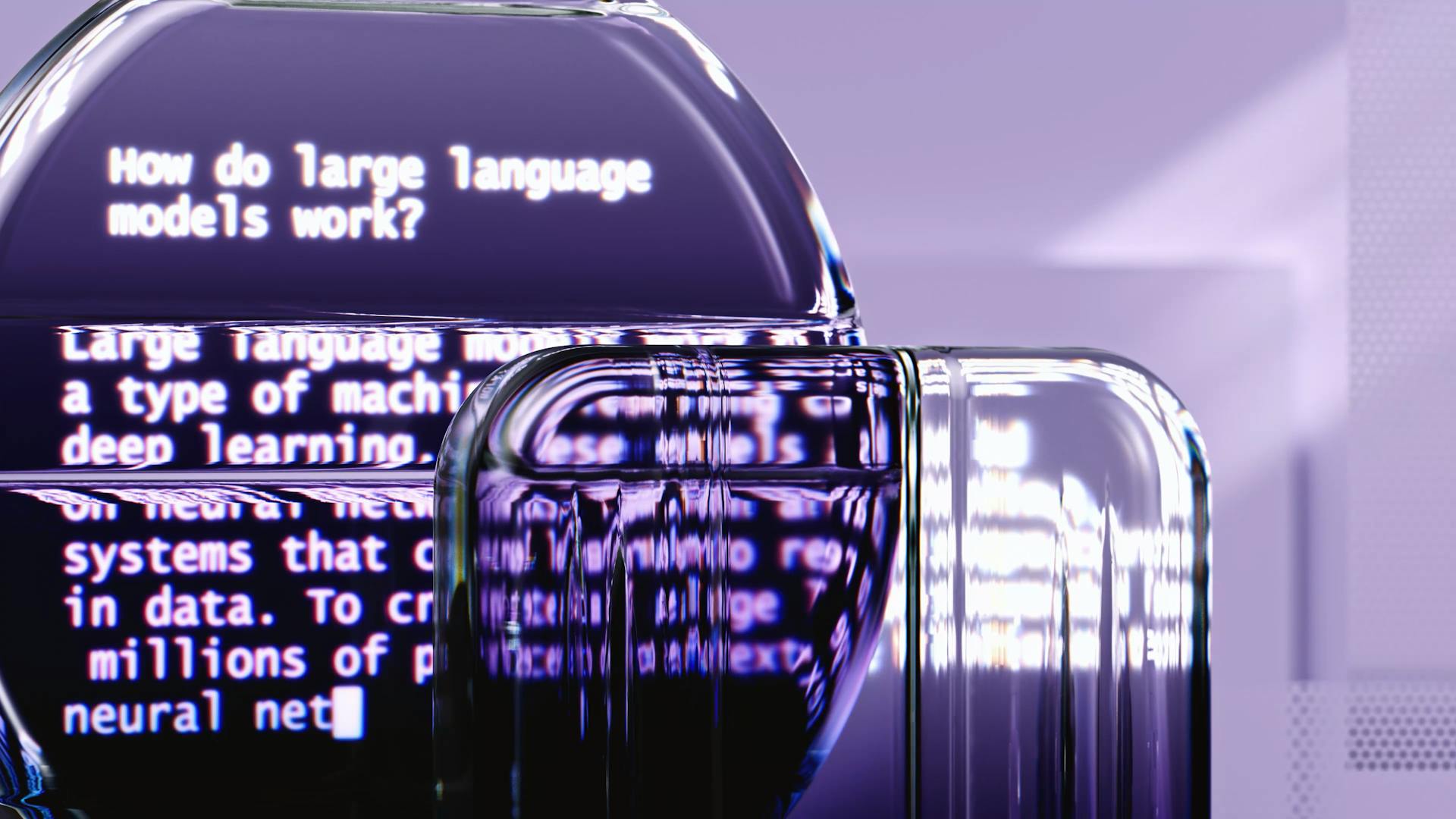
Generative AI can automatically generate new data that looks and feels like real data, making it a game-changer for data management.
By leveraging generative AI, organizations can create synthetic data that is indistinguishable from real data, allowing for more accurate testing and training of machine learning models.
This approach can significantly reduce the time and cost associated with collecting and preparing real-world data, making it a more efficient and cost-effective solution.
With generative AI, data scientists can focus on higher-level tasks, such as developing and refining models, rather than spending hours collecting and preprocessing data.
Check this out: Generative Ai in Real Estate
Generative AI for Data Management
Generative AI for Data Management is revolutionizing the way we work with data. It can detect anomalies in data, ensuring data quality, and identify patterns indicative of anomalies.
Automated data profiling becomes more effective with Large Language models, providing insights into the structure and quality of data. This empowers organizations to make informed decisions about data usage and maintenance.
Additional reading: Generative Ai Photoshop Increase Quality
Large Language models contribute to metadata enrichment by analyzing unstructured data sources, automatically extracting relevant metadata. This enriches the information available in the data catalog and makes it more comprehensive.
Generative AI can uncover relationships that typical algorithms might miss, revealing that a dip in sales is linked not only to seasonality but also to subtle shifts in consumer behavior. It can then convert insights into easy-to-understand visualizations, generating graphs, charts, or interactive dashboards.
The generation of visualizations and chart elements can be achieved based upon auto-prompt suggestions from a BI tool's native AI capabilities. This can be a significant timesaver in the creation of new dashboards and refining existing visualizations that have grown to be less intuitive over time.
Natural Language Querying
Natural Language Querying is a game-changer for data management. It enables business users to query data in natural language without needing special skills. This democratizes data access and empowers users to make informed decisions.
See what others are reading: Generative Ai with Large Language Models
The Denodo Platform is a great example of this in action. It allows users to query data in natural language and even recommends metadata to aid in self-service data preparation.
Large Language Models (LLMs) take this a step further by analyzing descriptions and providing insights into the structure and quality of data. This is particularly useful for automated data profiling, which becomes more effective with LLMs.
In fact, LLMs can even analyze unstructured data sources to automatically extract relevant metadata. This enriches the information available in the data catalog and makes it more comprehensive.
Here are some key benefits of Natural Language Querying:
- Enables business users to query data in natural language
- Recommends metadata for self-service data preparation
- Analyzes descriptions to provide insights into data structure and quality
- Automatically extracts relevant metadata from unstructured data sources
By leveraging these capabilities, organizations can make data more accessible and usable for everyone. This is a major step forward in data management and will have a significant impact on how we work with data in the future.
Auto-Generation of Visualizations and Dashboard Layouts
Generative AI can significantly reduce the time spent on creating new dashboards and refining existing visualizations. This is achieved through auto-prompt suggestions from a Business Intelligence (BI) tool's native AI capabilities.
The generation of visualizations and chart elements can be done automatically, streamlining the process of creating new dashboards and refining existing ones. This can be a game-changer for data analysts and professionals who spend a lot of time on data visualization.
By leveraging the same AI capabilities, the same visual aesthetics can be applied to the construction of an entire report layout. This ensures consistency and coherence in the presentation of data.
Auto-generation of visualizations and dashboard layouts can save a significant amount of time, allowing professionals to focus on higher-level tasks and more complex data analysis.
Related reading: Generative Ai Synthetic Data
Benefits and Features
The Denodo Platform makes your organization GenAI-ready by automating tedious tasks and providing actionable insights.
Generative AI is more than just another tool in the data management toolbox – it's a high-powered engine that brings in the ability to handle routine processes and provide intelligent autocompletion, proactive suggestions, and optimization recommendations.
Denodo Assistant, a core component of the Denodo Platform, leverages AI and machine learning to automate routine processes, providing intelligent autocompletion, proactive suggestions, and optimization recommendations.
Bad data derails entire projects, but generative AI in data management keeps that from happening by automating data validation and ensuring that what goes into your system is clean.
Data management traditionally calls for many repetitive processes, but generative AI in data management automates these tasks, automatically tagging incoming data based on context or creating complex data transformations without needing to write a single line of code.
DataFactory introduces a transformative feature known as GenAI Transformation, offering a novel approach to data transformation by enabling users to input transformation logic in natural language and select the code type to be generated.
Through advanced natural language processing and machine learning algorithms, generative AI can help users ask complex questions about their data in a more intuitive and natural language format to interact with their data more effectively.
Readers also liked: Can I Generate Code Using Generative Ai Models
Implementation and Challenges
Integrating Generative AI into data management practices is a significant undertaking, and it's not without its challenges. This transformative technology changes data processes, but it's not without its share of ethical quandaries.
Here's an interesting read: Generative Ai Not Working Photoshop
The implementation of Generative AI in data management is turbulent, to say the least. It requires a shift in how businesses handle information assets.
Businesses need to consider the potential consequences of using Generative AI, including the risk of biased or inaccurate data. This can have serious implications for decision-making and operations.
Generative AI changes data processes, but it's essential to have a plan in place to manage these changes effectively.
Real-World Applications
Generative AI is transforming the way organizations handle their data, with businesses across various industries improving efficiency and decision-making. This technology is being leveraged in real-world applications to drive tangible results.
Walmart is a prominent example of this, using Generative AI to optimize inventory and supply chain operations. By analyzing historical sales data and external factors like weather, AI models accurately predict inventory needs.
By doing so, Walmart reduced excess stock, improved product availability, and enhanced customer satisfaction while minimizing waste. This achievement showcases the potential of Generative AI in data management to drive business success.
Explore further: Walmart Generative Ai
Changing to Visualizations
Generative AI is changing the way we work with data, and one of the most exciting applications is turning insights into visualizations.
By analyzing massive datasets, generative AI can uncover relationships that typical algorithms might miss, revealing insights that are both surprising and actionable.
It can also convert insights into easy-to-understand visualizations, generating graphs, charts, or interactive dashboards that make complex data accessible to anyone.
This is a significant timesaver in the creation of new dashboards and refining existing visualizations that have grown to be less intuitive over time.
Generative AI can auto-generate visualizations and chart elements based on auto-prompt suggestions from a BI tool's native AI capabilities.
This means that the same visual aesthetics can be applied to the construction of an entire report layout, making it easier to create new dashboards and refine existing ones.
Through its ability to analyze and interpret vast amounts of data, generative AI can identify key patterns, trends, and relationships within datasets that can then be translated into visually appealing charts, graphs, and other visualizations.
This ensures that the visualizations are not only informative but also engaging and impactful, enhancing the overall user experience.
Recommended read: New Generative Ai
Retail Supply Chain Enhancement
Generative AI in data management is transforming the retail industry by optimizing inventory and supply chain operations.
Walmart is a prominent example of this, leveraging Generative AI to analyze historical sales data and external factors like weather to accurately predict inventory needs.
This approach has helped Walmart reduce excess stock, improve product availability, and enhance customer satisfaction while minimizing waste.
Generative AI can simulate various business scenarios to show what would happen if prices were adjusted or a new product was launched, allowing retailers to make informed decisions.
By presenting complex data in user-friendly formats like simple charts and graphs, Generative AI makes it easier for retailers to comprehend the findings and teach others, leading to better decision-making.
Retailers can also personalize visualizations to cater to different user groups' specific needs and preferences, ensuring the visualizations are informative, engaging, and impactful.
This technology has the power to change how retailers consume and present data, making it easier to identify key patterns, trends, and relationships within datasets.
Suggestion: Generative Ai for Product Management
Real-World Examples of Transformation
Generative AI is transforming data management in real-world applications across various industries. Businesses are improving efficiency and decision-making by leveraging this technology.
Walmart is a prominent example of a company that has successfully implemented Generative AI in data management. They used AI models to analyze historical sales data and external factors like weather to accurately predict inventory needs.
By doing so, Walmart reduced excess stock, improved product availability, and enhanced customer satisfaction while minimizing waste. This is a significant achievement that showcases the potential of Generative AI in data management.
Auto-generation of visualizations and dashboard layouts is another area where Generative AI is making a significant impact. This technology can generate visualizations and chart elements based on auto-prompt suggestions from a BI tool's native AI capabilities.
The same visual aesthetics can be applied to the construction of an entire report layout leveraging the same capabilities. This can be a significant timesaver in the creation of new dashboards and refining existing visualizations that have grown to be less intuitive over time.
On a similar theme: Roundhill Generative Ai & Technology Etf
Generative AI can also transform data transformation by automating the process. Large language models can generate code snippets based on natural language descriptions, accelerating the transformation process and enabling users to describe transformations in a more human-readable manner.
By uncovering relationships that typical algorithms might miss, Generative AI in data management can reveal hidden insights and patterns in data. This can help businesses make more informed decisions and improve their overall performance.
For more insights, see: Which Term Describes the Process of Using Generative Ai
Healthcare - Patient Enhancement
In the healthcare industry, AI is revolutionizing patient care by enhancing data integration. Mayo Clinic uses AI to unify complex data types from multiple sources, such as lab results and medical histories.
This integration enables accurate diagnoses and personalized treatment plans. As a result, patients receive better care and outcomes.
Generative AI models in data management are crucial in this process, allowing for the unification of disparate data types. This leads to more effective treatment plans and improved patient experiences.
By leveraging AI, healthcare providers can streamline patient data management and make more informed decisions. This ultimately benefits patients and drives better health outcomes.
Intriguing read: Generative Ai and Knowledge Management
Co-Pilot Ask Albus
Co-Pilot Ask Albus is a game-changer for data exploration. It's a feature driven by GenAI that empowers users to ask natural language questions directly to the data product, facilitating rapid insights without the necessity of creating dashboards or reports.
Ask Albus is versatile and can be utilized for obtaining insights on structured, semi-structured, or unstructured data. This means you can use it to analyze a wide range of data sources, from spreadsheets to PDFs.
To cater to diverse architectural requirements, DataMarket offers two distinct templates for Ask Albus. One template utilizes OpenAI as the Large Language Model (LLM), while the second employs a local LLM named Mistral. This flexibility ensures that Ask Albus can adapt to your specific needs.
The Ask Albus feature operates on structured or semi-structured data with a reference architecture that involves prompt engineering, driven by LangChain, to produce an optimized prompt. This prompt is then sent to OpenAI through a rest-API to generate SQL/Python code that answers the user's question.
You might enjoy: What Is a Prompt in Generative Ai
Ask Albus can also operate on unstructured data, such as PDFs, Excel, or Word documents, utilizing Mistral Local LLM. This means you can derive insights from a wide array of data sources, regardless of their structure.
DataMarket's Ask Albus feature is designed to unlock intuitive data exploration, making it easier for users to get the insights they need without requiring extensive technical knowledge. With Ask Albus, you can ask natural language questions and get answers in a user-friendly format.
Additional reading: Generative Ai Insights
Success Stories and Tips
Amazon has seen a 34% increase in cross-selling and upselling after implementing Generative AI in data management to personalize the shopping experience for its customers.
Generative AI has also streamlined inventory management by predicting demand, making it easier for Amazon to manage its inventory.
Airbnb uses Generative AI in data management to study booking patterns and host behavior, which has increased host earnings by 23% through dynamic pricing strategies.
Explore further: Amazon Connect Generative Ai
Allstate has reduced claim processing time by 57% and increased fraud detection accuracy by 52% after adopting Generative AI in data management.
To successfully adopt Generative AI in data management, start by providing training sessions that cover the fundamentals of Generative AI in data management, including hands-on workshops that simulate real-world scenarios.
Integrating Generative AI tools gradually into workflows minimizes disruption and helps staff understand how Generative AI complements current roles.
Offering advanced AI and data analytics courses and providing access to learning platforms such as Coursera, Udacity, or in-house training resources encourages continuous learning and helps staff stay up-to-date with the latest developments in Generative AI.
Sharing tangible success stories and return on investment (ROI) from early projects is a powerful motivator and can help showcase the benefits of Generative AI in data management.
Here are some key statistics that demonstrate the impact of Generative AI in data management:
Advanced Features and Tools
Generative AI for data management offers a range of advanced features and tools that can significantly boost efficiency and accuracy. These features include data augmentation, which can increase data size by up to 10 times, as seen in the example of a company that used generative AI to augment their customer data.
Data augmentation can be particularly useful for machine learning models, which require large amounts of data to train effectively. By augmenting existing data, companies can create more robust models that are better equipped to handle real-world scenarios.
Generative AI can also be used to generate synthetic data, which can be used to test and validate machine learning models. This can be especially useful for companies that don't have access to large amounts of real-world data.
One of the key benefits of generative AI is its ability to reduce the need for manual data curation, which can be a time-consuming and labor-intensive process. By automating data curation, companies can free up resources and focus on more strategic activities.
Generative AI can also be used to identify and mitigate data biases, which can be a major issue in machine learning models. By analyzing and generating new data, generative AI can help companies create more fair and accurate models.
Recommended read: Create with Confidence Using Generative Ai
Sources
- https://www.denodo.com/en/solutions/by-technology/generative-ai
- https://dataforest.ai/blog/generative-ai-for-data-management-get-more-out-of-your-data
- https://www.getrightdata.com/resources/unleashing-data-potential-a-journey-through-large-language-models-and-gen-ai-in-data-management
- https://www.analytics8.com/blog/4-use-cases-for-generative-ai/
- https://www.datagalaxy.com/en/blog/utilizing-generative-ai-for-data-analytics/
Featured Images: pexels.com