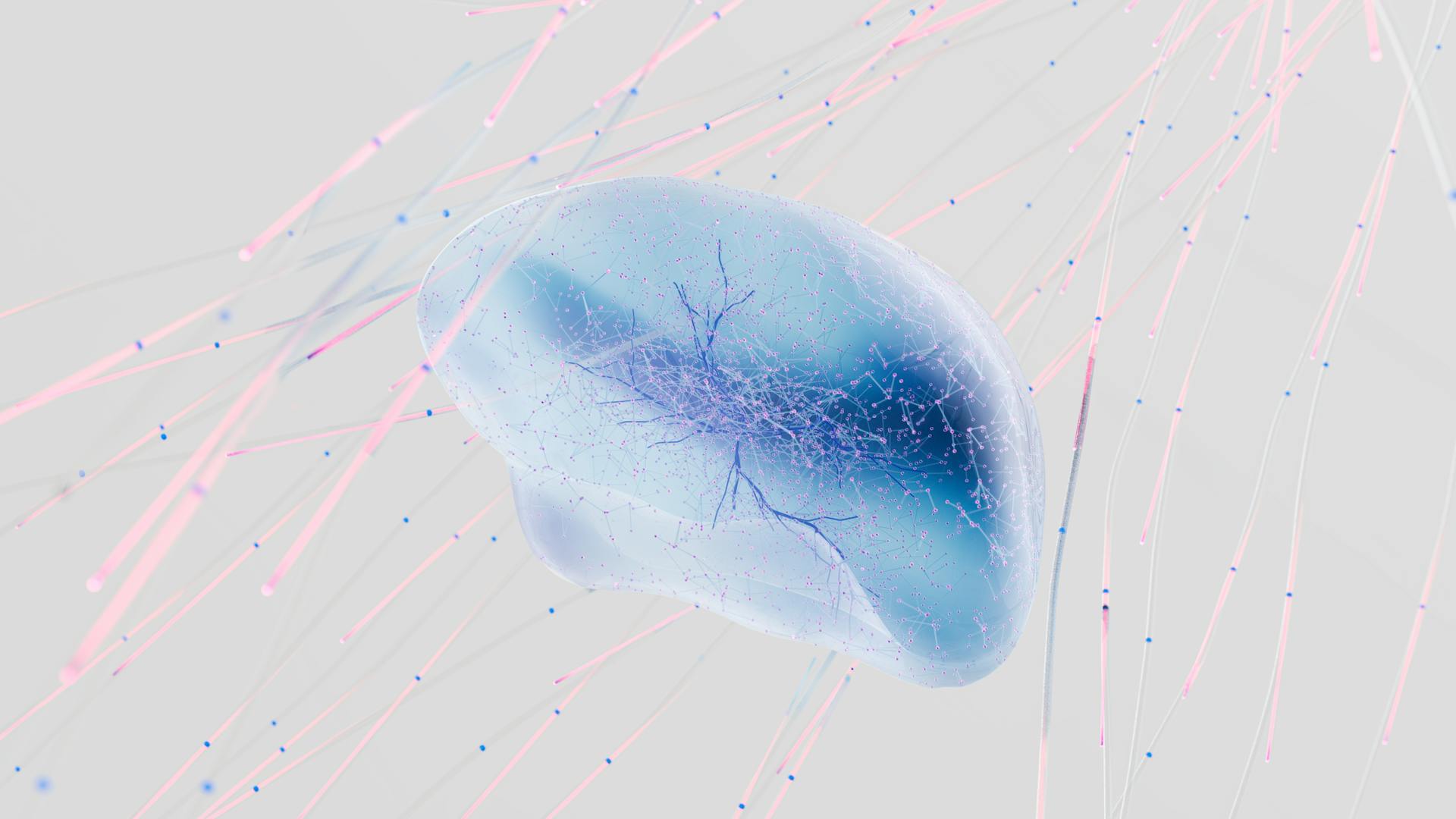
AutoML, or Automatización de Modelos de Aprendizaje, es una tecnología que permite a los usuarios no expertos en machine learning crear y entrenar modelos de aprendizaje automático sin necesidad de experiencia previa.
Esta tecnología se basa en algoritmos y técnicas de optimización que buscan encontrar el mejor modelo de aprendizaje automático para un problema específico, lo que reduce significativamente el tiempo y el esfuerzo requerido para entrenar modelos.
La implementación de AutoML en los negocios puede mejorar la eficiencia y la precisión de los modelos de aprendizaje automático, lo que a su vez puede llevar a decisiones mejor informadas y mayores ganancias.
What is AutoML
AutoML is a game-changer for organizations, making it easy to build, train, deploy, and serve custom machine learning models.
AutoML provides a simple experience to generate high quality models, making predictions, and testing business scenarios, allowing you to quickly apply machine learning across your organization.
This is especially great for both ML experts and citizen data scientists who can work with AutoML without needing to write code.
AutoML makes it possible to automate the machine learning process, making it more accessible and efficient for everyone involved.
Importance and Benefits
Automated machine learning, or AutoML, is a game-changer for businesses and individuals alike.
AutoML reduces the barrier of entry for non-data experts to participate in creating machine learning models.
It optimizes resources by saving time and effort in selecting and fine-tuning models and algorithms.
AutoML facilitates the management of large datasets and the implementation of models in a wide variety of applications.
This technology improves the speed of development and the quality of machine learning models generated.
Here are some key benefits of using AutoML:
- Reducción de la barrera de entrada: Permite a los no expertos en datos participar en la creación de modelos de aprendizaje automático.
- Optimización de recursos: Ahorra tiempo y esfuerzo en la selección y afinamiento de modelos y algoritmos.
- Escalabilidad: Facilita el manejo de grandes conjuntos de datos y la implementación de modelos en una amplia variedad de aplicaciones.
- Procesos eficientes: Mejora la velocidad de desarrollo y la calidad de los modelos de machine learning generados.
AutoML makes it faster and easier to give your analytics teams the power of predictive analytics, which can significantly improve business performance.
It allows non-ML-experts to leverage machine learning models and helps ML-experienced developers and data scientists to more quickly produce solutions.
By automating the machine learning process, AutoML reduces the risk of mistakes and improves the accuracy of machine learning models.
AutoML can be used on advanced artificial intelligence applications such as deep learning models, or simple problems in your business that you just don’t have the time or expertise to do.
Cómo Funciona
AutoML es un proceso de aprendizaje automático que se encarga de automatizar diversas etapas en la creación de modelos de aprendizaje automático.
La primera etapa del proceso de AutoML es la preparación de los datos, que incluye garantizar que el conjunto de datos esté correctamente etiquetado y formateado, y evitar la fuga de datos y el sesgo de entrenamiento-servicio.
Una vez preparados los datos, se puede entrenar el modelo, lo que implica la selección y optimización de técnicas y algoritmos adecuados para cada conjunto de datos.
Algunas de las técnicas empleadas en la entrenación del modelo incluyen la optimización de hiperparámetros, la limpieza de datos, la ingeniería de características y la búsqueda de arquitecturas neuronales.
Una vez entrenado el modelo, se puede evaluar su desempeño mediante la generación de informes de métricas, que incluyen métricas de predicción y regresión, como el error absoluto medio y la cuantil observado, y métricas de clasificación, como los resultados de predicción y el umbral de puntuación.
Para facilitar la interpretación de los resultados, AutoML a menudo incluye interfaces de usuario intuitivas que guían a los usuarios a través del flujo de trabajo del aprendizaje automático.
En resumen, el proceso de AutoML implica la automatización de diversas etapas en la creación de modelos de aprendizaje automático, desde la preparación de los datos hasta la selección y optimización de técnicas y algoritmos adecuados.
Getting Started
To get started with AutoML, you can download the AutoML guide with 5 factors for machine learning success.
AutoML is designed to be user-friendly, allowing you to interface with the models through a simple coding language like Python, even with minimal machine learning and deep learning knowledge.
Automated machine learning can be used for various tasks, including classification, regression, and prediction.
By using AutoML, you can tap into the power of neural architecture search, which facilitates the discovery of new architectures for complex problems.
Pretrained models can be adapted to new data sets through transfer learning, allowing AutoML to apply its knowledge to new tasks.
You can start exploring the capabilities of AutoML by learning how to use it, and the guide will provide you with a solid foundation to get started.
Types and Features
AutoML types can bring speed and transparency to your data science pipeline by automating several steps involved in building predictive models.
Even experienced data scientists and ML engineers must take multiple steps such as formulating hypotheses, collecting the right dataset, visualizing data, engineering features, training models with hyperparameters, and designing optimal deep neural network architectures.
AutoML tool features include end-to-end automation capabilities that allow you to quickly preprocess, clean, and connect your data.
Some popular AutoML tools provide a simple, code-free interface to easily auto-generate and refine ML models, make predictions, and test business scenarios.
Here are some key features of popular AutoML tools:
- Preprocessing, cleaning, and connecting data
- Auto-generating and refining ML models
- Making predictions and testing business scenarios
- Scoring and ranking multiple ML models
- Providing prediction-influencer data and full explainability
- Publishing data and integrating models via APIs
These features make it easier to build predictive models and get insights from your data.
Types
Building predictive models can be a daunting task, even for experienced data scientists and ML engineers. Experienced professionals must take several steps such as formulating hypotheses, collecting the right dataset, visualizing data, engineering features, training models with hyperparameters, and designing optimal deep neural network architectures.
AutoML types can bring speed and transparency to your data science pipeline. Automated machine learning can automate tasks such as model selection and hyperparameter tuning, freeing up time for more strategic work.
Experienced professionals often struggle with designing optimal deep neural network architectures. This can be a time-consuming and complex task that requires significant expertise.
Tool Features
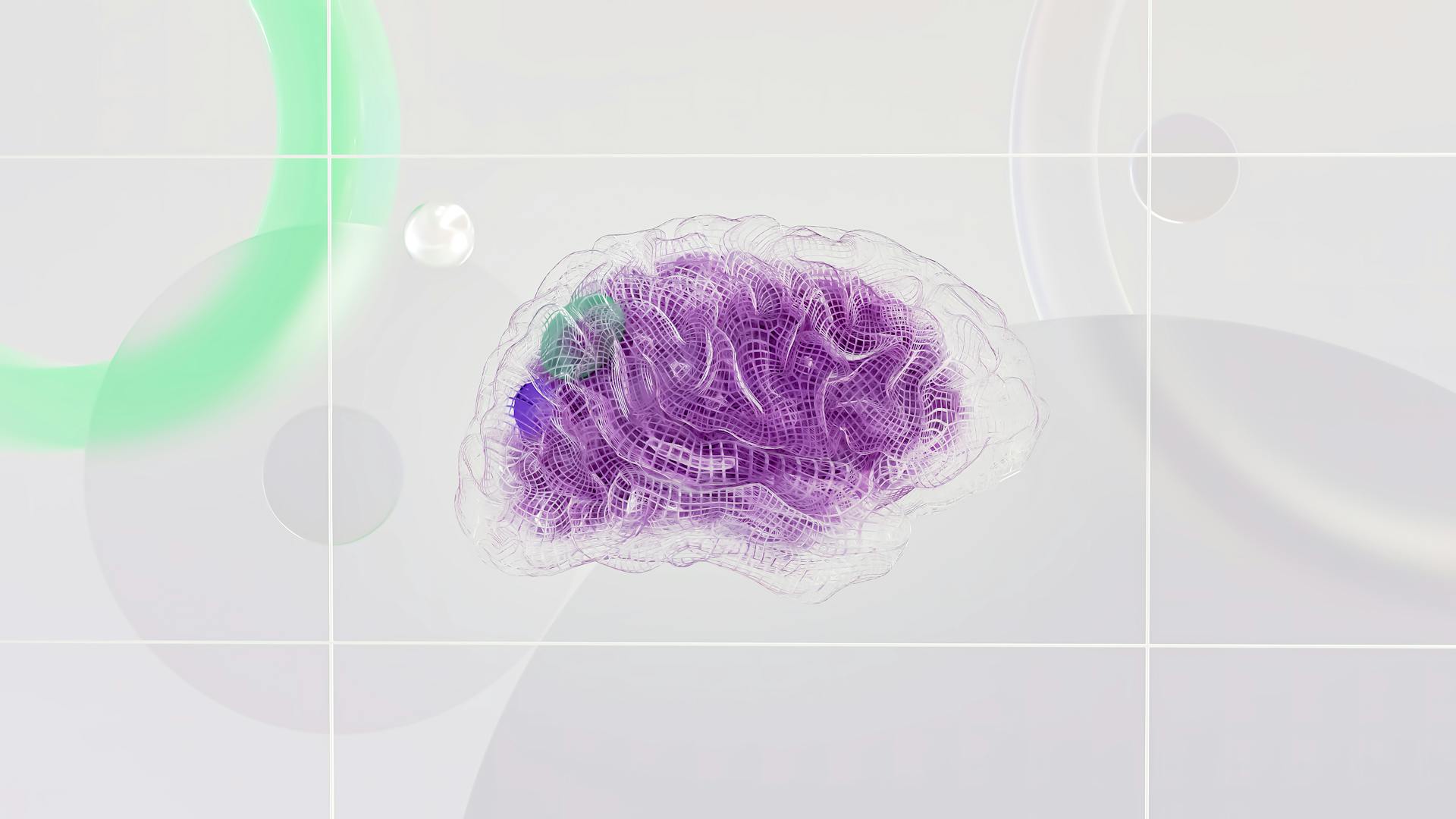
AutoML tool features are designed to make machine learning more accessible and efficient. They offer end-to-end automation capabilities that simplify the process of building and refining ML models.
Some popular AutoML tools, such as Vertex AI, AutoML Image, and AutoML Text, provide a suite of features to help you preprocess, clean, and connect your data. These tools also offer a simple, code-free interface to auto-generate and refine ML models, make predictions, and test business scenarios.
Here are some key features of AutoML tools:
- Quick data preprocessing, cleaning, and connection
- Code-free interface for auto-generating and refining ML models
- Automatic model scoring and ranking
- Prediction-influencer data and full explainability data
- Easy model publishing and integration via APIs
These features make it easier to develop high-quality ML models and integrate them into your workflow. By automating the process, you can save time and focus on more complex tasks.
Optimization of Hyperparameters
Automated hyperparameter optimization is a game-changer for machine learning.
Hyperparameters govern the behavior of your model, including settings like the learning rate, the number of hidden layers in a neural network, and the regularization strength. These parameters are usually configured before model training and can significantly influence your model’s performance.
Fine-tuning hyperparameters can be a challenging and time-intensive process, but AutoML systems automatically search for the optimal combination of hyperparameters for a specific ML model.
The impact of hyperparameter adjustments can be enormous, and in small-scale scientific data modeling, they can be easily set and optimized by trial and error.
Automated hyperparameter optimization allows data scientists to focus on the why of model creation, rather than the how. By automating this process, they can concentrate on what aspects of the model need to be optimized.
Feature Engineering
Feature engineering is a critical process that can significantly impact model performance. It involves transforming input data for machine learning models.
Creating a single feature can take hours, and achieving even basic accuracy requires hundreds of features. Manual feature engineering demands a major time investment.
Automated feature engineering (AFE) streamlines this process by automating feature space exploration. This reduces the time it takes to create features from many hours to just a few minutes.
Búsqueda de Redes Neuronales
Búsqueda de Redes Neuronales (NAS) es un proceso que automatiza el diseño de la arquitectura de las redes neuronales, lo que ahorra tiempo y esfuerzo a los equipos de data science.
El objetivo de NAS es seleccionar arquitecturas que se evalúen según métricas de rendimiento, como la precisión o la precisión de clasificación.
Una de las formas de NAS es el testing aleatorio para conjuntos de arquitecturas pequeñas, que puede ser rápido pero no muy efectivo.
Otras formas de NAS incluyen métodos basados en gradientes, que pueden ser más efectivos pero también más complejos.
Los equipos de data science pueden explorar algoritmos evolutivos, que evalúan arquitecturas aleatoriamente y mejoran iterativamente las que tienen buen rendimiento.
Machine Learning Basics
Machine learning is a process that uses algorithms to automatically select and optimize machine learning models. This process can be used to automatically select algorithms, pre-process data, and tune hyperparameters.
AutoML can speed up the machine learning process by reducing the need for manual intervention. It can also improve the accuracy of machine learning models by automatically selecting the best algorithms and hyperparameters.
Machine learning platforms and libraries make each step of the machine learning process easier. They can handle raw datasets and help build a practical machine learning model.
Comparison and Challenges
In a typical machine learning application, practitioners face significant hurdles, including challenging steps like data pre-processing, feature engineering, and algorithm selection.
Each of these steps can be tricky, making it difficult for non-experts to use machine learning techniques correctly and effectively.
AutoML aims to simplify these steps, making machine learning more accessible to a wider range of people.
AutoML plays an important role within the broader approach of automating data science, which includes challenging tasks like data engineering and model interpretation.
Comparison to Standard Approach
Using machine learning can be a daunting task, especially when you have to manually apply data pre-processing, feature engineering, feature extraction, and feature selection methods before even selecting an algorithm and optimizing its hyperparameters.
The standard approach requires a lot of expertise and can be time-consuming, resulting in significant hurdles to using machine learning correctly and effectively.
Each of these steps can be challenging, and if you're using deep learning, you also have to manually choose the architecture of the neural network, which can add to the complexity.
AutoML aims to simplify these steps and make it easier for non-experts to use machine learning techniques correctly and effectively by automating many of the tasks involved.
Challenges and Limitations
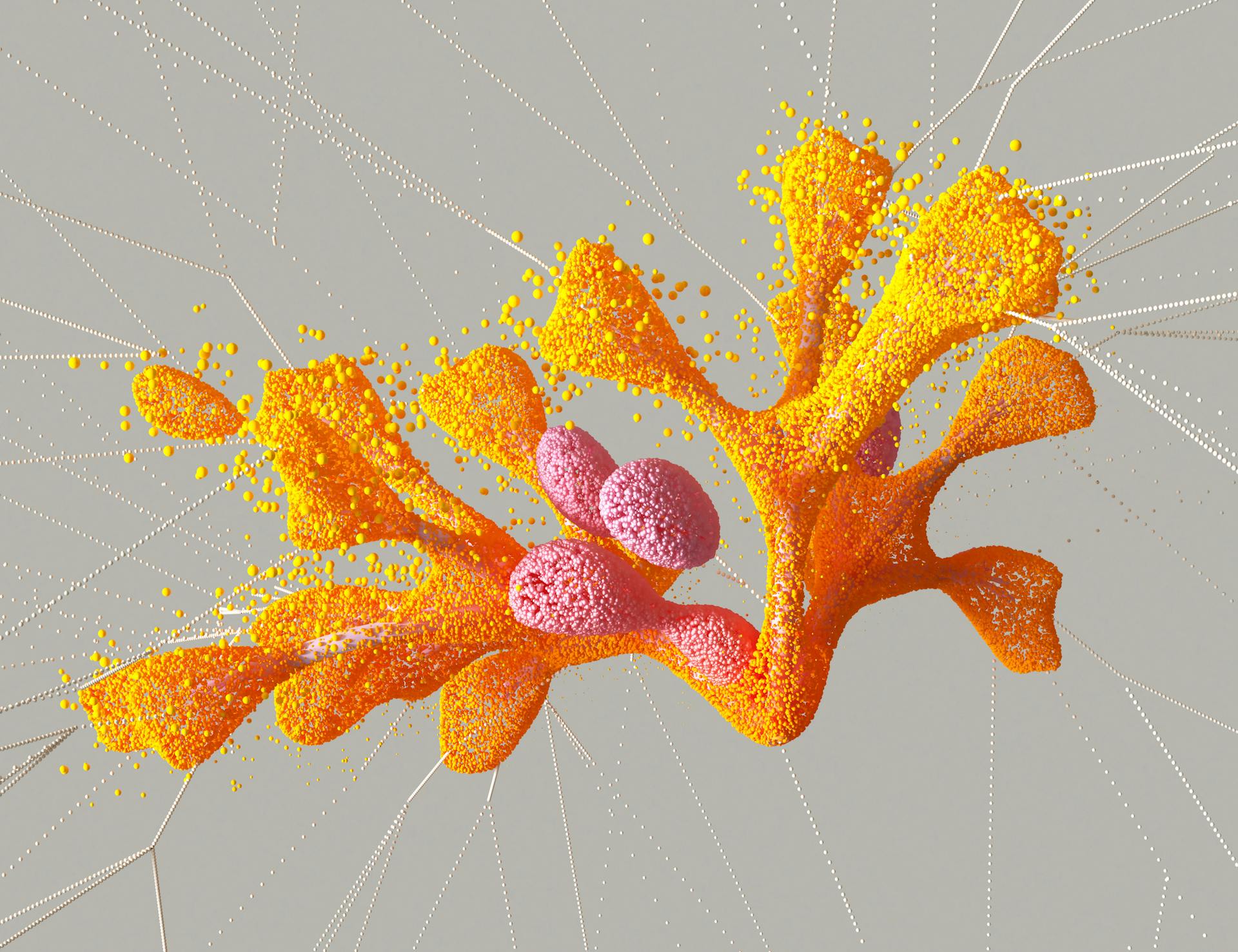
Automated machine learning faces a significant challenge known as "development as a cottage industry". This issue arises from relying on manual decisions and biases of experts, which contradicts the goal of creating systems that can learn and improve from their own usage and analysis of the data.
Experts and developers must help create and guide these machines to prepare them for their own learning, which requires labor-intensive work with knowledge of machine learning algorithms and system design.
Meta-learning challenges also pose a significant hurdle in automated machine learning, as it involves teaching machines to learn how to learn from other machines.
Computational resource allocation is another challenge that automated machine learning faces, as it requires significant processing power and memory to handle large amounts of data.
Featured Images: pexels.com