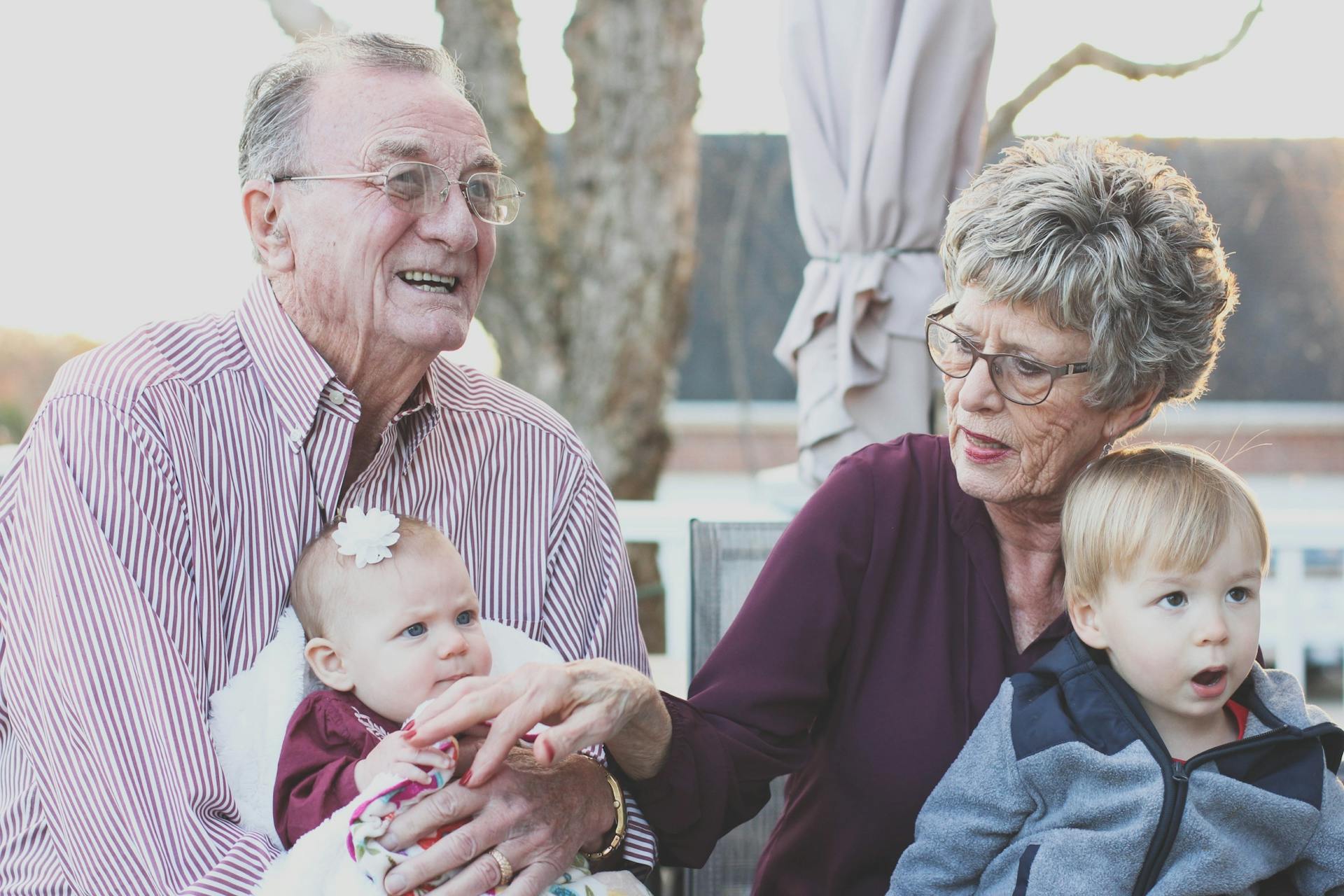
Non generative AI is a type of artificial intelligence that focuses on processing and understanding existing data, rather than generating new content.
It's primarily used for tasks like data analysis, natural language processing, and computer vision.
Non generative AI models are typically trained on large datasets and are designed to make predictions, classify objects, or recognize patterns within the data.
These models can be incredibly efficient, processing vast amounts of information in a matter of seconds.
Their applications are diverse, ranging from chatbots that can understand and respond to user queries to image recognition systems that can identify objects in real-time.
Intriguing read: Types of Ai Generative
Differences in Conversational Functionality
Conversational AI focuses on human conversations, while generative AI creates content in various forms. This fundamental difference in objectives sets the stage for distinct use cases and applications.
Conversational AI is used for applications like customer service, virtual assistants, and chatbots. These tools rely on human input and conversations to create responses. I recall using a virtual assistant to book a flight, and it was able to understand my queries and provide relevant information.
You might like: Conversational Ai vs Generative Ai
Here are the key differences in conversational functionality:
- Objectives: Conversational AI focuses on human conversations, while generative AI focuses on creating content.
- Use cases: Conversational AI is used for customer service, virtual assistants, and chatbots, whereas generative AI is used for writing works of fiction, marketing content, and meta descriptions.
- Learning and training data: Conversational AI is trained on large data sets with human input, conversations, user queries, and responses, whereas generative AI is trained on different sets of data to learn patterns and create content with predictive patterns.
- Input and output: Users input information in a conversation when using conversational AI, while generative AI uses input and data to generate new content by using learned patterns.
Classical vs Generative AI
Classical AI models are thriving and far from being obsolete, with classical AI models constituting more than half of total AI economic potential.
Generative AI, on the other hand, is designed to create something entirely new from scratch, like images, videos, text, and even human-sounding speech, using vast datasets and learned patterns.
Classical AI models focus on tasks such as classification, recognition, and prediction, like fraud detection in finance and healthcare, sentiment analysis for brand monitoring, and recommendation systems in e-commerce.
Generative AI models can be used for tasks like writing works of fiction, marketing content, and meta descriptions.
Some classical AI models can be used for tasks primarily associated with traditional AI, such as forecasting and recommender engines, but that doesn't make them non-generative.
Here's a breakdown of the key differences between classical and generative AI:
Is Generative AI Dead?
Generative AI has been around for a while, but it's not as dead as some might think. In fact, it's still a rapidly evolving field with many exciting developments.
According to the article, Generative AI has been used in various applications, such as text generation, image synthesis, and music composition. These capabilities have been demonstrated in the article section on "Text Generation with Large Language Models".
However, some concerns have been raised about the limitations of Generative AI, particularly in terms of its ability to generalize and adapt to new situations. This is highlighted in the article section on "Evaluation Metrics for Generative Models", where it's noted that current evaluation metrics may not be sufficient to capture the full range of generative AI capabilities.
Despite these limitations, Generative AI is still widely used in many industries, including education, marketing, and customer service. The article section on "Conversational AI in Customer Service" provides examples of how Generative AI is being used to improve customer experience and reduce support costs.
It's worth noting that Generative AI is not a single technology, but rather a collection of techniques and approaches that can be combined in different ways to achieve specific goals. This diversity of approaches is reflected in the article section on "Differences in Conversational Functionality", which highlights the various ways in which Generative AI can be used to support human-computer interaction.
Recommended read: Limitations of Generative Ai
Generative AI
Generative AI is a type of AI that can create new content, such as images, music, or text, based on a given prompt or input.
It's often used for tasks like generating new product designs, composing music, or even creating realistic-looking fake news articles.
Generative AI models use complex algorithms to analyze patterns in existing data and then generate new data that fits those patterns.
This process is often referred to as "deep learning", which involves training neural networks on large datasets to learn and improve over time.
Generative AI has many potential applications, including in art, music, and even healthcare, where it can be used to generate personalized treatment plans.
Check this out: Can I Generate Code Using Generative Ai Models
Alternative Techniques
Non generative AI techniques offer a range of alternatives to traditional generative AI methods. These alternatives can be more reliable and better understood, making them ideal for specific use cases.
Nongenerative Machine Learning is ideal for category predictions and regression tasks, such as customer segmentation, anomaly detection, and predictive maintenance. It's a powerful tool for getting insights from data.
Optimization is useful for planning tasks, such as inventory management, scheduling, and route planning, where it maximizes benefits by managing trade-offs. By using optimization, businesses can make informed decisions.
Simulation supports decision making by testing scenarios virtually, applicable in financial modeling, strategic analysis, and process simulations. It's a great way to explore different outcomes without risking real-world consequences.
Rules/Heuristics capture expert knowledge for decision making, suitable for fraud detection, loan approval, and medical diagnosis where explainability is crucial. These rules can be based on past experiences and industry best practices.
Graphs are better for tasks requiring explainability and precise data retrieval, such as recommendation systems and fraud detection. They provide a clear and structured way of representing complex data.
Alternative AI techniques can be summarized in the following table:
Sources
- https://www.techtarget.com/whatis/feature/Conversational-AI-vs-generative-AI-Whats-the-difference
- https://caylent.com/blog/differences-between-gen-ai-and-ai
- https://medium.com/@nonsibiventures/ai-series-part-1-is-non-generative-ai-dead-c5559fbcde29
- https://www.botkeeper.com/blog/not-all-artificial-intelligence-looks-like-chatgpt
- https://neurosys.com/blog/when-generative-ai-isnt-the-right-choice-for-your-business
Featured Images: pexels.com