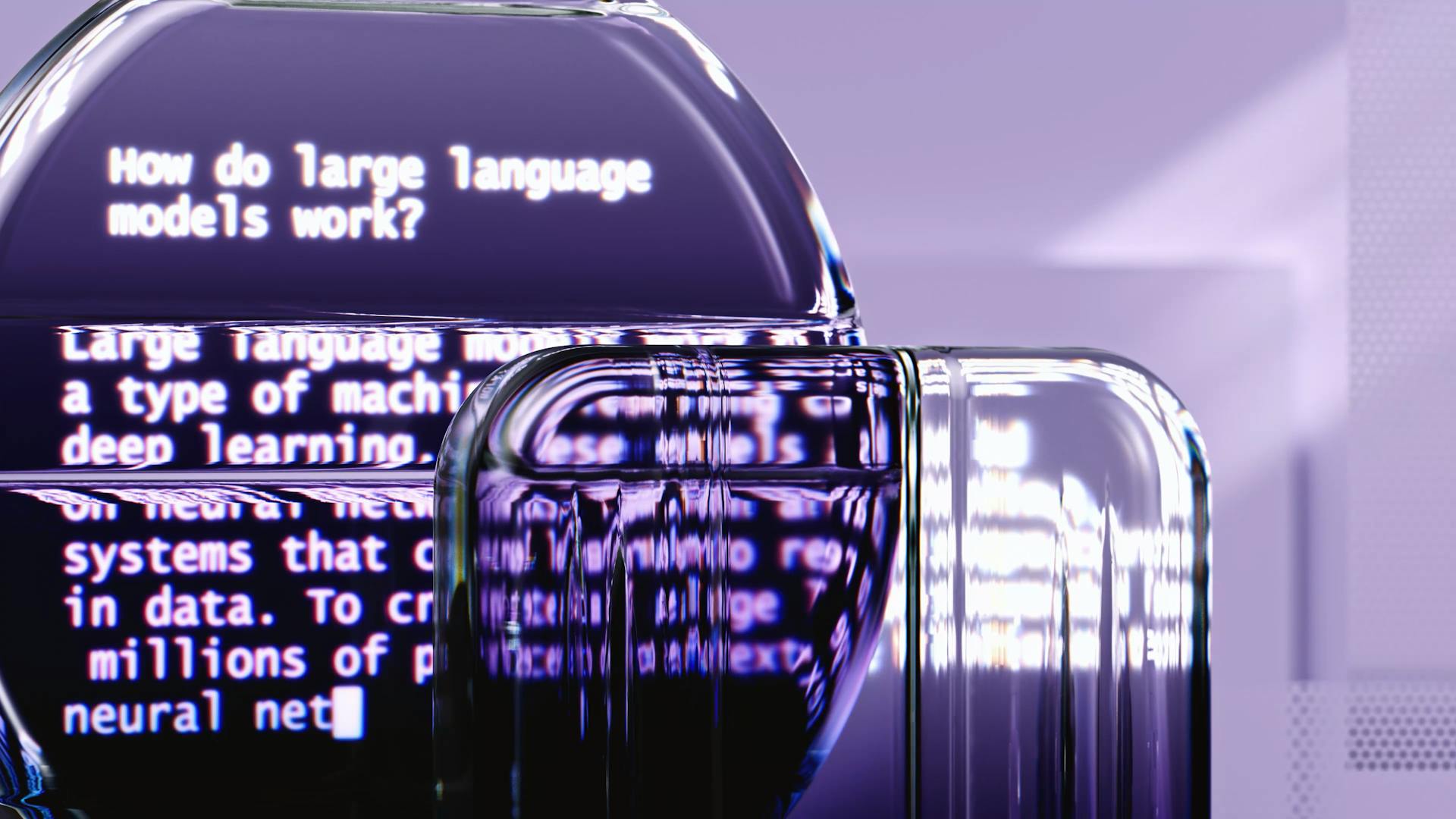
Generative AI is rapidly evolving, with applications in areas like art, music, and writing. The report highlights the potential of generative AI to revolutionize content creation.
According to the report, 75% of businesses are already using or planning to use generative AI. This technology has the potential to automate routine tasks, freeing up human creativity for more complex and high-value work.
As generative AI becomes more prevalent, it's essential to understand both its benefits and challenges. The report notes that 60% of users are concerned about the potential misuse of generative AI.
Take a look at this: Genrating Impression for Radiology Report Generative Ai
Why This Matters
Generative AI has reached a massive user base, exceeding 100 million users due to its enhanced capabilities and user interest.
This technology has the potential to dramatically increase productivity, transforming daily tasks across much of society.
Generative AI may also spread disinformation, posing substantial risks to national security and other domains.
For more insights, see: How Are Modern Generative Ai Systems Improving User Interaction
The Technology
Generative artificial intelligence (AI) is a technology that can create content, including text, images, audio, or video, when prompted by a user. It's trained on open-source information, such as text and images from the internet, using algorithms that learn patterns and relationships from massive amounts of data.
On a similar theme: Is Speech to Text Generative Ai
Generative AI has potential applications across various fields, including education, government, medicine, and law. This technology can quickly write a speech in a particular tone, summarize complex research, or assess legal documents.
Using prompts, generative AI systems can create artworks, including realistic images for video games, musical compositions, and poetic language, using only text prompts. It can also aid complex design processes, such as designing molecules for new drugs or generating programming codes.
Generative AI systems process and create content using sophisticated machine learning algorithms and statistical models. They learn to use many other data types, including programming codes, molecular structures, or images.
A user typically submits prompts that guide the generation of new content, and many iterations may be required to produce the intended result because generative AI is sensitive to the wording of prompts. Advanced chatbots, virtual assistants, and language translation tools are mature generative AI systems in widespread use.
Consider reading: Google Announces New Generative Ai Search Capabilities for Doctors
Opportunities
Generative AI has the potential to revolutionize various industries by automating tasks, improving productivity, and enhancing the customer experience. It can quickly aggregate and simplify information, making it easier for researchers to identify new chemical compounds for drug development. Generative AI can also automate administrative tasks, such as drafting legal templates, and improve customer support by creating nuanced automated responses.
Generative AI can be used to automate a wide range of tasks, including software development, customer operations, and retail management. In software engineering, generative AI can draft code based on context, automatically generate code tests, and review code to identify defects. This can accelerate the coding process and improve the quality of code.
Generative AI can also improve customer operations by providing digital self-service, enhancing and augmenting agent skills, and automating interactions with customers using natural language. This can increase issue resolution by 14 percent an hour and reduce the time spent handling an issue by 9 percent. Generative AI can also automate key functions in retail and CPG, such as customer service, marketing and sales, and inventory and supply chain management.
Here are some key opportunities for generative AI:
- Automating software development and customer operations tasks
- Improving code quality and accelerating the coding process
- Enhancing customer experience and agent productivity
- Automating key functions in retail and CPG
Struggling with Data
Over half of organizations are avoiding certain GenAI use cases because of data-related issues. These issues include data quality and accuracy, intellectual property, and usage rights.
Data deficiencies are a major roadblock for many organizations. They can't get started with GenAI because of it.
Intellectual property and usage rights are top concerns for organizations. They're worried about who owns the data and how it's being used.
Data quality and accuracy are also major issues. Organizations are struggling to find reliable data to train their GenAI models.
For another approach, see: Legal Implications of Ai
Software Engineering: Coding Assistant
Software engineering is a significant function in most companies, and it continues to grow as all large companies embed software in a wide array of products and services. Generative AI can significantly impact software engineering by speeding up the coding process.
Generative AI can be used in pair programming and augmented coding to train Large Language Models (LLMs) to develop applications that generate code when given a natural-language prompt describing what that code should do. This can reduce the time spent on certain activities, such as generating initial code drafts, code correction and refactoring, root-cause analysis, and generating new system designs.
For more insights, see: Generative Ai Code
According to our analysis, the direct impact of AI on the productivity of software engineering could range from 20 to 45 percent of current annual spending on the function. This value would arise primarily from reducing time spent on certain activities.
One study found that software developers using Microsoft’s GitHub Copilot completed tasks 56 percent faster than those not using the tool. An internal McKinsey empirical study of software engineering teams found those who were trained to use generative AI tools rapidly reduced the time needed to generate and refactor code—and engineers also reported a better work experience, citing improvements in happiness, flow, and fulfillment.
Generative AI tools are useful for software development in four broad categories. Here are some examples of how they can be applied:
- Draft code based on context via input code or natural language
- Automatically generate, prioritize, run, and review different code tests
- Optimize the integration and migration of legacy frameworks
- Review code to identify defects and inefficiencies in computing
Customer Interaction Pattern Reinvention
Consumers increasingly seek customization in everything from clothing and cosmetics to curated shopping experiences, personalized outreach, and food—and generative AI can improve that experience.
Generative AI can aggregate market data to test concepts, ideas, and models. Stitch Fix, which uses algorithms to suggest style choices to its customers, has experimented with DALL·E to visualize products based on customer preferences regarding color, fabric, and style.
Retailers can create applications that give shoppers a next-generation experience, creating a significant competitive advantage in an era when customers expect to have a single natural-language interface help them select products.
Generative AI can improve the process of choosing and ordering ingredients for a meal or preparing food—imagine a chatbot that could pull up the most popular tips from the comments attached to a recipe.
There is also a big opportunity to enhance customer value management by delivering personalized marketing campaigns through a chatbot. Such applications can have human-like conversations about products in ways that can increase customer satisfaction, traffic, and brand loyalty.
Generative AI offers retailers and CPG companies many opportunities to cross-sell and upsell, collect insights to improve product offerings, and increase their customer base, revenue opportunities, and overall marketing ROI.
Here are some examples of how generative AI can enhance customer interaction patterns:
- Customer self-service: Generative AI–fueled chatbots can give immediate and personalized responses to complex customer inquiries regardless of the language or location of the customer.
- Resolution during initial contact: Generative AI can instantly retrieve data a company has on a specific customer, which can help a human customer service representative more successfully answer questions and resolve issues during an initial interaction.
- Reduced response time: Generative AI can cut the time a human sales representative spends responding to a customer by providing assistance in real time and recommending next steps.
- Increased sales: Because of its ability to rapidly process data on customers and their browsing histories, the technology can identify product suggestions and deals tailored to customer preferences.
Factors for Retail and CPG Organizations
As retail and CPG organizations explore how to integrate generative AI in their operations, they should keep in mind several factors that could affect their ability to capture value from the technology.
Generative AI has increased the need to understand whether generated content is based on fact or inference, requiring a new level of quality control. This is a crucial consideration, especially in industries like retail and CPG where customer trust is paramount.
External inference is a key factor to consider, as it can impact the credibility of generated content. To mitigate this risk, companies will need to implement new quality checks for processes previously handled by humans.
Adversarial attacks are another concern, as foundation models are a prime target for attack by hackers and other bad actors. This increases the variety of potential security vulnerabilities and privacy risks that companies need to be aware of.
Companies will need to strategically keep humans in the loop to address these concerns and ensure that security and privacy are top considerations for any implementation. This may involve instituting new quality checks for AI-assisted processes such as product design.
Retail and CPG companies will need to perform more-detailed quality checks on AI-assisted processes to ensure that they meet the required standards. This may involve additional training for employees to ensure they can effectively review and validate AI-generated content.
Worth a look: How Has Generative Ai Affected Security
Challenges
Generative AI poses several challenges, including trust and oversight concerns. Generative AI systems can respond to harmful instructions, increasing the speed and scale of real-world harms. For example, they could help produce new chemical warfare compounds.
False information is another significant challenge. Generative AI tools may produce "hallucinations" - erroneous responses that seem credible. This can occur when a user requests information not in the training data, or when a user intentionally creates inaccurate or misleading text.
Economic issues, such as copyright concerns, are also a challenge. Generative AI systems can be trained on copyrighted, proprietary, or sensitive data without the owner's knowledge. There are unresolved questions about how copyright concepts, such as authorship, infringement, and fair use, will apply to content created or used by generative AI.
Here are some of the key challenges facing generative AI:
- Trust and oversight concerns
- False information
- Economic issues
- Privacy risks
- National security risks
Additionally, generative AI systems can pose privacy risks, including the inability to "forget" sensitive information and the potential for data to be used indefinitely without user knowledge.
High Costs
High costs associated with training data preparation can be a significant challenge for companies looking to implement generative AI technologies. Obtaining and preparing large diverse datasets can be expensive and time-consuming. Manual annotation, curation, and validation procedures are frequently needed to generate high-quality training data. These procedures require a substantial investment of time and knowledge from human resources.
Finding appropriate data that accurately reflects the desired results can be difficult and expensive, especially for specific or specialized purposes. For startups and smaller companies, these expensive data preparation requirements may serve as entry barriers, preventing them from fully utilizing generative AI technologies.
You might like: How to Learn Generative Ai
Navigating Obstacles
Navigating obstacles is crucial when it comes to Generative AI adoption. High costs associated with training data preparation can serve as entry barriers for startups and smaller companies. This is because obtaining and preparing large diverse datasets can be expensive and time-consuming.
Leaders report feeling ill-prepared on governance and risk as they work to address evolving challenges across accuracy, privacy, and security. The biggest barriers to GenAI adoption revolve around compliance, risk, and governance.
Consider reading: Generative Ai Governance
Organizations are increasingly basing decisions on risk, selecting effectively managing risks as the second-most reported way to drive the most value for GenAI initiatives. From a regulation standpoint, top preparatory actions include forecasting, monitoring, and working with external partners.
Here are some key challenges to consider:
- Trust and oversight concerns, such as ensuring accountability and responsible use of AI.
- False information, including "hallucinations" that seem credible but are actually erroneous responses.
- Economic issues, like copyright concerns and the potential for AI-generated content to infringe on existing rights.
- Privacy risks, including the potential for sensitive information to be stored and misused.
- National security risks, such as the potential for AI-generated content to be used for malicious purposes.
Frequently Asked Questions
How to generate AI report?
To generate an AI report, follow these 5 steps: define your topic, select a style and tone, craft a prompt, edit and customize, and download and share. Start creating your report today by following these simple steps.
What is generative AI summary?
Generative AI is a technology that creates various types of content, such as text, images, and audio. It uses artificial intelligence to produce original and unique content, revolutionizing the way we interact with technology
Is GPT a generative AI?
Yes, GPT is a generative AI that can create human-like text in various styles and formats. It uses this capability to transform and rewrite existing content into new, simplified, or rephrased versions.
Sources
- https://www.gao.gov/products/gao-23-106782
- https://www.mckinsey.com/capabilities/mckinsey-digital/our-insights/the-economic-potential-of-generative-ai-the-next-productivity-frontier
- https://www2.deloitte.com/us/en/pages/consulting/articles/state-of-generative-ai-in-enterprise.html
- https://www.marketsandmarkets.com/Market-Reports/generative-ai-market-142870584.html
- https://hginsights.com/blog/ai-market-report-generative-ai-industry-trends-insights
Featured Images: pexels.com