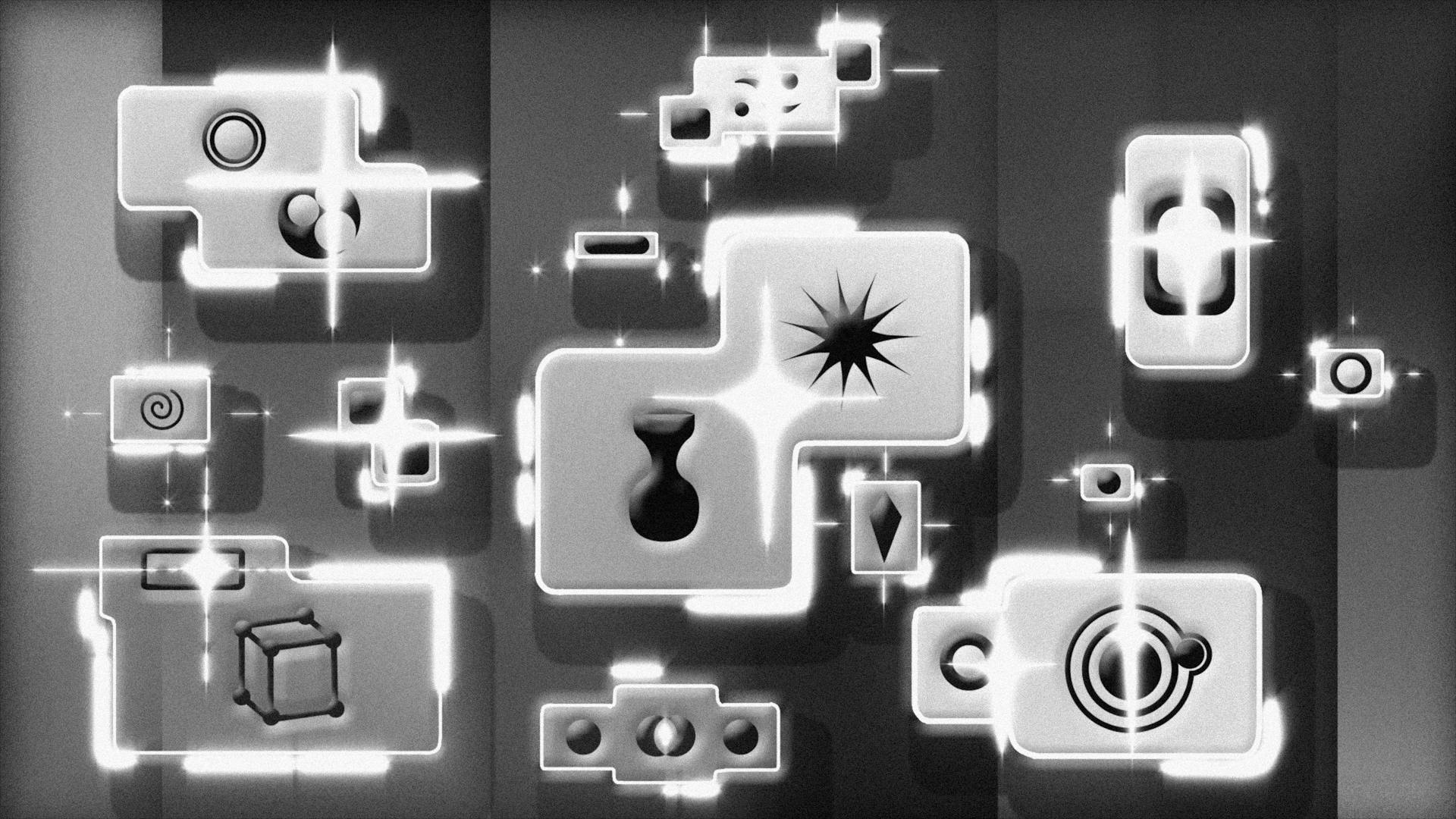
Generative AI response is a technology that can create new content based on a given prompt or example. This can include text, images, or even videos.
One of the key benefits of generative AI response is its ability to learn from large datasets and generate new content that is similar in style and tone. For example, it can create new articles, social media posts, or even entire books.
In the context of customer service, generative AI response can be used to generate personalized responses to customer inquiries, freeing up human customer support agents to focus on more complex issues. This can lead to faster response times and improved customer satisfaction.
Generative AI response is not just limited to text-based responses, it can also be used to generate visual content such as images and videos, allowing for a more immersive and engaging customer experience.
For your interest: Generative Ai for Customer Experience
What Is Generative AI?
Generative AI is a type of artificial intelligence that can create new content, such as written text, audio, images, or videos.
It's different from traditional AI, which follows predetermined rules or algorithms and can't learn from data. Generative AI, on the other hand, can learn from data and generate new data instances.
Generative AI uses machine learning techniques to learn from and create new data, which is a key difference from machine learning that enables a system to learn from data independently.
Conversational AI, which enables machines to understand and respond to human language, is also distinct from generative AI. While conversational AI is used to create interactive systems that can engage in human-like dialogue, generative AI is broader and encompasses the creation of various data types.
Generative AI is not equivalent to artificial general intelligence (AGI), which refers to highly autonomous systems that can outperform humans at most economically valuable work.
Explore further: Create with Confidence Using Generative Ai
How It Works
Generative AI uses neural networks to identify patterns in existing data to generate new content. It's like a super-smart computer that can learn from a large dataset and create new instances of content that mimic the properties of the input data.
A different take: Generative Ai Content
The process starts with data collection, where a large dataset is gathered that contains examples of the type of content to be generated. For example, a dataset of images for generating realistic pictures, or a dataset of text for generating coherent sentences.
Generative AI models use a type of machine learning called deep learning, which imitates the workings of the human brain in processing data and creating patterns for decision-making. Deep learning models use complex architectures known as artificial neural networks.
Here's a simplified overview of the generative AI workflow:
- Data collection: Gathering a large dataset of examples
- Model training: Using neural networks to learn patterns in the data
- Generation: Creating new content by sampling from the latent space or through a generator network
- Refinement: Further refining the generated content to improve its quality or meet specific requirements
Generative AI models can be trained using unsupervised and semi-supervised learning approaches, which allow organizations to create foundation models from large, unlabeled data sets. These foundation models can power tools like ChatGPT, DALL-E, and more.
Some examples of foundation models include LLMs, GANs, VAEs, and Multimodal, which are trained on large datasets to generate new content. For instance, ChatGPT draws data from GPT-3 to enable users to generate a story based on a prompt.
Related reading: Can I Generate Code Using Generative Ai
Types of Generative AI
Generative AI models are incredibly diverse, and understanding their types can help you harness their potential.
Transformer-based models, like GPT-3 and GPT-4, are designed for text generation and can consider the entire context of the input text, allowing them to generate highly coherent and contextually appropriate text.
Generative adversarial networks (GANs) consist of a generator and a discriminator, where the generator creates new data instances and the discriminator evaluates them for authenticity, engaging in a game-like process.
Variational autoencoders (VAEs) represent another type of generative model that leverages statistical inference, encoding input data into a latent space and decoding it to generate new data, introducing randomness to create diverse yet similar data instances.
Here are some of the most common types of generative AI models:
Types of
Generative AI models are diverse and each type has unique characteristics, making them suitable for different applications. One of the most common types of generative AI is transformer-based models, which are particularly good for text generation.
Transformer-based models, such as GPT-3 and GPT-4, use an architecture that allows them to consider the entire context of the input text, enabling them to generate highly coherent and contextually appropriate text.
Generative adversarial networks (GANs) are another type of generative AI model, consisting of a generator and a discriminator. The generator creates new data instances, while the discriminator evaluates these instances for authenticity, essentially engaging in a game to create highly realistic data instances.
GANs are particularly useful for creating synthetic data, which can be difficult or impractical to collect in real-world scenarios.
Variational autoencoders (VAEs) are a type of generative model that leverages statistical inference principles, encoding input data into a latent space and then decoding it to generate new data.
VAEs are useful for generating diverse yet similar data instances, making them suitable for applications such as image and video generation.
Other types of generative AI models include autoregressive models, which predict future data points based on previous ones, and normalizing flow models, which use a series of transformations to model complex data distributions.
Here are some examples of generative AI models and their characteristics:
Model Customization
Model customization is a crucial aspect of generative AI. It allows you to tailor the default behavior of foundation models to consistently generate desired results without using complex prompts.
You can customize these models through a process called model tuning, which helps reduce the cost and latency of your requests by simplifying your prompts. Model tuning is a key feature of Vertex AI, which also offers model evaluation tools to help you assess the performance of your tuned model.
Model tuning enables you to fine-tune your model to better suit your specific needs, making it more efficient and effective. This customization process is essential for real-world applications, where generative AI models need to perform unique tasks.
Here are the three key capabilities of generative AI models that need to be customized:
- Learn how to perform new tasks
- Access external information
- Block harmful content
By customizing these capabilities, you can ensure that your generative AI model generates content that's useful and safe for real-world applications.
Benefits and Opportunities
Generative AI can quickly automate a variety of tasks, making it a game-changer for industries looking to boost productivity. This technology has the potential to enhance the speed of administrative tasks and computer programming, although users may need to edit the generated result.
Researchers can use generative AI to identify new chemicals for drugs by analyzing established drugs. This is just one example of how generative AI can quickly aggregate and simplify complex information, making it easier to gather new knowledge.
By automating repetitive tasks, generative AI can help professionals focus on higher-level work, leading to improved productivity and efficiency. For instance, it can be used to draft legal templates, which can then be reviewed and completed by a lawyer.
If this caught your attention, see: Google Announces New Generative Ai Search Capabilities for Doctors
Why This Matters
Generative AI has exploded to over 100 million users due to enhanced capabilities and user interest.
This technology is transforming daily tasks across much of society, holding the potential to dramatically increase productivity.
Generative AI may also spread disinformation, presenting substantial risks to national security and other domains.
Opportunities
Generative AI can significantly speed up access to ideas and knowledge by rapidly aggregating a wide range of content and simplifying the search process.
For instance, researchers can identify a new chemical for a drug based on an AI-generated analysis of established drugs. This is a perfect example of how generative AI can help people gather new information more efficiently.
Generative AI can automate a wide variety of administrative or other repetitive tasks, freeing up time for more important tasks.
One way it can do this is by drafting legal templates, which can then be reviewed and completed by a lawyer. This can save a lot of time and effort in the long run.
Generative AI can also improve customer support by creating more nuanced automated responses to customer inquiries. This can lead to better customer satisfaction and reduced support costs.
By automating tasks and improving productivity, generative AI has the potential to enhance the speed of administrative tasks and computer programming.
A different take: New Generative Ai
Model Limitations and Overcoming Them
Generative AI models can produce outputs that sound convincing, but sometimes the information they generate is just plain wrong. This is because they're built on the biases of the internet and society, and can be manipulated to enable unethical or criminal activity.
To mitigate these risks, organizations should carefully select the initial data used to train these models to avoid including toxic or biased content. This means being intentional about the data they use and ensuring it's diverse and representative.
Using smaller, specialized models or customizing a general model based on their own data can also help minimize biases. And, it's essential to keep a human in the loop – having a real person check the output of a generative AI model before it's published or used.
Here are some potential limitations of generative AI models:
- Biased or incorrect output
- Manipulation to enable unethical or criminal activity
- Reputational and legal risks from publishing biased or copyrighted content
- Difficulty in controlling the output, leading to undesirable or irrelevant content
These limitations can be overcome by being mindful of the data used to train the models, using smaller or customized models, and implementing mechanisms to filter or check the generated content. By taking these steps, organizations can minimize the risks associated with generative AI and ensure its responsible use.
Use Cases and Examples
Generative AI has a wide range of use cases that can benefit various industries. Generative AI can produce human-like text responses, making chatbots more engaging and capable of maintaining natural and extended conversations.
In the realm of technology and communication, generative AI is used to produce human-like text responses. This makes virtual assistants much more sophisticated and helpful than previous generations of virtual assistant technology.
Generative AI is being used to create more interactive and engaging virtual assistants. These virtual assistants can understand and respond to user queries more effectively.
In the arts and entertainment sector, generative AI has been used to create unique pieces of art, compose music, and even generate scripts for movies. This has opened up new possibilities for creative professionals.
Generative AI can be used to generate design options and ideas to assist graphic designers in creating unique designs in less time. It can also be used by architects to generate unique and efficient floor plans based on relevant training data.
For your interest: Chatgpt Openai Generative Ai Chatbot Can Be Used for
Generative AI has also been used in science and medicine to design novel drug candidates, cutting the discovery phases to a matter of days instead of years. For medical imaging, GANs are now being used to generate synthetic brain MRI images for training AI.
Here are some examples of generative AI use cases across various sectors:
- Arts and entertainment: creating art, music, and scripts for movies
- Technology and communication: producing human-like text responses and creating interactive virtual assistants
- Design and architecture: generating design options and ideas for graphic designers and architects
- Science and medicine: designing novel drug candidates and generating synthetic brain MRI images
- E-commerce: creating hyper-realistic 3D models for advertising and producing personalized marketing content
Frequently Asked Questions
What will generative AI do?
Generative AI creates new, realistic content at scale, such as images, videos, music, and text, by learning from existing artifacts and producing novel output. It can generate a wide range of content types, from visual art to software code and product designs
Sources
- https://cloud.google.com/vertex-ai/generative-ai/docs/learn/overview
- https://www.mckinsey.com/featured-insights/mckinsey-explainers/what-is-generative-ai
- https://www.gao.gov/products/gao-23-106782
- https://www.coursera.org/articles/what-is-generative-ai
- https://www.sap.com/products/artificial-intelligence/what-is-generative-ai.html
Featured Images: pexels.com