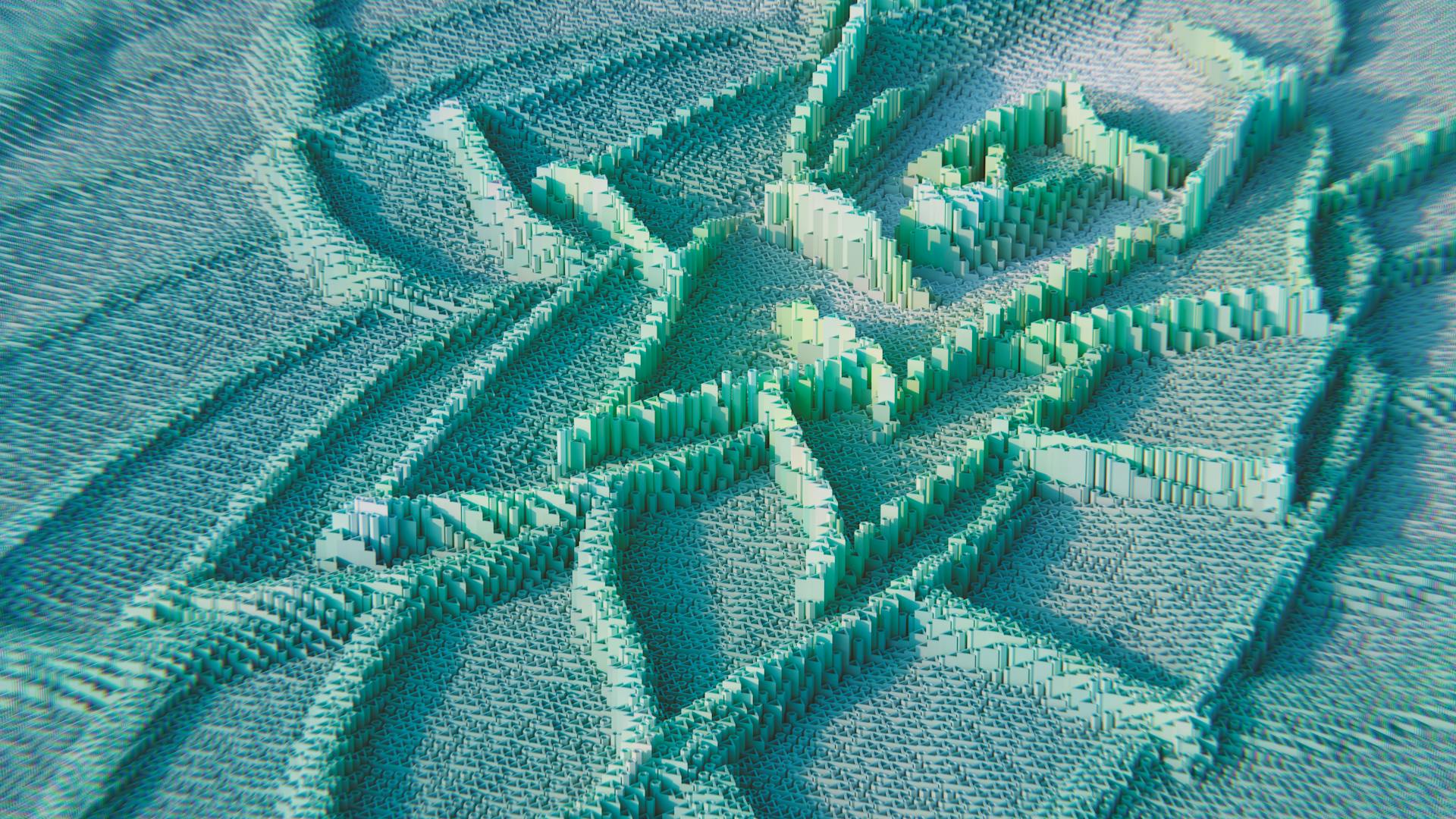
Generative AI is a powerful tool that can help you generate new ideas, improve your creativity, and even create new content. With generative AI, you can create realistic images, videos, and music that can be used for various purposes.
One of the most exciting applications of generative AI is in art and design. For example, you can use generative AI to create unique and personalized artwork, or to generate new designs for products and packaging.
Generative AI can also be used to improve your writing and communication skills. By using language models, you can generate new text, summarize long documents, and even create entire articles like this one.
What is Generative AI?
Generative AI, also known as GenAI, is a type of AI that allows users to input prompts to generate new content, such as text, images, videos, sounds, code, and 3D designs.
It learns and is trained on documents and artifacts that already exist online, which means it gets better with more data. This training process is complex and requires lots of computing power.
Generative AI operates on AI models and algorithms that are trained on large unlabeled data sets. These data sets help the AI predict outcomes in the same ways humans might act or create on their own.
The rise of generative AI is largely due to the fact that people can now use natural language to prompt AI, which has multiplied its use cases across different industries.
Tools and Applications
Generative AI tools exist for various modalities, such as text, imagery, music, code, and voices. Some popular AI content generators to explore include text generation tools like GPT, Jasper, AI-Writer, and Lex, and image generation tools like Dall-E 2, Midjourney, and Stable Diffusion.
Adobe Express has a generative AI tool that allows you to generate images from a text description, apply effects, and create designs. You can also use the NVIDIA AI Playground to generate landscapes, avatars, songs, and more.
Here are some examples of generative AI tools by modality:
- Text generation tools: GPT, Jasper, AI-Writer, Lex
- Image generation tools: Dall-E 2, Midjourney, Stable Diffusion
- Music generation tools: Amper, Dadabots, MuseNet
- Code generation tools: CodeStarter, Codex, GitHub Copilot, Tabnine
- Voice synthesis tools: Descript, Listnr, Podcast.ai
Tools
Generative AI tools are abundant and varied, with many requiring registration and possibly a cost. You can find more examples at Awesome Generative AI.
Adobe Express has a generative AI tool that allows you to generate images from a text description, apply effects, and create designs.
OpenAI's DALL.E 2 can generate images based on text input, edit existing images, and create variations of existing images. Consider any privacy implications before you upload photos.
Generative AI tools exist for various modalities, such as text, imagery, music, code, and voices. Some popular AI content generators to explore include:
- Text generation tools include GPT, Jasper, AI-Writer, and Lex.
- Image generation tools include DALL.E 2, Midjourney, and Stable Diffusion.
- Music generation tools include Amper, Dadabots, and MuseNet.
- Code generation tools include CodeStarter, Codex, GitHub Copilot, and Tabnine.
- Voice synthesis tools include Descript, Listnr, and Podcast.ai.
Microsoft's Copilot is based on Open AI's ChatGPT and can access the internet and read open webpages and documents. Make sure to always verify the sources.
Intriguing read: When Was Generative Ai Open Source
Model Development
Generative AI models combine various AI algorithms to represent and process content. This can include techniques such as natural language processing to transform raw characters into sentences and parts of speech.
Developers use encoding techniques to represent the world, which can also encode the biases and racism contained in the training data. This highlights the importance of careful data selection.
Neural networks like GANs and variational autoencoders (VAEs) are suitable for generating realistic human faces and synthetic data for AI training. These networks have a decoder and encoder, which enables them to create facsimiles of particular humans.
Recent progress in transformers has resulted in neural networks that can generate new content, such as language, images, and proteins. This is evident in models like Google's Bidirectional Encoder Representations from Transformers (BERT) and OpenAI's GPT.
Readers also liked: Generative Ai Transformers
How it Works
Generative AI starts with a prompt that could be in the form of a text, an image, a video, a design, musical notes, or any input that the AI system can process.
You can describe a request in plain language, and the AI will return new content in response. Early versions of generative AI required submitting data via an API or a complicated process, but now you can interact with it in a more intuitive way.
Developers used to need to familiarize themselves with special tools and write applications using languages like Python, but now pioneers in generative AI are making it easier to use.
Consider reading: Which Term Describes the Process of Using Generative Ai
What Sets Apart?
Generative AI has the ability to generate new data instances in various types, not just text.
This makes it useful for designing virtual assistants that generate human-like responses.
Generative AI can drive innovation and automate creative tasks.
It can also provide personalized customer experiences, transforming the way customers interact with technology.
Many businesses see generative AI as a powerful tool for creating content and solving complex problems.
It's already having a profound impact on business applications, making it a valuable asset for companies looking to stay ahead.
Expand your knowledge: Can I Generate Code Using Generative Ai Models
How Does Work?
Generative AI models use neural networks to identify patterns and structures within existing data to generate new and original content.
Neural networks are trained on large amounts of data, which enables them to learn and recognize patterns that humans might miss. This allows them to generate content that's not only new but also coherent and meaningful.
Foundation models, like GPT-3 and Stable Diffusion, are trained using different learning approaches, including unsupervised or semi-supervised learning. This gives organizations the ability to leverage large amounts of unlabeled data to create these models.
These foundation models can be used as a base for AI systems that can perform multiple tasks, making them incredibly versatile and powerful tools.
See what others are reading: Generative Ai Content
Neural Networks Transform
Neural networks are the backbone of generative AI, and they've come a long way since the 1950s and 1960s when they were first developed.
These early neural networks were limited by a lack of computational power and small data sets, making them impractical for generating content.
It wasn't until the advent of big data in the mid-2000s and improvements in computer hardware that neural networks became practical for generating content.
The field accelerated when researchers found a way to run neural networks in parallel across graphics processing units (GPUs), which were being used in the computer gaming industry to render video games.
Neural networks can now "learn" the rules from finding patterns in existing data sets, allowing them to generate new and original content.
This shift from rule-based systems to neural networks has enabled the development of foundation models like GPT-3 and Stable Diffusion, which can be used to generate a wide range of content, from text to images.
These foundation models have opened up new possibilities for applications like ChatGPT, which can generate essays based on a short text request, and Stable Diffusion, which can generate photorealistic images given a text input.
Broaden your view: Telltale Words Identify Generative Ai Text
The Benefits of
Generative AI can automate the manual process of writing content, reducing the effort required to create high-quality content.
With generative AI, you can create realistic representations of people, improving the response to specific technical queries.
Generative AI can also help improve the efficiency and accuracy of existing AI systems, such as natural language processing and computer vision.
This is achieved by creating synthetic data that can be used to train and evaluate other AI algorithms.
Generative AI can explore and analyze complex data in new ways, uncovering hidden patterns and trends that may not be apparent from the raw data alone.
By doing so, businesses and researchers can gain valuable insights and make more informed decisions.
Here are some specific benefits of generative AI:
- Automating the manual process of writing content
- Reducing the effort of responding to emails
- Improving the response to specific technical queries
- Creating realistic representations of people
- Summarizing complex information into a coherent narrative
Generative AI can also help improve the response to customer service and technical support queries, making it a valuable tool for businesses looking to enhance their customer experience.
Related reading: Generative Ai Customer Support
Popular Generators and Tools
Generative AI tools are incredibly diverse, and you can find them for various modalities like text, imagery, music, code, and voices. Most of these tools require you to register to use them, may have a cost, and free plans may have limits.
You can explore different types of generative AI tools, including text generation, image generation, music generation, code generation, and voice synthesis. Some popular AI content generators to explore include GPT, Jasper, AI-Writer, and Lex for text generation.
Let's take a look at some specific examples of generative AI tools. For text generation, you can use GPT, Jasper, AI-Writer, and Lex. For image generation, DALL-E 2, Midjourney, and Stable Diffusion are great options.
You may also want to check out some popular AI generators that can help automate certain processes in your workflow. ChatGPT is a helpful companion for research, strategy, and content creation, while DALL-E2 generates images from text prompts.
For your interest: Introduction to Generative Ai with Gpt
Here are some examples of popular generative AI platforms:
These are just a few examples of the many generative AI tools and platforms available. Be sure to check the usage policies or conditions of any tools before you start using them and review the terms regularly.
Explore further: Top Generative Ai Tools
History and Future
Generative AI has come a long way since the 1960s, when Joseph Weizenbaum created the Eliza chatbot, one of the earliest examples of generative AI. This early implementation used a rules-based approach that broke easily due to its limited vocabulary and lack of context.
The field saw a resurgence in 2010 with advances in neural networks and deep learning, enabling the technology to automatically learn to parse existing text, classify image elements, and transcribe audio. Ian Goodfellow introduced GANs in 2014, providing a novel approach for organizing competing neural networks to generate and rate content variations.
In the future, generative AI will continue to evolve, making advancements in translation, drug discovery, anomaly detection, and the generation of new content, from text and video to fashion design and music. Generative AI will seamlessly integrate into our workflows, making tools like grammar checkers and design tools more effective.
Intriguing read: Generative Ai Boosting Approach
History
The history of generative AI is a fascinating story that spans several decades. In the 1960s, Joseph Weizenbaum created the Eliza chatbot, one of the earliest examples of generative AI.
Early chatbots were limited by their rules-based approach, which broke easily due to a lack of context and overreliance on patterns. This led to the development of more advanced techniques, including neural networks and deep learning.
The 1980s saw the introduction of simple generative models like the Naive Bayes classifier, which marked the beginning of a generative approach to AI. Later in the decade, Hopfield Networks and Boltzmann machines were developed to create neural networks capable of generating new data.
However, scaling up to large datasets was difficult, and issues like the vanishing gradient problem made it hard to train deep networks. This challenge was finally overcome in 2006 with the introduction of the Restricted Boltzmann Machine (RBM).
The RBM solved the vanishing gradient problem, making it possible to pre-train layers in a deep neural network. This breakthrough led to the development of deep belief networks, one of the earliest deep generative models.
Discover more: Generative Ai in Software Development
The 2010s saw a resurgence in generative AI, thanks to advances in neural networks and deep learning. This led to the introduction of techniques like GANs, VAEs, and transformers, which have revolutionized the field and enabled the creation of realistic deepfakes.
Today, generative AI is a vibrant field with active research and diverse applications. The technology continues to evolve, with newer models like GPT-4 and DALL-E pushing the boundaries of what AI can generate.
The Future of
Generative AI has taken the world by storm, with tools like ChatGPT, Midjourney, Stable Diffusion, and Gemini leading the charge.
As these tools become more widespread, we're seeing a surge in training courses to help developers and business users alike harness their potential.
Grammar checkers will get better, and design tools will seamlessly embed more useful recommendations into our workflows, making our lives easier and more efficient.
The impact of generative AI will be felt across industries, with advancements in translation, drug discovery, anomaly detection, and the generation of new content, from text and video to fashion design and music.
Take a look at this: How Generative Ai Will Transform Knowledge Work
As we continue to integrate these capabilities into our tools, we'll find ourselves reevaluating the nature and value of human expertise.
Industry and society will build better tools for tracking the provenance of information, creating more trustworthy AI.
Generative AI will change the way we work, making tasks more automated and augmenting human capabilities.
On a similar theme: Generative Ai Human Creativity and Art
Evaluating and Getting Started
To get started with generative AI, you need to understand what makes a good model. Quality is key, especially for applications that interact directly with users. Poor speech quality, for instance, can be difficult to understand.
A good generative model should be able to capture minority modes in its data distribution without sacrificing generation quality. This helps reduce undesired biases in the learned models.
Speed is also crucial for many interactive applications. Real-time image editing, for example, requires fast generation to allow use in content creation workflows.
To evaluate generative AI models, consider these key factors:
Comparison and Best Practices
Generative AI can be a powerful tool, but it's essential to use it responsibly. Clearly label all generative AI content for users and consumers.
To ensure accuracy, vet the generated content using primary sources where applicable. Double-check the quality of AI-generated code and content using other tools. This will help you identify potential biases and errors.
To get the most out of generative AI, learn its strengths and limitations, and familiarize yourself with common failure modes in results. By understanding these factors, you can work around them and achieve your desired goals.
Best Practices
As you start working with generative AI, it's essential to consider accuracy, transparency, and ease of use. Clearly label all generative AI content for users and consumers.
To ensure accuracy, vet the generated content using primary sources where applicable. This will help you verify the information and reduce the risk of spreading misinformation.
Bias is another crucial factor to consider. Generative AI models can inherit biases from the data they're trained on, so it's essential to be aware of how bias might get woven into generated results.
Worth a look: Generative Ai for Content Creation
To evaluate the quality of generative AI models, consider the following key factors:
To get the most out of generative AI tools, learn their strengths and limitations. This will help you work around common failure modes and ensure you're using the right tool for the job.
Lastly, don't forget to double-check the quality of AI-generated code and content using other tools. This will help you catch any errors or inconsistencies that might have slipped through the cracks.
A fresh viewpoint: What Are the Generative Ai Tools
vs
Generative AI and traditional AI have distinct approaches to processing data and producing results. Generative AI relies on neural network techniques such as transformers, GANs, and VAEs to create new and original content.
One key difference between the two is that generative AI often starts with a prompt, allowing users to submit a starting query or data set to guide content generation. This can be an iterative process to explore content variations.
Generative AI is well-suited for tasks involving NLP and calling for the creation of new content. Traditional algorithms, on the other hand, are more effective for tasks involving rule-based processing and predetermined outcomes.
Additional reading: Google Announces New Generative Ai Search Capabilities for Doctors
Generative AI models can encode biases, racism, deception, and puffery contained in the training data. This is a caution developers should consider when using these techniques.
Techniques such as GANs and VAEs are suitable for generating realistic human faces, synthetic data for AI training, or even facsimiles of particular humans.
Take a look at this: Learn Generative Ai
Sources
- https://uq.pressbooks.pub/digital-essentials-artificial-intelligence/chapter/generative-ai-tools-and-examples/
- https://www.nvidia.com/en-us/glossary/generative-ai/
- https://www.techtarget.com/searchenterpriseai/definition/generative-AI
- https://www.coursera.org/articles/what-is-generative-ai
- https://www.sap.com/products/artificial-intelligence/what-is-generative-ai.html
Featured Images: pexels.com