sharing models, techniques, and best practices.
To effectively use TensorFlow and PyTorch, you need to balance quality and efficiency, as quantization can sometimes lead to a slight decrease in the quality of generated outputs. However, this trade-off can be managed by finding the right balance between efficiency and accuracy for your specific application.
Data management is a foundational aspect of LLMOps, ensuring that generative AI models have access to high-quality, relevant datasets. This involves collecting, cleaning, and organizing data, as well as making it easily accessible for model training.
Expand your knowledge: Top Generative Ai Tools
Bias mitigation is another critical component of LLMOps, involving identifying and addressing biases in both the data and the generative AI models themselves. Techniques for bias mitigation include diversifying data sources, implementing fairness constraints during model training, and conducting thorough evaluations of model outputs for any signs of bias.
Fine-tuning existing models to adapt to new tasks and challenges is essential for staying ahead in the rapidly evolving field of generative AI.
Check this out: Velocity Model Prediciton Using Generative Ai
From Management to Mitigation
Data management is a foundational aspect of LLMOps, ensuring that generative AI models have access to high-quality, relevant datasets. This involves collecting, cleaning, and organizing data, as well as making it easily accessible for model training.
Proper data management is essential for building effective models that can generate accurate and useful outputs. Bias mitigation is another critical component of LLMOps, involving identifying and addressing biases in both the data and the generative AI models themselves.
Consider reading: Knowledge Management Generative Ai
Techniques for bias mitigation include diversifying data sources, implementing fairness constraints during model training, and conducting thorough evaluations of model outputs for any signs of bias. This is crucial for developing models that produce fair and unbiased outputs.
The journey today in the AI field is as much about understanding the implications of AI as it is about technical mastery. To lead in this space, professionals must also hone their soft skills, such as problem-solving, critical thinking, and effective communication, enabling them to translate complex AI concepts into actionable insights for a broad audience.
The rise of generative AI is reshaping job markets, introducing new opportunities while also phasing out roles that become automated. As businesses integrate AI into their operations, the demand for professionals skilled in generative AI development, management, and ethics is surging.
Explore further: Bias in Generative Ai
Bridging the Gap
Generative AI models can be complex and require technical expertise to use, but AI App Building can make them accessible to a wider audience.
Imagine having a powerful tool hidden away in a dusty workshop – AI App Building is like building a user-friendly interface so anyone can use it to create amazing things.
This is especially true in the field of software development, where generative AI is opening new avenues for innovation. The intersection of technology and creativity is increasingly evident, enabling developers to explore novel approaches to problem-solving.
Generative AI models have a vast potential for various applications, but without user-friendly interfaces, that potential remains untapped. AI App Building allows you to unlock this potential by creating apps that cater to specific needs.
Here are some ways AI App Building can help bridge the gap:
- Creating interfaces that make generative AI models accessible to a wider audience
- Unlocking the potential of generative AI models for various applications
- Enabling developers to create software solutions that are both more efficient and more intuitive
As technology continues to advance, embracing the synergy between technical prowess and creative thinking will be key to unlocking the full potential of AI.
Continuous Adaptation
Continuous adaptation is key to staying ahead in the rapidly evolving field of generative AI. The field of generative AI is constantly evolving, and fine-tuning empowers you to adapt existing models to new tasks and challenges as they emerge.
To stay updated with the latest trends and technologies, developers must participate in online courses and workshops, engage with the AI community through forums and conferences, and read research papers and official documentation. This pursuit of knowledge enables individuals to adapt their skills to the evolving demands of the AI job market.
Effective prompts play a crucial role in the fine-tuning process, helping AI models to better understand the task and refine their predictions. By designing effective prompts, professionals can guide the AI model's learning process, enhancing its versatility and generalization capabilities.
The balance between specialization and adaptability is essential for staying ahead in the field. Specialization involves customizing models for specific tasks, ensuring they can handle particular challenges efficiently, while adaptability prepares the model to perform well under a variety of conditions.
To stay ahead of AI advancements and market demands, professionals must engage in ongoing education to keep pace with the latest developments in AI technology, methodologies, and ethical standards. This proactive approach to learning involves online courses, forums, and workshops, as well as building a strong professional network through collaborative projects and conferences.
Here are some essential skills for continuous adaptation:
- Participating in online courses and workshops
- Engaging with the AI community through forums and conferences
- Reading research papers and official documentation
- Building a strong professional network through collaborative projects and conferences
By mastering these skills, developers can effectively contribute to the advancement of generative AI technologies and applications, and stay ahead in the rapidly evolving field.
Handling
Handling data effectively is crucial in addressing challenges in AI development. Data is the backbone of generative AI, and developers must be adept at various techniques.
Data collection is a fundamental skill, requiring developers to gather data from various sources. This can involve web scraping, API calls, or even manual data entry.
Data preprocessing is a critical step, where developers clean and prepare data for analysis. This includes normalization, transformation, and handling missing values.
Data visualization is essential for understanding data trends and insights. Tools like Matplotlib and Seaborn can help developers create informative and engaging visualizations.
Developers should be familiar with data handling skills to effectively address challenges in AI development. By mastering data collection, preprocessing, and visualization, developers can unlock valuable insights and improve their AI models.
For more insights, see: Generative Ai Data Visualization
Efficiency and Optimization
Effective prompts can significantly reduce the trial-and-error process, saving time and resources.
Clear communication of intent is key to getting the results you want in fewer attempts. By providing well-crafted prompts, you can avoid the frustration of repeated failures.
Consider reading: Advanced Coders - Ai Training
Reducing the training time required to achieve good results is crucial for any generative AI project. Fine-tuning a pre-trained model can save a significant amount of time and resources compared to training a model from scratch.
Stable diffusion plays a key role in maximizing the creativity and efficiency of generative AI projects. By ensuring the stability of the learning process, developers can produce diverse and high-quality content.
The ability to navigate the complexities of stable diffusion requires a deep understanding of the underlying principles of generative models. This involves adjusting model parameters and training processes to maintain the delicate balance required for stable diffusion.
Streamlining operations with LLMOps is crucial for enhancing the efficiency and scalability of generative AI models. By automating the lifecycle of these models, teams can reduce the complexity and time involved in managing large language models.
Check this out: Geophysics Velocity Model Prediciton Using Generative Ai
Advanced Techniques
Fine-tuning generative AI models involves adjusting parameters and retraining the model on specific datasets. This process can be done using various techniques such as Transfer Learning, which utilizes pre-trained models and adapts them to specific tasks or domains by training on a smaller, task-specific dataset.
Data Augmentation is another technique that enhances the training dataset by creating variations of existing data, which can help the model generalize better. Hyperparameter Optimization is also crucial, where you experiment with different hyperparameters to find the optimal settings that improve model performance.
To gain hands-on experience with generative AI, you can participate in labs offered by Google Cloud. These labs provide a chance to work directly with generative AI models and explore their capabilities. Some popular labs include the Generative AI Studio, Generative AI with Vertex AI: Getting Started, and Prompt Design using PaLM.
To navigate the complexities of stable diffusion, you need to understand techniques used to maintain the stability of generative models while they learn to replicate complex data distributions. This involves leveraging frameworks such as TensorFlow and PyTorch, which provide a wealth of tools and libraries that streamline the process of model building.
Take a look at this: Google Labs Generative Ai
Redefining Creative Processes
Generative AI is transforming creative endeavors by providing tools that can generate novel ideas, simulate artistic techniques, and even create new forms of art. This technology is not replacing human creativity but is instead serving as a catalyst for innovation, allowing creators to explore new realms of possibility.
By automating aspects of the creative process, AI enables artists, designers, and writers to focus on the conceptual and experimental facets of their work. This synergy is fostering a new era of creativity, characterized by a blend of human intuition and AI's computational power.
The collaboration between humans and AI in creative fields is yielding results that neither could achieve alone. This synergy is fostering a new era of creativity, characterized by a blend of human intuition and AI's computational power.
The future of creative processes lies in the hands of those who can integrate AI into their work, leveraging its potential to enhance human creativity rather than diminish it. This integration marks a significant shift in how art is made and experienced, highlighting the endless possibilities that arise when human ingenuity and machine intelligence converge.
Here are some examples of how AI is redefining creative processes:
- Creating unique content formats like poems based on historical events or fictional news articles
- Generating novel ideas, simulating artistic techniques, and creating new forms of art
- Enhancing human creativity through AI's computational power and automation of creative tasks
- Fostering a new era of creativity through the synergy between human intuition and AI's computational power
Model Building Frameworks
Model Building Frameworks are instrumental in the development of machine learning models. TensorFlow and PyTorch are two popular frameworks that offer a wealth of tools and libraries to streamline the model building process.
TensorFlow and PyTorch provide the technical capabilities needed to develop advanced machine learning models. These frameworks are used by machine learning and deep learning professionals to experiment with neural networks, test new architectures, and optimize performance.
The flexibility and power of TensorFlow and PyTorch make them indispensable for cutting-edge AI research and development. They facilitate collaboration and innovation within the AI community by providing a common platform for sharing models, techniques, and best practices.
Here are some key features of TensorFlow and PyTorch:
These frameworks are crucial for anyone working in AI, as they not only provide the technical capabilities needed to develop advanced machine learning models but also foster a culture of innovation and collaboration. By mastering these tools, professionals can contribute to the rapid evolution of AI technologies, driving forward the future of tech innovation.
A fresh viewpoint: Generative Ai Innovation Center
Maintaining Smooth Running
LLMOps is like having a pit crew for your LLM, ensuring it's always tuned-up and avoids breakdowns. You can identify issues like biases in outputs, performance bottlenecks, or data errors, and take corrective actions.
Monitoring your LLM is crucial to catching problems early. LLMs are complex systems, and LLMOps provides the skills to manage them effectively.
To keep your LLM running optimally, you need to manage its data pipeline efficiently. LLMs are data-hungry beasts, and LLMOps helps you optimize data pipelines to avoid inefficiencies.
High-quality, relevant data is essential for training your LLM. Ensuring the LLM is trained with good data can make all the difference in its performance.
For more insights, see: Is Llm a Type of Generative Ai
Frequently Asked Questions
What is required to learn generative AI?
To learn generative AI, you need a solid understanding of neural networks, including CNNs and RNNs, and familiarity with popular deep learning frameworks like TensorFlow, Keras, and PyTorch. Mastering these fundamentals is essential for unlocking the power of generative AI.
What are the techniques of generative AI?
Generative AI uses deep learning, neural networks, and machine learning to create content. These techniques enable computers to learn from patterns and trends in data to produce human-like output.
What is an example of generative AI?
Jasper Campaigns is an example of generative AI, which can create tailored content for various communication channels with just a single brief. This AI-powered tool streamlines content creation for marketing efforts.
What does generative AI do?
Generative AI models create new content by transforming inputs from one format into another, such as text to image or video to text. This technology can generate a wide range of creative outputs, from images and music to written text and more.
Sources
- https://www.analyticsvidhya.com/blog/2024/03/generative-ai-skills/
- https://www.netguru.com/blog/generative-ai-skills
- https://www.restack.io/p/generative-ai-docs-answer-skills-list-cat-ai
- https://grow.google/ai-for-educators/
- https://cloud.google.com/blog/topics/training-certifications/new-generative-ai-trainings-from-google-cloud
Featured Images: pexels.com