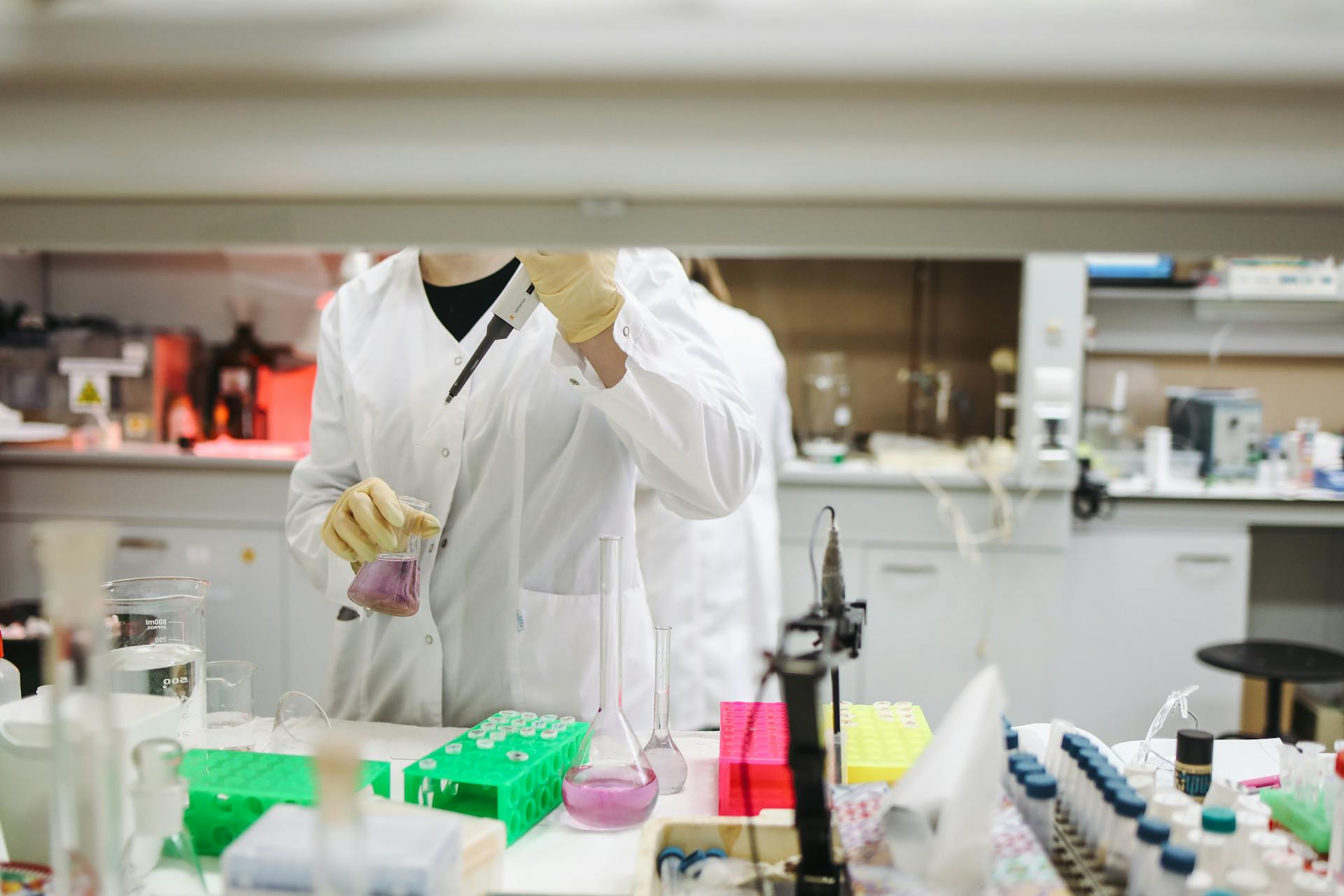
CRISPR technology has revolutionized the field of gene editing, allowing scientists to make precise changes to the DNA of living organisms. CRISPR stands for Clustered Regularly Interspaced Short Palindromic Repeats.
This technology has been widely adopted in research settings and has shown great promise in treating genetic diseases. In fact, CRISPR has been used to treat sickle cell anemia, a genetic disorder that affects hemoglobin production, in human patients.
The combination of CRISPR and generative AI is a game-changer in the field of gene editing. Generative AI can be used to predict the outcomes of CRISPR edits, allowing scientists to make more informed decisions about which edits to make. This collaboration has the potential to accelerate the discovery of new treatments for genetic diseases.
Worth a look: How Can Generative Ai Be Used in Cyber Security
Limitations and Optimisation
Traditional approaches to optimising CRISPR-Cas systems for gene editing have limitations. These include directed evolution, which can be limited by the complex nature of the 'fitness landscape', and structure-guided approaches, which depend on solved structures representing key functional states that are often difficult to obtain.
Directed evolution can be a slow and inefficient process, and structure-guided approaches require a deep understanding of the underlying biology. This is where AI comes in, offering a more efficient and scalable solution.
The CRISPR-Cas Atlas, an extensive dataset of over one million CRISPR operons, was compiled to overcome these limitations. This dataset was used to fine-tune ProGen2, a protein language model, to specialise in generating CRISPR-Cas proteins.
Addressing Limitations
Traditional approaches to optimising CRISPR-Cas systems for use in non-native environments can be limited by the need for explicit structural hypotheses or complex screening processes.
Directed evolution, for instance, can be limited by the complex nature of the 'fitness landscape', a way to visualise how different genetic variations perform.
Structure-guided approaches depend on solved structures representing key functional states that are often difficult to obtain for complex functions beyond simple binding interactions.
AI-Driven Design Optimisation
AI-driven design optimisation is revolutionising the field of gene editing. By leveraging large language models (LLMs) trained on vast amounts of biological data, researchers can generate novel CRISPR-Cas proteins that function as efficient gene editors in human cells.
The CRISPR-Cas Atlas, a dataset of over one million CRISPR operons from diverse microbial genomes, was compiled by mining 26 terabases of assembled genomes and metagenomes. This comprehensive dataset enabled the fine-tuning of ProGen2, a protein language model, to specialise in generating CRISPR-Cas proteins.
The researchers generated four million novel CRISPR-Cas protein sequences, with half generated directly and the other half prompted with short segments from natural proteins to guide generation towards specific families. This approach allowed for the generation of sequences across CRISPR-associated families as desired.
LLMs can analyse vast amounts of biological data, learning patterns and relationships that are not immediately obvious. This enables the generation of novel, functional CRISPR-Cas variants with high efficiency and accuracy, potentially uncovering designs that might be missed by traditional techniques.
On a similar theme: It Spend Million on Generative Ai
CRISPR Gene Editing
OpenCRISPR-1 is a functional, highly specific AI-designed gene editor that has been tested in human cells and shown to have comparable on-target editing efficiency to SpCas9.
This AI-designed editor was generated from scratch using a language model and has demonstrated a 95% reduction in off-target editing across multiple genomic sites tested.
The team that created OpenCRISPR-1 tested 209 Cas9-like proteins and found that many showed editing activity in human cells, with some performing on par with or better than SpCas9.
OpenCRISPR-1 has a high specificity, reminiscent of high-fidelity SpCas9 variants, and lacks immunodominant and subdominant T cell epitopes for HLA-A*02:01 that were previously identified in SpCas9.
This suggests a potentially low immunogenicity for the AI-designed editor, making it a promising tool for gene editing applications.
The OpenCRISPR-1 sequence has been publicly released to enable its broad usage across research applications and to further democratise gene editing.
Researchers are now exploring the potential of OpenCRISPR-1 as a base editor by converting the enzyme into a nickase and fusing it with adenine deaminases, including the established ABE8.20 and novel AI-generated deaminases.
These base editors have shown robust A-to-G conversion rates across multiple genomic loci, similar to those achieved with previously established base editor systems.
The team has also developed a sequence-to-sequence gRNA model to generate optimised single-guide RNAs (sgRNAs) for the AI-designed Cas proteins, which have shown enhanced editing efficiency for several AI-generated Cas9-like proteins.
What's Next
The field of generative AI gene editing with CRISPR is rapidly advancing, and we're just scratching the surface. According to Dr. Madani, the team is enthusiastic about refining OpenCRISPR-1, an AI-generated CRISPR enzyme, and exploring its potential in various settings.
Future studies will focus on expanding the capabilities of OpenCRISPR-1 to include other CRISPR-Cas proteins, such as Cas12 and Cas13, and exploring more diverse functionalities. This will enable the development of novel gene therapies with improved specificity and activity.
Prof Kaulich notes that the use of AI-generated gene-editing tools may raise ethical concerns and exacerbate inequalities if access to these technologies is limited to certain groups. Ensuring the safety, accuracy, and reliability of AI-generated tools is crucial, requiring thorough experimental validation and robust regulatory oversight.
Dr. Madani emphasizes that OpenCRISPR-1 is only the first milestone in a long journey to cure disease, and AI-designed gene editors will accelerate innovation in genetic medicines by lowering costs and barriers to entry. The team hopes to shift the drug development paradigm from accidental discovery to intentional and rapid design of bespoke gene-editing solutions.
For your interest: Generative Ai in Software Development
Evo, another AI system, can generate DNA sequences of over 1 million bases, larger than the genomes of many simple life forms. The team aims to scale Evo to more complex organisms and apply it to larger scales of biological organization.
The ultimate goal is to create a new field of 'genome design' where researchers can create entire cellular pathways and potentially entire organisms.
A unique perspective: Create with Confidence Using Generative Ai
AI Applications
AI applications are revolutionizing various industries, and one of the most exciting areas is gene editing with CRISPR.
CRISPR technology has been used to develop AI-powered tools for editing genes, allowing for more precise and efficient editing.
AI can analyze vast amounts of genetic data, identifying potential off-target effects and reducing the risk of unintended consequences.
Researchers are using AI to optimize CRISPR-Cas9 protocols, improving the accuracy and efficiency of gene editing.
AI-powered tools are also being used to design and predict the outcomes of gene editing experiments.
You might enjoy: What Are the Generative Ai Tools
AI can help scientists identify the most effective CRISPR guide RNAs for specific gene editing tasks.
AI is being used to develop new gene editing tools and techniques, pushing the boundaries of what is possible with CRISPR technology.
AI can also be used to analyze the results of gene editing experiments, providing valuable insights into the underlying biology.
AI is helping to accelerate the discovery of new gene editing applications, such as treating genetic diseases.
Discover more: Chatgpt Openai Generative Ai Chatbot Can Be Used for
Collaboration Drives Discovery
A team of over twenty scientists from various disciplines worked together to develop Evo, a generative AI tool for gene editing with CRISPR.
This interdisciplinary team included computational and biological experts, showcasing the power of diverse perspectives in driving innovation.
Eric Nguyen and Michael Poli led the machine learning subteam, focusing on architecture development and model training.
The computational biology subteam, led by Matthew Durrant, curated massive datasets of biological sequences and rigorously evaluated the model on downstream tasks.
Intensive biological experiments were performed by the experimental biology subteam, led by Brian Kang, David Li, and Dhruva Katrekar, to validate the complex designs generated by Evo.
The team's goal was to demonstrate capabilities that would spark imagination and collaboration among machine learning researchers, computational biologists, and experimentalists.
Hsu emphasized that Evo is a foundation model for biological research, enabling a general set of tasks across the central dogma of molecular biology.
Stanford Professor Tina Hernandez-Boussard and her team conducted an ethics and safety investigation to address potential risks and outline precautionary measures for the responsible development and deployment of Evo.
Frequently Asked Questions
What is generative AI in gene sequencing?
Generative AI in gene sequencing uses advanced algorithms to analyze and predict genetic sequences, enabling scientists to design new ones. This powerful tool is revolutionizing genomic research and discovery
How is CRISPR used for gene editing?
CRISPR/Cas9 edits genes by precisely cutting DNA and leveraging the body's natural repair processes to make specific changes. This precise editing allows for targeted modifications to genes, enabling breakthroughs in biomedical research and medicine.
Sources
- https://crisprmedicinenews.com/news/opencrispr-1-generative-ai-meets-crispr/
- https://arcinstitute.org/news/blog/evo-science
- https://newsroom.uw.edu/news-releases/ai-designed-protein-awakens-silenced-genes-one-one
- https://www.frontiersin.org/research-topics/67273/convergence-of-crispr-with-artificial-intelligence-ai-the-rise-of-intelligent-gene-editing-in-plants
- https://www.nyu.edu/about/news-publications/news/2023/july/ai-crispr-gene-expression.html
Featured Images: pexels.com