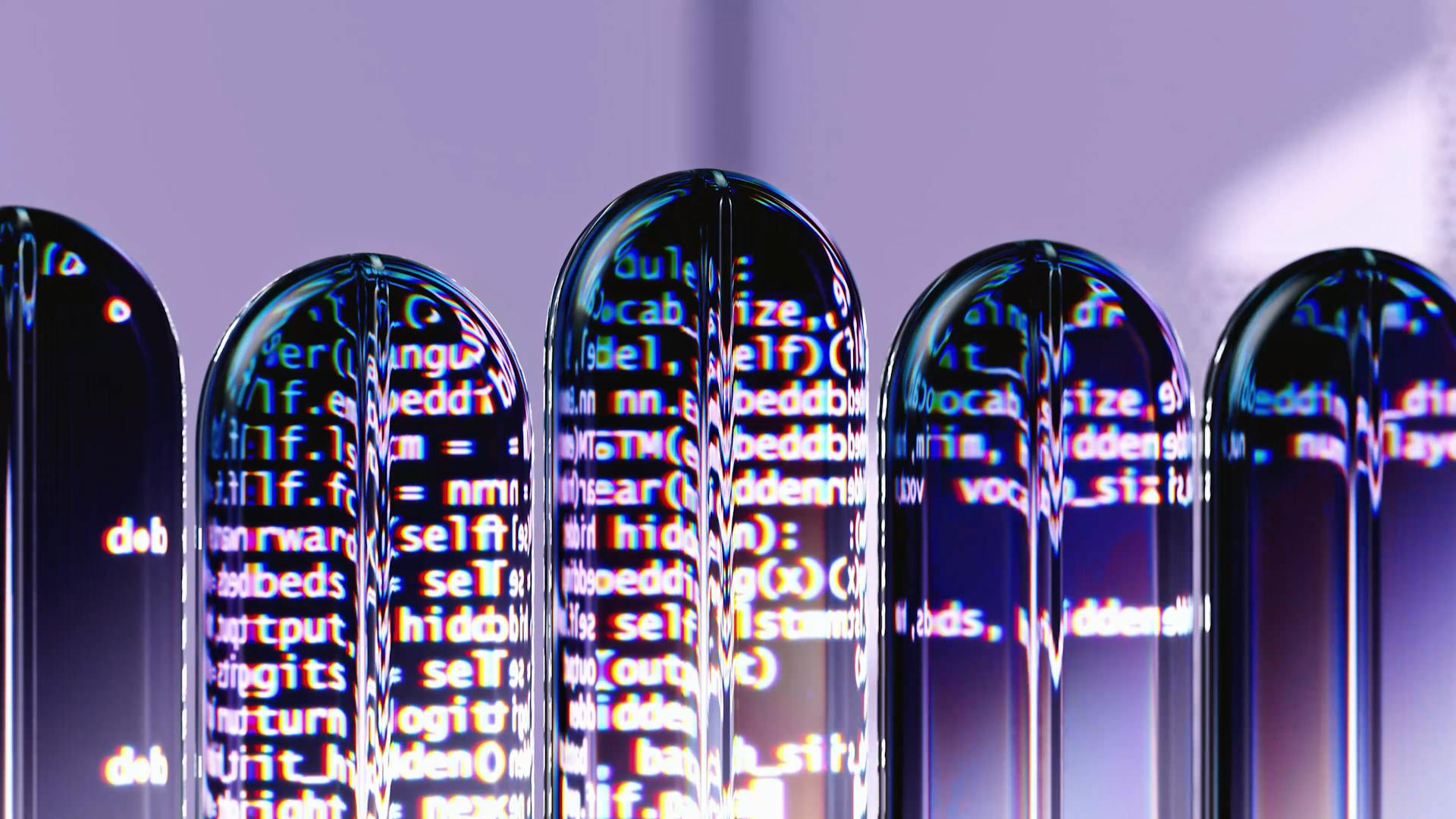
To get the most out of generative AI in research, it's essential to follow best practices and guidance. This involves understanding the limitations and potential biases of these tools.
Data quality is crucial when training generative AI models, as poor data can lead to inaccurate or misleading results. This can be seen in the example of a study that used a dataset with biased labels, resulting in a model that perpetuated these biases.
Generative AI models can be prone to overfitting, especially if they're not trained on a diverse enough dataset. This can be mitigated by using techniques such as regularization and cross-validation, as demonstrated in a study that achieved better results by implementing these methods.
Regularly evaluating and updating your generative AI models is also vital to ensure they remain accurate and relevant. This can be done by tracking their performance over time and retraining them on new data as needed.
Generative AI for Research
Using generative AI for research can be a powerful tool, but it's essential to be aware of its limitations. Generative AI tools are not the same as research databases, they're designed to generate their own content or output, unlike discovery platforms that point you directly to the source literature.
When searching for timely or current information, keep in mind that an AI tool will only be as good as the dates of coverage included in the data set it was trained on. This means that if the data set only goes up to 2020, the AI tool won't be able to provide information on events or developments that occurred after that date.
To get the most out of generative AI tools, it's crucial to be intentional about what you input into them. Avoid putting private or confidential information into these systems, as it may become part of the tool's dataset and resurface in response to other prompts.
For more insights, see: Generative Ai Market Research
Common Use Cases
Generative AI for Research is a game-changer, especially when it comes to ideation. AI can automatically generate research questions based on a given dataset or topic that can serve as starting points for researchers to refine and develop into hypotheses.
You can use tools like ChatGPT to generate topics for your research paper, and resources like "Writing Effective Text Prompts" by Sheridan College Library and Learning Services can help you brainstorm and narrow topics.
AI can accelerate the literature review process by analyzing and summarizing a body of literature on a topic, identifying relevant trends, patterns, and gaps in existing knowledge. Tools like Elicit, Consensus, and ResearchRabbit can help with this, but be aware that they may not have access to the same materials as your library and may require additional quality checking.
Generative AI can also aid in processing and analyzing large datasets, making it easier to identify emerging trends, correlations, outliers, and other patterns. You can use tools like Sage Research Methods to learn more about using large-language models for text analysis.
You might like: Guidance for Generative Ai in Education and Research
AI-driven recommendation systems can help researchers connect with peers, collaborators, and experts in their field, fostering interdisciplinary collaboration and knowledge sharing.
Here are some recommended resources to get you started:
Keep in mind that AI tools can reproduce biases inherent in the data sets they're trained on, so it's essential to be mindful of what you input into these systems and verify your results carefully.
Prompt Engineering for Research
Prompt Engineering for Research is a deliberate approach to crafting questions for AI tools to ensure helpful responses. This involves designing prompts that elicit specific and relevant information.
By intentionally structuring prompts, you can influence the quality of the output. Prompt Patterns are different structures you can use to engineer AI prompts.
One effective Prompt Pattern is the Persona approach, where you specify the role or identity of the person asking the question. This can significantly impact the response. For instance, asking an AI to imagine being an anthropologist can yield different keywords and terms than asking a more general question.
The Persona approach can lead to more targeted and relevant answers, as seen in the example where ChatGPT responded to a prompt imagining an anthropologist writing about social hierarchies.
For your interest: What Is a Prompt in Generative Ai
Best Practices
To get the most out of generative AI for research, you need to put in the effort to structure your prompts carefully. This means taking the time to think about what you want to achieve and crafting your questions or prompts accordingly.
The extent of your expectations should directly correspond with the degree of your efforts. By doing so, you'll be more likely to get the best possible outputs from your generative AI.
In other words, if you want high-quality results, you need to put in the work to create high-quality prompts. This might mean rephrasing or reorganizing your questions, or even trying out different approaches to see what works best.
A different take: Generative Ai Photoshop Increase Quality
Best Practices for 3rd Party Systems
Before using 3rd party generative AI systems, carefully consider the impact of using generative AI before entering data. This means thinking about how your input might be used, and whether it's okay to use a system that might reproduce biases or perpetuate misinformation.
Be mindful of what you input into these systems, as all content entered may become part of the tool's dataset and may inadvertently resurface in response to other prompts. Avoid putting private or confidential information into these systems.
To work with generative AI tools on assignments, consult the campus honor code, syllabus/assignment instructions, and/or check with your instructor. Check publisher websites for any guidelines provided about generative AI use and acknowledgement.
Some generative AI tools are open source, while others are commercial and capture information about users. These biases can be derived from the data sets used to train them, which can perpetuate harm and misinformation.
Generative AI tools are not the same as research databases - they're designed to 'generate' their own content or output, rather than pointing you directly to the source literature. This means you need to be cautious when using them, as the accuracy and relevance of the information they provide can vary.
Here are some key things to keep in mind when using 3rd party generative AI systems:
- Carefully consider the impact of using generative AI before entering data.
- Critically evaluate and corroborate information obtained from generative AI.
- Understand the expectations of disclosure requirements for using generative AI in academic work.
- Stay informed about conversations around AI technology and adhere to updated guidance from the university.
Getting the Best Possible Outputs
To get the best possible outputs from generative AI, you need to match the extent of your expectations with the degree of your efforts. This means putting in the time and effort to carefully structure and restructure your prompts.
Careful prompt design is key. By intentionally designing your prompts, you can ensure you get helpful responses from AI tools. This is known as Prompt Engineering.
Different structures can be used to engineer AI prompts. These are called Prompt Patterns. Using the right pattern can help you get the best possible outputs from your AI tools.
See what others are reading: What Are Generative Ai Tools
Frequently Asked Questions
What is the best AI for research?
For research purposes, ZAIA AI Assistant stands out as a top choice, offering a domain-specific LLM that helps researchers grasp key concepts and find relevant papers. Its integration with tools like Semantic Scholar and Paperguide further enhances its research capabilities.
Featured Images: pexels.com