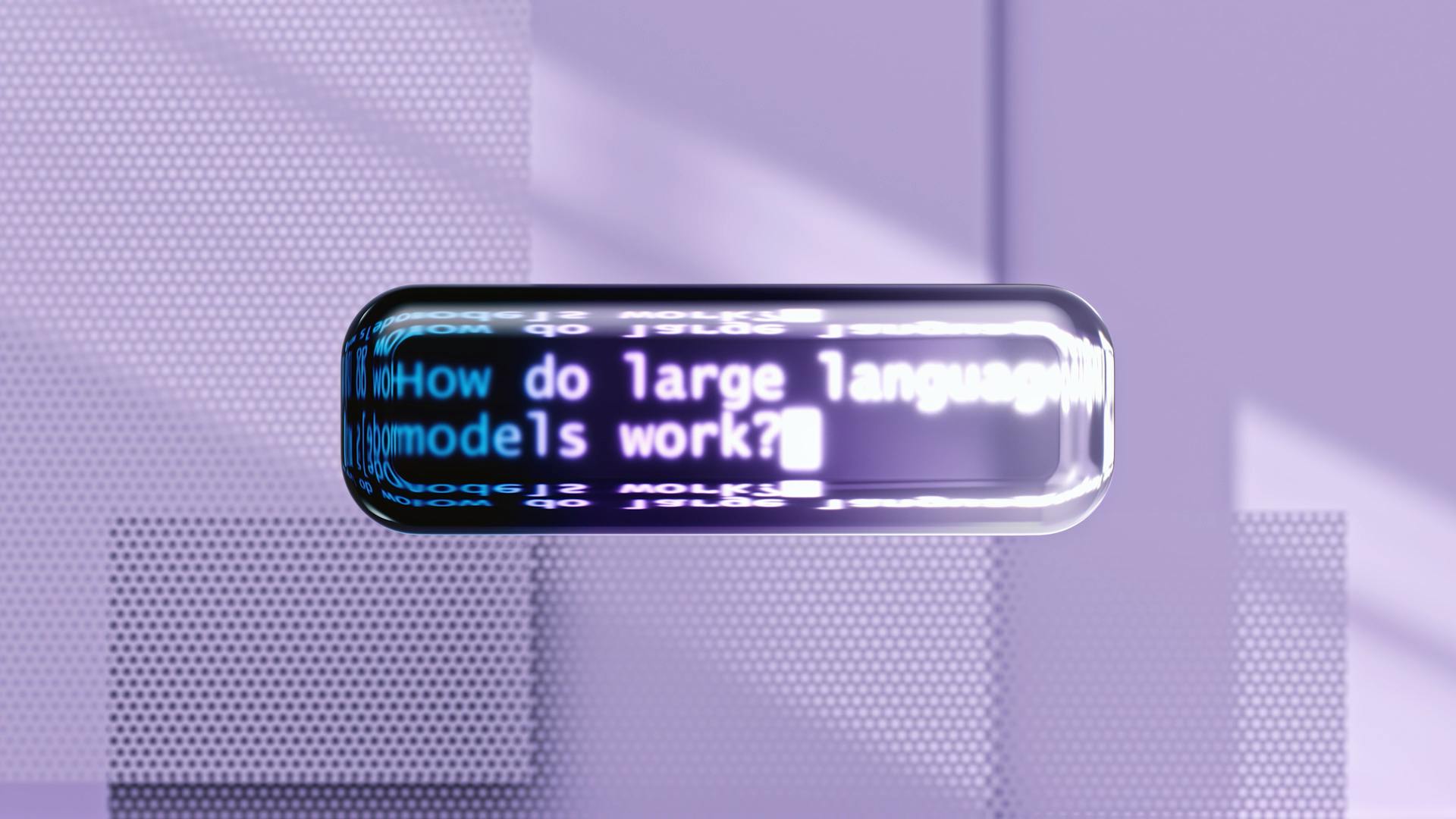
Generative AI is revolutionizing the way we manage knowledge, making it more efficient and accessible than ever before. According to a study, generative AI can process and analyze large amounts of data in a matter of seconds, reducing the time it takes to find relevant information from hours to minutes.
This newfound efficiency has significant implications for businesses and organizations, enabling them to make data-driven decisions faster and more accurately. By leveraging generative AI, companies can automate routine tasks and free up human resources to focus on higher-value activities.
One notable example of generative AI's impact on knowledge management is its ability to summarize lengthy documents and reports, distilling complex information into actionable insights. This is particularly useful for professionals who need to quickly grasp the essence of a project or proposal.
On a similar theme: How Generative Ai Will Transform Knowledge Work
What is Generative AI?
Generative AI is a type of artificial intelligence that can create new content, such as text, images, or music, based on a given prompt or pattern.
It uses complex algorithms and machine learning models to generate new and original content, often mimicking human-like creativity.
See what others are reading: New Generative Ai
Generative AI Basics
Generative AI is a type of AI that can create new content such as images, music, and text.
It uses complex algorithms to generate new data that is similar in style and structure to existing data.
Generative AI models are trained on large datasets, which allows them to learn patterns and relationships within the data.
These models can then use this knowledge to generate new content that is unique but still coherent and relevant.
Generative AI has many potential applications, including art, music, and even writing.
A fresh viewpoint: Generative Ai Music
How it Works
Generative AI uses complex algorithms to analyze vast amounts of data and identify patterns, allowing it to generate new content that's similar in style and structure to the original.
These algorithms are trained on large datasets, which can include text, images, or audio, and learn to recognize the underlying rules and relationships between the data points.
Generative AI can then use this knowledge to create new, unique content that's tailored to a specific context or purpose.
Here's an interesting read: Generative Ai Content
For example, a generative AI model might be trained on a dataset of images of cats, and then use that training to generate new images of cats that are similar but not identical.
This process involves a combination of machine learning and natural language processing (NLP) techniques, which enable the AI to understand and manipulate the underlying structure of the data.
The output of generative AI can take many forms, including text, images, audio, or even entire videos.
Generative AI has many potential applications, including content creation, data augmentation, and even artistic collaboration.
A fresh viewpoint: Generative Ai by Getty Images
Benefits and Improvements
Generative AI improves knowledge management in several key ways, including creating, finding, and managing information more efficiently.
Having instant access to a company's knowledge makes many tasks simpler, especially in complex enterprise knowledge management systems. This is because all of a company's knowledge is easily available from one point through an intuitive chatbot interface.
Fast access to organizational knowledge streamlines daily operations for all employees, eliminating tedious searching for information. This means more effective customer support, as customer service agents can find the correct answers to customer queries, regardless of the database size.
For your interest: Generative Ai for Customer Experience
The resolution time of tickets decreases, and customer satisfaction goes up, as customer service agents can quickly find the information they need. This also benefits sales teams, who can access up-to-date knowledge about products or services to answer potential clients' questions.
Having all documents, internal procedures, and regulations in one, easily searchable place helps new employees get trained and onboarded faster and more efficiently. This is a huge help for every newbie.
Maintenance technicians can perform intelligent searches for technical information needed on tablets connected to the company's knowledge base, streamlining the maintenance process of different machinery. This is especially useful for maintenance technicians working in the field.
Establishing metrics to evaluate the success of the AI-driven knowledge management system is critical, including user engagement rates, information retrieval accuracy, user satisfaction scores, and the impact on decision-making and innovation. Regular reviews of these metrics can inform iterative improvements to the system.
Readers also liked: Generative Ai for Customer Support
Concerns and Challenges
Implementing knowledge management can be a daunting task, especially for enterprises. Enterprises face specific challenges when it comes to knowledge management, such as navigating the complexities of their own data.
Data governance, privacy, and security are major concerns, as organizations must adhere to data protection regulations and ethical guidelines. This requires a thoughtful approach to implementing knowledge mining.
The opportunities presented by knowledge mining are immense, including enhancing organizational knowledge, improving efficiency, and driving growth.
Consider reading: Generative Ai for Data Management
Concerns about Hallucinations
Hallucinations are a concern in knowledge management, especially when users prompt generative AI to write about general knowledge. This can lead to inaccurate responses that sound credible but have no factual basis.
After ChatGPT's public launch in 2022, users noticed the tool often gave them inaccurate responses to questions, also known as AI hallucinations. These responses can be detailed but still have no factual basis to support them.
The potential for hallucinations occurs most often when users prompt the tool to write about broad topics, like a marketer for a vitamin company writing blog posts on nutrition. In such cases, the risk of hallucinations is higher because the websites where LLMs get information may contain conflicting or outdated information.
Organizations that use generative AI in knowledge management can reduce the risk of hallucinations by training the tools on their own internal knowledge. This approach reduces the reliance on external information and minimizes the chances of hallucinations.
Expand your knowledge: When Was Generative Ai Open Source
Challenges in Enterprises
Enterprises face specific challenges in knowledge management, including a lack of a centralized platform for sharing information, as seen in Fig. 1. This can lead to knowledge silos and inefficient use of resources.
Implementing knowledge mining requires a thoughtful approach to data governance, privacy, and security, as organizations must adhere to data protection regulations and ethical guidelines. Organizations must balance the benefits of knowledge mining with the need to protect sensitive information.
Data protection regulations and ethical guidelines are crucial for knowledge mining, as organizations must ensure that they are using data responsibly. This involves not just technological solutions but also organizational policies and practices designed to safeguard data and ensure its ethical use.
Knowledge mining is not just about analyzing data, it's about transforming data into a strategic asset, which requires a thoughtful approach to data governance, privacy, and security. This can be a complex task, but it's essential for navigating the complexities of the modern business environment.
Intriguing read: Generative Ai Not Working Photoshop
Implementation and Integration
Implementing generative AI in knowledge management requires careful consideration of various factors. This includes transforming knowledge bases into dynamic resources, indexing vast amounts of data, and training the AI on organizational content.
Organizations must ensure that they adhere to data protection regulations and ethical guidelines while harnessing the power of their data. Implementing knowledge mining requires a thoughtful approach to data governance, privacy, and security.
To integrate generative AI with knowledge bases, a company needs to possess capabilities in natural language processing, data science, and machine learning operations, as well as IT infrastructure and IT security. This can be done by integrating the language model of a third-party provider, such as ChatGPT, with the knowledge bases.
Deploying RAG within an organization's knowledge management system involves careful consideration of data sources, indexing strategies, and the training of the AI model to ensure relevance and accuracy. Privacy and security measures must be paramount, given the sensitive nature of the information being processed.
Intriguing read: Are Large Language Models Generative Ai
Automates Governance Processes
Automating governance processes is a game-changer for organizations.
Generative AI can automate and speed up the process of checking knowledge articles for personally identifiable information, freeing up human teams to focus on more important tasks.
Instead of having a person manually review articles, an AI tool can apply an engineered prompt to flag any suspicious information, such as social security numbers or phone numbers, and prevent publication until a human reviewer gives the green light.
This automated governance check can help organizations make knowledge available more quickly, which is especially important in today's fast-paced business environment.
Articles that pass the automated governance check can skip the manual review process, streamlining the knowledge management process and reducing the risk of human error.
For your interest: Generative Ai Governance
Integration Across Platforms
The integration of AI-driven knowledge management systems across various work platforms and tools will become more seamless, ensuring that knowledge access is not siloed but ubiquitously available, regardless of the digital environment employees are working in.
This seamless integration will allow employees to access knowledge from anywhere, at any time, making it accessible whenever and wherever it's needed.
Organizations can achieve this by combining Generative AI with various knowledge bases, as mentioned in Example 6, which requires knowledge of natural language processing, data science, and machine learning operations, as well as IT infrastructure and IT security.
The integration of AI-driven knowledge management systems across platforms will enable knowledge to flow freely across organizational boundaries, making it accessible whenever and wherever it's needed.
This can be achieved by using a combination of Generative AI for natural language processing and interaction, along with RAG for enhanced retrieval and generation, as mentioned in Example 9.
Here are some key components to consider when integrating AI-driven knowledge management systems across platforms:
- Azure components, such as Chatbot APP, Azure Container Apps, Azure OpenAI, Azure AI Search, Azure Storage Account, Azure Functions, Azure Document Intelligence, and Azure Monitor, can be used to build a knowledge management AI solution, as shown in Figure 6 in Example 15.
- Organizations should consider the types of data they deal with, the desired functionalities of the system, and the integration capabilities with existing IT infrastructure when selecting the right technologies.
- Seamless integration across platforms will enable knowledge to flow freely across organizational boundaries, making it accessible whenever and wherever it's needed.
Features and Solutions
Generative AI can speed up the writing process, transforming bits of information into full-length articles. This automation lets employees like customer service and support agents build up their organization's knowledge base as they take customer calls and resolve tickets.
With generative AI, employees can create knowledge base articles from notes on service desk tickets, making the process more efficient. This leads to more complete knowledge bases, which helps customers and agents resolve problems more quickly in the future.
Users can ask complex, nuanced questions and receive answers generated based on a wide array of relevant information. This capability makes the interaction with the system more natural and effective, closely resembling a conversation with a knowledgeable human colleague.
Best Practices and Considerations
To deploy Generative AI in your knowledge management system, consider carefully indexing vast amounts of unstructured and structured data.
Data sources and indexing strategies are crucial for ensuring relevance and accuracy in your AI model. This involves transforming your knowledge bases into dynamic resources that are more accessible and useful to employees.
Privacy and security measures must be paramount, given the sensitive nature of the information being processed. This is a critical consideration for organizations deploying Retrieval-Augmented Generation within their knowledge management systems.
Worth a look: Generative Ai for Product Management
User feedback is invaluable for refining the AI model, ensuring that it continually improves in delivering precise and useful responses. In fact, user interactions and feedback are key to continuously refining the system based on user interactions and feedback.
By prioritizing data quality, indexing strategies, and user feedback, you can create a Generative AI-driven knowledge management system that actively facilitates information discovery and use. This transforms the quest for information from a mere retrieval process into an organisational process and culture of discovery.
You might enjoy: Generative Ai Drug Discovery
The Future of
The Future of Knowledge Management is here, and it's driven by AI. AI-driven knowledge management is a paradigm shift that redefines how organizational knowledge is captured, accessed, and utilized.
AI is not just an incremental improvement over existing systems, but a game-changer that will revolutionize the way we work with knowledge. The future of knowledge management with AI is not just about automating tasks, but about unlocking new possibilities and improving decision-making.
Organizational knowledge will be captured more efficiently, and accessed more easily, thanks to AI-driven systems. This will enable teams to work more collaboratively and make better decisions, faster.
Broaden your view: How Are Modern Generative Ai Systems Improving User Interaction
Frequently Asked Questions
Is NLP part of generative AI?
NLP is not a subset of generative AI, but rather a complementary technology that will likely evolve alongside it to create more powerful systems. The future of NLP and generative AI is intertwined, enabling more human-like interactions and applications.
Sources
- https://www.techtarget.com/searchcontentmanagement/feature/How-generative-AI-can-improve-knowledge-management
- https://www.techtarget.com/searchcontentmanagement/opinion/Generative-AIs-role-in-the-rebirth-of-knowledge-management
- https://comma-soft.com/en/case/knowledge-management-with-generative-ai/
- https://fabrity.com/blog/generative-ai-in-knowledge-management-a-practical-solution-for-enterprises/
- https://www.sumoanalytics.ai/post/transforming-knowledge-management-with-generative-ai-uniting-chat-and-search-for-deeper-insight
Featured Images: pexels.com